ABSTRACT
Preeclampsia is a multifactorial disorder defined by hypertension and increased urinary protein excretion during pregnancy. It is a significant cause of maternal and neonatal deaths worldwide. Despite various research efforts to clarify pathogenies of preeclampsia and predict this disease before beginning of symptoms, the pathogenesis of preeclampsia is unclear. Early prediction and diagnosis of women at risk of preeclampsia has not markedly improved. Therefore, the objective of this study was to perform a review on metabolomic articles assessing predictive and diagnostic biomarkers of preeclampsia. Four electronic databases including PubMed/Medline, Web of Science, Sciencedirect, and Scopus were searched to identify studies of preeclampsia in humans using metabolomics from inception to March 2018. Twenty-one articles in a variety of biological specimens and analytical platforms were included in the present review. Metabolite profiles may assist in the diagnosis of preeclampsia and discrimination of its subtypes. Lipids and their related metabolites were the most generally detected metabolites. Although metabolomic biomarkers of preeclampsia are not routinely used, this review suggests that metabolomics has the potential to be developed into a clinical tool for preeclampsia diagnosis and could contribute to an improved understanding of disease mechanisms.
Abbreviations: PE: preeclampsia; sFlt-1: soluble FMS-like tyrosine kinase-1; PlGF: placental growth factor; GC–MS: gas chromatography–mass spectrometry; LC–MS: liquid chromatography–mass spectrometry; NMR: nuclear magnetic resonance spectroscopy; HMDB: human metabolome database; RCT: randomized control trial; e-PE: early-onset PE; l-PE: late-onset PE; PLS-DA: partial least-squares-discriminant analysis; CRL: crown-rump length; UtPI: uterine artery Doppler pulsatility index; BMI: body mass index; MAP: mean arterial pressure; OS: oxidative stress; PAPPA: plasma protein A; FTIR: Fourier transform infrared; BCAA: branched chain amino acids; Arg: arginine; NO: nitric oxide.
Introduction
Preeclampsia (PE) is a pregnancy syndrome and a main cause of maternal mortality, which complicates 5–10% of pregnancies worldwide (Sibai Citation1992). This disease is characterized by hypertension, proteinuria, and/or edema occurring after 20 weeks of gestation (Sibai et al. Citation2005). The clinical maternal risk factors for PE include increasing maternal age, previous history of PE, family history of PE, nulliparity, obesity, multifetal gestation, diabetes mellitus, chronic hypertension, and chonic renal disorders (Sibai et al. Citation1995; Poon et al. Citation2010; Polsani et al. Citation2013). It can be divided into two subgroups: early- and late-onset disease, where early onset is characterized as occurrence of symptoms and preterm delivery (PD) before 34 weeks of gestation, whereas clinical manifestation and delivery of late-onset occurs after 34 weeks of pregnancy (Austdal et al. Citation2015b). Early onset is also defined as a placental disorder in which abnormal trophoblast invasion along with decreased remodeling in the maternal spiral artery can reduce transfer of oxygen and nutrients to fetus, subsequently, during compensation the mother’s blood pressure is enhanced and PE symptoms manifested (Bahado-Singh et al. Citation2012). In contrast, late-onset is considered a maternal disorder because of pre-existent maternal metabolic and vascular disorders (Wikström et al. Citation2007).
Despite decades of studies on PE, the major cause of this disease remains unclear. Furthermore, the only treatment is delivery and removal of the fetus and placenta (Staff Citation2011). Of note, prophylactic treatment can be influenced if the women at risk for developing PE were identified early. Diagnostic criteria for PE to date include clinical parameters and laboratory examinations such as some proteins related to angiogenesis (soluble FMS-like tyrosine kinase-1 (sFlt-1), placental growth factor (PlGF), and endoglin and so on) along with abnormal Doppler findings which have a relatively low predictive value (Cnossen et al. Citation2009; Austdal et al. Citation2014). Therefore, investigations on novel and noninvasive diagnostic methods for predicting PE prior to the onset of symptoms would be important and may improve clinical decisions for using preventive intervention.
Because PE encompasses multifactorial disorders, high-throughput technologies that can identify multiple markers simultaneously can be effective screening methods for biomarker discovery rather than traditional tools which detect single marker. One of the most important high-throughput techniques is metabolomics in which metabolites, the end products of cellular regulatory processes, can be identified by metabolomics methods including nuclear magnetic resonance spectroscopy (NMR), gas- and liquid chromatography–mass spectrometry (GC– and LC–MS) (Nobakht et al. Citation2015, Citation2016a, Citation2016b, Citation2017; Kalantari et al. Citation2016; Bahado-Singh et al. Citation2017b; Ghoochani et al. Citation2018). Of note, there are limitations and challenges in metabolomics studies, as explained below: (1) heterogeneity, (2) analytical metabolomics platforms, (3) sample collection, storage and preparation, (4) data mining and metabolite identification, and (5) reproducibility.
Heterogeneity. The metabolome is more heterogeneous than the genome and proteome, because metabolites contain a variety of molecules including aldehydes, ketones, fatty acids, amino acids, while nucleotides and amino acids are as building blocks of genes and proteins, respectively. Therefore, metabolomics analysis is more difficult than other macromolecules, so analytical and biological variations must be controlled in metabolomics investigations.
Analytical metabolomics platforms. Most technologies that are used for metabolomics studies are NMR (based on the magnetic properties of atomic nuclei) and MS (GC– and LC–MS based on ionization and fragmentation of metabolites). Each method has some advantages and disadvantages. For instance, NMR is a highly reproducible and nondestructive approach that requires no or minimal sample preparation (protein precipitation and chemical derivatization) (Nicholson and Lindon Citation2008; Emwas et al. Citation2013). However, one of the biggest drawbacks of NMR is the generation of spectra with many overlapping signals; so the use of the two-dimensional NMR technique has been developed to overcome this limitation. Another drawback is differences in sensitivity, and the sensitivity of NMR is lower than MS methods (nM to pM range); thus, it is not able to detect low abundance small molecules, resulting in requiring high volumes of samples (Monteiro et al. Citation2013).
GC–MS methods require extensive sample preparation (protein precipitation and derivatization). They enable the detection of volatile compounds or metabolites which can be volatilized by derivatization. Of note, the derivatization step is time-consuming and costly. Furthermore, it can lead to the formation several derivatized compounds from a single molecule due to the presence of various active hydrogens in the metabolite. (Dunn and Ellis Citation2005).
LC–MS is an analytical approach for the detection of both hydrophilic and hydrophobic metabolites with key advantages, including high sensitivity, small sample volumes, and not requiring chemical derivatization (Wang et al. Citation2010; Monteiro et al. Citation2013). However, it has limited databases and software in comparison with GC–MS. Furthermore, run times in MS-based approaches are usually long (Monteiro et al. Citation2013).
Sample collection, storage and preparation. Sample collection and storage are important in order to prevent metabolite degradation and unrelated metabolite production, especially metabolites related to bacterial growth in urine (Lauridsen et al. Citation2007); thus, adding NaN3 (because of limited interferences to NMR spectra) to urine before storage is recommended to avoid contamination (Scalbert et al. Citation2009).
The details of blood collection including clotting time, clotting temperature and centrifuge time, and speed are able to alter metabolite profile (Scalbert et al. Citation2009). Furthermore, some materials released from blood collection tubes may interfere with the mass spectrometry results (Drake et al. Citation2004). The studies showed that the metabolomic profile is different between samples that were collected at different times of day (in the morning and afternoon). Therefore, sample collection time is another important factor that can affect metabolomic profile (Slupsky et al. Citation2007).
In order to reduce effect of macromolecules, sample preparation including protein precipitation and metabolite extraction are performed in some metabolomics studies (Monteiro et al. Citation2013). Since there is no a single procedure that covers all metabolites in mass spectrometry, combined protocols for extraction of polar and non-polar metabolites are used (Yanes et al. Citation2011). However, metabolomics-based NMR is able to detect two groups of metabolites with no or minimal extraction (Emwas et al. Citation2013).
Data mining and metabolite identification. There is no global software for analysis of metabolomics data (Wang et al. Citation2010). Because of differences in instrument sensitivity on different days and also in different laboratories, one of the biggest drawbacks of metabolomic data analysis is data normalization. To overcome this limitation, internal standards are used in MS-based methods (Wang et al. Citation2010).
Metabolite identification is dependent on electronic databases (Wishart et al. Citation2008). Despite development of metabolome databases such as human metabolome database (HMDB: www.hmdb.ca), these databases could not overcome drawbacks of metabolite identification. For example, in MS methods, a given ion mass can be matched with several compounds or ion suppression may be occurred by endogenous matrix compounds of MS; so metabolites cannot be accurately identified (Yanes et al. Citation2011; Monteiro et al. Citation2013). The workflow of metabolomics studies is illustrated in .
Figure 1. Representative workflow of metabolomics studies. ppm: parts of million (chemical shift in NMR is usually expressed in ppm), RT: retention time, MW: molecular weight, HMDB: human metabolome database, BMRB: biological magnetic resonance bank, KEGG: Kyoto Encyclopedia of Genes and Genomes.
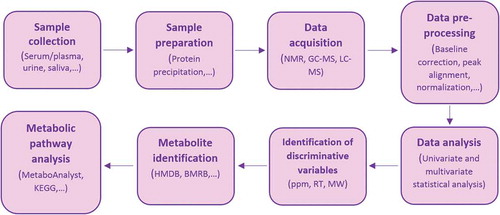
Reproducibility. Low reproducibility has been reported in metabolomics experiments, which may be due to both internal factors including age, gender, gut microflora, and genetics as well as external factors such as diet, physical activity, analytical approaches and experimental conditions (experimental temperature, salt, and pH) (Emwas et al. Citation2015).
Despite existing limitations in metabolomics studies, there are lots of published metabolomics literature on PE. These literature have suggested that metabolomics can recognize metabolic biomarkers involved in disease and improve the management of patients with PE. The aim of this review was to summarize metabolomics studies on PE patients to find whether metabolites are able to distinguish PE patients from normotensive pregnancies and its different subtypes (e-PE vs. l-PE). Another aim was to obtain a better understanding of the underling mechanisms of this disease based on metabolomics markers.
Literature summary
Data sources and search strategy inclusion/exclusion criteria
A literature search was performed using the electronic databases PubMed/Medline, Web of Science, Sciencedirect, and Scopus from inception to March 2018, with no language restriction. All published and in press articles except conference publications were used in the search. The following keywords were chosen in titles and abstracts: ‘Preeclampsia’, ‘Pregnancy Toxemia’, ‘Pre-eclamptic Toxemia’, ‘Pre-eclampsia’, ‘metabolomic’, ‘metabolomics’, ‘metabolomic biomarker’. Boolean operators (AND and OR) were used for designing key terms. Studies that met the following criteria were included: (1) being an original article, (2) being an observational study, (3) conducted amongst adults of any ethnic origin. Exclusion criteria were: (1) being a review article, (2) being a randomized control trial (RCT), and (3) being animal, in vitro or ex vivo studies.
Characteristics of studies included
The keyword search in four databases provided 204 articles. After removing duplicates, 111 studies remained. After reviewing titles and/or abstracts, 21 studies were eligible for review (). The articles selected were reviewed and the following information is summarized in : name of the first author, year of publication, country where study was done, number of participants, biological specimen, parity, statistical analysis, type of metabolomics method, a list of metabolites, direction of metabolites compared with control, and model characteristics (sensitivity, specificity and accuracy). Within this group, 16 studies investigated blood (serum/plasma) (Kenny et al. Citation2005, Citation2008, Citation2010; Odibo et al. Citation2011; Bahado-Singh et al. Citation2012, Citation2013, Citation2015, Citation2017a, Citation2017b; Senyavina et al. Citation2013; Austdal et al. Citation2014, Citation2015a; Kuc et al. Citation2014; Mukherjee et al. Citation2014; Koster et al. Citation2015; Chen et al. Citation2017) and two of which also evaluated urine (Austdal et al. Citation2014, Citation2015a). One study used urine (Diaz et al. Citation2013) and finally four studies used placenta (Jain et al. Citation2004; Dunn et al. Citation2012; Austdal et al. Citation2015b; Zhou et al. Citation2017), one of which assessed placental mitochondria (Zhou et al. Citation2017). The studies in are summarized below.
Table 1. Summary of recent metabolomic studies in the field of PE patients.
Study summary
Bahado-Singh et al. Citation2012 investigated the plasma metabolomic profile on women who subsequently developed PE and normal pregnancies in different racial origin (white, black, Asian, and other) during the first trimester of pregnancy for detection of early-onset PE (e-PE) using NMR. This group reported two models: the accuracy of the first model, metabolites alone, had 90.4% () but the second model, metabolites (acetate, glycerol, hydroxyisovalerate) in combination with uterine artery Doppler pulsatility index (UtPI) and fetal crown-rump length (CRL), was 98%. Hence, their results showed that a metabolite profile during the first trimester of gestation in women who later developed PE may be a sensitive method for e-PE prediction (Bahado-Singh et al. Citation2012). Kenny et al. examined Caucasian women with PE and normal pregnancies at the third trimester of gestation and analyzed their plasma by GC–TOF–MS. They reported that three metabolite peaks (two peaks were decreased and one peak was raised in PE patients vs. controls) may discriminate PE patients from controls with sensitivity and specificity 100 and 98%, respectively (Kenny et al. Citation2005).
Kenny et al. utilized ultra-performance liquid chromatography and LTQ Orbitrap mass spectrometry (UPLC–LTQ–MS) for analyzing plasma untargeted metabolomics to validate their previous findings of metabolites that could be used to distinguish patients with PE from controls. They recruited completely different Caucasian women with PE and controls (cohort 1) from different location at different times during the third trimester of pregnancy. This group used subjects from prior work (cohort 2). Significant metabolites from cohort 2 were searched for raw spectra of cohort 1 based on molecular masses. Their results showed that eight metabolites were significantly altered in PE patients in comparison with controls () (Kenny et al. Citation2008).
In another study of Kenny et al., plasma was collected from two groups of women who were from New Zealand and Australia for the discovery and validation phase, respectively. Distinguishing metabolites between patients who went on to develop PE and normotensive pregnancies during the second trimester of gestation were evaluated by UPLC–MS. They used two powerful multivariate statistical analyses including PLS–DA and genetic algorithm to analyze metabolomics data. The PLS–DA results illustrated that 34 significant metabolites were detected in the discovery and the validation sets. Of these 34 metabolites, 14 metabolites were present using genetic algorithm that changed between women who later developed PE and normal pregnancies in the discovery and the validation groups () (Kenny et al. Citation2010).
In another investigation by Mukherjee et al., serum metabolomic profiles of Indian PE patients (early and late onset PE) were analyzed using Fourier transform infrared (FTIR) spectroscopy and followed by NMR to validate the FTIR results (Mukherjee et al. Citation2014). Two groups of normotensive pregnant women who underwent medical checkups were matched for gestational age. Their FTIR findings showed that five spectral peaks were able to discriminant two subtypes of PE according to their matched controls with more than 80% accuracy. Then, NMR-based metabolomics was used and reported six significant metabolites in differentiating early and late PE (). This study indicated that the metabolomics had a good clinical significance in the diagnosis of PE patients as compared with its controls as well as in the identification sub-types of PE.
In another study by Bahado-Singh et al., serum from 59 normal pregnancies and 30 late-onset PE (l-PE) at 11–13 weeks of gestation were collected and analyzed using an NMR. A panel of 17 metabolite biomarkers, of which 14 metabolites increased and 3 metabolites decreased, which was able distinguish l-PE patients from control cases. They also compared the 30 e-PE subjects (their prior work Bahado-Singh et al. Citation2012) with 30 l-PE women and reported that first-trimester metabolic markers including glycerol, acetate, trimethylamine, and succinate seem to discriminate late- from early-onset PE (Bahado-Singh et al. Citation2013).
As a continuation of their previous investigation, they compared the serum metabolomic profile in e-PE with healthy pregnant women (Bahado-Singh et al. Citation2015). In addition l-PE was compared to healthy pregnant women (Bahado-Singh et al. Citation2017b). To assess the diagnostic accuracy of the metabolite markers during the first-trimester of the two types of PEs both groups were compared using a larger sample size. This group reported that five and three metabolites () using multivariate statistical analysis had high predictive accuracy for first trimester of e-PE (Bahado-Singh et al. Citation2015) and l-PE (Bahado-Singh et al. Citation2017b), respectively. They also showed that the combination of metabolic markers and UtPI appear to suggest more accurate predictive markers for both kind of PEs. For e-PE, 3-hydroxyisovalerate, arginine, glycerol when combined with UtPI achieved a 91.6% accuracy, 90% sensitivity, and 88.4% specificity for the validation group. These metabolites are related to vascular dilation and impaired glucose regulation. For l-PE, several metabolites increased during first trimester of gestation (). Metabolic pathways involved in l-PE were branched chain amino-acid metabolism, propanoate metabolism, glycolysis, gluconeogenesis, and ketone body metabolism.
Another study on serum metabolomic profile of PE patients was performed by Bahado-Singh et al. (Citation2017a) who applied metabolomics using 1HNMR and LC–MS/MS along with investigating proteomics by matrix-assisted laser desorption ionization time of flight MS (MALDI–TOF/TOF–MS) for further understanding of mechanisms this disease. They recruited serum at the first and third trimesters from PE and healthy pregnant women. Their finding showed in the first trimester, combined with several proteins, some discriminant metabolites (presented in ): tumor necrosis factor-α (TNF-α), 60S ribosomal protein L41(RPL41), ATP synthase subunit epsilon (ATP5E), and TATA box binding protein (TBP) with a 74.5% accuracy, 78.8% sensitivity, and 64.8% specificity. In third-trimester metabolites in combination with proteins including GTP binding protein-3 (GTPBP3), human leukocyte antigen D-related beta-1 (HLA-DR B1) and beta 1 major histocompatibility complex (β-1 MHC) and maternal properties such as body mass index (BMI) and mean arterial pressure (MAP) are able to predict PE with 94.1% accuracy, 91.4% sensitivity, and 96.8% specificity. These results showed that metabolomics in combination with proteomics revealed significant changes in signal transduction, G-protein-coupled receptors, serotonin, and glycosaminoglycan metabolisms as well as it improved understanding of underling mechanisms of PE.
Chen et al. (Citation2017) studied serum metabolomic profiling to identify potential biomarkers for the diagnosis of PE in Chinese patients with PE as compared to normotensive controls by LC–Q–TOF/MS. They reported 49 significant metabolites, most of them were phospholipids and their derivatives. Their results showed that phosphatidylcholine (PC) (14:0/00), proline betaine, and proline were potential biomarkers for diagnosis and prognosis of PE with high accuracy. Pathway analysis of their reported metabolites showed alterations in some metabolic pathways such as nitric-oxide signaling along with glycine degradation and mitochondrial dysfunction.
Another investigation on PE patients was performed by Koster et al. (Citation2015). They analyzed acylcarnitines in the first-trimester maternal serum of 68 e-PE, 99 l-PE patients, and 500 normal pregnant subjects using UPLC–MS/MS. Their findings showed that 12 and 11 metabolites were used to separate e-PE and l-PE versus a control group (). In this study, some factors such as plasma protein A (PAPPA) and PlGF in maternal serum were measured as well. This group investigated the accuracy of the combination of some maternal protein markers, maternal characteristics, and acylcarnitines and reported that the best prediction model for e-PE is a combination of prior risk, MAP, PAPPA, PlGF, stearoylcarnitine, and taurine, while the best prediction model for l-PE consisted of prior risk, MAP, PAPPA, PlGF, and stearoylcarnitine. The accuracy, sensitivity, and specificity of the best models are presented in .
Another metabolomics study was performed by Kuc et al. using UPLC–MS/MS. Serum was collected from 167 PE (e-PE and l-PE) patients and 500 healthy pregnancies to predict metabolites (amines and oxylipins) that are significantly changed during the first trimester of two variants of PE in comparison with controls. Of note, in their study patients with PE were often smokers and more often had a history of gestational hypertension. They found that three metabolites significantly distinguished PE patients (taurine and asparagine for e-PE; glycylglycine for l-PE) from healthy controls, but after adding some maternal characteristics to the model (prior risk and MAP) and additional statistical analysis only one metabolic marker (taurine) along with prior risk and MAP were presented as predictive for women who later developed PE. For the l-PE, the model based on prior risk and MAP had a good accuracy, no metabolite indicated any improvement in this group (Kuc et al. Citation2014).
In the study of Odibo, LC–MS/MS-based metabolomics was performed to assess the diagnostic potential of metabolites to predict patients with PE from first-trimester maternal serum. In this investigation, PE patients were African-American volunteers and had higher BMI and were pregestational diabetic subjects. This group showed that seven metabolic markers were raised in PE patients in comparison with controls. However, because of exciting onfounders (BMI, ethnicity, and pregestational diabetes) data were adjusted. Ultimately, a combination of four metabolites () was sufficient for separation between patients and controls (Odibo et al. Citation2011).
Senyavina et al. (Citation2013) also investigated purine metabolites in serum for PE and PD as compared with normotensive pregnancies using reverse phase HPLC. This study included 39 participants (6 PE, 9 PD patients, and 25 healthy pregnancies) at trimester of gestation. The concentration of some purine metabolites changed in PE compared with to control group (). In comparison, Austdal et al. utilized NMR for analyzing serum and urine-untargeted metabolites to identify Scandinavian patients with PE from normal pregnant and non-pregnant healthy women at 18–38 weeks of gestation. Their results indicated that nine urine metabolites were altered in PE patients compared to normotensive women. In the case of serum metabolites, increased lipid content has been shown during normal pregnancy, and a further increase was observed in women with PE (). This investigation illustrated that metabolomics might distinguish PE patients from two control groups in the second trimester of gestation (Austdal et al. Citation2014).
As a continuation of their previous study, they evaluated urine and serum metabolites from three different groups of pregnant women with PE, gestational hypertension, and normotensive women in the first trimester of gestation to predict PE before the initiation of symptoms using NMR. They reported that some metabolites in serum and urine were able to discriminate women with PE and gestational hypertension compared women with normotensive pregnancies (). The most important metabolites in women who later developed PE or gestational hypertension presented as decreased hippurate and increased creatinine excretion in addition to elevated levels of serum lipids. Test accuracy in predicting PE improved when metabolites were accompanied by maternal characteristics such as age, MAP at enrolment, and UtPI at enrolment (accuracy: 80.7%) (Austdal et al. Citation2015a).
Diaz et al. (Citation2013) investigated metabolite profile for the early diagnosis of some delivery disorders including PD (n = 26), intra-uterine growth restriction (n = 10), premature rupture of membranes (n = 68), PE (n = 9), and gestational diabetes mellitus (n = 42) along with chromosomal disorders (n = 33) such as trisomy 21 and fetal malformations (n = 35) in comparison with a control group (n = 84). They collected 307 urine samples in the second trimester of gestation and analyzed urine metabolites using NMR. They reported that more than 20 metabolites can predict PE patients, before PE symptoms appear (some significant metabolites are shown in ). This research indicated that metabolomics can be a promising tool for early diagnosis and prognosis of gynecological disorders.
Additionally, Austdal et al. studied the placenta metabolites of women with PE compared to normal pregnant subjects by high-resolution magic angle spinning magnetic resonance spectroscopy (HR–MAS MRS). They also investigated correlation between the placental metabolites and several maternal serum markers. Their results revealed that discriminating placental metabolites that separated PE patients from normotensive pregnancies were involved in phospholipid biosynthesis and decreasing bile acid biosynthesis, taurine metabolism, ammonia and urea cycles, and protein biosynthesis. They also showed that there was a significant correlation between maternal serum markers (including sFlt-1, triglycerides, uric acid, and creatinine) and the placental metabolic profiles (such as glycerophosphocholine, 3-hydroxybutyrate, aspartate, choline, creatine, glycine, glutamate, taurine, glutamine, valine and ethanolamine) (Austdal et al. Citation2015b).
In another study (Dunn et al. Citation2012), placenta metabololites were investigated in PE patients (n = 6, five subjects with vaginal delivery and one subject with caesarean section) as compared to normal pregnancies (n = 11, six subjects with vaginal delivery and five subject with caesarean section) using GC–MS and UPLC–MS. Ethnicity of the women was Caucasian and Asian. This group also compared the metabololites of placenta in two types of delivery (vaginal delivery and caesarean section). No metabolite was significantly observed between normal pregnancies delivered by caesarean section and vaginal delivery in PE patients by GC–MS. However, the results by UPLC–MS showed that seven metabolites including phosphatidylinositol (PI 38:4), nitrate, peroxynitrite, and four unidentified metabolites were significantly different between mentioned two groups (normal pregnancies delivered by caesarean section and PE patients delivered vaginally). Metabolomic analysis between normotensive and PE pregnancies with vaginal delivery showed that 409 and 13 metabolites were statistically different for UPLC–MS and GC–MS, respectively. These small molecules were in several classes such as acyl glycerides, phospholipids, metabolites related to fatty acids, amino acid, vitamin D, isoprenoids, and steroids, which placenta of PE patients showed a reduction in metabolite levels most of these classes.
Because OS is the one of the features of PE and produced by mitochondria, Zhou et al. (Citation2017) studied whether alterations in mitochondrial metabolome of placenta may play a role in this disease. They obtained placental tissues after cesarean section from 11 severe PE patients and 11 normotensive pregnancies. Metabolome analysis of placental mitochondria was undertaken using GC–MS. Some candidate proteins related to mitochondrial repair regulation were evaluated by this group. Their results showed that the most placental mitochondrial metabolic pathways involved in PE vs. healthy pregnancies were amino acids, fatty acids, and organic acids (some important metabolites are presented in ). The accumulation of some fatty acids such as linoleate, arachidonate, and omega-3 fatty acids in placental mitochondria of PE is able to produce inflammatory mediators, vasodilators/vasoconstrictors which are features of this disease. According to the metabolomic results that revealed alterations in the mitochondrial metabolome of PE patients, downregulation of proteins involved in mitochondrial fusion, mitophagy, and biosynthesis were also shown.
Another investigation (Jain et al. Citation2004) used electrospray ionization mass spectrometry (ESI-MS) for evaluating of placental phospholipids and fatty acids in PE patients compared with healthy pregnancies. This group reported alterations in fatty acids and plasmenyl phosphatidylethanolamine levels in PE patients ().
Discussion
As mentioned above, metabolic differences between the women with PE and those with healthy pregnancies, in blood (Kenny et al. Citation2005, Citation2008, Citation2010; Odibo et al. Citation2011; Bahado-Singh et al. Citation2012, Citation2013, Citation2015, Citation2017b; Austdal et al. Citation2014, Citation2015a; Kuc et al. Citation2014; Koster et al. Citation2015), urine (Austdal et al. Citation2014, Citation2015a) and placenta (Austdal et al. Citation2015b) are apparent. There is no clear consensus about the pathophysiological basis of PE. Since this disease is associated with mortality and morbidity of the maternal and fetus, and only treatment is delivery, the identification of possible predictive markers of this disease before symptoms using novel ‘omics’ methods is necessary. The metabolomics approach has revealed underlying mechanisms involved in the disease based on metabolic profiles. Herein, we describe some important metabolites that may play a role in the etiology and early identification of PE patients.
Lipid metabolism and its related metabolites
Some metabolomic investigations reported increased levels of lipids (LDL and VLDL) and several fatty acids in PE patients in comparison with normal pregnancies (Kenny et al. Citation2010; Austdal et al. Citation2014). This is in line with other reports that showed altered lipid metabolism in pregnancies of women who develop hypertensive disorders of pregnancy (Enaruna et al. Citation2014; Keshavarz et al. Citation2017). Maternal serum lipid levels are elevated in healthy pregnancies and are higher in preeclamptic women (Diareme et al. Citation2009; Lima et al. Citation2011). These lipid alterations are likely because of increased level of estrogens during the gestation period that diminish the activity of hepatic lipase (HL) (Jones et al. Citation2002). Abnormal lipid metabolism may elevate the risk of vascular disorders, which induce endothelial dysfunction and cardiovascular disorders in PE (Gofman et al. Citation2007; Nelson Citation2013). Furthermore, carnitine plays a key role in lipid transport. Thiele et al. reported that total, short-, and long-chain acylcarnitines (except medium-chain acylcarnitines) is elevated in PE patients (Thiele et al. Citation2004). In accordance with these observations, a metabolomics study that was performed by Koster et al. (Citation2015) showed that acylcarnitines may be predictive markers for early identification of PE patients during the first trimester of pregnancy. There is a positive correlation between diastolic blood pressure and both total and short-chain acylcarnitines (Thiele et al. Citation2004). The increase in serum carnitine and its acylcarnitines levels in PE are not fully clear. However, there are two explanations for that. First, downregulation of the expression and activity of placental cytochrome c oxidase, a mitochondrial respiratory chain enzyme, in women with PE may lead to impaired mitochondrial energy production (Zehua et al. Citation1999; He et al. Citation2004). Second, hypoxia (due to impaired placentation) may downregulate the expression and activity of organic cation/carnitine transporter (OCTN2) in human trophoblasts (Rytting and Audus Citation2007). As a result, decreased placental transfer of carnitine to the fetus in the PE patients could ultimately result in the enhanced levels of maternal carnitine and acylcarnitines.
Alterations in the concentration of polyunsaturated fatty acids in PE patients were reported. An increase in arachidonate in placenta and mitochondrial placenta of PE patients was shown. This metabolite may produce prostaglandins (vasodilators/vasoconstrictors) and leukotrienes (inflammatory mediator). Furthermore, arachidonate promotes apoptosis and its accumulation in mitochondria can damage these organelles, thus resulting in reduced β-oxidation and the accumulation of fatty acids occur (Zhou et al. Citation2017).
Other polyunsaturated fatty acids such as docosapentaenoate and docosahexaenoate increased in mitochondrial placenta and plasma of PE patients, respectively (Kenny et al. Citation2010; Zhou et al. Citation2017). Reports indicate that these metabolites can inhibit angiogenesis through limiting vascular endothelial growth factor (Tsuji et al. Citation2003; Chao et al. Citation2014). Therefore, these metabolites may be the cause of damaged endothelial remodeling as observed in PE patients.
Amino-acid metabolism
The levels of several amino acids are altered during PE. For instance, higher concentrations of degradation products from branched chain amino acids (BCAA), 3-methyl-2-oxovaleric acid, 3-hydroxyisobutyrate, and 3-hydroxyisovalerate, in blood/urine have been reported (Kenny et al. Citation2010; Bahado-Singh et al. Citation2012, Citation2015; Austdal et al. Citation2015a). This may correlate with the elevated consumption of BCAA in PE patients, as reported previously in Intrauterine Growth Restriction (IUGR) (Cetin et al. Citation1988).
Another important amino acid that is reduced in e-PE compared to l-PE was arginine (Mukherjee et al. Citation2014). Arginine (Arg) produces a vasodilatory effect by elevating nitric oxide (NO) production through Arg–NO pathway (Hishikawa et al. Citation1993). The studies showed that Arg supplementation can reduce blood pressure and PE (Altun et al. Citation2008; Vadillo-Ortega et al. Citation2011). This finding suggests that Arg may play important role in the pathogenesis of PE. Further investigations are needed to clarify if these alterations are the cause or effect of PE.
Inflammation and oxidative stress pathways
Another important metabolite is taurine that has antihypertensive and vasoprotective effects (Wójcik et al. Citation2010). Taurine is derived from cysteine during fetal development (Chen et al. Citation1998). Reduced activity of taurine transporters in the placental tissue in PE has been shown (Desforges et al. Citation2013a). Decreases in placental taurine levels could impair placental trophoblast invasion of the maternal spiral arteries (Desforges et al. Citation2013b) and reduce nutrient transfer to the fetus (Desforges et al. Citation2013a) as well as produce relative hypoxia (Roberts et al. Citation1989; Reuvekamp et al. Citation1999). Subsequently, pro-inflammatory cytokines are upregulated under hypoxic condition (Ghezzi et al. Citation1991; Klausen et al. Citation1997). There is a positive correlation between inflammation and oxidative stress which are the features of PE and may induce endothelial dysfunction (Hubel Citation1999; Borzychowski et al. Citation2006; Sánchez-Aranguren et al. Citation2014). This finding is in accordance with two studies that showed blood uric acid, the end product of purine catabolism, and increase in PE patients (Kenny et al. Citation2008; Senyavina et al. Citation2013). Under hypoxia condition, the degradation of purine nucleotides increases (Braghiroli et al. Citation1993). Increased levels of serum uric acid are related to high blood pressure and hypertension (Scheepers et al. Citation2017).
The increased levels of blood ketone bodies (Kenny et al. Citation2010; Bahado-Singh et al. Citation2015, Citation2017b) (3-hydroxybutyrate, acetoacetate and acetone) concurrent with decreased levels of serum pyruvate (Austdal et al. Citation2015a) in PE patients may suggest a metabolism conversion from glycolysis to ketosis for energy production (Laffel Citation1999).
Coagulation and angiogenesis pathways
Although the etiology of PE remains unclear, the coagulation and angiogenic pathways are dysfunctional in PE (Manten et al. Citation2003; Williams et al. Citation2007; Kårehed et al. Citation2010). One of important proteins that is involved in angiogensis is histidine-rich glycoprotein (HRG) (Simantov et al. Citation2001). A decreased level of this protein that can be incorporated in coagulation system and angiogenic pathway has been reported in patients with PE (Bolin et al. Citation2011). In agreement with these findings, metabolomic studies showed elevated excretion of histidine in urine and the levels of this metabolite decreased in serum of PE patients (Austdal et al. Citation2014, Citation2015a). Of note, two types of histidine (histidine in glycoproteins and free histidine) are different, but their metabolism can be relevant.
One of risk factors for PE is obesity (Walsh Citation2007). As mentioned above, some maternal properties such as BMI in combination with metabolomic and proteomic biomarkers are able to predict PE with high accuracy (Bahado-Singh et al. Citation2017a). The obese subjects have a reduced level of vitamin D in serum that it is likely because of its deposition in body fat (Wortsman et al. Citation2000). Moreover, a meta-analysis (Wei et al. Citation2013) showed that maternal vitamin D deficiency can increase the prevalence of PE via elevated pro-inflammatory response, OS, and endothelial dysfunction (Tarcin et al. Citation2009; Jablonski et al. Citation2011). Therefore, vitamin D supplementation is recommended for reduction of PE incidence.
Reports showed increased risk of cardiovascular and kidney diseases [end-stage renal disease (ESRD)] after PE (Vikse et al. Citation2008; Berks et al. Citation2013). Interestingly, there are various similarities between the metabolites identified in the PE literature and those reported in studies of kidney disease including acylcarnitines, urate, cresol sulfate, and hippurate (increased cresol sulfate and hippurate were in the second half of gestation) (Kenny et al. Citation2008; Senyavina et al. Citation2013; Austdal et al. Citation2014; Niewczas et al. Citation2014; Koster et al. Citation2015), as well as triglycerides, fatty acids, and carnitine that are associated with inflammatory disorders and cardiovascular disease (Famularo et al. Citation2004; Ruiz-Núñez et al. Citation2016). These relationships may clarify the pathophysiology among these disorders.
It should be noted that there are inconsistencies in the reported metabolites among these studies. There are several explanations for these differences including, age, and gestational age at the time blood sampling, parity, obesity, smoking, ethnic origin, sort and severity of disease, status of nutrition, different analytical platforms (e.g., NMR, GC– and LC–MS), and various statistical analyses which may influence the calculation of the composition of metabolites can all impact the results. Besides these heterogeneities that make it difficult to compare the results from the different investigations, there are other limitations highlighted in this review. These include the lack of validation for identified metabolic biomarkers that may introduce bias to the biological interpretation of the results. Other biases such as publication bias, the tendency in reporting and publishing only positive findings, or experimental bias although minimized may also affect results.
Despite decades of research on PE, there are no predictive biomarkers to early diagnosis of women at risk of PE to reduce health care costs and avoid dispensable hospitalization of pregnant women with suspected PE. Regarding the complexity and heterogeneity of PE, it is unlikely to find a single biomarker that could predict all sorts of PE disorders. Although lots of predictive markers have been investigated hitherto, none of them were selected as predictive or diagnostic biomarkers for PE based on WHO definition. Therefore, further studies may be necessary to recognize a combination of predictive and diagnostic biomarkers using ‘omic’ technologies such as metabolomics and proteomics that can identify multiple markers simultaneously. Metabolomics studies showed that several metabolomic markers such as lipids and amino acids and their derivatives are promising, especially when these metabolites are combined with maternal characteristics and other ‘omic’ approaches. The most commonly identified small molecules are involved in metabolic pathways such as inflammation, oxidative stress, coagulation, and angiogenesis. Further research is necessary to confirm.
Disclosure statement
None declared.
Additional information
Funding
Notes on contributors
B. Fatemeh Nobakht M. Gh
B. Fatemeh Nobakht M. Gh Conceived the study, carried out the literature search, and wrote the manuscript: BFNMG.
References
- Altun ZS, Uysal S, Guner G, Yilmaz O, Posaci C. 2008. Effects of oral L‐arginine supplementation on blood pressure and asymmetric dimethylarginine in stress‐induced preeclamptic rats. Cell Biochem Funct. 26(5):648–653.
- Austdal M, Skråstad RB, Gundersen AS, Austgulen R, Iversen A-C, Bathen TF. 2014. Metabolomic biomarkers in serum and urine in women with preeclampsia. PLoS One. 9(3):e91923.
- Austdal M, Tangerås LH, Skråstad RB, Salvesen K, Austgulen R, Iversen A-C, Bathen TF. 2015a. First trimester urine and serum metabolomics for prediction of preeclampsia and gestational hypertension: a prospective screening study. Int J Mol Sci. 16(9):21520–21538.
- Austdal M, Thomsen LCV, Tangerås LH, Skei B, Mathew S, Bjørge L, Austgulen R, Bathen TF, Iversen A-C. 2015b. Metabolic profiles of placenta in preeclampsia using HR-MAS MRS metabolomics. Placenta. 36(12):1455–1462.
- Bahado-Singh R, Poon LC, Yilmaz A, Syngelaki A, Turkoglu O, Kumar P, Kirma J, Allos M, Accurti V, Li J, et al. 2017a. Integrated proteomic and metabolomic prediction of term preeclampsia. Sci Rep. 7(1):16189.
- Bahado-Singh RO, Akolekar R, Mandal R, Dong E, Xia J, Kruger M, Wishart DS, Nicolaides K. 2012. Metabolomics and first-trimester prediction of early-onset preeclampsia. J Matern Fetal Neonatal Med. 25(10):1840–1847. eng.
- Bahado-Singh RO, Akolekar R, Mandal R, Dong E, Xia J, Kruger M, Wishart DS, Nicolaides K. 2013. First-trimester metabolomic detection of late-onset preeclampsia. Am J Obstet Gynecol. 208(1):58.e1–7.
- Bahado-Singh RO, Syngelaki A, Akolekar R, Mandal R, Bjondahl TC, Han B, Dong E, Bauer S, Alpay-Savasan Z, Graham S. 2015. Validation of metabolomic models for prediction of early-onset preeclampsia. Am J Obstet Gynecol. 213(4):530.e531–530. e510.
- Bahado-Singh RO, Syngelaki A, Mandal R, Graham SF, Akolekar R, Han B, Bjondahl TC, Dong E, Bauer S, Alpay-Savasan Z, et al. 2017b. Metabolomic determination of pathogenesis of late-onset preeclampsia. J Matern Fetal Neonatal Med. 30(6):658–664. eng.
- Berks D, Hoedjes M, Raat H, Duvekot J, Steegers E, Habbema J. 2013. Risk of cardiovascular disease after pre‐eclampsia and the effect of lifestyle interventions: a literature‐based study. BJOG: Int J Obstetrics Gynaecol. 120(8):924–931.
- Bolin M, Åkerud P, Hansson A, Åkerud H. 2011. Histidine-rich glycoprotein as an early biomarker of preeclampsia. Am J Hypertens. 24(4):496–501.
- Borzychowski AM, Sargent IL, Redman CWG. 2006. Inflammation and pre-eclampsia. Semin Fetal Neonatal Med. 11(5):309–316.
- Braghiroli A, Sacco C, Erbetta M, Ruga V, Donner CF. 1993. Overnight urinary uric acid: creatinine ratio for detection of sleep hypoxemia. Am Rev Respir Dis. 148(1):173–178.
- Cetin I, Marconi AM, Bozzetti P, Sereni LP, Corbetta C, Pardi G, Battaglia FC. 1988. Imbilical amino acid concentrations in appropriate and small for gestational age infants: a biochemical difference present in utero. Am J Obstetrics Gynecol. 158(1):120–126.
- Chao C-Y, Lii C-K, Ye S-Y, Li -C-C, Lu C-Y, Lin A-H, Liu K-L, Chen H-W. 2014. Docosahexaenoic acid inhibits vascular endothelial growth factor (VEGF)-induced cell migration via the GPR120/PP2A/ERK1/2/eNOS signaling pathway in human umbilical vein endothelial cells. J Agric Food Chem. 62(18):4152–4158.
- Chen T, He P, Tan Y, Xu D. 2017. Biomarker identification and pathway analysis of preeclampsia based on serum metabolomics. Biochem Biophys Res Commun. 485(1):119–125. eng.
- Chen X-C, Pan Z-L, Liu D-S, Han X. 1998. Effect of taurine on human fetal neuron cells: proliferation and differentiation. Taurine. 3:397–403.
- Cnossen JS, Riet GT, Mol BW, Van Der Post JA, Leeflang MM, Meads CA, Hyde C, Khan KS. 2009. Are tests for predicting pre‐eclampsia good enough to make screening viable? A review of reviews and critical appraisal. Acta Obstet Gynecol Scand. 88(7):758–765.
- Desforges M, Ditchfield A, Hirst CR, Pegorie C, Martyn-Smith K, Sibley CP, Greenwood SL. 2013a. Reduced placental taurine transporter (TauT) activity in pregnancies complicated by pre-eclampsia and maternal obesity. Taurine. 8:81–91.
- Desforges M, Parsons L, Westwood M, Sibley C, Greenwood S. 2013b. Taurine transport in human placental trophoblast is important for regulation of cell differentiation and survival. Cell Death Dis. 4(3):e559.
- Diareme M, Karkalousos P, Theodoropoulos G, Strouzas S, Lazanas N. 2009. Lipid profile of healthy women during normal pregnancy. J Med Biochem. 28(3):152–160.
- Diaz SO, Barros AS, Goodfellow BJ, Duarte IF, Galhano E, Pita C, Almeida Mdo C, Carreira IM, Gil AM. 2013. Second trimester maternal urine for the diagnosis of trisomy 21 and prediction of poor pregnancy outcomes. J Proteome Res. 12(6):2946–2957. eng.
- Drake SK, Bowen RA, Remaley AT, Hortin GL. 2004. Potential interferences from blood collection tubes in mass spectrometric analyses of serum polypeptides. Clinic Chem. 50(12):2398–2401.
- Dunn WB, Brown M, Worton SA, Davies K, Jones RL, Kell DB, Heazell AEP. 2012. The metabolome of human placental tissue: investigation of first trimester tissue and changes related to preeclampsia in late pregnancy. Metabolomics. 8(4):579–597.
- Dunn WB, Ellis DI. 2005. Metabolomics: current analytical platforms and methodologies. TrAC Trends Anal Chem. 24(4):285–294.
- Emwas A-H, Luchinat C, Turano P, Tenori L, Roy R, Salek RM, Ryan D, Merzaban JS, Kaddurah-Daouk R, Zeri AC. 2015. Standardizing the experimental conditions for using urine in NMR-based metabolomic studies with a particular focus on diagnostic studies: a review. Metabolomics. 11(4):872–894.
- Emwas A-HM, Salek RM, Griffin JL, Merzaban J. 2013. NMR-based metabolomics in human disease diagnosis: applications, limitations, and recommendations. Metabolomics. 9(5):1048–1072.
- Enaruna NO, Idemudia JO, Aikoriogie PI. 2014. Serum lipid profile and uric acid levels in preeclampsia in University of Benin Teaching Hospital. Niger Med J. 55(5):423.
- Famularo G, Simone C, Trinchieri V, Mosca L. 2004. Carnitines and its congeners: a metabolic pathway to the regulation of immune response and inflammation. Ann N Y Acad Sci. 1033(1):132–138.
- Ghezzi P, Dinarello CA, Bianchi M, Rosandich ME, Repine JE, White CW. 1991. Hypoxia increases production of interleukin-1 and tumor necrosis factor by human mononuclear cells. Cytokine. 3(3):189–194.
- Ghoochani BFNM, Aliannejad R, Oskouie AA, Rezaei-Tavirani M, Kalantari S, Naseri MT, Baghban AA, Parastar H, Aliakbarzadeh G. 2018. Metabolomics diagnostic approach to mustard airway diseases: a preliminary study. Iran J Basic Med Sci. 21(1):59.
- Gofman JW, Delalla O, Glazier F, Freeman NK, Lindgren FT, Nichols AV, Strisower B, Tamplin AR. 2007. The serum lipoprotein transport system in health, metabolic disorders, atherosclerosis and coronary heart disease. J Clin Lipidol. 1(2):104–141.
- He L, Wang Z, Sun Y. 2004. Reduced amount of cytochrome c oxidase subunit I messenger RNA in placentas from pregnancies complicated by preeclampsia. Acta Obstet Gynecol Scand. 83(2):144–148.
- Hishikawa K, Nakaki T, Suzuki H, Kato R, Saruta T. 1993. Role of L-arginine-nitric oxide pathway in hypertension. J Hypertens. 11(6):639–645.
- Hubel CA. 1999. Oxidative stress in the pathogenesis of preeclampsia. Proc Soc Exp Biol Med. 222(3):222–235.
- Jablonski KL, Chonchol M, Pierce GL, Walker AE, Seals DR. 2011. 25-Hydroxyvitamin D deficiency is associated with inflammation-linked vascular endothelial dysfunction in middle-aged and older adults. Hypertension. 57(1):63–69.
- Jain S, Jayasimhulu K, Clark JF. 2004. Metabolomic analysis of molecular species of phospholipids from normotensive and preeclamptic human placenta electrospray ionization mass spectrometry. Front Biosci: J Virtual Library. 9:3167–3175. eng.
- Jones DR, Schmidt RJ, Pickard RT, Foxworthy PS, Eacho PI. 2002. Estrogen receptor-mediated repression of human hepatic lipase gene transcription. J Lipid Res. 43(3):383–391.
- Kalantari S, Nafar M, Samavat S, Parvin M, Gh NM, Fatemeh B, Barzi F. 2016. 1H NMR‐based metabolomics exploring urinary biomarkers correlated with proteinuria in focal segmental glomerulosclerosis: a pilot study. Magn Reson Chem. 54(10):821–826.
- Kårehed K, Wikström AK, Olsson AK, Larsson A, Olovsson M, Åkerud H. 2010. Fibrinogen and histidine‐rich glycoprotein in early‐onset preeclampsia. Acta Obstet Gynecol Scand. 89(1):131–139.
- Kenny LC, Broadhurst D, Brown M, Dunn WB, Redman CW, Kell DB, Baker PN. 2008. Detection and identification of novel metabolomic biomarkers in preeclampsia. Reprod Sci. 15(6):591–597.
- Kenny LC, Broadhurst DI, Dunn W, Brown M, North RA, McCowan L, Roberts C, Cooper GJ, Kell DB, Baker PN. 2010. Robust early pregnancy prediction of later preeclampsia using metabolomic biomarkers. Hypertension. 56(4):741–749.
- Kenny LC, Dunn WB, Ellis DI, Myers J, Baker PN, Kell DB, Consortium G. 2005. Novel biomarkers for pre-eclampsia detected using metabolomics and machine learning. Metabolomics. 1(3):227–234.
- Keshavarz P, Gh BFNM, Mirhafez SR, Nematy M, Azimi-Nezhad M, Afin SA, Esmaily H, Pourali L, Hakak AM, Soukhtanloo M. 2017. Alterations in lipid profile, zinc and copper levels and superoxide dismutase activities in normal pregnancy and preeclampsia. Am J Med Sci. 353(6):552–558.
- Klausen T, Olsen NV, Poulsen TD, Richalet J-P, Pedersen BK. 1997. Hypoxemia increases serum interleukin-6 in humans. Eur J Appl Physiol. 76(5):480–482.
- Koster MP, Vreeken RJ, Harms AC, Dane AD, Kuc S, Schielen PC, Hankemeier T, Berger R, Visser GH, Pennings JL. 2015. First-trimester serum acylcarnitine levels to predict preeclampsia: a metabolomics approach. Dis Mark. 2015:857108. eng.
- Kuc S, Koster MP, Pennings JL, Hankemeier T, Berger R, Harms AC, Dane AD, Schielen PC, Visser GH, Vreeken RJ. 2014. Metabolomics profiling for identification of novel potential markers in early prediction of preeclampsia. PLoS One. 9(5):e98540. eng.
- Laffel L. 1999. Ketone bodies: a review of physiology, pathophysiology and application of monitoring to diabetes. Diabetes Metab Res Rev. 15(6):412–426.
- Lauridsen M, Hansen SH, Jaroszewski JW, Cornett C. 2007. Human urine as test material in 1H NMR-based metabonomics: recommendations for sample preparation and storage. Anal Chem. 79(3):1181–1186.
- Lima V, Andrade C, Ruschi GE, Sass N. 2011. Serum lipid levels in pregnancies complicated by preeclampsia. Sao Paulo Med J. 129(2):73–76.
- Manten G, Sikkema J, Franx A, Hameeteman T, Visser G, De Groot P, Voorbij H. 2003. Increased high molecular weight fibrinogen in pre-eclampsia. Thromb Res. 111(3):143–147.
- Monteiro M, Carvalho M, Bastos M, Guedes de Pinho P. 2013. Metabolomics analysis for biomarker discovery: advances and challenges. Curr Med Chem. 20(2):257–271.
- Mukherjee R, Ray CD, Ray S, Dasgupta S, Chaudhury K. 2014. Altered metabolic profile in early and late onset preeclampsia: an FTIR spectroscopic study. Pregnancy Hypertens. 4(1):70–80. eng.
- Nelson RH. 2013. Hyperlipidemia as a risk factor for cardiovascular disease. Primary Care. 40(1):195.
- Nicholson JK, Lindon JC. 2008. Systems biology: metabonomics. Nature. 455(7216):1054.
- Niewczas MA, Sirich TL, Mathew AV, Skupien J, Mohney RP, Warram JH, Smiles A, Huang X, Walker W, Byun J. 2014. Uremic solutes and risk of end-stage renal disease in type 2 diabetes: metabolomic study. Kidney Int. 85(5):1214–1224.
- Nobakht BF, Aliannejad R, Rezaei-Tavirani M, Arefi Oskouie A, Naseri MT, Parastar H, Aliakbarzadeh G, Fathi F, Taheri S. 2016a. NMR-and GC/MS-based metabolomics of sulfur mustard exposed individuals: a pilot study. Biomarkers. 21(6):479–489.
- Nobakht BF, Arefi Oskouie A, Rezaei-Tavirani M, Aliannejad R, Taheri S, Fathi F, Taghi Naseri M. 2017. NMR spectroscopy-based metabolomic study of serum in sulfur mustard exposed patients with lung disease. Biomarkers. 22(5):413–419.
- Nobakht M, Aliannejad R, Oskouie A, Fathi F, Safakhah H, Rezaei-Tavirani M. 2016b. Nuclear magnetic resonance-based metabolomics analysis of patients exposed to sulfur mustard in different stages using random forest method. Koomesh. 17:3.
- Nobakht MGBF, Aliannejad R, Rezaei-Tavirani M, Taheri S, Oskouie AA. 2015. The metabolomics of airway diseases, including COPD, asthma and cystic fibrosis. Biomarkers. 20(1):5–16.
- Odibo AO, Goetzinger KR, Odibo L, Cahill AG, Macones GA, Nelson DM, Dietzen DJ. 2011. First‐trimester prediction of preeclampsia using metabolomic biomarkers: a discovery phase study. Prenat Diagn. 31(10):990–994.
- Polsani S, Phipps E, Jim B. 2013. Emerging new biomarkers of preeclampsia. Adv Chronic Kidney Dis. 20(3):271–279.
- Poon L, Kametas N, Chelemen T, Leal A, Nicolaides K. 2010. Maternal risk factors for hypertensive disorders in pregnancy: a multivariate approach. J Hum Hypertens. 24(2):104.
- Reuvekamp A, Velsing‐Aarts FV, Poulina IE, Capello JJ, Duits AJ. 1999. Selective deficit of angiogenic growth factors characterises pregnancies complicated by pre‐eclampsia. BJOG: Int J Obstetrics Gynaecol. 106(10):1019–1022.
- Roberts JM, Taylor RN, Musci TJ, Rodgers GM, Hubel CA, McLaughlin MK. 1989. Preeclampsia: an endothelial cell disorder. Am J Obstet Gynecol. 161(5):1200–1204.
- Ruiz-Núñez B, Dijck-Brouwer DJ, Muskiet FA. 2016. The relation of saturated fatty acids with low-grade inflammation and cardiovascular disease. J Nutr Biochem. 36:1–20.
- Rytting E, Audus KL. 2007. Effects of low oxygen levels on the expression and function of transporter OCTN2 in BeWo cells. J Pharm Pharmacol. 59(8):1095–1102.
- Sánchez-Aranguren LC, Prada CE, Riaño-Medina CE, Lopez M. 2014. Endothelial dysfunction and preeclampsia: role of oxidative stress. Front Physiol. 5:1–11.
- Scalbert A, Brennan L, Fiehn O, Hankemeier T, Kristal BS, van Ommen B, Pujos-Guillot E, Verheij E, Wishart D, Wopereis S. 2009. Mass-spectrometry-based metabolomics: limitations and recommendations for future progress with particular focus on nutrition research. Metabolomics. 5(4):435.
- Scheepers LE, Boonen A, Pijnenburg W, Bierau J, Staessen JA, Stehouwer CD, Thijs C, Arts IC. 2017. Associations of plasma uric acid and purine metabolites with blood pressure in children: the KOALA Birth Cohort Study. J Hypertens. 35(5):982–993.
- Senyavina NV, Khaustova SA, Grebennik TK, Pavlovich SV. 2013. Analysis of purine metabolites maternal serum for evaluating the risk of gestosis. Bull Exp Biol Med. 155(5):682–684.
- Sibai B, Dekker G, Kupferminc M. 2005. Pre-eclampsia. Lancet. 365(9461):785–799.
- Sibai BM. 1992. Hypertension in pregnancy. Obstet Gynecol Clin North Am. 19(4):615–632.
- Sibai BM, Gordon T, Thom E, Caritis SN, Klebanoff M, McNellis D, Paul RH. 1995. Risk factors for preeclampsia in healthy nulliparous women: a prospective multicenter study. Am J Obstet Gynecol. 172(2):642–648.
- Simantov R, Febbraio M, Crombie R, Asch AS, Nachman RL, Silverstein RL. 2001. Histidine-rich glycoprotein inhibits the antiangiogenic effect of thrombospondin-1. J Clin Invest. 107(1):45.
- Slupsky CM, Rankin KN, Wagner J, Fu H, Chang D, Weljie AM, Saude EJ, Lix B, Adamko DJ, Shah S. 2007. Investigations of the effects of gender, diurnal variation, and age in human urinary metabolomic profiles. Anal Chem. 79(18):6995–7004.
- Staff AC. 2011. Circulating predictive biomarkers in preeclampsia. Pregnancy Hypertens. 1(1):28–42.
- Tarcin O, Yavuz DG, Ozben B, Telli A, Ogunc AV, Yuksel M, Toprak A, Yazici D, Sancak S, Deyneli O. 2009. Effect of vitamin D deficiency and replacement on endothelial function in asymptomatic subjects. J Clin Endocrinol Metabol. 94(10):4023–4030.
- Thiele IG, Niezen-Koning KE, van Gennip AH, Aarnoudse JG. 2004. Increased plasma carnitine concentrations in preeclampsia. Obstet Gynecol. 103(5, Part 1):876–880.
- Tsuji M, Murota S-I, Morita I. 2003. Docosapentaenoic acid (22: 5, n-3) suppressed tube-forming activity in endothelial cells induced by vascular endothelial growth factor. Prostaglandins Leukot Essent Fatty Acids. 68(5):337–342.
- Vadillo-Ortega F, Perichart-Perera O, Espino S, Avila-Vergara MA, Ibarra I, Ahued R, Godines M, Parry S, Macones G, Strauss JF. 2011. Effect of supplementation during pregnancy with L-arginine and antioxidant vitamins in medical food on pre-eclampsia in high risk population: randomised controlled trial. BMJ. 342:d2901.
- Vikse BE, Irgens LM, Leivestad T, Skjærven R, Iversen BM. 2008. Preeclampsia and the risk of end-stage renal disease. New England J Med. 359(8):800–809.
- Walsh SW. 2007. Obesity: a risk factor for preeclampsia. Trends Endocrinol Metabol. 18(10):365–370.
- Wang JH, Byun J, Pennathur S. 2010. Analytical approaches to metabolomics and applications to systems biology. Semin Nephrol. 30(5):500–511.
- Wei S-Q, Qi H-P, Luo Z-C, Fraser WD. 2013. Maternal vitamin D status and adverse pregnancy outcomes: a systematic review and meta-analysis. J Matern Fetal Neonatal Med. 26(9):889–899.
- Wikström A-K, Larsson A, Eriksson UJ, Nash P, Nordén-Lindeberg S, Olovsson M. 2007. Placental growth factor and soluble FMS-like tyrosine kinase-1 in early-onset and late-onset preeclampsia. Obstet Gynecol. 109(6):1368–1374.
- Williams VK, Griffiths AB, Carbone S, Hague WM. 2007. Fibrinogen concentration and factor VIII activity in women with preeclampsia. Hypertens Pregnan. 26(4):415–421.
- Wishart DS, Knox C, Guo AC, Eisner R, Young N, Gautam B, Hau DD, Psychogios N, Dong E, Bouatra S. 2008. HMDB: a knowledgebase for the human metabolome. Nucl Acids Res. 37(suppl_1):D603–D610.
- Wójcik OP, Koenig KL, Zeleniuch-Jacquotte A, Costa M, Chen Y. 2010. The potential protective effects of taurine on coronary heart disease. Atherosclerosis. 208(1):19–25.
- Wortsman J, Matsuoka LY, Chen TC, Lu Z, Holick MF. 2000. Decreased bioavailability of vitamin D in obesity–. Am J Clin Nutr. 72(3):690–693.
- Yanes O, Tautenhahn R, Patti GJ, Siuzdak G. 2011. Expanding coverage of the metabolome for global metabolite profiling. Anal Chem. 83(6):2152–2161.
- Zehua W, Guanglan Z, Meihua L. 1999. Mitochondrial tRNA leu (UUR) gene mutation and the decreased activity of cytochrome c oxidase in preeclampsia. J Huazhong Univ Sci Technol Med Sci. 19(3):209–211.
- Zhou X, Han TL, Chen H, Baker PN, Qi H, Zhang H. 2017. Impaired mitochondrial fusion, autophagy, biogenesis and dysregulated lipid metabolism is associated with preeclampsia. Exp Cell Res. 359(1):195–204. eng.