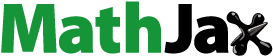
Abstract
As the global ambition is directed at net-zero 2050 amidst energy intensity-efficiency targets, the advanced economies, such as the United States of America (USA) has been consistently charged with more target-driven commitments. Considering this, the current study finds the influence of commercial, industrial, and household energy intensities on both the economic and environmental indicators. A set of cointegration approaches was employed to evaluate the long-run and short-run relationship between covariates and carbon emission over the period 1974–2019. Empirical findings reveal that all the covariates are positive and significantly related to carbon emissions. For instance, the emission of carbon dioxide is worsened by economic growth in both the short- and long-run. Additionally, intense use of energy across the commercial, household, and industrial sectors is responsible for an increase in environmental degradation arising from the emission of carbon emission. Importantly, environmental degradation that is attributed to energy intensity is far more (twice) in the commercial sector and household sector, than in the industrial sector. Regarding the economic aspects, there is statistical evidence that research and development expenditure in energy efficiency improves economic growth while higher energy intensities in the commercial and industrial sectors are detrimental to economic expansion. As a policy, the study suggests that the share of renewable or clean energy technology in the country’s energy mix should be significantly increased to over-turn the undesirable economic, environmental, and global warming-related issues in the United States. Other few directions for policy implication were addressed.
Introduction
Energy intensity is determined by the amount of energy required per unit yield or preferably per economic output, i.e. Gross Domestic Product (GDP). In essence, utilizing less energy to deliver both economic and non-economic output is desirable, especially from the perspective of energy and environmental sustainability. The intensity of energy use measures the energy productivity of a specific economy, such as joules (or Btu) per US dollar. Thus, the energy power proportion of a simple electrical device is modest when there is a low energy requirement. This implies that low energy power is the ideal global objective, particularly in the era of carbon neutrality discussion. Although efforts show a consistent reduction in energy intensity through innovative approaches and governments’ policy interventions globally, the total level of energy intensity and its environmental consequences are yet to show significant improvement over time [Citation1]. For instance, the United States (US) Energy Information Administration (EIA) expects an annual growth of 1.5% in energy use for business activities globally, even in non-industrial economies [Citation2].
As a major contributor to energy consumption and environmental issues, household energy is a major part of energy consumption, and the level of its intensity will continue to influence the level of environmental emissions, such as greenhouse gas (GHG). As stated by the US energy data, more than half of US household energy consumption, i.e. about 51% is associated with space heating and conditioning [Citation2]. However, the amount of energy consumed in households largely depends on geographic location; type of home, the efficiency of energy-consuming devices in the home, and the number of household members. Given that economic activities and inefficient use of energy resources potentially result to increase in energy intensity and emission of GHG, consistently improving the efficiency of energy technologies as well as energy affordability is not negotiable [Citation3]. From the global perspective, while total energy intensity consistently declined between 1990 and 2012, the metric tons per capita of carbon emissions either remained the same or increased slightly year in and out (). Interestingly, the scenario is almost the same for the USA and the globe, i.e. the USA scenario is shown in . The amount of carbon dioxide (CO2) emissions from the United States of America (USA)’s commercial, industrial, and household sectors may differ depending on the level of energy intensity in these sectors.Footnote1
In line with the motivation, this study aims at investigating and finding the relevance of commercial, industry, and household energy intensities on carbon emission in the United States. In a unique approach, largely different from perspectives in the extant literature, the current study further examines the economic dimensions of the energy intensities in the named sectors amidst research and development (R&D) expenditure in energy efficiency. Thus, the environmental quality and economic aspects of the intensity of energy consumption by the main energy sectors in the United States is examined over an extended period of 1974–2019. By employing suitable estimation techniques, the findings caution on the implication of increased intensities of energy use while also recommending relevant policy to improve the country’s environmental quality without hindering economic output.
In the other part of the study, the related literature is reviewed in section Literature review. We illustrate the Data description with methodological approaches and discussion of the findings in sections Data and methodology and Empirical analysis and results, respectively. Lastly, the summary of the study with related policies is presented in section Discussion of results.
Literature review
Increased human activities in the last 150 years is attributed to GHG emissions and the warming of the earth’s surface arising from the consumption of conventional energy sources for the purpose of cooling and heating, power, and other energy-induced activities [Citation4–9]. According to the [Citation10], the primary sources of greenhouse gas are transportation, electricity production, industry, commercial and residential, agriculture, land use, and forestry. Energy consumption which is a major contributor to carbon dioxide emissions cut across the use of energy resources for commercial, industrial, and/or residential activities. Besides, according to the EPA report, the level of carbon emission from transportation accounted for 28.2% of total carbon emissions in 2018. This transportation-related emissions which is the highest comes basically from the consumption of non-renewable energy sources by vehicles, trucks, ships, prepares, and planes. It should be noted that transportation process may occur differently across the sectors thus difference in their intensity. Roughly 63% of power comes from consumption of petroleum derivatives, coal, and flammable gas. Consequently, the intensity of the energy resources which measures the level of energy inefficiencies of countries is usually calculated as units of energy per unit of GDP [Citation11,Citation12]. High energy intensities show the high cost of converting energy to gross domestic product and vice-versa.
Energy intensity and carbon emissions
Ref. [Citation3] found that the determinants of energy efficiency in an energy-exporting country are attributed to structural changes in the economic activities and inefficiency associated with energy consumption. The findings show that total factor productivity, real energy prices as well as industrial development may also be a determinant of energy intensity in both immediate and long term. Additionally, green energy innovation improves energy consumption with low carbon emissions, thus minimizing environmental degradation effect. Ref. [Citation13] found that there is a short run and long-run relationship between energy intensities and activities of green energy innovation. The innovation in green energy space ranges from innovations in the form of energy storage, such as smart grid which improves the viability of wind and solar power. Ref. [Citation14] seek to understand the important of renewable and non-renewable energy in the reduction of carbon emissions across 28 selected Sub-Saharan African countries by using fully modified ordinary least squares (OLS) and generalized method of moments (GMM) estimation techniques. By covering the period from 1980 to 2014, it was discovered that conventional and non-conventional energy consumptions contribute to emission of carbon dioxide in the long run. But the positive influence of non-renewable energy consumption on carbon emissions is both in the short and long run.
Similarly, how carbon emission changes with renewable energy consumption alongside other factors, such as foreign direct investment and globalization between period 1980 and 2015 was investigated in Ref. [Citation15]. By employing a panel of 46 sub-Saharan African countries and fixed and random effect approaches, the study found that renewable energy and foreign investments lead to mitigation of carbon emissions. By implementing a relatively different approach over the period from 1952 to 2012, determinants of carbon emission and economic growth were observed in the case of China [Citation16]. The outcome of the investigation reveals that coal energy exerts a dominant influence on CO2 emission and economic growth. Additionally, gross domestic products have a two-way causal relationship with carbon emissions, electricity consumption, coal, and gas consumption, thus suggesting the need to change the country’s energy portfolio by increasing clean and renewable energy investments.
Ref. [Citation17] investigated the determinants of carbon emissions with specific attention to non-renewable and renewable energy across the European Union (EU) member states during the period from 1980 to 2012. By employing the dynamic ordinary least squares (DOLS) estimator and validating the Environmental Kuznets Curve (EKC) hypothesis, the study found renewable energy and trade are responsible for a decrease in carbon emissions but the reverse is observed with non-renewable energy. Furthermore, there is a two-way causal link between renewable energy and carbon emissions. But there is a one-way causality from real income to carbon and one-way relationship from carbon emissions to non-renewable energy. Ref. [Citation18] seeks to understand which is beneficial toward the mitigation of carbon emissions between renewable energy and nuclear energy. By using the case of 20 countries associated with nuclear energy usage during the period from 1990 to 2014, it was established that emission of carbon dioxide responds negatively only to the use of renewable energy consumption and not nuclear energy consumption in the long run.
According to the European Environment Agency (EEA), agricultural activities accounted for about 464.3 million tons of CO2 emissions which is about 10% of the total greenhouse gas emissions. The majority of the agricultural-related emissions come from soils, manure management, and others from field burning of agricultural residues, rice cultivation, etc. In their study, Ref. [Citation19] investigated emissions from biomass production and transportation in horticulture and ranger service of the Swedish agriculture and forestry. They carried out an analysis of the possibility of transitioning from fossil product-based energy framework to carbon emissions neutral biomass-based system. The outcome of this process was to reveal how energy efficiency in biomass production as well as transportation is influenced. Although the investigation found that both biomass-based energy and fossil fuel-based energy contribute immensely to carbon emissions, carbon emission from fossil-fuel inputs are far intense. Additionally, the result found that a subsequent increase in yield and innovative developments are likely to reduce the carbon emissions from biomass production by 30–50% in a system dominated by fossil fuel-based energy.
Importantly, there are varying dimensions of sector-specific emissions. For instance, land use and forestry are vital for carbon interaction with the atmosphere. However, land-use activities, such as agriculture may contribute greenhouse gas emissions. While afforestation constitutes a natural absorbent for greenhouse gas emissions, forest fires remained an important source of GHG emission [Citation20]. Similarly, Ref. [Citation21] discovered that emission from forest fire may depend on the tree species and fuel typology. Moreover, the effect of silicate fertilizer application was investigated by Ref. [Citation22], The revelation asserts that applying silicate fertilizer may be an effective method of combatting climate change through minimizing soil-related greenhouse gas emissions. Similarly, Ref. [Citation23] seeks to understand how forest fires affect greenhouse gas emissions and discovered that even though a large amount of carbon is released during bush burning, post-fire emissions of greenhouse gases contribute immensely toward climate change and global warming. Given the environmental effect of international travel and trade, Refs. [Citation24,Citation25] investigated the pro-environmental tourism lessons from Adventure, Wellness as well as Eco-tourism (AWE). It was discovered that AWE inspires the adoption of pro-environmental tourism actions.
Conclusively, the industrial greenhouse gas emissions also have its primary source from fossil fuel burning from the use of energy as well as the emission of greenhouse gas from chemical reactions in the industry to produce goods from raw materials. This implies that the emissions of carbon dioxide from energy use and industrial production processes thus the need or environmentally friendly energy consumption policies. Additionally, the commercial and residential emissions of greenhouse gases arise from the burning of fossil fuels heat, energy, the use of some household or business appliances contain the emission of carbon. Previous studies on energy intensities, agricultural emissions, emissions from tourism, and the consumption of energy and carbon emissions have been reviewed in this study. However, supposedly no research has presented specifically to the impact of energy intensities in the different sectors like commercial, residential, and industry intensities. Therefore, this study attempts to fill this gap by investigating the impact of commercial, industry, and household energy intensities on carbon emissions in the USA. Also, this study presents evidence for policy guidelines on the energy intensities and efficiencies of the sectors considered and as a guide for subsequent researches on energy intensity and environmental quality.
Data and methodology
Data
shows the dataset (span over the period 1974–2019) used for this study. It reveals the unit of measurement (column 2), the source of data for each variable (column 3), and then the statistical properties of the variables (column 4–column 8) which contain the mean, maximum, minimum, and Jarque-Bera statistic for checking normality of the data. The average of carbon emission, in million tons of carbon dioxide, is 5136.36 which is between 4358.70 and 5861.10 with a deviation of 425.62 which indicates that there is wide dispersion from the sample average. The average value of the gross domestic product (GDP), in US dollars, is 9.45 trillion with the variability of 5.87 trillion and minimum and maximum value of 1.55 trillion and 21.4 trillion. In the same vein, commercial energy intensity, in millions of Btu per 1000, has a mean of 251.30, variability of 37.76, minimum of 194.40, and maximum of 332.90. On average, the household energy intensity (million Btu per household) has a value of 188.64 with a standard deviation of 10.66 which measures the small amount of variation from the sample mean. Finally, the industrial energy intensity (trillion Btu per industrial production complex) has an average value of 441.18 between the range of 290.90 and 697.80 with a standard variability score of 126.84.
Table 1. Statistics properties.
Furthermore, to assess the nature and trend of the data over time, reveals the time plot of the log of the examined variables. The figures reveal that the behavior of carbon emission and household energy intensity have downward trend from 1975 till 1980 before rising again. Meanwhile, on the contrary, the industrial energy intensity and the commercial energy intensity declined throughout the studied years. Furthermore, the values are high between 1995 to 2010 before it begins to decline again. So, currently, there is a reduction in the emission of carbon dioxide and the intensity of household energy in the country. Additionally, there is a huge improvement in the gross domestic product as the trend is observed to be increasing ().
Model and method
Amidst the economic growth of the United States of America (USA), this study estimates the impact of commercial, industry, and household energy intensities on carbon emission (Carbon function). On the other hand, the study reveals the sector intensities amidst the research and development expenses for energy efficiency on economic expansion in the United States (Cobb-Douglas function). To avoid spurious regression, this study, rather than the usual Ordinary Least Square (OLS) method, adopts three cointegration techniques, such as Fully Modified OLS (FMOLS), Dynamic OLS (DOLS), and Canonical Cointegration Regression (CCR) as well as Autoregressive Distributed Lag (ARDL) model to investigate the long-term and short-term relationships between the dependent variable (CEM) and the independent variables. For this purpose, the main model for this study is:
(1)
(1)
where CEM denotes carbon emission, GDP denotes gross domestic product, commercial denotes commercial energy intensities, household denotes household energy intensities, and industrial denotes industrial energy intensities. To get a more appropriate and better result, the variables were transformed using a logarithmic function. Thus, the environmental model becomes:
(2)
(2)
where:
is the constant term,
represent the coefficient of the independent variables, and lastly,
represents the error term.
From the second perspective, the growth aspect of the United States economy is examined from the below econometric model (EquationEquation 3(3).
(3). ).
(3).
(3).
Empirical analysis and results
Pre-estimation tests
A unit root test of stationarity needs to be checked for before running a cointegration model on any time series data. This is because the regression could lead to spurious or bias result when the data are non-stationary. There are different methods to perform unit root test, but the one adopts in this study is augmented dickey fuller (ADF) test and Lee Strazicich unit root. The series is checked at the level and first difference. If there is evidence of unit root at the level term of the series, it means the series is nonstationary, and thus it needs to be the difference. If the first difference of the series is stationary, then it denotes that the series has no unit root, and thus it is integrated of order one I(1). Hence, the stationary data can be used in the model to generate a reasonable result.
Despite checking the stationarity of the variables, it is essential to assess the long-run relationships of the studied variables. This will be done using a cointegration test to assure of the meaningful result of residuals and regression result. two methods of cointegration rank test, that is, Trace and Eigenvalue, are considered. The null hypothesis of both methods suggested that there is evidence of cointegration in the null hypothesis, while the alternative hypothesis suggested that there is evidence of cointegration relationship. Hence, if the statistic value of each test is greater than the critical value, the null hypothesis will be rejected in favor of the alternative hypothesis. The stationarity and cointegration evidence are displayed in .
Table 2. Unit root and cointegration.
Long-run and short-run coefficients
After confirming the stationarity and cointegration relationship between the independent variables and the dependent one, then long-run and short-run were estimated using FMOLS [Citation27], DOLS [Citation28], CCR, and ARDL estimation techniques. The FMOLS eliminate the association between the independent variables and error term while addressing the serial correlation heteroscedasticity [Citation29]. The DOLS adds the lag terms of the independent variables while eliminating the association between the dependent variables and the error term [Citation30,Citation31]. CCR provides results by generating smaller bias regression than OLS [Citation32,Citation33]. As for robustness estimation, ARDL of Ref. [Citation34] is further deploy to ascertain the reliability of the named cointegration techniques (see result in ). In specific, the FMOLS model is employed for EquationEquation (1)(1)
(1) , thus estimating the coefficient for EquationEquation (2)
(2)
(2) , and all the results are shown in (for the environmental model) while presents the results of the economic model. However, the step-by-step expressions for the DOLS and CCR are not provided here because of space.
Table 3A. Environmental model.
Table 3B. Autoregressive distributed lag.
Table 4. FMOLS, DOLS, and CCR estimations (economic model).
Diagnostic checks
Finally, diagnostic checks were performed to check the suitability of the model for policy direction. For instance, Jarque-Bera Statistic test whether the data sample is from the normal distribution, which is of significant interest. Breusch-Godfrey (SC LM test) assess if the regression model contains autocorrelation or serial correlation in the error term while Breusch-Pagan-Godfrey (H test) assess the presence of heteroscedasticity, that is, whether the standard error of the regression and the independent variables are dependent. Finally, the cumulative sum (CUSUM) and cumulative sum of square (CUSUMSQ) are also presented to show the stability of model estimates (see ). These results are indicated in the lower part of .
Discussion of results
In (Upper panel), the two methods (ADF with intercept and trend and Lee Strazicich with break date) of unit root tests present, indicating the stationarity of the variables at first difference, i.e. the variables are integrated in order 1, I(1). Additionally, (Lower panel) reports the cointegration result test. Since the statistic value is greater than the critical value, the null hypothesis of no cointegration was rejected, thus indicating that there is evidence of long-run relationships between the covariates and the carbon emission.
revealed the estimation of the long-run and short-run relationships of the economic model described in section Data and methodology. The result shows that FMOL, DOLS, CCR (panel A), and ARDL (panel B) estimations are consistent and significant. All the coefficients of independent variables are positive in the long run and short run. For instance, there is a significant impact of GDP, commercial, household, industrial energy intensities on carbon emission in the long run for all the estimators. This means that a percentage increase in GDP, commercial, household, and industrial energy intensities in the USA is responsible for about 0.36, 1.02, 0.49, and 0.19% increase in carbon emission, respectively. In this scenario, the positive impact of these indicators on carbon emission could be attributed to the longer period of upward trend in the USA’s energy-related carbon emission. The environmental degradation effect arising from the increase in GDP is consistent with the findings of Refs. [Citation35,Citation36] on the influence of economic growth on environmental degradation. Furthermore, the increase in the intensities of energy use in household, commercial, and industrial specifically indicate that the USA’s energy mix is yet predominantly from fossil sources. Thus, this outcome is in line with the study of Refs. [Citation37,Citation38] using renewable and nonrenewable as a proxy for energy use. Moreover, in the short run, GDP, commercial, and household energy intensities have a significantly positive impact on carbon emission. However, industrial energy intensity does not significantly predict the carbon emission.
In the second part (see ), the economic aspects of sectoral energy intensities are presented in . For the employed estimators, the result generally supports the evidence that R&D expenditure on energy efficiency (leading to cost-effectiveness) trigger economic expansion in the United States and the relationship is essentially significant for the CCR. Even for the FMOLS and DOLS outcomes, where the relationship is not significant, there is a possibility for a positive association. This is desirable and suggests that economic output is productive or optimized even at a minimized energy utilization. A similar evidence positive nexus between energy efficiency and growth has been established in the literature [Citation39–42]. Regarding energy intensities, energy intensity in the household sector tends to increase output, but the relationship is not statistically significant for all the estimators. However, increase in energy intensities in the commercial and industrial sectors are significantly causing downturn in economic expansion in the United States. This result is expected considering the high energy intensity is associated with high cost of productivity, thus hampering economic performance. Importantly, the study of Ref. [Citation42] for the high income, upper-middle income, and lower-middle-incomes illustrates related statistical evidence.
The model fit is free from diagnostic error and is suitable for policy direction. The insignificant value of Jarque-Bera Statistic suggests that null hypothesis of a normal distribution is not rejected. Additionally, the Breusch-Godfrey (SC LM test) suggests that there is no serial correlation and the Breusch-Pagan-Godfrey (H test) result suggests that heteroscedasticity is not present (see lower part of ). Finally, to test the stability of the estimated models, CUSUM and CUSUMSQ (see ) were performed. Since the blue lines are within the critical bonds at the 5% level, then it indicates that the estimates are stable and ready making decision by policymakers.
Conclusion and policy implication
This study is focused on combating the issue of environmental consequences from energy intensities and improving the economic development in the USA. Thus, the result shows the influence of commercial, industrial, and household energy intensities on carbon emission as well as the gross domestic product as measure of economic growth. For pre-investigation, the presence of stationary and cointegration relationship were tested. Then, FMOLS, DOLS, CCR, and ARDL were deployed to evaluate the long-run and short-run relationship between covariates and carbon emission. The cointegration techniques reveal that all the covariates are positive and significantly related to carbon emission in the long-run. For instance, an increase in economic growth alleviates the emission of carbon dioxide to the environment. Similarly, as more energy is used across the commercial, household, and industrial sectors, there is an increase in the release of carbon emission. Stretching further, in the short-run, carbon emission is influenced significantly and positively by GDP, commercial, and household energy intensities while industrial energy intensities have no significant short-run impact. On the economic dimension, the results show that commercial and industrial section energy intensities are detrimental to economic expansion while energy efficiency promotes economic expansion. Importantly, it is revealed that increased expenditure on energy efficiency is a significant catalyst to improve the country’s output. Of course, this study is limited in the scope of sector relevance. Therefore, future studies can accommodate other sectors of the economy while also considering other related potential indicators.
Policy recommendation
In spite of the limitation of this investigation, the outcome potent useful policy insight. More economy-wide approach is essential for the decoupling of economic performance from environmental degradation. This sector-wide approach should specifically encourage adoption of environmental-related behaviors that directly and indirectly influences consumer choices. Additionally, it is obvious that energy efficiency and energy saving technologies, and other approaches to improve and scale up efficient use of energy resources are strongly desirable in the USA to mitigate the effect of environmental degradation arising from carbon emissions. Therefore, investment in the forms of energy saving technologies should be scaled up by government and privates’ active participation in related research and development, and innovations activities. This recommendation is particularly vital toward improving economic performance considering that improvement in energy efficiency potentially avert “energy waste” and energy intensity, thus favoring cost-effectiveness in the long-run. The indication from the R&D in energy efficiency and output nexus suggests that the United States is on the path to achieving the total economic electricity savings potential by state (2035) and the estimated economic potential electricity savings by state (2012–2042 and 2010–2040). Thus, fiscal policies that further encourage investment in energy technology development cannot be over-emphasized.
Ethical approval
Not applicable.
Consent to participate
Not applicable.
Consent to publish
Not applicable.
Acknowledgments
The authors appreciate the Editor and the anonymous referees.
Disclosure statement
No potential conflict of interest was reported by the author(s).
Data availability statement
Data are available upon request from the corresponding author.
Additional information
Funding
Notes
1 The USA sectoral energy intensity is measured as the amount of energy consumed by a sector per dollar of sectoral gross output while the aggregate energy intensity (by country or globally) is by measured as the amount of primary energy consumed per dollar of GDP.
References
- Hille E, Lambernd B. The role of innovation in reducing South Korea’s energy intensity: regional-data evidence on various energy carriers. J Environ Manage. 2020;262(December 2019):110293. doi: 10.1016/j.jenvman.2020.110293.
- EIA. U.S. energy facts explained; 2020 [cited 2021 Jan 26]. Available from: https://www.eia.gov/energyexplained/us-energy-facts/
- Dargahi H, Khameneh KB. Energy intensity determinants in an energy-exporting developing economy: case of Iran. Energy. 2019;168:1031–1044. doi: 10.1016/j.energy.2018.12.015.
- Alola AA. The trilemma of trade, monetary and immigration policies in the United States: accounting for environmental sustainability. Sci Total Environ. 2019;658:260–267. doi: 10.1016/j.scitotenv.2018.12.212.
- Alola AA, Adebayo TS, Olagunju I. Assessment of sectoral greenhouse gas emission effects of biomass, fossil fuel, and (non) metallic ore utilization of the Nordic economy. Miner Econ. 2023. doi: 10.1007/s13563-023-00390-2.
- Bekun FV, Alola AA, Sarkodie SA. Toward a sustainable environment: nexus between CO2 emissions, resource rent, renewable and nonrenewable energy in 16-EU countries. Sci Total Environ. 2019;657:1023–1029. doi: 10.1016/j.scitotenv.2018.12.104.
- Ramzan M, Abbasi KR, Salman A, et al. Towards the dream of go green: an empirical importance of green innovation and financial depth for environmental neutrality in world’s top 10 greenest economies. Technol Forecast Soc Change. 2023;189:122370. doi: 10.1016/j.techfore.2023.122370.
- Yi S, Abbasi KR, Hussain K, et al. Environmental concerns in the United States: can renewable energy, fossil fuel energy, and natural resources depletion help? Gondwana Res. 2023;117:41–55. doi: 10.1016/j.gr.2022.12.021.
- Zhang M, Abbasi KR, Inuwa N, et al. Does economic policy uncertainty, energy transition and ecological innovation affect environmental degradation in the United States? Econ Res. 2023;36(2):2177698. doi: 10.1080/1331677X.2023.2177698.
- United States Environmental Protection Agency. Energy and the environment explained; 2023 [cited 2023 Sep 4]. Available from: https://www.eia.gov/energyexplained/energy-and-the-environment/where-greenhouse-gases-come-from.php
- European Commission. 2023 [cited 2023 Sep 2]. Available from: https://ec.europa.eu/eurostat/statistics-explained/index.php?title=Energy_statistics_-_an_overview#Energy_intensity
- World Bank. 2023 [cited 2023 Sep 2]. Available from: https://data.worldbank.org/indicator/EG.EGY.PRIM.PP.KD?locations=EU
- Chakraborty SK, Mazzanti M. Energy intensity and green energy innovation: checking heterogeneous country effects in the OECD. Struct Change Econ Dyn. 2020;52:328–343. doi: 10.1016/j.strueco.2019.12.002.
- Adams S, Nsiah C. Reducing carbon dioxide emissions; does renewable energy matter? Sci Total Environ. 2019;693:133288. doi: 10.1016/j.scitotenv.2019.07.094.
- Acheampong AO, Adams S, Boateng E. Do globalization and renewable energy contribute to carbon emissions mitigation in sub-Saharan Africa? Sci Total Environ. 2019;677:436–446. doi: 10.1016/j.scitotenv.2019.04.353.
- Long X, Naminse EY, Du J, et al. Nonrenewable energy, renewable energy, carbon dioxide emissions and economic growth in China from 1952 to 2012. Renew Sustain Energy Rev. 2015;52:680–688. doi: 10.1016/j.rser.2015.07.176.
- Dogan E, Seker F. Determinants of CO2 emissions in the European Union: the role of renewable and non-renewable energy. Renew Energy. 2016;94(2016):429–439. doi: 10.1016/j.renene.2016.03.078.
- Jin T, Kim J. What is better for mitigating carbon emissions – renewable energy or nuclear energy? A panel data analysis. Renew Sustain Energy Rev. 2018;91(April):464–471. doi: 10.1016/j.rser.2018.04.022.
- Börjesson PII. Emissions of CO2 from biomass production and transportation in agriculture and forestry. Energy Convers Manage. 1996;37(6–8):1235–1240. doi: 10.1016/0196-8904(95)00326-6.
- Volkova L, Roxburgh SH, Surawski NC, et al. Improving reporting of national greenhouse gas emissions from Forest fires for emission reduction benefits: an example from Australia. Environ Sci Policy. 2019;94(May 2018):49–62. doi: 10.1016/j.envsci.2018.12.023.
- Guo L, Ma Y, Tigabu M, et al. Emission of atmospheric pollutants during Forest fire in boreal region of China. Environ Pollut. 2020;264:114709. doi: 10.1016/j.envpol.2020.114709.
- Xu L, Deng X, Ying J, et al. Silicate fertilizer application reduces soil greenhouse gas emissions in a Moso bamboo forest. Sci Total Environ. 2020;747:141380. doi: 10.1016/j.scitotenv.2020.141380.
- Ribeiro-Kumara C, Köster E, Aaltonen H, et al. How do Forest fires affect soil greenhouse gas emissions in upland boreal forests? A review. Environ Res. 2020;184(February):109328. doi: 10.1016/j.envres.2020.109328.
- Bilgic A, Florkowski WJ, Yoder J, et al. Estimating fishing and hunting leisure spending shares in the United States. Tour Manage. 2008;29(4):771–782. doi: 10.1016/j.tourman.2007.09.001.
- Hunt CA, Harbor LC. Pro-environmental tourism: lessons from adventure, wellness and eco-tourism (AWE) in Costa Rica. J Outdoor Recreat Tour. 2019;28(July 2018):100202. doi: 10.1016/j.jort.2018.11.007.
- IEA. Energy efficiency indicators: fundamentals on statistics. Paris: IEA; 2014. doi: 10.1787/9789264215672-en.
- Pedroni P. Purchasing power parity tests in cointegrated panels. Rev Econ Stat. 2001;83(4):727–731. doi: 10.1162/003465301753237803.
- Kao C, Chiang M. On the estimation and inference of a cointegrated regression in panel data. Adv Econ. 2000;15(1):109–141. doi: 10.1016/S0731-9053(00)15007-8.
- Phillips PC, Hansen BE. Statistical inference in instrumental variables regression with I (1) processes. Rev Econ Stud. 1990;57(1):99–125. doi: 10.2307/2297545.
- Saikkonen P. Estimation and testing of cointegrated systems by an autoregressive approximation. Econ Theory. 1992;8(1):1–27. doi: 10.1017/S0266466600010720.
- Hu H, Xie N, Fang D, et al. The role of renewable energy consumption and commercial services trade in carbon dioxide reduction: evidence from 25 developing countries. Appl Energy. 2018;211:1229–1244. doi: 10.1016/j.apenergy.2017.12.019.
- Stock JH, Watson MW. A simple estimator of cointegrating vectors in higher order integrated systems. Econometrica. 1993;61(4):783–820. doi: 10.2307/2951763.
- Montalvo JG. Comparing cointegrating regression estimators: some additional Monte Carlo results. Econ Lett. 1995;48(3–4):229–234. doi: 10.1016/0165-1765(94)00632-C.
- Pesaran MH, Shin Y, Smith RJ. Bounds testing approaches to the analysis of level relationships. J Appl Econ. 2001;16(3):289–326. doi: 10.1002/jae.616.
- Apergis N, Payne JE. CO2 emissions, energy usage, and output in Central America. Energy Policy. 2009;37(8):3282–3286. doi: 10.1016/j.enpol.2009.03.048.
- Ma M, Cai W. Do commercial building sector-derived carbon emissions decouple from the economic growth in tertiary industry? A case study of four municipalities in China. Sci Total Environ. 2019;650(Pt 1):822–834. doi: 10.1016/j.scitotenv.2018.08.078.
- Farhani S, Shahbaz M. What role of renewable and non-renewable electricity consumption and output is needed to initially mitigate CO2 emissions in MENA region? Renew Sustain Energy Rev. 2014;40:80–90. doi: 10.1016/j.rser.2014.07.170.
- Bilgili F, Koçak E, Bulut Ü. The dynamic impact of renewable energy consumption on CO2 emissions: a revisited environmental Kuznets curve approach. Renew Sustain Energy Rev. 2016;54:838–845. doi: 10.1016/j.rser.2015.10.080.
- Howarth RB. Energy efficiency and economic growth. Contemp Econ Policy. 1997;15(4):1–9. doi: 10.1111/j.1465-7287.1997.tb00484.x.
- Cantore N, Calì M, Te Velde DW. Does energy efficiency improve technological change and economic growth in developing countries? Energy Policy. 2016;92:279–285. doi: 10.1016/j.enpol.2016.01.040.
- Bataille C, Melton N. Energy efficiency and economic growth: a retrospective CGE analysis for Canada from 2002 to 2012. Energy Econ. 2017;64:118–130. doi: 10.1016/j.eneco.2017.03.008.
- Rajbhandari A, Zhang F. Does energy efficiency promote economic growth? Evidence from a multicountry and multisectoral panel dataset. Energy Econ. 2018;69:128–139. doi: 10.1016/j.eneco.2017.11.007.