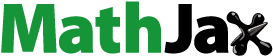
Abstract
Rock mass strength parameters are critical when applying the permanent-displacement analysis to landslide susceptibility assessment. Previous studies use limited measured data to assign a constant value for each lithology. Since the rock mass strength is spatially heterogeneous, assigning a constant value to each lithology cannot effectively reflect the spatial heterogeneity of rock mass strength, reducing the reliability of seismic landslide susceptibility assessment. Here we present an analysis of a well-documented seismic landslide inventory and the lithological environment, and develop an empirical model to quantify the rock mass strength heterogeneity. The rock mass strength heterogeneity was subsequently taken as an input of the permanent-displacement analysis to assessing regional seismic landslide susceptibility. Results on two case studies show that considering the rock mass strength heterogeneity would significantly improve seismic landslide susceptibility assessment. The findings of this study are valuable for earthquake emergency rescue and post-earthquake land plans.
1. Introduction
A strong earthquake may instantly trigger a large number of landslides that can be catastrophic, resulting in life loss, fatalities, or economic damage. For example, the 2008 Wenchuan earthquake-induced 197,481 seismic landslides in an area of ∼110,000 km2 (Xu et al. Citation2014). Apart from seismic landslides, strong earthquakes also generate numerous discontinuity sets prone to slope instability. Heavy precipitation after the quake causes the slope stability to deteriorate, presenting serious threats to lives and properties (Guzzetti et al. Citation2006; Robinson et al. Citation2017). For instance, after the Wenchuan earthquake, the landslide frequency increased substantially compared with that before the earthquake (Huang and Li Citation2014). Thus, timely and reliably assessing the seismic landslide susceptibility is essential to prevent and mitigate the effects of seismic landslides (Tian et al. Citation2019; Segoni et al. Citation2020; Liu et al. Citation2021).
Statistical analysis and permanent-displacement analysis are two commonly used methods for seismic landslide susceptibility assessment (Pourghasemi et al. Citation2018; Ibrahim et al. Citation2021; Zou et al. Citation2021). The statistical analysis establishes a mathematical model by exploring the relationship between landslides and geo-environmental factors from seismic landslide inventory. The established mathematical model is then applied to assess the seismic landslide susceptibility for the whole area (Huang and Zhao Citation2018; Reichenbach et al. Citation2018; Merghadi et al. Citation2020). Its reliability relies on the quality and completeness of the landslide inventory. A high-quality and complete landslide inventory, however, is challenging or even impossible to produce, which limits the statistical analysis applications (Du et al. Citation2020; Chen et al. Citation2021). In contrast, the physically-based permanent-displacement analysis does not require the landslide inventory, and thus can be used to assess seismic landslide susceptibility in various scenarios (Wieczorek et al. Citation1985; Jibson Citation2011; Liu et al. Citation2018; Kumar et al. Citation2021). It takes a slope as a sliding-block with a certain critical acceleration. The critical acceleration represents the minimum acceleration that causes the sliding-block to slide. Once an earthquake’s ground motion acceleration exceeds the block’s minimum acceleration, the block initiates sliding deformation (also called permanent seismic displacement). Numerous studies have validated that the displacement is an important indicator to describe the seismic landslide susceptibility (Salvatici et al. Citation2018; Martino et al. Citation2020). To date, this method has been widely applied to landslide susceptibility assessment for many earthquakes, such as the 1994 Northridge earthquake (Jibson Citation1993; Jibson et al. Citation2000), 1999 Chichi earthquake (Tsai et al. Citation2019), 2008 Wenchuan earthquake (Godt et al. Citation2008; Wang et al. Citation2013; Ma and Xu Citation2019), 2014 Ludian earthquake (Chen et al. Citation2019; Huang et al. Citation2020), and 2017 Jiuzhaigou earthquake (Chen et al. Citation2020).
When applying the permanent-displacement analysis to seismic landslide susceptibility assessment, critical acceleration of the slope is a prerequisite, which can be calculated based on the rock mass shear strength of the slope. Rock mass shear strength can be described by different types of strength models (e.g. Mohr-Coulomb, Hoek-Brown, Barton). Among them, the Mohr-Coulomb strength parameters have received much attention in permanent-displacement analysis (Jibson Citation1993; Gallen et al. Citation2015; Shinoda and Miyata Citation2017), which can be obtained from the laboratory test on selected samples (i.e. direct shear or triaxial tests) (Labuz and Zang Citation2012). However, the sample in laboratory is too small to reflect the widely developed scale-related structural features (e.g. fractures, joints, and discontinuities) (Hoek and Brown Citation1980; Citation1997). Compared to the laboratory test, in-situ test can obtain reliable rock mass strength parameters, while conducting such a test at a regional scale is time-consuming, labour-intensive, or even impractical. Besides, the limited measurement data cannot represent the real rock mass strength parameters in a region. Therefore, obtaining reliable rock mass strength parameters remains an immense challenge, particularly at a regional scale.
Previous studies usually assigned a constant value for each lithology in a region based on the outcropping lithologies (Jibson et al. Citation2000; Godt et al. Citation2008; Wang et al. Citation2017; Salinas-Jasso et al. Citation2019; Kumar et al. Citation2021). This assignment ignores the variability of rock mass strength in space, resulting in uncertainty in seismic landslide susceptibility. To tackle this issue, researchers tried to obtain reliable rock mass strength parameters from different perspectives (Wang et al. Citation2008; Wang and Rathje Citation2015; Lee and Park Citation2016; Nguyen and Kim Citation2020). For example, the Monte Carlo method was applied to simulate the variability of rock mass strength parameters by assuming that the rock mass strength fits a specific distribution (e.g. normal, uniform) (Murphy and Mankelow Citation2004; Lee and Park Citation2016). Rodríguez-Peces et al. (Citation2020) designed a logic tree for descripting the uncertainty in rock mass strength parameter for the displacement analysis method. Although these methods could improve the reliability of the rock mass strength parameters, they require a large number of measurements, which limits the application of these methods. Given the availability of seismic landslide inventories, rock mass strength parameters can be inverted using ground motion parameters and seismic landslides (Gallen et al. Citation2015; Bunn et al. Citation2020; Huang et al. Citation2020; Townsend et al. Citation2020). For example, Shinoda et al. (Citation2019) used different combinations of rock mass strength parameters to calculate permanent seismic displacements and determined the most appropriate rock mass strength parameters (i.e. effective cohesion and friction angle) by comparing the calculated displacement with the observed landslides. Jeandet et al. (Citation2019) used Coulomb mechanics and relief constraints to explain the landslide size distribution, finding that reasonable low ranges of Mohr-Coulomb shear strength properties can effectively model seismic landslide size distribution. However, these methods also assumed a constant strength value for a lithology and assigned an average or residual strength parameter for each lithology based on the geological map. Although this assumption makes the operation easy, the variability within a geological unit is typically ignored due to practice constraints (Dreyfus et al. Citation2013; Rodríguez-Peces et al. Citation2020).
Rock mass properties depend not only on lithology but also on rock mass structure and weathering characteristics. Studies shown that, compared with lithology differences, rock mass structure (e.g. fractures, joints, and discontinuities) and weathering characteristics have a more substantial influence on rock mass properties (Schmidt and Montgomery Citation1995; Alberti et al. Citation2022; Medwedeff et al. Citation2022). Influenced by the lithological environment, the rock mass structure and weathering characteristics exhibit a strong degree of spatial heterogeneity (Baroni et al. Citation2010; Bicocchi et al. Citation2019; Fusco et al. Citation2021). For example, Medwedeff et al. (Citation2022) evaluated landscape-scale patterns in near-surface mechanical characteristics and their relationship to environmental factors that affect rock strength by collecting in-situ measurements. They found that shallow bedrock strength is more dependent on the degree of chemical and physical weathering, rather than the mineral and textural differences between the metamorphic lithologies. Obviously, assigning a constant value for each lithology cannot reflect the spatial heterogeneity of rock mass strength in complex environment. Therefore, to obtain a reliable rock mass strength parameter, its spatial heterogeneity should be given reasonable consideration.
Here, we proposed a novel method to estimate the rock mass strength heterogeneity and applied it as the input of the permanent displacement analysis method for regional seismic landslide susceptibility assessment. We considered lithological environmental factors known to affect rock mass strength to estimate the rock mass strength heterogeneity. An empirical model was developed based on a well-documented seismic landslide inventory of the 2008 Wenchuan earthquake. The estimated spatial heterogeneity of rock mass strength and the permanent-displacement analysis were then jointly used for regional seismic landslide susceptibility assessment. Finally, two earthquakes with similar geo-environmental conditions were selected for model verification.
2. Study areas
This study selected the Wenchuan and Ludian earthquakes () as study cases. The Wenchuan Mw 7.9 earthquake occurred on May 12, 2008, with the epicentre located southwest of Yingxiu, Wenchuan (103.4°E, 31.0°N), at a focal depth of 14 km. The seismic rupture of this earthquake was located in the Longmenshan Fault on the eastern edge of the Qinghai–Tibet Plateau. By September 25, 2008, the Wenchuan earthquake resulted in 69,227 people dead, 17,923 people missing, and 374,643 people injured (Wang Citation2008). This earthquake also resulted in numerous mountain fractures, seismic landslides, and other secondary disasters, forming many new potential geological hazard sites, which adds to the difficulty of post-disaster recovery (Huang et al. Citation2012). This article selected a rectangular area (240 km 95 km) around the epicentre as the study area to evaluate seismic landslide susceptibility ().
Following the Wenchuan earthquake, a Mw 6.1 earthquake occurred on August 3, 2014, with the epicentre located near Ludian (103.3°E, 27.1°N), at a focal depth of 12 km. The seismic rupture of this earthquake was the northwest-oriented Baogushan–Xiaohe Fault, which is a secondary strike-slip fault of the Zhaotong–Ludian Fault (). By August 8, 2014, the Ludian earthquake resulted in 617 people dead, 112 people missing, and 3,143 people injured (Hong et al. Citation2016). Given that the Ludian and Wenchuan earthquakes have similar characteristics of geo-environment, the former was selected to verify the transferability of the empirical model of spatial heterogeneity developed based on the latter earthquake.
3. Data and methodology
3.1. Data preparation
Data sources used in this article include landslide inventories, a digital elevation model (DEM), a geological map, ground motion parameters, fault, and river databases (). These data were converted into raster data with a spatial resolution of 90 m and 30 m for Wenchuan and Ludian, respectively, and re-projected to a unified spatial coordinate system (UTM-Zone 48, WGS84 datum).
Table 1. Data sources used in Wenchuan and Ludian.
3.1.1. Seismic landslide inventory
Seismic landslide inventory records the number, location, and size of landslides triggered by earthquakes (Harp et al. Citation2011). A complete landslide inventory is critical for seismic landslide susceptibility assessment and is also an important reference to verify the reliability of assessment results (Chen et al. Citation2020; Citation2021). After the Wenchuan earthquake, different landslide inventories were compiled (Dai et al. Citation2011; Gorum et al. Citation2011; Xu et al. Citation2014). This study selected the landslide inventory released by Xu et al. (Citation2014), compiled based on pre-and post-earthquake high-resolution remote sensing imagery with visual interpretation, due to its high-quality and completeness. The inventory includes 197,481 seismic landslides, affecting an area of ∼110,000 km2. The distribution of landslides triggered by the Wenchuan earthquake shows that seismic landslides were closely related to geo-environmental factors (Yin et al. Citation2009; Qi et al. Citation2010; Xu et al. Citation2014). For example, influenced by river distribution, seismic landslides mainly developed along both sides of rivers, and most seismic landslides distributed near faults, especially the seismogenic fault.
Given that only the landslide source area represents the occurring area, accumulation areas should be excluded from the inventory. This study defined landslide source areas as grid cells with elevations above the median elevation of each landslide (Jibson et al. Citation2000). In the Wenchuan earthquake, landslide source areas comprised nearly 1061.88 km2, accounting for 5.09% of the total area (). For the Ludian earthquake, this article selected the landslide inventory compiled by Xu et al. (Citation2014) for the same consideration and extracted landslide source areas using the same method for Wenchuan (see ).
Seismic landslides fall into deep-seated landslides and shallow landslides according to the landslide thickness. Since the developed method is mainly applicable to shallow landslides, deep-seated landslides need to be removed from the landslide inventory. However, precise landslide thickness is extremely difficult to obtain, making it challenging to distinguish shallow landslides from deep-seated landslides. This article, therefore, calculated the landslide thickness using an area-volume empirical model:
(1)
(1)
(2)
(2)
where
and
represent the landslide volume and the landslide area, respectively. The model parameters mainly obtained from an ordinary regression on data sets from the Longmen Shan landslide site measurements (Parker et al. Citation2011), which has been widely used to quantify the volume of the Wenchuan seismic landslide (Li et al. Citation2014; Fan et al. Citation2018). A landslide is taken as a deep-seated landslide if its thickness is above 10 m (Roering et al. Citation2003; Dou et al. Citation2015) and will be removed. For Wenchuan, the calculated landslide thickness ranges from 0.4 m to 46 m, with 93% shallow landslides. Finally, for Wenchuan and Ludian areas, after removing deep-seated landslides from the landslide inventories, the average thickness values of shallow landslides are 3.54 m and 1.80 m, respectively. This information will be used as the depth information in the subsequent permanent-displacement analysis.
3.1.2. Topography data
DEM is one of the most used topography data in landslide susceptibility assessment. This study used a Shuttle Radar Topography Mission DEM (Van Zyl Citation2001), which can be downloaded from the official website of United States Geological Survey (USGS). Topography-related factors, including slope angle and topography curvature, were extracted from the DEM ().
3.1.3. Geological map and rock mass shear strength parameters
Shear strength parameters ( and
) are assigned based on the mapped geologic units. The distribution of geological units is mainly derived from the 1:250,000 geological map published by China Geological Survey. This study aims to explore the influence of spatial heterogeneity of rock mass on seismic landslide susceptibility assessment, not the produce of accurate rock mass shear strength parameters. Thus, we assigned the rock mass shear strength parameters according to Ma and Xu (Citation2019). Similarly, for the Ludian earthquake area, the lithology was grouped into three lithological groups and assigned representative shear strength for each lithological group (Chen et al. Citation2019). shows rock mass strength parameters assigned in different lithological groups in Wenchuan and Ludian, and shows the lithological group distributions in Wenchuan and Ludian.
Figure 4. Maps of lithological groups of rock mass strength in (a) Wenchuan and (b) Ludian earthquakes.

Table 2. Rock mass strength parameters assigned in different lithological groups in Wenchuan and Ludian.
3.1.4. Ground motion parameters
Ground motion parameters are important input parameters in permanent seismic displacement calculation, including peak ground acceleration (PGA), peak ground velocity, and Arias intensity. The ground motion parameters released by ShakeMap (Wald et al. Citation2005) are considered to be the best estimates of regional ground motions, which are widely used in seismic landslide susceptibility assessment. ShakeMap can provide several ground motion parameters, including PGA, peak ground velocity, and spectral acceleration at different periods. The PGA was selected as the ground motion parameter, since it is an important input parameter for the empirical displacement model selected in this article. shows the PGA maps of the Wenchuan and Ludian earthquakes.
3.1.5. Fault and river data
This article also considered the influence of fault and river distribution on rock mass properties. Specifically, distance to fault and river density were used to represent the fault and river distribution. Distance to fault was calculated based on fault distribution using Euclidean distance tool in QGIS and were then reclassified into six categories using the natural breakpoint classification algorithm, as shown in . For river density, this study selected river distribution with the 5th order, and the river density was calculated using density calculation tool in QGIS and was then reclassified into six categories, as shown in .
3.2. Methodology
3.2.1. Principle of permanent-displacement analysis
3.2.1.1. Infinite-slope model
The infinite-slope model takes a potential landslide as a sliding rigid block resting on an inclined plane, which is a commonly used model in permanent-displacement analysis and can effectively simulate thinner landslides in a stiffer material, such as shallow landslides (Jibson Citation2011). Since shallow landslides are the main type of earthquake-induced landslides (Keefer Citation1984; Citation2002), the infinite-slope model is suitable for modelling seismic landslides and can be used for seismic landslide susceptibility assessment (Wieczorek et al. Citation1985). shows an infinite-slope model under static conditions
3.2.1.2. Critical acceleration
In infinite-slope model, the resistance force () of the sliding-block is determined by the rock mass properties, which are usually expressed by the Mohr-Coulomb parameter (i.e. the effective cohesion (
) and the internal friction angle (
)).
(3)
(3)
Where, and
denote the unit weight of the sliding-block and water, respectively.
and
are the saturation and depth of the sliding-block, respectively.
is the contact area between the sliding-block and the sliding surface.
represents the slope angle of the sliding surface.
Under the earthquake condition, the effective sliding force () of the sliding-block is mainly composed of a gravity and the seismically driven load along the downward direction, which can be calculated as follow:
(4)
(4)
where
is the ground motion acceleration and
is the acceleration of gravity, usually taken 9.80 m/s2. Suppose that the sliding-block is in the limit equilibrium state (
), the ground motion acceleration at this time is the critical acceleration of the sliding-block (
), which is the minimum ground motion acceleration required to make the sliding-block slide. By combining EquationEq. (3)
(3)
(3) and EquationEq. (4)
(4)
(4) , the critical acceleration can be calculated as follows (Newmark Citation1965):
(5)
(5)
The above equation can also be further simplified as a function of the static safety factor of the sliding-block and the inclination angle of sliding surface.
(6)
(6)
where
is the static safety factor of the sliding-block, which can be expressed as:
(7)
(7)
The critical acceleration of the sliding-block depends on 1) the physical-mechanical parameters of the material of sliding-block (i.e. the effective cohesion, the effective friction, and the unit weight of the rock mass), 2) the inclination angle of the sliding surface, 3) the depth and saturation of the sliding-block. Here, the physical-mechanical parameters of the rock mass can be obtained using in-situ measurements or assigned empirical values based on geological unit. The inclination angle of the sliding surface is usually replaced by the topography slope. The depth () and the saturation (
) of the sliding-block are two important parameters that affect the sliding force of the sliding-block, which are challenging to obtain at the regional scale. State-of-the-art often assigned constant values to
and
In this article, the average landslide thickness in landslide inventory is used as the depth (
) parameter of the sliding-block for Wenchuan and Ludian earthquakes, and the saturation parameter of the sliding-block (
) is set to 0 since there was no heavy precipitation before Wenchuan and Ludian earthquakes.
3.2.1.3. Permanent seismic displacement calculation
Permanent seismic displacements can be calculated in two ways. The first way requires a complete time record of ground motion acceleration, and the displacement is calculated by quadratic integration of the time record of ground motion acceleration above the critical acceleration. Since the time record of ground motion acceleration contains the earthquake characteristics (e.g. magnitude, source mechanism), the calculated displacements are reliable. However, a complete time record of ground motion acceleration is limited, especially at a regional scale, which makes it relatively difficult to apply. The second way uses an empirical displacement model to calculate the displacement. The empirical displacement model can be established based on a large number of time records of ground motion acceleration from global/regional. For the latter one, the time record of ground motion acceleration was replaced with ground motion parameters, such as PGA. Compared to the first method, the second method has been widely used in practice, and thus we compared four benchmark empirical displacement models and selected the best one to calculate the permanent seismic displacement.
3.2.2. Spatial heterogeneity analysis of rock mass strength
3.2.2.1. Lithological environment analysis
Lithological environment refers to the external geo-environment in which the outcropping lithology is located, which is one of the main causes leading to the spatial heterogeneity of rock mass strength. Accordingly, the spatial heterogeneity of rock mass strength can be quantified based on the lithological environment difference. Considering the importance, availability, and generalization of the lithological environment, we selected three major factors to represent the lithological environment, including river, fault, and topography curvature.
The river represents the hydrological impact of geo-environment. The seasonal fluctuation of river level can affect rock mass properties. Besides, the erosion and scouring of the river are easy to form high-steep terrains that in turn accelerate the deformation and destruction of the slope. Distance to river or river density is commonly used to reflect the river distribution. This study selected the river density as a hydrological factor.
The fault distribution reflects the regional tectonic environment. Influenced by tectonic activity, the rock mass in the near-fault often develops dense structural surfaces, leading to low rock mass strength, and such region is prone to form seismic landslides. In this article, distance to fault was selected as the tectonic factor.
Topography curvature reveals the degree of concavity and convexity of the terrain. In abrupt topographic change areas, such as concave and convex slopes, the stress redistribution and stress cracks are easily formed, which reduces the rock mass strength on the slope. Therefore, the topography curvature was also selected as a lithological environmental factor.
3.2.2.2. Multivariate analysis
Earthquakes and their associated seismic landslides provide an opportunity to quantify the rock mass strength at a regional scale (Jeandet et al. Citation2019; Shinoda et al. Citation2019). Under earthquake condition, the earthquake provides a regional shear-strength test where the earthquake generates a measurable forcing (ground motion acceleration) and an observable response (seismic landslide) which depend on the rock mass strength (Gallen et al. Citation2015). Hence, a well-documented seismic landslide inventory, including location, volume, source area, and types of all seismic landslides, is critical in quantifying the rock mass properties.
Lithologic environmental factors contribute differently to the rock mass strength, affecting the spatial distribution of seismic landslides. Therefore, the spatial distribution of seismic landslides synthetically reflects the differences of lithologic environments. This article adopted logistic regression analysis (LRA) (Mousavi et al. Citation2011; Xie et al. Citation2018; Cao et al. Citation2019) to estimate contributions of different lithologic environmental factors to the rock mass strength based on the seismic landslide inventory.
The dependent variable in LRA is binary (e.g. 0/1), where Y = 0 represents the non-landslide occurrence and Y = 1 represents the landslide occurrence. The landslide occurrence is influenced by various geo-environmental factors, which can be expressed as:
(8)
(8)
where
are the geo-environmental factors, including the factors related to rock mass properties, topography factors, and earthquake-related factors; and
are the coefficients of
representing the contribution of each geo-environmental factor to the seismic landslide occurrence.
Odds ratio is the ratio of landslide occurrence probability () to non-landslide occurrence probability (
). The natural logarithm of the odds ratio is a linear expression of the independent variable
(9)
(9)
The coefficient is derived using the maximum likelihood estimation. If
is positive, the geo-environmental factor positively contributes to landslides, while the opposite is a negative contribution.
To estimate the rock mass strength heterogeneity, a linear equation was used to establish the spatial correlation between the rock mass strength heterogeneity and the lithological environment, which can be express as follows:
(10)
(10)
where f is the comprehensive influence of different lithological environmental factors on the rock mass strength. A high value of f represents a high influence of the lithological environment on rock mass strength, indicating a low rock mass strength.
3.2.2.3. Empirical model of the rock mass strength heterogeneity
We selected the landslide inventory compiled by Xu et al. (Citation2014), which recorded most of landslides induced by the Wenchuan earthquake, to develop an empirical model of rock mass strength heterogeneity.
Specifically, to develop an empirical model of rock mass strength heterogeneity, a data set that includes an equal number of landslide points and non-landslide points was required. Landslide points were randomly selected in the landslide source areas, and non-landslide points were randomly selected in areas far from the landslide source areas (at least 1 km away). According to the established data set, the regression coefficient of each geo-environmental factor was calculated, see . A higher coefficient indicates a higher contribution of the geo-environmental factor to landslides. shows that all selected factors positively contribute to seismic landslides except the topography curvature and distance to fault. All geo-environmental factors are statistically correlate to landslides, with significance being 5% confidence level. Among them, the lithology group and PGA have the greatest contributions to the seismic landslide. Besides, since the hard lithology group tends to form high and steep topography, its contribution to seismic landslides is higher than that of the low lithology group.
Table 3. Coefficients of geo-environmental factors calculated using LRA.
According to the lithological environment and the weight coefficients calculated using LRA, an empirical model was developed to estimate the rock mass strength heterogeneity, which can be expressed as follows:
(11)
(11)
where
and
represent the topography curvature, distance to fault, and river density, respectively. It is worth noting that different lithologic environmental factors have different effect on rock mass in different regions, which needs to be reasonably determined according to local conditions. Since the empirical model is developed based on the landslide induced by the Wenchuan earthquake, it is only suitable for areas with similar geo- environments to the Wenchuan earthquake, such as Ludian earthquake.
3.2.3. Procedure of seismic landslide susceptibility assessment
Critical acceleration positively correlates with the rock mass strength and plays an essential role in permanent seismic displacement calculation. Therefore, the rock mass strength heterogeneity can also affect the critical acceleration distribution. To incorporate the rock mass strength heterogeneity in the permanent-displacement calculation, the rock mass strength heterogeneity was normalized to [0.5 1] using a linear equation (EquationEq. (12)(12)
(12) ), and the critical acceleration can be modified with the normalized heterogeneity as follows (EquationEq. (13)
(13)
(13) ):
(12)
(12)
(13)
(13)
where
is the rock mass strength heterogeneity after normalization;
is the critical acceleration without considering the rock mass strength heterogeneity, and
is the modified critical acceleration considering the rock mass strength heterogeneity.
Combining yield acceleration and ground motion parameters, permanent seismic displacements can be quickly obtained. Based on the distribution of permanent seismic displacements, the earthquake affected areas can be categorized into different seismic landslide susceptibility levels.
4. Experimental results
To evaluate the performance of the developed method, this article selected three evaluation metrics: percentage of ground failures captured (), percentage of the area predicted landslides (
), and the difference between
and
(
) (Dreyfus et al. Citation2013), see EquationEq. (14)
(14)
(14) to EquationEq. (16)
(16)
(16) .
(14)
(14)
(15)
(15)
(16)
(16)
where
represents permanent seismic displacement in centimetre, and
represents critical displacement.
In general, a high means that calculated permanent seismic displacement is more reliable, which can capture more observed earthquake-induced landslides. However, a high
often corresponds to a high
in which permanent seismic displacement overestimates landslide susceptibility. To balance
and
this study introduced the
to reflect the validity of permanent seismic displacement. A high
corresponds to a high
and low
As the instabilities frequently occur with a displacement larger than 1 cm (Bray and Travasarou Citation2007), we set the critical displacement threshold to 1 cm. The area with a displacement larger than 1 cm will be predicted as a seismic landslide.
4.1. Case study: Wenchuan earthquake
4.1.1. Permanent seismic displacement calculation
shows the estimated rock mass strength heterogeneity using the empirical model developed in Wenchuan. As shown in , fault distribution controls the spatial distribution of rock mass strength, compared to topographic curvature and river distribution. Influenced by the fault distribution, the rock mass in the near-fault area corresponds to a lower strength, which is conducive to the occurrence of landslides. shows the critical acceleration calculated without considering the rock mass heterogeneity in a previous study (Ma and Xu Citation2019). The critical acceleration ranges from 0.01 g to 1.18 g, with most regions having a critical acceleration greater than 0.2 g. shows the modified critical acceleration considering the rock mass strength heterogeneity in Wenchuan. Critical acceleration ranges from 0.01 g to 1.64 g (). Considering the influence of complex lithologic environmental factors on the rock mass strength, the area with high critical acceleration in is greatly reduced. For example, the critical acceleration in the near fault area has lower value compared to that far away from the fault. Besides, the difference of critical acceleration in is more evident than that presented in , which is meaningful to subsequently divide regional landslide susceptibility.
Figure 8. Spatial heterogeneity of the rock mass strength and the critical acceleration in Wenchuan. (a) the spatial heterogeneity of rock mass strength in Wenchuan estimated using the empirical model, (b) the critical acceleration in Wenchuan calculated without considering the spatial heterogeneity of rock mass strength, and (c) the critical acceleration modified after considering the spatial heterogeneity of rock mass strength.

A suitable empirical displacement model is essential to calculate the regional permanent seismic displacement. To this end, this article compared four empirical displacement models developed based on medium and strong earthquakes, see . The calculated displacements were then compared with the observed seismic landslides of the Wenchuan earthquake. shows that the permanent seismic displacements calculated using different empirical displacement models with and without considering the rock mass strength heterogeneity. This article used a uniform colour scale for display and superimposed seismic landslides of the Wenchuan earthquake. As shown in , different empirical displacement models can obtain different displacements, which corresponds to different seismic landslide assessment result. Specifically, without considering the rock mass strength heterogeneity, the displacement calculated with RS09 has the best prediction accuracy in capturing 71.49% of the observed landslides, while the displacement calculated with Jib07A the lowest prediction accuracy in capturing only 38.41% of the observed landslides. Consideration of the rock mass strength heterogeneity substantially improved the prediction accuracy and validity of displacements calculated by four empirical displacement models. Among them, the prediction accuracy of displacement calculated with Jib07A has improved significantly from 38.41% to 46.26%, and the validity of displacement has increased from 26.42% to 31.37%. Besides, the prediction accuracy of displacement calculated with RS09 also increased from 71.49% to 76.62%, and the validity increased from 42.13% to 47.26%.
Figure 9. Permanent seismic displacements in Wenchuan calculated using different empirical displacement models with and without considering the spatial heterogeneity of rock mass strength.

Table 4. List of empirical displacement models used in this study for permanent seismic displacement calculation.
In summary, among four models, the displacement calculated with Jib07A and Jib07B underestimates the regional seismic landslide susceptibility. By contrast, the RS08 and RS09 have higher prediction accuracy, and thus they are more applicable to the permanent seismic displacement calculation of the Wenchuan earthquake. Compared to RS08, RS09 has higher predictive accuracy as it considers the effect of earthquake magnitude. Therefore, we chose RS09 to calculate the permanent seismic displacement.
4.1.2. Landslide susceptibility assessment
We divided the calculated displacement into three susceptibility levels: low susceptibility (0 < D < 1cm), moderate susceptibility (1 cm ≤ D < 5cm), and high susceptibility (5 cm ≤ D), according to the USGS division criteria (Dreyfus et al. Citation2013). presents two landslide susceptibility maps that consider with/without the rock mass strength heterogeneity. We can see that compared to , areas with moderate susceptibility level in are reduced significantly, especially in the northeast of the study area (e.g. subarea A). Although this area has a dense distribution of faults, it is still of high rock strength after integrating other lithologic environments, and therefore corresponds to a low susceptibility level. In contrast, areas with high susceptibility level in are increased significantly compared to , see subarea B. shows the seismic landslide susceptibility level and the observed seismic landslide developed in each susceptibility level of Wenchuan. After considering the rock mass strength heterogeneity, areas with high susceptibility level increased by 2.74%, and the observed landslides in high susceptible areas increased by 7.37%, compared with that ignoring the rock mass strength heterogeneity. Results suggest that when the rock mass strength heterogeneity is ignored in the permanent seismic displacement calculation, the calculated displacements may underestimate the seismic landslide susceptibility. Therefore, it is necessary to consider the rock mass strength heterogeneity in permanent-displacement analysis for seismic landslide assessment.
Figure 10. Seismic landslide susceptibility assessment of Wenchuan earthquake using the permanent-displacement analysis method without considering the spatial heterogeneity of rock mass strength (a) and with considering the spatial heterogeneity of rock mass strength (b).

Table 5. Seismic landslide susceptibility levels and the observed seismic landslide developed in each susceptibility level of the Wenchuan earthquake.
4.2. Case study: Ludian earthquake
To validate the transferability of the empirical model developed in the Wenchuan earthquake, this study selected Ludian which has a similar geo-environment to Wenchuan, as another case study.
4.2.1. Permanent seismic displacement calculation
shows the permanent seismic displacements calculated using different empirical displacement models with and without considering the spatial heterogeneity of the rock mass strength. Without considering the spatial heterogeneity of the rock mass strength, the displacement calculated with the RS08 model has the best prediction accuracy and validity, with the and
being 67.74% and 55.40%, respectively; while the Jib07B has the worst prediction accuracy and validity, with the
and
being 52.93% and 44.16%, respectively. Unlike the Wenchuan earthquake, when the earthquake magnitude is considered in the empirical displacement model, the prediction accuracy and validity of the calculated displacement are significantly reduced (58.74% vs. 52.93%, 67.74% vs. 56.02%). This may be due to insufficient consideration of ground motion acceleration for moderate earthquake magnitudes when constructing the empirical displacement equation. With considering the spatial heterogeneity of rock mass strength, the prediction accuracy and the validity of the displacements with different empirical regress models were significantly improved, especially for the RS08 model, where the prediction accuracy increased from 67.74% to 82.66%, and the validity increased from 55.40% to 67.30%. Results indicate that considering the spatial heterogeneity of rock strength in the permanent seismic displacement calculation, the calculated displacement can effectively predict seismic landslides. To this end, with considering the spatial heterogeneity of rock mass strength, the RS08 was selected to calculate the permanent seismic displacement in Ludian.
4.2.2. Landslide susceptibility assessment
Similar to the first case study, the calculated permanent seismic displacements in Ludian were divided into low, moderate, and high susceptibility levels, as shown in . We can find that considering the spatial heterogeneity of rock mass strength, the area with moderate to high susceptibility levels increases to some extent (e.g. subarea B) compared to that without considering spatial heterogeneity of the rock mass strength. shows the seismic landslide susceptibility level and the observed seismic landslide developed in each susceptibility level of the Ludian earthquake. After considering the spatial heterogeneity of the rock mass strength, areas fallen in high susceptibility level increased by 1.83%, and the observed landslides in high susceptible areas increased by 14.34%, compared with that without considering the spatial heterogeneity of rock mass strength. The result also indicates that when the rock mass strength heterogeneity is ignored in the permanent seismic displacement calculation, the calculated displacements may underestimate the seismic landslide susceptibility. Besides, as the result in Ludian earthquake, the established empirical model is also applicable to the seismic landslide susceptibility assessment with similar scenarios to Wenchuan, such as Ludian.
Figure 12. Seismic landslide susceptibility assessment of Ludian earthquake using the permanent-displacement analysis method without considering the spatial heterogeneity of rock mass strength (a) and with considering the spatial heterogeneity of rock mass strength (b).

Table 6. Seismic landslide susceptibility levels and the observed seismic landslide developed in each susceptibility level of the Ludian earthquake.
5. Discussions and conclusions
5.1. Discussions
Prior studies demonstrated that assigning rock mass strength is critical in permanent-displacement analysis, and the estimation of spatial heterogeneity of rock mass strength is critical in making a significantly reliable regional seismic landslide susceptibility assessment (Dreyfus et al. Citation2013). Although the importance of spatial heterogeneity of rock mass strength has been recognized (Rathje and Saygili Citation2009; Gallen et al. Citation2015; Qi and Li Citation2018; Rodríguez-Peces et al. Citation2020), few studies have considered the influence of spatial heterogeneity of rock mass strength in permanent-displacement analysis due to the difficulty of obtaining detailed rock strength measurements with spatial location.
Many scholars have quantified rock strength heterogeneity from different perspectives. For example, Gischig et al. (Citation2016) quantifies the spatial heterogeneity of post-earthquake rock strength based on the pre-earthquake damage of slopes and the amplification effect of ground shaking; Cudjoe (Citation2020) quantifies the heterogeneity of rock strength based on engineering rock classification methods using various quantifiers (e.g. joints, fissures). These methods are mainly for specific scenario areas or single slopes, which are not universal for regional scale. On the contrary, this article considers the spatial heterogeneity of rock mass strength, which is caused by lithological environment, and quantifies the spatial heterogeneity based on the lithological environment difference. To this end, we first examined the influence of lithological environment difference on the rock mass strength heterogeneity. Secondly, we proposed a novel method to invert the rock mass strength heterogeneity from a well-documented landslide inventory. Results on two cases show that there is significant heterogeneity in rock mass strength even in the same lithology. Besides, the permanent-displacement model that considers the rock mass strength heterogeneity can more reliably assess the seismic landslide susceptibility, and the susceptibility levels are in line with the observed landslides.
One of the common methods for calculating regional permanent seismic displacements is using empirical displacement models. Various empirical displacement models have been established based on datasets of seismic events in different regions. For example, Delgado et al. (Citation2020) compared and analyzed the applicability of several empirical displacement models in Spain. Results indicate that the selection of an appropriate empirical displacement model depends on the earthquake magnitude, or the critical acceleration used to calculate the displacement. In the same manner, this study compared four empirical displacement models in the permanent-displacement analysis method and selected the empirical displacement model with the best performance by compared with the observed landslides. This study again verifies that different empirical displacement models significantly affect the regional seismic landslide susceptibility assessment. On the other hand, we also found that for moderate to high earthquake magnitude, reasonable consideration of magnitude in the empirical displacement model can improve the reliability of seismic landslide susceptibility assessment, while reduce its reliability for moderate to low earthquake magnitude.
Although this study improves the reliability of the permanent displacement-based approach for assessing seismic landslide susceptibility by considering the spatial heterogeneity of rock strength, some limitations of this study must be acknowledged. The empirical model to estimate the spatial heterogeneity of rock mass strength was developed under a specific geo-environmental condition and earthquake event. When applying the developed method to other regions, the similarity of the geo-environmental conditions and earthquake events with this study must carefully check. Second, source mechanism of earthquake also partially controls the seismic landslide distribution. This article did not consider these two factors due to the data availability. Third, we only established an empirical model of the rock mass strength heterogeneity on a single earthquake event that limits its application to a certain area. To broaden the scope of application, we may consider regional/global earthquake events to further study the rock mass heterogeneity.
5.2. Conclusions
This study proposes a permanent displacement model that takes into account the rock mass strength heterogeneity for regional seismic landslide susceptibility assessment. First, we developed a novel method to quantify the rock mass strength heterogeneity that results from different lithology environmental factors, and an empirical model was designed to estimate the rock mass strength heterogeneity based on the landslide inventory of Wenchuan earthquake. Second, we consider the rock mass strength heterogeneity in the calculation of critical acceleration of the permanent-displacement model. Finally, combining the critical acceleration and ground motion parameters, the permanent seismic displacement and seismic landslide susceptibility were derived. Results showed that the spatial heterogeneity significantly affects regional rock mass strength that in turn influences the seismic landslide susceptibility. The permanent-displacement model that considers the rock mass strength heterogeneity is more suitable for assessing seismic landslide susceptibility than that without considering the rock mass strength heterogeneity.
Disclosure statement
No potential conflict of interest was reported by the authors.
Data availability statement
Datasets used in this study are available from the corresponding author on reasonable request.
Additional information
Funding
References
- Alberti S, Leshchinsky B, Roering J, Perkins J, Olsen MJ. 2022. Inversions of landslide strength as a proxy for subsurface weathering. Nat Commun. 13(1):6049.
- Baroni G, Facchi A, Gandolfi C, Ortuani B, Horeschi D, van Dam JC. 2010. Uncertainty in the determination of soil hydraulic parameters and its influence on the performance of two hydrological models of different complexity. Hydrol Earth Syst Sci. 14(2):251–270.
- Bicocchi G, Tofani V, Ambrosio, M D, Tacconi-Stefanelli C, Vannocci P, Casagli N, Lavorini G, Trevisani M, Catani F. 2019. Geotechnical and hydrological characterization of hillslope deposits for regional landslide prediction modeling. Bull Eng Geol Environ. 78(7):4875–4891.
- Bray JD, Travasarou T. 2007. Simplified procedure for estimating earthquake-induced deviatoric slope displacements. J Geotech Geoenviron Eng. 133(4):381–392.
- Bunn M, Leshchinsky B, Olsen MJ. 2020. Geologic trends in shear strength properties inferred through three-dimensional back analysis of landslide inventories. J Geophys Res Earth Surf. 125(9):e2019JF005461.
- Cao J, Zhang Z, Wang C, Liu J, Zhang L. 2019. Susceptibility assessment of landslides triggered by earthquakes in the western Sichuan plateau. Catena. 175:63–76.
- Chen X, Liu C, Wang M. 2019. A method for quick assessment of earthquake-triggered landslide hazards: a case study of the Mw6. 1 2014 Ludian, China earthquake. Bull Eng Geol Environ. 78(4):2449–2458.
- Chen S, Miao ZL, Wu LX, He YG. 2020. Application of an incomplete landslide inventory and one class classifier to earthquake-induced landslide susceptibility mapping. IEEE J Sel Top Appl Earth Observ Remote Sens. 13:1649–1660.
- Chen S, Miao ZL, Wu LX, Zhang AS, Li QR, He YG. 2021. A one-class-classifier-based negative data generation method for rapid earthquake-induced landslide susceptibility mapping. Front Earth Sci. 9:609896.
- Chen X, Shan X, Wang M, Liu C, Han N. 2020. Distribution pattern of coseismic landslides triggered by the 2017 Jiuzhaigou Ms 7.0 earthquake of China: control of seismic landslide susceptibility. IJGI. 9(4):198.
- Cudjoe F. 2020. Stochastic slope stability analysis accounting for heterogeneity in rock mass [masters thesis]. University of Toronto.
- Dai FC, Xu C, Yao X, Xu L, Tu XB, Gong QM. 2011. Spatial distribution of landslides triggered by the 2008 Ms 8.0 Wenchuan earthquake, China. J Asian Earth Sci. 40(4):883–895.
- Delgado J, Rosa J, Peláez JA, Rodríguez-Peces MJ, Garrido J, Tsigé M. 2020. On the applicability of available regression models for estimating newmark displacements for low to moderate magnitude earthquakes. The case of the Betic Cordillera (S Spain). Eng Geol. 274:105710.
- Dou J, Paudel U, Oguchi T, Uchiyama S, Hayakawa YS. 2015. Shallow and deep-seated landslide differentiation using support vector machines: a case study of the Chuetsu Area, Japan. Terr Atmos Ocean Sci. 26(2–2):227–239.
- Dreyfus D, Rathje EM, Jibson RW. 2013. The influence of different simplified sliding-block models and input parameters on regional predictions of seismic landslides triggered by the Northridge earthquake. Eng Geol. 163:41–54.
- Du J, Glade T, Woldai T, Chai B, Zeng B. 2020. Landslide susceptibility assessment based on an incomplete landslide inventory in the Jilong Valley, Tibet, Chinese Himalayas. Eng Geol. 270:105572.
- Fan X, Juang CH, Wasowski J, Huang R, Xu Q, Scaringi G, van Westen CJ, Havenith H. 2018. What we have learned from the 2008 Wenchuan earthquake and its aftermath: a decade of research and challenges. Eng Geol. 241:25–32.
- Fusco F, Mirus B, Baum R, Calcaterra D, De Vita P. 2021. Incorporating the effects of complex soil layering and thickness local variability into distributed landslide susceptibility assessments. Water. 13(5):713.
- Gallen SF, Clark MK, Godt JW. 2015. Coseismic landslides reveal near-surface rock strength in a high-relief, tectonically active setting. Geology. 43(1):11–14.
- Gischig V, Preisig G, Eberhardt E. 2016. Numerical investigation of seismically induced rock mass fatigue as a mechanism contributing to the progressive failure of deep-seated landslides. Rock Mech Rock Eng. 49(6):2457–2478.
- Godt JW, Sener B, Verdin KL, Wald DJ, Earle PS, Harp EL, Jibson RW. 2008. Rapid assessment of earthquake-induced landsliding. In: Proceedings of the First World Landslide Forum. Tokyo: United Nations University; p. 219–222.
- Gorum T, Fan X, van Westen CJ, Huang RQ, Xu Q, Tang C, Wang G. 2011. Distribution pattern of earthquake-induced landslides triggered by the 12 May 2008 Wenchuan earthquake. Geomorphology. 133(3–4):152–167.
- Guzzetti F, Reichenbach P, Ardizzone F, Cardinali M, Galli M. 2006. Estimating the quality of landslide susceptibility models. Geomorphology (Amsterdam, Netherlands), 81(1–2):166–184.
- Harp EL, Keefer DK, Sato HP, Yagi H. 2011. Landslide inventories: the essential part of seismic landslide hazard analyses. Eng Geol. 122(1–2):9–21.
- Hoek E, Brown ET. 1980. Empirical strength criterion for rock masses. J Geotech Engrg Div. 106(9):1013–1035.
- Hoek E, Brown ET. 1997. Practical estimates of rock mass strength. Int J Rock Mech Min Sci. 34(8):1165–1186.
- Hong H, You J, Bi X. 2016. The Ludian earthquake of 3 August 2014. Geomatics Nat Hazards Risk. 7(2):450–457.
- Huang R, Li W. 2014. Post-earthquake landsliding and long-term impacts in the Wenchuan Earthquake area, China. Eng Geol. 182:111–120.
- Huang R, Pei X, Fan X, Zhang W, Li S, Li B. 2012. The characteristics and failure mechanism of the largest landslide triggered by the Wenchuan earthquake, May 12, 2008, China. Landslides. 9(1):131–142.
- Huang D, Wang G, Du C, Jin F, Feng K, Chen Z. 2020. An integrated sem-newmark model for physics-based regional coseismic landslide assessment. Soil Dyn Earthquake Eng. 132:106066.
- Huang Y, Zhao L. 2018. Review on landslide susceptibility mapping using support vector machines. Catena. 165:520–529.
- Ibrahim MB, Mustaffa Z, Balogun A, Hamonangan Harahap IS, Ali Khan M. 2021. Advanced data mining techniques for landslide susceptibility mapping. Geomatics Nat Hazards Risk. 12(1):2430–2461.
- Jeandet L, Steer P, Lague D, Davy P. 2019. Coulomb mechanics and relief constraints explain landslide size distribution. Geophys Res Lett. 46(8):4258–4266.
- Jibson RW. 1993. Predicting earthquake-induced landslide displacements using Newmark’s sliding block analysis. Transp Res Rec. 1411:9–17.
- Jibson RW. 2007. Regression models for estimating Coseismic landslide displacement. Eng Geol. 91(2–4):209–218.
- Jibson RW. 2011. Methods for assessing the stability of slopes during earthquakes—a retrospective. Eng Geol. 122(1–2):43–50.
- Jibson RW, Harp EL, Michael JA. 2000. A method for producing digital probabilistic seismic landslide hazard maps. Eng Geol. 58(3–4):271–289.
- Keefer DK. 1984. Landslides caused by earthquakes. Geol Soc America Bull. 95(4):406–421.
- Keefer DK. 2002. Investigating landslides caused by earthquakes - a historical review. Survey Geophys. 23(6):473–510.
- Kumar S, Gupta V, Kumar P, Sundriyal YP. 2021. Coseismic landslide hazard assessment for the future scenario earthquakes in the Kumaun Himalaya, India. Bull Eng Geol Environ. 80(7):5219–5235.
- Labuz JF, Zang A. 2012. Mohr-Coulomb failure criterion. Rock Mech Rock Eng. 45(6):975–979.
- Lee JH, Park HJ. 2016. Assessment of shallow landslide susceptibility using the transient infiltration flow model and GIS-based probabilistic approach. Landslides. 13(5):885–903.
- Li G, West AJ, Densmore AL, Jin Z, Parker RN, Hilton RG. 2014. Seismic mountain building: landslides associated with the 2008 Wenchuan earthquake in the context of a generalized model for earthquake volume balance. Geochem Geophys Geosyst. 15(4):833–844.
- Liu J, Shi J, Wang T, Wu S. 2018. Seismic landslide hazard assessment in the Tianshui area, China, based on scenario earthquakes. Bull Eng Geol Environ. 77(3):1263–1272.
- Liu L, Yang C, Wang X. 2021. Landslide susceptibility assessment using feature selection-based machine learning models. Geomechanics and Engineering. 25(1):1–16.
- Ma S, Xu C. 2019. Applicability of two Newmark models in the assessment of Coseismic landslide hazard and estimation of slope-failure probability: an example of the 2008 Wenchuan Mw 7.9 earthquake affected area. J Earth Sci. 30(5):1020–1030.
- Martino S, Battaglia S, Alessandro, F D, Della Seta M, Esposito C, Martini G, Pallone F, Troiani F. 2020. Earthquake-induced landslide scenarios for seismic microzonation: application to the Accumoli area (Rieti, Italy). Bull Earthquake Eng. 18(12):5655–5673.
- Medwedeff WG, Clark MK, Zekkos D, West AJ, Chamlagain D. 2022. Near‐surface geomechanical properties and weathering characteristics across a tectonic and climatic gradient in the Central Nepal Himalaya. JGR Earth Surface. 127(2):e2021JF006240.
- Merghadi A, Yunus AP, Dou J, Whiteley J, ThaiPham B, Bui DT, Avtar R, Abderrahmane B. 2020. Machine learning methods for landslide susceptibility studies: a comparative overview of algorithm performance. Earth Sci Rev. 207:103225.
- Mousavi SZ, Kavian A, Soleimani K, Mousavi SR, Shirzadi A. 2011. GIS-based spatial prediction of landslide susceptibility using logistic regression model. Geomatics Nat Hazards Risk. 2(1):33–50.
- Murphy W, Mankelow JM. 2004. Obtaining probabilistic estimates of displacement on a landslide during future earthquakes. J Earthquake Eng. 8(1):133–157.
- Newmark NM. 1965. Effects of earthquakes on dams and embankments. Geotechnique. 15(2):139–160.
- Nguyen VB, Kim Y. 2020. Rainfall-earthquake-induced landslide hazard prediction by Monte Carlo simulation: A case study of Mt. Umyeon in Korea. KSCE J Civ Eng. 24(1):73–86.
- Parker RN, Densmore AL, Rosser NJ, de Michele M, Li Y, Huang R, Whadcoat S, Petley DN. 2011. Mass wasting triggered by the 2008 Wenchuan earthquake is greater than orogenic growth. Nature Geosci. 4(7):449–452.
- Pourghasemi HR, Teimoori Yansari Z, Panagos P, Pradhan B. 2018. Analysis and evaluation of landslide susceptibility: A review on articles published during 2005-2016 (periods of 2005-2012 and 2013-2016). Arab J Geosci. 11(9):193.
- Qi X, Li D. 2018. Effect of spatial variability of shear strength parameters on critical slip surfaces of slopes. Eng Geol. 239:41–49.
- Qi S, Xu Q, Lan H, Zhang B, Liu J. 2010. Spatial distribution analysis of landslides triggered by 2008.5.12 Wenchuan Earthquake, China. Eng Geol. 116(1–2):95–108.
- Rathje EM, Saygili G. 2009. Probabilistic assessment of earthquake-induced sliding displacements of natural slopes. BNZSEE. 42(1):18–27.
- Reichenbach P, Rossi M, Malamud BD, Mihir M, Guzzetti F. 2018. A review of statistically-based landslide susceptibility models. Earth Sci Rev. 180:60–91.
- Robinson TR, Rosser NJ, Densmore AL, Williams JG, Kincey ME, Benjamin J, Bell HJA. 2017. Rapid post-earthquake modelling of coseismic landslide intensity and distribution for emergency response decision support. Nat Hazards Earth Syst Sci. 17(9):1521–1540.
- Rodríguez-Peces MJ, Román-Herrera JC, Peláez JA, Delgado J, Tsige M, Missori C, Martino S, Garrido J. 2020. Obtaining suitable logic-tree weights for probabilistic earthquake-induced landslide hazard analyses. Eng Geol. 275:105743.
- Roering JJ, Schmidt KM, Stock JD, Dietrich WE, Montgomery DR. 2003. Shallow landsliding, root reinforcement, and the spatial distribution of trees in the Oregon coast range. Can Geotech J. 40(2):237–253.
- Salinas-Jasso JA, Ramos-Zuñiga LG, Montalvo-Arrieta JC. 2019. Regional landslide hazard assessment from seismically induced displacements in Monterrey metropolitan area, Northeastern Mexico. Bull Eng Geol Environ. 78(2):1127–1141.
- Salvatici T, Tofani V, Rossi G, D’Ambrosio M, Tacconi Stefanelli C, Masi EB, Rosi A, Pazzi V, Vannocci P, Petrolo M, et al. 2018. Application of a physically based model to forecast shallow landslides at a regional scale. Nat Hazards Earth Syst Sci. 18(7):1919–1935.
- Saygili G, Rathje EM. 2008. Empirical predictive models for earthquake-induced sliding displacements of slopes. J Geotech Geoenviron Eng. 134(6):790–803.
- Schmidt KM, Montgomery DR. 1995. Limits to relief. Science. 270(5236):617–620.
- Segoni S, Pappafico G, Luti T, Catani F. 2020. Landslide susceptibility assessment in complex geological settings: sensitivity to geological information and insights on its parameterization. Landslides. 17(10):2443–2453.
- Shinoda M, Miyata Y. 2017. Regional landslide susceptibility following the mid Niigata prefecture earthquake in 2004 with Newmark’S sliding block analysis. Landslides. 14(6):1887–1899.
- Shinoda M, Miyata Y, Kurokawa U, Kondo K. 2019. Regional landslide susceptibility following the 2016 Kumamoto earthquake using back-calculated geomaterial strength parameters. Landslides. 16(8):1497–1516.
- Tian Y, Xu C, Hong H, Zhou Q, Wang D. 2019. Mapping earthquake-triggered landslide susceptibility by use of artificial neural network (ANN) models: An example of the 2013 Minxian (China) Mw 5.9 event. Geomatics Nat Hazards Risk. 10(1):1–25.
- Townsend KF, Gallen SF, Clark MK. 2020. Quantifying near-surface rock strength on a regional scale from hillslope stability models. JGR Earth Surface. 125(7):e2020JF005665.
- Tsai H, Tsai C, Chang W. 2019. Slope unit-based approach for assessing regional seismic landslide displacement for deep and shallow failure. Eng Geol. 248:124–139.
- Van Zyl JJ. 2001. The Shuttle Radar Topography Mission (SRTM): a breakthrough in remote sensing of topography. Acta Astronaut (UK). 48(5–12):559–565.
- Wald DJ, Worden BC, Quitoriano V, Pankow KL. 2005. Shakemap manual: technical manual, user’s guide, and software guide. https://pubs.usgs.gov/tm/2005/12A01/pdf/508TM12-A1.pdf.
- Wang Z. 2008. A preliminary report on the great Wenchuan earthquake. Earthq Eng Eng Vib. 7(2):225–234.
- Wang T, Liu J, Shi J, Wu S. 2017. The influence of dem resolution on seismic landslide hazard assessment based upon the Newmark displacement method: a case study in the loess area of Tianshui, China. Environ Earth Sci. 76(17):604.
- Wang Y, Rathje EM. 2015. Probabilistic seismic landslide hazard maps including epistemic uncertainty. Eng Geol. 196:313–324.
- Wang H, Wang G, Wang F, Sassa K, Chen Y. 2008. Probabilistic modeling of seismically triggered landslides using Monte Carlo simulations. Landslides. 5(4):387–395.
- Wang T, Wu SR, Shi JS, Xin P. 2013. Application and validation of seismic landslide displacement analysis based on Newmark model: a case study in Wenchuan earthquake. Acta Geologica Sinica (English Edition). 87(z1):393–397.
- Wieczorek GF, Wilson RC, Harp EL. 1985. Map showing slope stability during earthquakes in San Mateo County, California. https://pubs.er.usgs.gov/publication/i1257E.
- Xie P, Wen H, Ma C, Baise LG, Zhang J. 2018. Application and comparison of logistic regression model and neural network model in earthquake-induced landslides susceptibility mapping at mountainous region, China. Geomatics Nat Hazards Risk. 9(1):501–523.
- Xu C, Xu X, Shen L, Dou S, Wu S, Tian Y, Li X. 2014. Inventory of landslides triggered by the 2014 Ms6. 5 Ludian earthquake and its implications on several earthquake parameters. Seismology and Egology. 36(4):1186.
- Xu C, Xu X, Yao X, Dai F. 2014. Three (nearly) complete inventories of landslides triggered by the May 12, 2008 Wenchuan Mw 7.9 earthquake of China and their spatial distribution statistical analysis. Landslides. 11(3):441–461.
- Yin Y, Wang F, Sun P. 2009. Landslide hazards triggered by the 2008 Wenchuan earthquake, Sichuan, China. Landslides. 6(2):139–152.
- Zou Q, Jiang H, Cui P, Zhou B, Jiang Y, Qin M, Liu Y, Li C. 2021. A new approach to assess landslide susceptibility based on slope failure mechanisms. Catena. 204:105388.