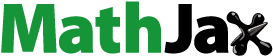
ABSTRACT
Statistical relationships between surface ozone (O3) concentration, precursor species and meteorological conditions in continental South Africa were examined from data obtained from measurement stations in north-eastern South Africa. Three multivariate statistical methods were applied in the investigation, i.e. multiple linear regression (MLR), principal component analysis (PCA) and –regression (PCR), and generalised additive model (GAM) analysis. The daily maximum 8-h moving average O3 concentrations were considered in these statistical models (dependent variable). MLR models indicated that meteorology and precursor species concentrations are able to explain ~50% of the variability in daily maximum O3 levels. MLR analysis revealed that atmospheric carbon monoxide (CO), temperature and relative humidity were the strongest factors affecting the daily O3 variability. In summer, daily O3 variances were mostly associated with relative humidity, while winter O3 levels were mostly linked to temperature and CO. PCA indicated that CO, temperature and relative humidity were not strongly collinear. GAM also identified CO, temperature and relative humidity as the strongest factors affecting the daily variation of O3. Partial residual plots found that temperature, radiation and nitrogen oxides most likely have a non-linear relationship with O3,while the relationship with relative humidity and CO is probably linear. An inter-comparison between O3 levels modelled with the three statistical models compared to measured O3 concentrations showed that the GAM model offered a slight improvement over the MLR model. These findings emphasise the critical role of regional-scale O3 precursors coupled with meteorological conditions in daily variances of O3 levels in continental South Africa.
1. Introduction
Surface O3 is a secondary pollutant, which is considered a relatively short-lived (lifetime ranging between days to weeks) greenhouse gas (Ordonez et al. Citation2005). In general, high surface O3 concentrations are a concern because of its detrimental impacts on human health and ecosystem functioning (NRC Citation2008). The potential for O3 damage to plants is, especially, a concern when agricultural yields are reduced, which threatens the food security and economies of countries that rely strongly on agricultural production. However, an important consequence of plant damage caused by increased O3 levels relate to the reduced removal of CO2 in the atmosphere and thereby O3 also indirectly contributes to climate change. In addition, tropospheric O3 can also affect new particle formation in the atmosphere (e.g. Mikkonen et al. Citation2011), which also impacts climate change directly (e.g. scattering) and indirectly (e.g. cloud formation).
O3 in the troposphere is produced by the photochemical oxidation of nitrogen dioxide (NO2):
The photolytically formed O3 reacts with NO to regenerate NO2:
This is a continuous process termed the NOx-dependent photo-stationary state (PSS), which results in no net O3 production (Seinfeld and Pandis Citation2006; Awang et al. Citation2018). However, when this PSS is altered in the presence of carbon monoxide (CO) and volatile organic compounds (VOCs), net O3 production occurs. High O3 levels are not only a result of chemistry associated with precursor emissions but are also related to meteorological conditions conducive to the formation, transport and removal of air pollutants (Melkonyan and Kuttler Citation2012). Local meteorological parameters, such as temperature, relative humidity, sunlight, and wind speed and -direction play a significant role in O3 variability (Ooka et al. Citation2011; Tsakiri and Zurbenko Citation2011). These multiple factors influencing surface O3 levels have confounded the effect of individual parameters on ground-level O3, thereby making it challenging to separate the impacts of local emissions, meteorology and transport on surface O3 concentrations (Gorai et al. Citation2015).
Statistical models relating ambient O3 concentrations to meteorological variables have been developed for the purpose of the prediction of O3 concentrations, the estimation of long-term O3 trends, as well as explaining the underlying chemical and meteorological processes affecting O3 concentrations (Thompson et al. Citation2001). Some of these statistical methods were critically reviewed by Thompson et al. (Citation2001), which included regression-based methods (Fiore et al. Citation1998; Abdul-Wahab et al. Citation2005; Ooka et al. Citation2011), time-series filtering (Rao and Zurbenko Citation1994; Milanchus et al. Citation1998; Tsakiri and Zurbenko Citation2011), multivariate statistical techniques such as cluster analysis and principal component analysis (PCA) (Abdul-Wahab et al. Citation2005; Melkonyan and Kuttler Citation2012; Dominick et al. Citation2012; Awang et al. Citation2015), as well as neural networks (Comrie Citation1997; Gardner and Dorling Citation1998, Citation2000; Guardani et al. Citation2003). The most widely used statistical technique to relate O3 concentrations to influencing factors is linear regression, because of its user-friendliness and straightforward interpretability (Comrie Citation1997; Cardelino et al. Citation2001). However, the relationship between O3 levels and certain meteorological effects is typically non-linear, while some explanatory variables are collinear (Neter et al. Citation1996). Although non-linear regression models for O3 forecasting have been developed (Bloomfield et al. Citation1996; Thompson et al. Citation2001; Lin and Cobourn Citation2007), these models are difficult to interpret and explain in summarized form to the public (Thompson et al. Citation2001; Pearce et al. Citation2011). However, generalized additive models (GAM), which are an extension of linear regression, are able to handle non-linear associations between atmospheric parameters and are simpler to interpret or justify (Hastie and Tibshirani Citation1990). Melkonyan and Kuttler (Citation2012) suggested that PCA is the most appropriate method to identify multivariate relationships between pollutants and meteorological factors.
Southern Africa is the largest industrialized region in Africa, where high O3 levels may be expected due to the high rate of precursor emissions from anthropogenic sources, coupled with the abundance of sunlight throughout the year (Zunckel et al., 2006). In addition, this region is also influenced by large-scale open biomass burning, which is considered to be a significant source of O3 precursor species. Laban et al. (Citation2018) indicated that CO emissions associated with biomass burning (household combustion and open biomass burning) contributed significantly to high O3 levels, while it was also indicated that large parts of the regional background in South Africa can be considered VOC-limited. Although the temporal and spatial variability is generally attributed to meteorological conditions and/or precursor emissions, the response of O3 with respect to changing emission levels and meteorological fluctuations is not well understood for this region (Laban et al. Citation2018). Therefore, the aim of this study was to utilize statistical models to distinguish the complex effects of meteorological parameters and precursor emissions influencing O3 chemistry and concentrations in continental South Africa, as well as to quantify the strength of association of O3 with these factors in order to better understand the underlying mechanisms responsible for the changes in surface O3 levels in this region.
2. Material and methods
2.1. Description of the study area
Data from continuous in-situ measurements conducted at four measurement sites (indicated in ) in the north-eastern interior of South Africa were obtained for statistical analysis. This region is the largest industrial area in South Africa, with substantial emissions of atmospheric pollutants from anthropogenic activities, e.g. industries, domestic fuel burning and vehicles (Lourens et al. Citation2011, Citation2012). A combination of meteorology and anthropogenic activities has amplified pollution levels within the region. Detailed descriptions of the locations of these four measurement stations and their surroundings are provided in Laban et al. (Citation2018).
Table 1. Measurement stations from which meteorological- and air pollutant data utilized for statistical analysis were obtained
Measurements were conducted from 20 July 2006 until 5 February 2008 at Botsalano, 8 February 2008 to 16 May 2010 at Marikana, 20 May 2010 to 31 December 2015 at Welgegund and 11 February 2009 to 31 December 2010 at Elandsfontein. These four measurement stations represent high quality, high resolution data, which include comprehensive continuous measurements of aerosols, trace gases and meteorological parameters. Data quality was ensured through regular site visits, while data collected from these four sites were subjected to meticulous cleaning (e.g. excluding measurements recorded during calibrations and maintenance). The data were available as 15-min averages.
2.2. Data treatment
Respiratory symptoms have been found to be associated with the daily maximum of the eight-hour average O3 concentration (Schlink et al. Citation2006). Therefore, the South African National Ambient Air Quality Standards and other international standards, designed to protect human health, are based on this metric. Consequently, the daily maximum 8-h moving average O3 concentrations (daily max 8-h O3) were utilized in the statistical analysis (dependent variable). The choice of input (independent) variables for the models was based on literature (Dueñas et al. Citation2002; Ordonez et al. Citation2005; Abdul-Wahab et al. Citation2005; Camalier et al. Citation2007; Awang et al. Citation2015), as well as exploratory analysis and a general understanding of O3-related processes (EquationEquation 1.1(1.1)
(1.1) –Equation1.3)
(1.3)
(1.3) . Daytime (11:00–17:00 local time) daily average concentrations were calculated for NO2, NO and CO, while daily mean values for zonal (u) wind component, meridional (v) wind component, relative humidity and solar radiation were determined. Daily maximum temperatures were included in models. Only daytime measurements were used in the statistical models, since the boundary layer is deep and well mixed during this period, as well as to exclude night-time chemistry (Cooper et al. Citation2012). Other variables such as soil moisture and precipitation, as well as SO2- and H2S levels were also explored, but were found to have only a minor influence on daily max 8-h O3. Since the O3 data utilized in this study were normally distributed, it was not necessary to log-transform the original data to satisfy parametric test assumptions.
Exploratory descriptive statistics (calculation of mean, median, minimum, maximum and standard deviation) were employed prior to the statistical analyses in order to gain a general understanding of meteorological, O3, NOx and CO variations at the measurement locations. Correlation coefficients were also calculated as a measure of the linear relationship between O3 and each variable.
2.3. Statistical methods
Three different statistical methods, namely MLR, PCA and GAM were used to statistically evaluate the datasets. A separate model was built for each measurement site and used to investigate the influence of meteorological and precursor species (indicated in section 2.2.) variability on daily max 8-h O3 at each site. The statistical calculations were performed using MATLAB version R2013a or R software environment (R Development Core Team Citation2009).
2.3.1. Multiple linear regression (MLR)
Multiple linear regression modelling was used to relate O3 concentrations (daily max 8-h O3) to meteorological and pollutant factors, as well as the relative contribution of each of these factors. The general equation for an MLR model is given by
where is the response variable,
are the exploratory variables,
are the regression coefficients, and
is an error term or residual value associated with deviation between the observed value of
and the predicted
value from the regression equation. The ordinary least squares procedure is the standard method to estimate the coefficients in the MLR equation. With this method, the regression procedure is based on finding coefficient values that minimize the sum of the squares of the residuals. A forward stepwise regression procedure was used in which each variable was added individually to the starting model according to their statistical significance and overall increase in the explanation capability of the model. This was done to remove the least important predictor variables and to obtain the optimal combination of variables depending on the statistical indices.
The strength of relationship between each independent variable and O3 was evaluated in terms of the magnitude of the t-statistic and associated p-value for statistical significance. The performance of the model was evaluated with R2, adjusted R2 and root mean square error (RMSE). The adjusted-R2 is an R2 measure that does not increase unless the new variables have additional predictive capability (unlike R2 that increases when variables are added to the equation even when the new variables have no real predictive capability). The optimum MLR models considered had the largest R2 and adjusted R2, and smallest RMSE from a minimum number of independent variables. The main assumptions of the model are true underlying linearity, residuals are mutually independent with constant variance (homoscedasticity), and residuals are normally distributed (Ordonez et al. Citation2005). Multicollinearity in the regression model was verified by examining the variance inflation factor (VIF) for each of the predictor variables (Abdul-Wahab et al. Citation2005; Otero et al. Citation2016).
2.3.2. Principal component analysis (PCA) and -regression (PCR)
Parameters such as solar radiation, temperature and relative humidity are related properties, which could be inessential in MLR. PCA is a statistical procedure that uses an orthogonal transformation to convert a set of interrelated variables into a set of uncorrelated variables, i.e. principal components. Therefore, PCA is able to separate interrelationships (collinearity) into statistically independent basic components (Abdul-Wahab et al. Citation2005) and determine the most important uncorrelated variables. Each principal component is a linear combination of the original predictor variables that account for the variance in the data. All the principal components are orthogonal to each other, which implies that they are uncorrelated to each other. The first principal component is calculated such that it accounts for the highest possible variance in the dataset, followed by the concurrent components. Since the variables are measured in different units, it is necessary to standardize data before a principal component analysis is carried out, which involves scaling every variable to have a mean equal to 0 and a standard deviation equal to 1. The principal component model presents the ith principal component as a linear function of the p measured variables as expressed in EquationEq. (2)(2)
(2) below:
where “Z” is the principal component, “a” is the component loading, and “X” is the measured variable. The full set of principal components is as large as the original set of variables, but it is common for the sum of the variances of the first few principal components to exceed 80% of the total variance of the original data. By examining plots of these few new variables, researchers often develop a deeper understanding of the driving forces that generated the original data.
PCA was first applied to the original independent variables to transform these variables into an equal number of principal components. Only those principal components with an eigenvector greater than 1 were retained (according to the Kaiser criterion), which were then subjected to Varimax rotation to maximize the loading of a predictor variable on one component (Abdul-Wahab et al. Citation2005). Since the eigenvectors are the correlation of the component variables with the original variables, they comprise coefficients (loadings) that indicate the relative weight of each variable in the component, which is important, since they represent the extent of the correlation between the measured variable and the principal components. Variables that load highly on a specific principal component form a related group.
PCR is a combination of PCA and MLR (Awang et al. Citation2015), where the outputs from the PCA are used as potential predictors in order to improve the original MLR model (Abdul-Wahab et al. Citation2005; Awang et al. Citation2015). Either the original independent variables associated with each of the principal components with high loadings (Abdul-Wahab et al. Citation2005) or the principal components with high loadings (Awang et al. Citation2015) are selected to be included in the regression equation.
2.3.3. Generalized additive models (GAMs)
GAMs extend traditional linear models by allowing for an alternative distribution for the modelling of response variables that have a non-normal error distribution. In addition, GAMs do not force dependent variables to be linearly related to independent variables as in MLR, and recognize that the relationship of some explanatory variables (e.g. daily temperature) and the response variable (i.e. ozone in this study) may not be linear (Gardner and Dorling Citation2000). In GAMs, the response variable depends additively on unknown smoothing functions of the individual predictors that can be (linear) parametric or non-parametric (Hastie and Tibshirani Citation1990). The GAM model equation developed by Hastie and Tibshirani (Citation1990) is given by
where is the response variable,
denotes the expected value and
denotes the link function that links the expected value to the predictor variables
,
is an intercept and
is an i.i.d. random error. For the purposes of the analysis performed in this study, the link function chosen was the identify transformation
The terms
are smooth functions that are estimated in a nonparametric fashion (Hastie and Tibshirani Citation1990). We can estimate these smooth relationships simultaneously from the data and then predict
by simply adding up these functions. The estimated smooth functions
are the analogues of the coefficients
in linear regression. In contrast to MLR, an additive regression is done by using a back-fitting procedure and thereby controlling the effects of the other predictors. GAM is able to identify covariates,
relevant to
for a large set of potential factors (Hayn et al. Citation2009), while it does not require any prior knowledge on the underlying relationship between
and its covariates. The latter can be obtained through separate partial residual plots, which allow visualization of the relationships between each variable
and the response variable,
, after accounting for the effects of the other explanatory variables in the model.
Smooth parameters were automatically selected in the “mgcv” package (Wood Citation2017) in the R software environment used in this study, which is based on maximum probability methods that minimize the Akaike information criterion (AIC) score. The AIC measures the goodness-of-fit of the model in such a manner that the final model selected has the smallest AIC. The models were also evaluated with R2 values and generalized cross-validation (GCV) scores (estimate of the prediction error).
3. Results and discussion
3.1. Exploratory analysis
3.1.1. Descriptive statistics
As indicated in Section 2.2, descriptive statistics were performed prior to the statistical analyses in order to gain a general understanding of meteorological, O3, NOx and CO variations at the measurement locations, which are presented in . It is evident that Elandsfontein and Marikana are the more polluted sites, as indicated by higher NO2, NO and CO median values, whereas Botsalano had the lowest median values for NO2, NO and CO. Note that O3 concentrations are similar at all sites, even though Botsalano and Welgegund are considered regional background sites. The regional problem associated with O3 in southern Africa was indicated by Laban et al. (Citation2018). The large standard deviations of NO2 and NO concentrations can be attributed to occasional high pollution events.
Table 2. Descriptive statistics of the daily summaries of the key variables used in the study
3.1.2. Calculation of correlation coefficients
In , Pearson correlation coefficients (r) relating O3 concentration with individual atmospheric parameters at the four measurement locations are presented. It is evident that O3 has a positive correlation with temperature and global radiation, while it is negatively correlated with relative humidity. A relatively strong positive correlation with CO was observed at Welgegund, Botsalano and Marikana, with NO2 and NO correlations with O3 almost negligible at these sites due to the time scale. The correlations with u and v wind components are also weak, as given by their low correlation coefficients. Exploratory Pearson correlations indicate that variability in O3 levels is in general associated (positively or negatively) with CO (r(O3,CO) = 0.3 to 0.6), relative humidity (r(O3, RH) = −0.2 to −0.5) and temperature (r(O3, T) = 0.2 to 0.5). The significance of CO on O3 levels in this north-eastern interior of South Africa was indicated by Laban et al. (Citation2018). The relative significance of CO, relative humidity and temperature highlighted with these correlations is further explored in subsequent sections through more advanced statistical methods, as indicated in section 2.3.
Table 3. Pearson correlation coefficient (r) for the different variables with their associated p-values (P) for data from the four sites
3.2. Multiple linear regression (MLR) analysis
A summary of the contributions of independent variables to variation of the dependent variable (daily max 8-h O3) included in the optimum MLR models obtained for each of the measurement sites is presented in . VIF values ranging between 1.00 and 2.00 for all the independent variables indicated moderate collinearity, which did not contribute to unstable parameter estimates or the necessity to remove any independent variables from the models. Regression analysis explained approximately 50% of the variability (R2 ≈ 0.5) of daily max 8-h O3 concentrations at Welgegund, Botsalano and Marikana, with lower R2 (0.261) at Elandsfontein attributed to CO not measured at this site and not included in the MLR.
Table 4. Summary of the optimum MLR models for each site showing the individual variable contributions to daily max 8-h O3.
From , it is evident that CO, T and RH make the most significant contributions to the variance in daily max 8-h O3 at Welgegund, Botsalano and Marikana as indicated by the magnitude of the t-statistics. In the absence of CO measurements at Elandsfontein, RH and NO predominantly contributed to variances in daily max 8-h O3, while notable contributions are also made by NO levels at Welgegund. A positive regression coefficient associated with temperature is expected due to the photochemical production of O3 (EquationEquations 1.1(1.1)
(1.1) –Equation1.3)
(1.3)
(1.3) . In addition, evaporative emissions of anthropogenic VOCs increase at high temperatures (Ordonez et al. Citation2005; Jaars et al. Citation2014), which could favour O3 formation as previously mentioned. Relative humidity had a negative regression coefficient and a significant t-statistic at three of the sites, which indicate that low relative humidity is associated with high daily max 8-h O3. This influence of relative humidity on O3 variances suggests that atmospheric wet conditions can affect O3 production and loss, which will be explored later in this paper. Surprisingly, the contribution of relative humidity to O3 variation was similar to that of temperature at Welgegund, while it had the most significant contribution at Elandsfontein (in the absence of any CO measurements). CO levels have the highest contribution to variations in daily max 8-h O3 at Welgegund and Botsalano, i.e. the two regional background sites, while it had the second highest contribution at the industrialized Marikana site. Laban et al. (Citation2018) indicated that CO emissions associated with regional open biomass burning, as well as household combustion for space heating and cooking, contributed significantly to O3 levels in the interior of southern Africa. Negative regression coefficients associated with NO at Welgegund and Elandsfontein can be attributed to O3 titration in the presence of high NO levels (EquationEquation 1.3
(1.3)
(1.3) ).
Since O3 has strong seasonal variation, MLR analysis was also performed for each season: winter (JJA), spring (SON), summer (DJF) and autumn (MAM) in order to evaluate the major factors driving O3 variability during different seasons. Maximum O3 concentrations generally occur in late winter and spring (August–November) for continental southern Africa (Zunckel et al., Citation2004; Combrink et al. Citation1995; Diab et al. Citation2004). In , the independent variables with the most significant contributions (i.e. highest t-statistic values in the optimum model) to O3 variability for different seasons are presented for each site.
Table 5. Most important explanatory variables for daily max 8-h O3 for each season (ranked in decreasing order of importance as given by the magnitude of their t-statistic)
CO makes the highest contribution to the variance in daily max 8-h O3 during all the seasons at Botsalano, during autumn, winter and spring at Welgegund, as well as during spring (second highest in winter) at Marikana, which signifies the influence of CO levels on O3 concentrations in continental South Africa. The seasonal pattern of CO is also reflected in the seasonal variations of contributing factors to O3 variability as indicated by a less important influence of CO levels on the variance in O3 during summer at Welgegund and Marikana. Increased CO emissions in this region are associated with increased household combustion and open biomass burning during winter and spring (Laban et al. Citation2018). This is also indicated by increased contributions of NO and NO2 to O3 variances at Welgegund and Marikana during summer, i.e. increased O3 titration/formation mainly associated with NO and NO2 levels (EquationEquation 1.1(1.1)
(1.1) –Equation1.3)
(1.3)
(1.3) . CO has the highest influence on variation O3 throughout the year at Botsalano, which can be ascribed to the site being more removed from source regions compared to Welgegund. The important influence of relative humidity on O3 levels is also apparent, as indicated by increases in its contribution to O3 variances during months coinciding with the wet season, i.e. mid-October to mid-May (mostly summer and autumn). The wet season is also characterized by lower concentrations of air pollutants (and O3 precursors) due to wet deposition. Daily maximum temperature remains an important contributor to variance in daily max 8-h O3, except during summer at Welgegund, Botsalano and Marikana. This can be attributed to relatively constant higher temperatures occurring during summer, with O3 variability associated with other influencing factors, e.g. relative humidity. In the absence of CO measurements at Elandsfontein, daily maximum temperature contributes most significantly to O3 variability at Elandsfontein on a seasonal scale, which can be attributed to the influence of temperature on the vertical mixing of tall stack emissions of power plants (Ordonez et al. Citation2005). The highest contribution of NO on O3 variance at Elandsfontein in winter can be attributed to more pronounced inversion layers, as well as increased household combustion for space heating and cooking.
3.3. Principal component analysis (PCA)
PCA revealed four principal components (factors) with eigenvalues greater than 1 at each of the sites, which explained approximately 80% of the variation in the data. Only these four factors (labelled Factor 1, Factor 2, Factor 3 and Factor 4) were subjected to Varimax rotation, which are presented with their respective loadings, eigenvalues and variances in . Factor loadings ≥0.5 (or close to 0.5) were considered significant, i.e. strongly correlated within each principal component.
Table 6. Factor loadings after PCA followed by Varimax rotation at the four measurement sites. Loadings ≥ 0.5 (or close to 0.5) are indicated in bold
Similar factor loadings were determined for each of the four principal components identified for each site, i.e. a factor with high loadings of T and Rad, a factor with high loadings of NO and NO2 and a factor with a high loading of RH. A factor with a high loading of CO was determined at Welgegund, Botsalano and Marikana, while one factor was highly loaded with the wind direction vectors at Elandsfontein where CO was not measured. Therefore, PCA indicated that the predominant factors identified by MLR driving variances in daily max 8-h O3, i.e. CO, T and RH (as well as NO levels in certain instances) are not inter-correlated. Collinearity is expected between T and radiation, as well as NO and NO2 as revealed by PCA. In addition, Factor 1 at Marikana with high loadings of CO and NO2 (and NO) is indicative of the influence of household combustion at this site, as indicated by Venter et al. (Citation2012). Furthermore, the correlation between NO2, NO and CO at Welgegund in Factor 1 also reflects the influence of similar sources of these species at Welgegund and signifies that Welgegund lies in a region between a NOx- and VOC-limited O3 production regime, as indicated by Laban et al. (Citation2018). CO is also strongly correlated to meridional wind vector in Factor 4 at the regional background site Welgegund, which can be attributed the regional transport of CO emissions. Welgegund is influenced by the major source regions in the interior of South Africa and a relatively clean background sector to the west (Tiitta et al. Citation2014; Jaars et al. Citation2014). In addition, Welgegund is also impacted on by regional biomass burning, contributing to increased CO emissions (Vakkari et al. Citation2013). In contrast to Welgegund, CO at Botsalano is not correlated to NO and NO2 and is the only major loading in Factor 4 at this site.
3.4. Generalized additive model (GAM) analysis
Given the complex and non-linear chemistry of O3 (NRC Citation1991), the datasets were also statistically analysed with GAM. A summary of the optimum (highest R2 and lowest AIC) GAM models is shown in . According to the F-statistics of the optimum models obtained with GAM, RH and CO make the highest contributions to variances in O3 concentrations Welgegund, Botsalano and Marikana, with T and NO also contributing to O3 variances at these sites. NO, RH and T contributed to O3 variability at Elandsfontein where no CO measurements were conducted. These results correspond to the most significant independent variables contributing to variance in O3 levels indicated by MLR.
Table 7. Summary of the optimum GAM for each site showing the individual variable contributions to daily max 8-h O3. This was done with the function gamm in R, which takes into account autocorrelation in the O3 data
To diagnose the nature of the relationships between O3 and each of the independent variables, partial residual plots were examined (). The partial residual plot of each independent variable, , versus the smooth function,
, shows the relationship between
and
given that the other independent variables are also included in the model. These residual plots indicate that, in the temperature range 20°C to 35°C, the relationship between daily max 8-h O3 and T is positive and linear at Welgegund, Botsalano and Elandsfontein, while a change in slope is evident at lower temperatures. At Marikana, however, T is linearly and positively correlated for the entire T range. At all four sites, the change in O3 with a change in relative humidity is linear and negatively correlated over the entire humidity range. For CO, the partial residual plot identified a positive linear relationship (although there is a small change in slope around 150–200 ppb for Welgegund and Botsalano) across the concentration range for Marikana. For NO and NO2, there is sometimes a more complex (non-linear) fit in their partial residual response, suggesting other effects confounding with NO and NO2.
Figure 1. Partial residual plots of independent variables contained in the optimum solution from the GAM for O3. The solid line in each plot is the estimate of the spline smooth function bounded by 95% confidence limits (i.e. ±2 standard errors of the estimate). The tick marks along the horizontal axis represent the density of data points of each explanatory variable (rug plot)

3.5. Comparison of statistical models
In order to relate the statistical models utilized in this study, the differences between O3 concentrations calculated with each model and measured O3 levels (expressed as R2 and RMSE) were compared and presented in . The factors obtained with PCA were also included in an MLR model to perform PCR, as indicated in section 2.3.2, which are presented in . Previous-day daily max 8-h O3 was also included as an independent variable in the evaluation of these models in order to deal with the autocorrelation (persistence) in the data and to increase model performance (Comrie Citation1997), since it could also contribute to daily max 8-h O3 (Otero et al. Citation2016). Previous-day daily max 8-h O3 was not included in sections 3.2 to 3.4 where the influence of different independent variables on variances of O3 was evaluated, since it could suppress the influence of other independent variables (Achen Citation2001). The complete statistics from each of the models are presented in of the appendix. It is evident from that inclusion of the previous-day daily max 8-h O3 increases the performance of the MLR and GAM models, as reflected by the relative contribution to total explained variance (i.e. R2 significantly increases). The results show that the O3 concentrations calculated with non-parametric GAM compared slightly better to measured O3 concentrations than O3 levels calculated with MLR and PCR, as indicated by the highest R2- and smallest RMSE values for GAM. However, less complicated MLR models are also suitable to evaluate contributions of factors to variances in O3 levels. In addition, the inclusion of only previous-day daily max 8-h O3, T, RH and CO in these statistical models explained approximately 70% of the variance in daily max 8-h O3, which implies that these are the main factors influencing variations in O3 concentrations in continental South Africa.
Table 8. Comparison of statistical models in predicting daily max 8-h O3 at the four measurement sites
3.6. Insights into major factors driving O3 variances
As indicated above, CO, RH and T were identified by all three statistical models as the major factors driving variances in O3 levels in southern Africa. In many empirical and modelling studies, temperature is generally considered the most strongly correlated with O3 concentrations (Jacob et al. Citation1993; Ryan Citation1995; Hubbard and Cobourn Citation1998; Baertsch-Ritter et al. Citation2004; Camalier et al. Citation2007; Dawson et al. Citation2007; Lin and Cobourn Citation2007; Cobourn Citation2007), which therefore has been used as a reasonable proxy to account for the combined influence of meteorological and chemical factors on O3 concentrations (Jacob et al. Citation1993; Tsakiri and Zurbenko Citation2011; Rasmussen et al. Citation2012). High temperatures are usually associated with high solar radiation that contributes to increased photochemical reaction rates (EquationEquation 1.1(1.1)
(1.1) and Equation1.2
(1.2)
(1.2) ), as well as other meteorological conditions favouring O3 production, such as high pressure, stagnation of air masses and reduced cloud cover (NRC Citation1991; Jacob et al. Citation1993). Jaars et al. (Citation2014) also indicated that increased ambient VOC concentrations at Welgegund were associated with higher temperatures resulting from higher evaporation rates, which could also contribute to the increased O3 formation potential of VOCs. The positive correlation between O3 and temperature is also largely driven by the chemical equilibrium between NOx and peroxyacetylnitrate (PAN), which serves as a reservoir for NOx (Jacob et al. Citation1993). The enhanced decomposition of PAN at high temperatures to regenerate stored NOx results in local O3 production being maximized (Jacob et al. Citation1993; Sillman and Samson Citation1995; Sillman Citation1999).
Some studies have indicated the significance of relative humidity to surface O3 concentrations (Camalier et al. Citation2007; Davis et al. Citation2011; Awang et al. Citation2018). In the eastern United States, for instance, a north-south divide in terms of meteorological parameters controlling O3 levels has been discussed in various studies (Camalier et al. Citation2007; Zheng et al. Citation2007; Davis et al. Citation2011; Rasmussen et al. Citation2012; Tawfik and Steiner Citation2013), with temperature most strongly correlated with O3 at high latitude and strongly negatively correlated with relative humidity at lower latitude. This strong negative relationship between O3 and relative humidity is not widely understood, with several authors presenting possible explanations:
The O3-relative humidity correlation is closely related to the O3-temperature correlation, where temperature is the actual cause of O3 variability, simultaneously affecting relative humidity and O3 concentration (Camalier et al. Citation2007; Bloomer et al. Citation2009);
High relative humidity can be associated with increased cloud cover and reduced UV radiation, which limits the photochemical production of O3 to occur (Camalier et al. Citation2007; Davis et al. Citation2011; Porter et al. Citation2015);
High relative humidity is associated with wet deposition (precipitation), which does not affect O3 directly, but leads to the removal of soluble species such as HNO3 and H2O2 and consequently the availability of NOx and OH (Wild Citation2007). Furthermore, increased relative humidity increases the stomatal conductance of plants (Kavassalis and Murphy (Citation2017)) and therefore also the dry deposition of surface O3;
Increased concentrations of atmospheric water vapour provide a chemical sink for O3 through the reaction with water after photolysis, instead of the quenching reaction where O3 is regenerated;
Higher relative humidity can lead to more liquid water on aerosol particles, causing increased loss of gas phase NOx via the heterogeneous reaction of dinitrogen pentoxide (N2O5) on particulates (Bertram and Thornton Citation2009). Jia and Xu (Citation2014) also showed that increased relative humidity can greatly reduce O3 through the transfer of NO2- and ONO2-containing species (reactive nitrogen species) to the particulate phase;
Increased surface O3 concentrations associated with stratospheric intrusions are associated with low water vapour (Thompson et al. Citation2014, Citation2015; Stauffer et al. Citation2017);
O3-relative humidity correlation can also result from a shift in the soil-moisture atmosphere coupling regime (evapotranspiration-limiting regimes), reflecting the simultaneous impact of soil moisture deficit on near-surface humidity, temperature and radiation (Tawfik and Steiner Citation2013).
All these afore-mentioned explanations could contribute to the significant (negative) correlation between O3 variances and relative humidity observed for southern Africa. However, the relative role of temperature and relative humidity in driving O3 variability is not yet fully disentangled due to their interdependency with the order of their significance possibly related to short-term dependencies, i.e. weather- and precursor emissions fluctuations. The significance of the influence of temperature and relative humidity on surface O3 is also indicated by substantial higher O3 concentrations measured during spring in 2015 at Welgegund. Dry and warm conditions were associated with the El Niño weather cycle, which persisted into the first half of 2016 with the 2015/2016 rain season being one of the warmest and driest in approximately 35 years.
The influence of CO on tropospheric O3 formations is well known. CO and VOCs are the main sources of peroxy radicals that alter the PSS of O3 production. Laban et al. (Citation2018) indicated the important influence of CO on surface O3 levels in southern Africa. CO emissions were attributed to household combustion for space heating and regional open biomass burning. Source maps indicated that O3 and CO had similar regional sources with the highest concentrations of these species corresponding with the regions where a large number of wild fire events occurred. Furthermore, it was also indicated by Laban et al. (Citation2018) that increased surface O3 levels correlated with higher CO concentrations at Welgegund, Botsalano and Marikana, while it was implied that regional background regions in southern Africa could be considered VOC limited.
4. Conclusions
Three multivariate statistical models were utilized in order to provide some insights into major factors driving surface O3 variability in continental southern Africa. Concentrations of precursors species and meteorological parameters measured at four sites located in the north-eastern interior of South Africa were included as input parameters. MLR indicated that CO, temperature and relative humidity made the largest contribution in explaining variances in daily max 8-h O3. PCA indicated that parameters calculated with MLR are not strongly collinear and contributed independently to variances. Nonlinear GAM also revealed that CO, temperature and relative humidity were the most important parameters influencing variances in O3 levels. Partial residual plots indicated that NOx most likely have a non-linear relationship with O3, while the relationship with temperature, relative humidity and CO is probably linear. Comparison of the measured O3 concentrations with O3 levels calculated with MLR and GAM indicated that O3 levels calculated with both these models compared well to measured O3 values, with GAM performing slightly better.
The influence of temperature on O3 variability is expected, while Laban et al. (Citation2018) indicated the significance of CO emissions associated with biomass burning on surface O3 levels in southern Africa. The significant effect of relative humidity on O3 variability, i.e. lower O3 associated with increased relative humidity, was unexpected. Therefore, the influence of relative humidity should not be underestimated in atmospheric O3 formation and prediction models.
In conjunction with variables utilized in this study, other synoptic-scale meteorological contributions to surface O3 should also be investigated, e.g. large-scale atmospheric circulation over this region. It is also important that VOCs are included in statistical models. No continuous long-term VOC measurements were conducted at any of the sites. Although Jaars et al. (Citation2014) and Jaars et al. (Citation2016) did report on VOCs collected with grab samples during a two-year sampling campaign at Welgegund, this data was not from a statistical perspective considered sufficient to be included in the statistical models. Photochemical box models can also be used to investigate the main reactions that participate in O3 formation. A greater scientific understanding of the factors influencing surface O3 concentrations in South Africa will allow regional air quality models to be improved for the prediction of surface O3 concentrations. It could be a step towards developing operational O3 forecast models for cities and towns in South Africa.
Acknowledgments
V Vakkari is a beneficiary of an AXA Research Fund postdoctoral grant. The authors are also grateful to Eskom for supplying the Elandsfontein data., North-West University, Private Bag x6001, Potchefstroom 2520, South Africa.
Disclosure statement
The authors declare that they have no conflict of interest.
Data availability
The data of this paper are available upon request to Pieter van Zyl ([email protected]) or Johan Paul Beukes ([email protected]).
Additional information
Funding
References
- Abdul-Wahab SA, Bakheit CS, Al-Alawi SM. 2005. Principal component and multiple regression analysis in modelling of ground-level ozone and factors affecting its concentrations. Environ Model Softw. 20(10):1263–1271. doi:10.1016/j.envsoft.2004.09.001.
- Achen CH. 2001. Why lagged dependent variables can suppress the explanatory power of other independent variables. Ann Arbor. 1001:41248–48106.
- Awang NR, Ramli NA, Shith S, Zainordin NS, Manogaran H. 2018. Transformational characteristics of ground-level ozone during high particulate events in urban area of Malaysia. Air Quality, Atmosphere & Health. 11(6):715–727. doi:10.1007/s11869-018-0578-0.
- Awang NR, Ramli NA, Yahaya AS, Elbayoumi M. 2015. Multivariate methods to predict ground level ozone during daytime, nighttime, and critical conversion time in urban areas. Atmos Pollut Res. 6(5):726–734. doi:10.5094/APR.2015.081.
- Baertsch-Ritter N, Keller J, Dommen J, Prevot A. 2004. Effects of various meteorological conditions and spatial emission resolutions on the ozone concentration and ROG/NOx limitation in the Milan area (I). Atmos Chem Phys. 4(2):423–438. doi:10.5194/acp-4-423-2004.
- Bertram T, Thornton J. 2009. Toward a general parameterization of N2O5 reactivity on aqueous particles: the competing effects of particle liquid water, nitrate and chloride. Atmos Chem Phys. 9(21):8351–8363. doi:10.5194/acp-9-8351-2009.
- Bloomer BJ, Stehr JW, Piety CA, Salawitch RJ, Dickerson R. 2009. R.: observed relationships of ozone air pollution with temperature and emissions. Geophys Res Lett. 36(9). doi:10.1029/2009GL037308.
- Bloomfield P, Royle JA, Steinberg LJ, Yang Q. 1996. Accounting for meteorological effects in measuring urban ozone levels and trends. Atmos Environ. 30(17):3067–3077. doi:10.1016/1352-2310(95)00347-9.
- Camalier L, Cox W, Dolwick P. 2007. The effects of meteorology on ozone in urban areas and their use in assessing ozone trends. Atmos Environ. 41(33):7127–7137. doi:10.1016/j.atmosenv.2007.04.061.
- Cardelino C, Chang M, John JS, Murphey B, Cordle J, Ballagas R, Patterson L, Powell K, Stogner J, Zimmer-Dauphinee S. 2001. Ozone predictions in Atlanta, Georgia: analysis of the 1999 ozone season. J Air Waste Manage Assoc. 51(8):1227–1236. doi:10.1080/10473289.2001.10464342.
- Cobourn WG. 2007. Accuracy and reliability of an automated air quality forecast system for ozone in seven Kentucky metropolitan areas. Atmos Environ. 41(28):5863–5875. doi:10.1016/j.atmosenv.2007.03.024.
- Combrink J, Diab R, Sokolic F, Brunke E. 1995. Relationship between surface, free tropospheric and total column ozone in two contrasting areas in South Africa. Atmos Environ. 29(6):685–691. doi:10.1016/1352-2310(94)00313-A.
- Comrie AC. 1997. Comparing neural networks and regression models for ozone forecasting. Air Waste Manage. Assoc. 47(6):653–663. doi:10.1080/10473289.1997.10463925.
- Cooper OR, Gao RS, Tarasick D, Leblanc T, Sweeney C. 2012. Long‐term ozone trends at rural ozone monitoring sites across the United States, 1990–2010. J Geophys Res, 117: D22307. doi:10.1029/2012JD018261.
- Davis J, Cox W, Reff A, Dolwick P. 2011. A comparison of CMAQ-based and observation-based statistical models relating ozone to meteorological parameters. Atmos Environ. 45(20):3481–3487. doi:10.1016/j.atmosenv.2010.12.060.
- Dawson JP, Adams PJ, Pandis SN. 2007. Sensitivity of ozone to summertime climate in the eastern USA: A modeling case study. Atmos Environ. 41(7):1494–1511. doi:10.1016/j.atmosenv.2006.10.033.
- Diab R, Thompson A, Mari K, Ramsay L, Coetzee G. 2004. Tropospheric ozone climatology over Irene, South Africa, from 1990 to 1994 and 1998 to 2002. J Geophys Res. 109(D20). doi:10.1029/2004JD004793.
- Dominick D, Juahir H, Latif MT, Zain SM, Aris AZ. 2012. Spatial assessment of air quality patterns in Malaysia using multivariate analysis. Atmos Environ. 60:172–181. doi:10.1016/j.atmosenv.2012.06.021.
- Dueñas C, Fernández MC, Cañete S, Carretero J, Liger E. 2002. Assessment of ozone variations and meteorological effects in an urban area in the Mediterranean Coast. Sci Total Environ. 299(1–3):97–113. doi:10.1016/S0048-9697(02)00251-6.
- Fiore AM, Jacob DJ, Logan JA, Yin JH. 1998. Long‐term trends in ground level ozone over the contiguous United States, 1980–1995. J Geophys Res. 103(D1):1471–1480. doi:10.1029/97JD03036.
- Gardner M, Dorling S. 2000. Meteorologically adjusted trends in UK daily maximum surface ozone concentrations. Atmos Environ. 34(2):171–176. doi:10.1016/S1352-2310(99)00315-5.
- Gardner MW, Dorling S. 1998. Artificial neural networks (the multilayer perceptron)—a review of applications in the atmospheric sciences. Atmos Environ. 32(14–15):2627–2636. doi:10.1016/S1352-2310(97)00447-0.
- Gorai A, Tuluri F, Tchounwou P, Ambinakudige S. 2015. Influence of local meteorology and NO2 conditions on ground-level ozone concentrations in the eastern part of Texas, USA, Air Quality. Atmos Health. 8(1):81–96. doi:10.1007/s11869-014-0276-5.
- Guardani R, Aguiar JL, Nascimento CA, Lacava CI, Yanagi Y. 2003. Ground-level ozone mapping in large urban areas using multivariate statistical analysis: application to the Sao Paulo Metropolitan area. J Air Waste Manage Assoc. 53(5):553–559. doi:10.1080/10473289.2003.10466188.
- Hastie T, Tibshirani R. 1990. Generalized additive models. Wiley Online Library.
- Hayn M, Beirle S, Hamprecht FA, Platt U, Menze BH, Wagner T. 2009. Analysing spatio-temporal patterns of the global NO2-distribution retrieved from GOME satellite observations using a generalized additive model. Atmos Chem Phys. 9(17):6459–6477. doi:10.5194/acp-9-6459-2009.
- Hubbard MC, Cobourn WG. 1998. Development of a regression model to forecast ground-level ozone concentration in Louisville, KY. Atmos Environ. 32(14–15):2637–2647. doi:10.1016/S1352-2310(97)00444-5.
- Jaars K, Beukes JP, van Zyl PG, Venter AD, Josipovic M, Pienaar JJ, Vakkari V, Aaltonen H, Laakso H, Kulmala M, et al. 2014. Ambient aromatic hydrocarbon measurements at Welgegund, South Africa. Atmos Chem Phys. 14(13):7075–7089. doi:10.5194/acp-14-7075-2014.
- Jaars K, van Zyl PG, Beukes JP, Hellén H, Vakkari V, Josipovic M, Venter AD, Räsänen M, Knoetze L, Cilliers DP, et al. 2016. Measurements of biogenic volatile organic compounds at a grazed savannah grassland agricultural landscape in South Africa. Atmos Chem Phys. 16(24):15665–15688. doi:10.5194/acp-16-15665-2016.
- Jacob DJ, Logan JA, Gardner GM, Yevich RM, Spivakovsky CM, Wofsy SC, Sillman S, Prather MJ. 1993. Factors regulating ozone over the United States and its export to the global atmosphere. J Geophys Res. 98(D8):14817–14826. doi:10.1029/98JD01224.
- Jia L, Xu Y. 2014. Effects of relative humidity on ozone and secondary organic aerosol formation from the photooxidation of benzene and ethylbenzene. Aerosol Sci and Tech. 48(1):1–12. doi: 10.1080/02786826.2013.847269.
- Kavassalis SC, Murphy JG. 2017. Understanding ozone‐meteorology correlations: A role for dry deposition. Geophys Res Lett. 44(6):2922–2931. doi:10.1002/2016GL071791.
- Laban TL, van Zyl PG, Beukes JP, Vakkari V, Jaars K, Borduas-Dedekind N, Josipovic M, Thompson AM, Kulmala M, Laakso L. 2018. Seasonal influences on surface ozone variability in continental South Africa and implications for air quality. Atmos Chem Phys Discuss. 18(20):15491–15514. doi:10.5194/acp-2017-1115.
- Lin Y, Cobourn WG. 2007. Fuzzy system models combined with nonlinear regression for daily ground-level ozone predictions. Atmos Environ. 41(16):3502–3513. doi:10.1016/j.atmosenv.2006.11.060.
- Lourens AS, Beukes JP, Van Zyl PG, Fourie GD, Burger JW, Pienaar JJ, Read CE, Jordaan JH. 2011. Spatial and temporal assessment of gaseous pollutants in the Highveld of South Africa. S Afr J Sci. 107(1/2):1–8. doi:10.4102/sajs.v107i1/2.269.
- Lourens ASM, Butler TM, Beukes JP, Van Zyl PG, Beirle S, Wagner TK, Heue K-P, Pienaar JJ, Fourie GD, Lawrence MG. 2012. Re-evaluating the NO2 hotspot over the South African Highveld. South African J Sci. doi:10.4102/sajs.v108i11/12.1146.
- Melkonyan A, Kuttler W. 2012. Long-term analysis of NO, NO2 and O3 concentrations in North Rhine-Westphalia, Germany. Atmos Environ. 60:316–326. doi:10.1016/j.atmosenv.2012.06.048.
- Mikkonen S, Korhonen H, Romakkaniemi S, Smith JN, Joutsensaari J, Lehtinen KEJ, Hamed A, Breider TJ, Birmili W, Spindler G, et al. 2011. Meteorological and trace gas factors affecting the number concentration of atmospheric Aitken (Dp= 50 nm) particles in the continental boundary layer: pparameterization using a multivariate mixed effects model. Geosci Model Dev. 4(1):1–13. doi:10.5194/gmd-4-1-2011.
- Milanchus ML, Rao ST, Zurbenko IG. 1998. Evaluating the effectiveness of ozone management efforts in the presence of meteorological variability. J Air Waste Manage Assoc. 48(3):201–215. doi:10.1080/10473289.1998.10463673.
- Neter J, Kutner M, Nachtsheim C, Wasserman W. 1996. Applied linear statistical models. 4th ed. New York: McGraw-Hill; p. 283.
- NRC. 1991. Rethinking the ozone problem in urban and regional air pollution. Washington (DC): The National Academies Press; p. 524.
- NRC. 2008. Estimating mortality risk reduction and economic benefits from controlling ozone air pollution. Washington, DC: National Academies Press. https://doi.org/10.17226/12198.
- Ooka R, Khiem M, Hayami H, Yoshikado H, Huang H, Kawamoto Y. 2011. Influence of meteorological conditions on summer ozone levels in the central Kanto area of Japan. Procedia Environ. Sci. 4:138–150. doi:10.1016/j.proenv.2011.03.017.
- Ordonez C, Mathis H, Furger M, Henne S, Hüglin C, Staehelin J, Prévôt A. 2005. Changes of daily surface ozone maxima in Switzerland in all seasons from 1992 to 2002 and discussion of summer 2003. Atmos Chem Phys. 5(5):1187–1203. doi:10.5194/acp-5-1187-2005.
- Otero N, Sillmann J, Schnell JL, Rust HW, Butler T. 2016. Synoptic and meteorological drivers of extreme ozone concentrations over Europe. Environ Res Lett. 11(2):024005. doi:10.1088/1748-9326/11/2/024005.
- Pearce JL, Beringer J, Nicholls N, Hyndman RJ, Tapper NJ. 2011. Quantifying the influence of local meteorology on air quality using generalized additive models. Atmos Environ. 45(6):1328–1336. doi:10.1016/j.atmosenv.2010.11.051.
- Porter WC, Heald CL, Cooley D, Russell B. 2015. Investigating the observed sensitivities of air-quality extremes to meteorological drivers via quantile regression. Atmos Chem Phys. 15(18):10349–10366. doi:10.5194/acp-15-10349-2015.
- R Development Core Team. 2009. R: A language and environment for statistical computing. [accessed 2018 Mar 12]. http://www.R-project.org.
- Rao ST, Zurbenko IG. 1994. Detecting and tracking changes in ozone air quality. Air Waste. 44(9):1089–1092. doi:10.1080/10473289.1994.10467303.
- Rasmussen D, Fiore A, Naik V, Horowitz L, McGinnis S, Schultz M. 2012. Surface ozone-temperature relationships in the eastern US: A monthly climatology for evaluating chemistry-climate models. Atmos Environ. 47:142–153. doi:10.1016/j.atmosenv.2011.11.021.
- Ryan WF. 1995. Forecasting severe ozone episodes in the Baltimore metropolitan area. Atmos Environ. 29(17):2387–2398. doi:10.1016/1352-2310(94)00302-2.
- Schlink U, Herbarth O, Richter M, Dorling S, Nunnari G, Cawley G, Pelikan E. 2006. Statistical models to assess the health effects and to forecast ground-level ozone. Environ Model Softw. 21(4):547–558. doi:10.1016/j.envsoft.2004.12.002.
- Seinfeld JH, Pandis SN. 2006. Atmospheric chemistry and physics: from air pollution to climate change. Vol. xxviii, 2nd ed. New York: Wiley. p. 1202
- Sillman S. 1999. The relation between ozone, NOx and hydrocarbons in urban and polluted rural environments. Atmos Environ. 33(12):1821–1845. doi:10.1016/S1352-2310(98)00345-8.
- Sillman S, Samson PJ. 1995. Impact of temperature on oxidant photochemistry in urban, polluted rural and remote environments. J Geophys Res Atmospheres. 100(D6):11497–11508. doi:10.1029/94JD02146.
- Stauffer RM, Thompson AM, Oltmans SJ, Johnson BJ. 2017. Tropospheric ozonesonde profiles at long‐term US monitoring sites: 2. Links between Trinidad Head, CA, profile clusters and inland surface ozone measurements. J Geophys Res. 122:1261–1280.
- Tawfik AB, Steiner AL. 2013. A proposed physical mechanism for ozone-meteorology correlations using land–atmosphere coupling regimes. Atmos Environ. 72:50–59. doi:10.1016/j.atmosenv.2013.03.002.
- Thompson AM, Balashov NV, Witte JC, Coetzee JGR, Thouret V, Posny F. 2014. Tropospheric ozone increases over the southern Africa region: bellwether for rapid growth in Southern Hemisphere pollution? Atmos Chem Phys. 14(18):9855–9869. doi:10.5194/acp-14-9855-2014.
- Thompson AM, Stauffer RM, Miller SK, Martins DK, Joseph E, Weinheimer AJ, Diskin GS. 2015. Ozone profiles in the Baltimore-Washington region (2006–2011): satellite comparisons and DISCOVER-AQ observations. J Atmos Chem. 72(3–4):393–422. doi:10.1007/s10874-014-9283-z.
- Thompson ML, Reynolds J, Cox LH, Guttorp P, Sampson PD. 2001. A review of statistical methods for the meteorological adjustment of tropospheric ozone. Atmos Environ. 35(3):617–630. doi:10.1016/S1352-2310(00)00261-2.
- Tiitta P, Vakkari V, Croteau P, Beukes JP, van Zyl PG, Josipovic M, Venter AD, Jaars K, Pienaar JJ, Ng NL, et al. 2014. Chemical composition, main sources and temporal variability of PM1 aerosols in southern African grassland. Atmos Chem Phys. 14(4):1909–1927. doi:10.5194/acp-14-1909-2014.
- Tsakiri KG, Zurbenko IG. 2011. Prediction of ozone concentrations using atmospheric variables. Air Qual Atmos Health. 4(2):111–120. doi:10.1007/s11869-010-0084-5.
- Vakkari V, Beukes JP, Laakso H, Mabaso D, Pienaar JJ, Kulmala M, Laakso L. 2013. Long-term observations of aerosol size distributions in semi-clean and polluted savannah in South Africa. Atmos Chem Phys. 13(4):1751–1770. doi:10.5194/acp-13-1751-2013.
- Venter AD, Vakkari V, Beukes JP, Van Zyl PG, Laakso H, Mabaso D, Tiitta P, Josipovic M, Kulmala M, Pienaar JJ, et al. 2012. An air quality assessment in the industrialised western Bushveld Igneous Complex, South Africa. S Afr J Sci. 108(9/10). doi:10.4102/sajs.v108i9/10.1059.
- Wild O. 2007. Modelling the global tropospheric ozone budget: exploring the variability in current models. Atmos Chem Phys. 7(10):2643–2660. doi:10.5194/acp-7-2643-2007.
- Wood SN. 2017. Generalized additive models: an introduction with R. Boca Raton, FL: CRC press.
- Zheng J, Swall JL, Cox WM, Davis JM. 2007. Interannual variation in meteorologically adjusted ozone levels in the eastern United States: A comparison of two approaches. Atmos Environ. 41(4):705–716. doi:10.1016/j.atmosenv.2006.09.010.
- Zunckel M, Venjonoka K, Pienaar JJ, Brunke EG, Pretorius O, Koosialee A, Raghunandan A, van Tienhoven AM. 2004. Surface ozone over southern Africa: synthesis of monitoring results during the cross border air pollution impact assessment project. Atmos Environ. 38:6139–6147. doi:10.1016/j.atmosenv.2004.07.029
Appendix
Table A1. MLR models for prediction of daily max 8-h O3 for each measurement site
Table A2. PCR models for prediction of daily max 8-h O3 for each measurement site
Table A3. GAMs for prediction of daily max 8-h O3 for each measurement site: includes tests for each smooth, the degrees of freedom for each smooth, adjusted R-squared for the model and deviance for the model