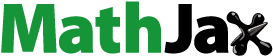
Abstract
The current study analyzed the impact of Land Use Land Cover (LULC) change on streamflow and surface water availability in the Akaki catchment of the Awash Basin, Ethiopia. Soil and Water Assessment Tool (SWAT) and Climatic Water Balance (CWB) models were used. The SWAT model calibrated and validated the daily streamflow. The results indicated that from 1993 to 2016, built-up and barren land areas increased by 5.3% and 3.4%, respectively. The SWAT model over-simulated the peak flows and best simulated the medium and low flows. Based on the calibrated daily streamflow, the runoff was 236.01 mm and 272.59 mm under the 1993 and 2016 LULC scenarios. The total water yield of the catchment was 366.7 mm and 382.01 mm for the 1993 and 2016 LULC scenarios, respectively. The seasonal CWB of the catchment depicted decreasing trend except for the Tsedey (SON) season. Based on the current study’s findings, since LULC change enhanced surface runoff and total water yield in the catchment, proactive flood management is recommended to reduce the impact of the flood hazard on life and property.
1. Introduction
The Land Use Land Cover (LULC) change caused by natural processes and anthropogenic alterations heavily influences the global hydrologic system (Kumar et al. Citation2018; Aragaw et al. Citation2021). These global hydrological processes can determine water availability in a river basin (Yang et al. Citation2021). The change affects soil and water interaction (Gashaw et al. Citation2018; Caballero et al. Citation2022). The impact can also affect surface and atmospheric systems, which are the main sources of surface water availability (Chaemiso et al. Citation2021; Senbore and Oke Citation2021). Hence, the LULC change’s impact on the hydrological processes at a river basin scale is still a timely topic of study worldwide (Muhammed et al. Citation2021).
The impacts of LULC change on river basins are difficult to manage in developing countries due to the ever-increasing population growth (Chaemiso et al. Citation2021; Daba and You Citation2022). The reasons for this are the high rate of LULC change due to the rural-urban migration, increasing demand for agricultural land, and a high rate of unsustainable human-nature interactions (Acebes et al. Citation2021; Degefu et al. Citation2021). The LULC change caused fluctuations in the amount, duration, and pattern of streamflow and hence water availability in the river basins in many regions of Africa (Chanapathi and Thatikonda Citation2020; Measho et al. Citation2020).
In Ethiopia, studies showed that LULC change affected streamflow and hydrological cycle processes in different basins, sub-basins, and catchments (Dibaba et al. Citation2020a; Affessa et al. Citation2022). For instance, a study by Hassen (Citation2022) in Baro Basin indicated that converting forests, shrubs, and grasslands into agricultural lands affects the river’s streamflow and evapotranspiration. Leta et al. (Citation2021) extrapolated that for the period 2019–2050, surface runoff increased by 4.23 percent during the short rain season and 2.0 percent during the dry season due to LULC alterations in the Nashe watershed of the Blue Nile Basin. Cultivated land enhances streamflow throughout the major rainy season and decreases evapotranspiration, but there is unpredictability during the short rainy and dry seasons (Adnan and Atkinson Citation2011; Hyandye et al. Citation2018). In Northwestern Ethiopia, according to Dibaba et al. (Citation2020b), agricultural land expanded by 16 percent over the last 30 years, while forest land areas decreased by 12 percent. This indicated that significant changes in LULC change mean that the catchment’s water balance components are further disrupted since infiltration is reduced and surface runoff is increased (Chilagane et al. Citation2021; Kiprotich et al. Citation2021).
Awash is a highly utilized river in Ethiopia (Gedefaw et al. Citation2018). The sugar factories rely on the river’s water for their sugarcane plantation, and small-scale irrigation schemes that use the river’s water are common in the basin (Wendimu et al. Citation2016). Public and private investors own large-scale agricultural investments (Tufa et al. Citation2018). Moreover, due to the continuous population growth, agricultural land expansion, and urbanization, the Awash basin is known for its high rate of LULC change (Shawul and Chakma Citation2019; Tadese et al. Citation2020a; Damtew et al. Citation2022). These LULC changes increase the burden on the water in the basin, and the net impact is the fluctuation in water availability.
Global scale studies have been conducted on LULC change and its impact on streamflow. For example, Shahid et al. (Citation2018) conducted a study on the impacts of climate change and human activities on streamflow using the ABCD hydrological model. The model was employed to examine how land use and climate change affected runoff generation at the basin level in Pakistan. Cong et al. (Citation2017) also examined runoff record step change points and hydrological variable trends, attributing the changes to environmental variances in China. Previous studies in the Awash basin and elsewhere in Ethiopia identified the impacts of LULC change on surface runoff (Woldesenbet et al. Citation2017; Gashaw et al. Citation2018; Birhanu et al. Citation2019; Dinka and Klik Citation2019; Bulti and Abebe Citation2020; Dibaba et al. Citation2020a). These studies focused on analyzing the impact of climate change and land use change on runoff and runoff changes using diverse methodologies. Studies also tried to assess the impacts of LULC change on surface water availability (Kumar et al. Citation2018; Tadese et al. Citation2020b; Chaemiso et al. Citation2021). These studies contributed to the current understanding of the impact of LULC changes on surface runoff and the water availability in the watershed. However, they are presented in a disentangled way, and the exact relationship between the impact of LULC change on streamflow and hence the surface water availability is missing.
This can be achieved by applying the Soil and Water Assessment Tool (SWAT) to determine the impact of LULC change on surface water and quantify the surface water availability. The analysis of the LULC change on surface water and the subsequent surface water availability provides a complete picture of the LULC dynamics and related water use, irrigation expansion, and watershed management (Gebremicael et al. Citation2018; Aghsaei et al. Citation2020).
LULC change substantially impacts the hydrological processes and affects the process’s elements at the basin and sub-basin levels (Dinka and Klik Citation2019; Karakuş Citation2019). By affecting important water balance elements like groundwater recharge, interception, infiltration, and evaporation, LULC change modifies the precipitation path and basin’s water availability (Mekonnen et al. Citation2018; Wang et al. Citation2020; Nannawo et al. Citation2021). Hence, to fill the research gaps identified earlier, this study analyzed the impact of LULC change on streamflow using the SWAT model and, therefore, the surface water availability using the CWB model. The study was conducted in the Akaki catchment of the Awash basin, central Ethiopia
2. Materials and methods
2.1. Description of the study area
Akaki catchment is situated in the Awash basin, particularly in the upper part of the basin. The absolute location of the catchment is between 8°50'01′'N and 9°13'10′'N latitude and 38°43'42′'E and 39°00'26′'E longitude (). The total area of the catchment is about 801.61 square kilometers. As shown in , there are 15 streamflow outlets in the catchment.
The elevation of the Akaki catchment ranges between 2033 to 3217 meters above mean sea level. The topography undulates the catchment’s northern, western, and southwestern parts and creates a plateau. Rolling plains, steep river banks, valleys, hills, and mountains make up the physiographic elements of the area (Tolera and Chung Citation2021). The southern and southeastern parts of the catchment have gentle morphology and flat land regions (Zeberie Citation2019).
According to the Ministry of Agriculture (Ministry of Agriculture (MoA) Ethiopia, Citation1998), Ethiopia’s climate classification, which was based on temperature and moisture regime, Akaki catchment has a humid to subhumid climate in the highlands and a semiarid climate in the lowlands. The average annual temperature in humid and subhumid highlands is between 16 to 17 °C. The semiarid lowlands have a yearly mean temperature of 19 to 20 °C (Tessema et al. Citation2015). The catchment’s daily maximum temperature range is between 17.1 and 36.3oC, whereas the daily minimum temperature range is 0.6 to 26.1 °C. The precipitation is seasonal in the catchment. The major rainy season lasts from June to September and accounts for over 70% of the annual total precipitation (Tolera and Chung Citation2021). The daily range of total precipitation in the catchment is between 0 and 102 mm. The average annual precipitation in the catchment ranges from 800 to 1400 mm, depending on the elevation difference (Maru et al. Citation2021). Large-scale droughts and floods in the catchment are occasionally caused by the inter-annual precipitation variability in the Belg (MAM) and Kiremt (JJA) seasons (Shawul and Chakma Citation2019; Maru et al. Citation2022).
2.2. Trend analysis of streamflow, precipitation, and temperature
Before running the SWAT and Climatic Water Balance models, the time series trends of the hydro-meteorological data, such as streamflow, precipitation, and temperature, were conducted. The Mann Kendall Sen’s slope was used to analyze the trends of the main hydro-meteorological datasets. The Mann-Kendall test statistic 'S' is calculated using EquationEquation 1(1)
(1) , based on Mann (Citation1945) and Kendall (Citation1948).
(1)
(1)
where
is the Mann-Kendall statistics,
is a time series where the trend is done, which is ranked from
and
ranked from
Each of the data point is taken as a reference point which is compared with the rest of the data points
so that:
(2)
(2)
where
and
are the annual values in years
and
respectively.
It has been documented that when the number of observations is more than 10, the statistic '' is approximately normally distributed with the mean and
becomes 0 (Kendall Citation1948). In this case, the variance statistic is given as (EquationEquation 3
(3)
(3) ):
(3)
(3)
where
is the number of observations and
are the ties of the sample time series. The test statistics
is calculated as EquationEquation 4
(4)
(4) :
(4)
(4)
2.3. Model description
Two models were used in the current study. The impact of the LULC change on streamflow was conducted using the SWAT model. The surface water availability was quantified using the Climatic Water Balance model.
2.3.1. Swat model
SWAT estimates major hydrological processes such as percolation, surface flow, infiltration, evapotranspiration (ET), aquifer flows, and shallow aquifer (Mapes and Pricope Citation2020). It is a popular model for simulating the influence of LULC change on streamflow at the catchment level. It can simulate bulk input data such as LULC change, soil, streamflow, precipitation, temperature, and digital elevation model (Anand et al. Citation2018). The other quality of the SWAT model is that it is integrated with ArcGIS software as an extension. This makes the model suitable for simulating LULC change and its impact on streamflow (Belihu et al. Citation2020).
The SWAT model uses the water balance equation to simulate the hydrological cycle presented in EquationEquation 5(5)
(5) (Neitsch et al. Citation2011).
(5)
(5)
where
is the final soil water content (mm),
is the initial soil water content on day
(mm),
is the amount of precipitation on day
(mm),
is the amount of surface runoff on day
(mm),
is the amount of evapotranspiration on day
(mm),
is the amount of water entering the vadose zone from the soil profile on day
(mm), and
is the amount of return flow on day
(mm).
This study used the ArcMap extension of ArcSWAT and the computer program SWAT-CUP 12. The ArcSWAT was employed to set up the SWAT project, delineate the watershed, input DEM, LULC, soil, and slope data, integrate weather data, and run the SWAT model in Arc Map. The SWAT-CUP model was utilized to calibrate and validate the SWAT model using streamflow data. This model enables the SWAT model’s calibration, validation, sensitivity, and uncertainty analyses (Khalid et al. Citation2016).
In calibrating and validating the SWAT model, three performance metrics were applied. These are the R-Square Coefficient (R2), Nash–Sutcliffe Efficiency (NSE), and Percent bias (PBIAS). The R2 is a metric that measures the strength of the association between the data and the fitted regression line (Bennour et al. Citation2022). It ranges between 0 and 1; the closer the R2 value to 1, the less error variance is. The NSE compares the relative magnitudes of the residual ‘noise’ and the variance of the data. Its value is between -∞ and 1, where NSE > 0.5 is acceptable (Schuol and Abbaspour Citation2007). The relative bias, or PBIAS, determines whether the model data are greater or smaller than the observations. Its best value is 0 (Goshime et al. Citation2019).
2.3.2. Climatic water balance (CWB)
CWB characterizes the water availability in an area using precipitation and PET as factors (Hargreaves and Samani Citation1982). Before applying the CWB model, it is necessary to calculate the PET for a specific time. Although there are different methods of PET calculations based on the data availability, the Hargreaves and Samani (Citation1982) method is the most convenient one. The method uses the minimum and maximum temperatures and solar radiation to estimate PET using EquationEquation 6(6)
(6) .
(6)
(6)
where
is the potential evapotranspiration (mm/day),
is the average temperature (in oC),
and
are the maximum and minimum temperatures (in oC), respectively, and
is extra-terrestrial radiation (in mm/day).
Then climatic water balance is the net difference between precipitation and potential evapotranspiration in a defined period (EquationEquation 7(7)
(7) ).
(7)
(7)
where
is climatic water balance (mm),
is precipitation (mm), and
is potential evapotranspiration (mm/day). The climatic water balance was used to quantify the surface water availability seasonally in the catchment. The model produces the surface water availability in relation to the climate change impacts.
2.3.3. Image processing and classification
After acquiring the Landsat Mapper and Enhanced Thematic Mapper Plus (ETM+) from the United States Geological Survey (USGS) Earth Explorer, image pre-processing was done to increase the quality of the image for classification. Geometrical (spatial georeferencing) and radiometric (image enhancement, noise, and dark object removals) corrections were made to enhance the image. Stacking was done using ENVI 5.3 software to bring the image bands together. Then the corrected image was brought to ArcMap 10.5, masking the image with the Akaki catchment. Using ERDAS Imagine 2015, supervised image classification was performed. Supervised image classification was preferred because we have ground-truthing GPS points to verify the classified land uses on the image. The concept behind supervised classification is that a user can choose sample pixels from an image characteristic of particular classes and then instruct the image processing software to utilize these training sites as references for categorizing all other pixels in the image (Ahmed and Ahmed Citation2012). The high-resolution image of Google Earth linked with ERDAS Imagine and knowledge about the study area aided the classification. Repeated classifications were done to increase the accuracy using the signature editor tool.
Individual pixels on the images were used as validation units for the image accuracy assessment. User’s, producer’s, overall, and Kappa Coefficient assessments were done as part of the accuracy assessment. User’s accuracy measures commission mistakes that correspond to pixels from other classes that the classifier has categorized as belonging to the class of interest (Rwanga and Ndambuki Citation2017). The producer’s assessment indicates the number of omission mistakes corresponding to pixels belonging to the class of interest that the classifier missed (Thapa and Murayama Citation2009). The overall accuracy refers to the percentage of correctly classified samples (Story and Congalton Citation1986). The Kappa Coefficient quantifies the proportionate reduction in error caused by a classification algorithm (Fahsi et al. Citation2000).
Kappa Coefficient was calculated using EquationEquation 8(8)
(8) (Congalton Citation1991).
(8)
(8)
where
2.3.4. Data types and sources
The current study simulated the SWAT model using the required datasets, including LULC, Digital Elevation Model (DEM), soil, 4 km × 4km gridded meteorological data (precipitation, minimum and maximum temperature, relative humidity, solar radiation, wind speed), and observed streamflow gauged at Akaki station.
The LULC map was developed for two different years. Hence, the LULC was done for 1993 and 2016. The years were selected based on a previous study by Maru et al. (Citation2022). These years are non-drought and drought years, respectively, in the Awash basin. This was done to link the SWAT model outputs with the water availability in wet and dry years. The Landsat Thematic Mapper (TM) and Enhanced Thematic Mapper Plus (ETM+) were input data for the LULC maps of 1993 and 2016, respectively. The data were accessed from the USGS Earth Explorer site with a spatial resolution of 30 m × 30 m. Then, as shown in , the LULC maps of 1993 (a) and 2016 (b) were developed to simulate the SWAT model.
The high-resolution ASTER Global Digital Elevation Model (ASTER-GDEM) was also obtained from the USGS Earth Explorer (). It was used as DEM input data for SWAT and to develop a slope map. The slope map of the study area was developed using the slope tool in ArcGIS using DEM as input data. The resolution of the ASTER-GDEM was 30 m × 30m, with the elevation ranges between 2033 and 3217 m above mean sea level ().
The soil data was found from the Food and Agriculture Organization (FAO) of the United Nations digital soil map of the world database and the Ministry of Water and Energy (MoWE). The soil types and codes were manually entered into the SWAT user soil database and their attributes (). There are six soil types in the Akaki catchment (). The Mollic Nitisols (Vp1-3a-283) is the dominant soil type in the catchment, with 58.1% of the catchment’s coverage. The Chromic Cambisols (Lc75-2b-3781) and Eutric Leptosols (Le19-3a-6578), covering 15.6% and 14.1% of the catchment’s area, are the second and third largest soil types, respectively. The other soil types of the catchment are Rhodic Nitisols (Lv2-3b-3535), Eutric Arenosols (Be9-3c-26), and Pellic Vertisols (Ne61-2-3a-5938), which cover the rest area of the catchment.
The meteorological data were obtained from two sources. Daily precipitation, minimum, and temperature (1990–2016) were accessed from Ethiopia’s National Meteorological Agency (NMA). Due to the absence of the data in expected spatio-temporal scales from the NMA, the study area’s daily relative humidity and wind speed were accessed from the mentioned-above USGS site by adding the location of stations. Ethiopia’s MoWE provided the observed daily streamflow data (1990–2016).
The general methodological flow of the study that combines the use of SWAT, SWAT CUP 12 and R software is presented in .
3. Results and discussions
3.1. Trend analysis of hydro-meteorological data
The hydro-meteorological parameters are the core input datasets for the current study. Such parameters’ increasing or decreasing trend helps compare the outputs with the SWAT and CWB models. For this reason, annual-based Mann Kendall Sen’s slope trend analysis was done for 27 years (1990–2016). The results of the trend analysis are presented in . Accordingly, the annual streamflow trend in the Akaki catchment showed a statistically significant increasing trend at a 99% significance level over the study period. A previously conducted study in the Awash basin by Tadese et al. (Citation2019) indicated a statistically significant increasing trend of streamflow based on station data. An increasing streamflow trend was also found in studies done in different parts of Ethiopia (Gebremicael et al. Citation2017; Gurara et al. Citation2021; Orke and Li Citation2021; Malede et al. Citation2022).
Table 1. Mann Kendall and Sen’s Slope trend analysis for hydro-meteorological data.
As shown in , the total precipitation trend was statistically insignificant, with a p-value of 0.420. The precipitation trend was statistically insignificant in most parts of Ethiopia and the Awash basin (Mulugeta et al. Citation2019; Tadese et al. Citation2019). However, its seasonal variability was high (Esayas et al. Citation2018). In some cases, the precipitation appears during an unexpected season (Belay et al. Citation2017), is delayed from its usually expected season (Kassie et al. Citation2013), or shifts its appearance between two seasons (Orke and Li Citation2021). This high rate of variability affected the smallholder rainfed agricultural production and resulted in food insecurity and poverty in most rural areas in Ethiopia (Mekore and Yaekob Citation2018; Mekonnen et al. Citation2021).
The 27-year trends also indicated that the maximum and minimum temperatures showed a statistically increasing positive trend at 99% of the significance level. This result indicates that the temperature rises in the study catchment, implying high evapotranspiration rates that could affect the surface water availability. This is because temperature increment and evapotranspiration have a direct positive relationship (Al-Sudani Citation2019). The increasing precipitation trend in the catchment could result in an increasing trend of streamflow. This is because precipitation in the catchment is the direct source of streamflow. As recent studies indicate (Shawul and Chakma Citation2019; Daba and You Citation2020; Emiru et al. Citation2021), the long-term precipitation analysis in the Awash basin revealed an increasing trend.
3.2. LULC Classification accuracy assessment
For a supervised classification of different year images, the acceptable standard of accuracy results is above 85% overall accuracy level and over 85% Kappa coefficient (Fugara et al. Citation2009). We took 50 ground truth Global Positioning System (GPS) points for each of the seven land-use types in the Akaki catchment to validate the LULC classification on the 2016 image. The accuracy assessment results indicated a valid supervised image classification for the 2016 image with 96% of the user’s accuracy (calculated by dividing the row total by the total number of valid classifications for a certain class) and 96.38% of the producer’s accuracy (how often does the classified map accurately depict actual ground features). The overall accuracy was 96%, and the Kappa coefficient was 95% ().
Table 2. Classification Accuracy Assessment Matrix (2016).
3.3. LULC Change
Seven LULC classes, including Bush and Shrub Land (BSHL), Forest (FRST), Barren Land (BARR), Grassland (GRSL), Water Body (WATR), Agriculture Land (AGRL), and Built-up Area (BLTU), were classified from the supervised image classification to detect the changes. The classification was performed to detect changes in LULC between 1993 and 2016, with the results summarized in .
Table 3. LULC change between changes the 1993 and 2016.
The results indicated that bush and shrubland, forest, barren land, and built-up area increased from 1993 to 2016, while grassland, water body, and agricultural land decreased. The built-up area was the highest gain LULC class with an increment of 42.08 km2 (5.3%) between 1993 and 2016. This is because of the alarming expansion of Addis Ababa on the surrounding grasslands and agricultural land, which showed a significant decrement of 101.62 km2 (12.7%) and 4.06 km2 (0.5%), respectively. Addis Ababa has been expanded to the surrounding areas mainly because of condominiums and related housing constructions (Koroso et al. Citation2020). The increment in the urban area implies a greater tendency for higher surface runoff (Leta et al. Citation2021; Demissie Citation2022).
Although its amount is small (0.2%), the LULC change of water bodies exhibited a shrinking trend over 27 years (). Many previously conducted studies in Ethiopia (Elias et al. Citation2019; Regasa et al. Citation2021) and the Awash basin (Tadese et al. Citation2020a; Tessema et al. Citation2020) have reported a shrinkage trend of water bodies. This could be due to the transformation of wetlands and swampy areas into bare land and the decrease in river water in the catchment.
On the other hand, the barren land and forest coverages showed an expansion trend in the Akaki catchment. In the Awash basin, as Damtew et al. (Citation2022) reported, the barren land expanded in the basin mainly due to the expansion of fuelwood gathering and overgrazing. Area coverage of the forest land has shown an expansion trend of 5.8% between 1993 and 2016 (). This could be linked to the country’s recent recovery of forest resources (Betru et al. Citation2019) due to government and non-governmental organization-supported household and community-level reforestation and afforestation initiatives (Damtew et al. Citation2022).
The change of LULC in the catchment has many implications for the river’s streamflow and surface water availability. LULC type, soil parameters, and yearly precipitation directly relate to average annual watershed stream flows. For instance, due to the impermeable cover and low infiltration in specific catchment areas, urban land has the largest potential for increased runoff (Chaemiso et al. Citation2021). The reduced water storage capacity of the expanded barren land and the shrinkage in grassland as of 2016 resulted in increased surface water runoff and lateral flows in the catchment.
3.4. SWAT model results
3.4.1. Hydrological response unit (HRU) definition
In the SWAT simulation, the Akaki catchment was divided into 15 sub-basins. The sub-basins were further subdivided into HRUs based on the dominant land use, soil, and slope within each sub-basin. The HRUs are the results of the overlay between the generated slope map () and the soil () and land use maps (). The combination of 12% land use, 15% soil, and 5% slope threshold was used to create the HRUs. Based on the overlay, 153 HRUs were created by the SWAT simulation. These HRUs were also used as input to calibrate the streamflow using SWATCUP12. The HRUs are the smallest divisions of the sub-basin, and an individual HRU does not necessarily reflect the characteristics of the sub-basin.
3.4.2. Sensitivity analysis
The first phase in the SWAT CUP simulation process is sensitivity analysis, which is used to find the model input parameters. Sensitivity analysis aims to evaluate the rate of change in a model’s output for changes in watersheds that cause a significant variation in hydrologic sensitivity (Wang et al. Citation2019). The model’s calibration necessitates identifying critical (sensitive) parameters and precision.
Ten parameters were used in the sensitivity analysis (). Parameters highly related to streamflow, such as temperature, precipitation, groundwater, land use, land cover, surface characteristics, and soil, were prioritized in the selection.
Table 4. Parameters used in the SWAT model sensitivity analysis.
After the sensitivity analysis, the top 4 ranked parameters with better sensitivity were used to calibrate the streamflow (). The sensitivity analyses for the parameters were conducted for 4 years in SWAT CUP using SUFI-2 global sensitivity analysis. The parameters’ sensitivity was evaluated using t-stat and p-value. The ratio between the coefficient of parameters and the standard error gives a t-stat. The parameter is sensitive when the standard error is less than the coefficient. During the sensitivity analysis of any parameter, the smaller the p-value, the more sensitive that parameter is to calibrate the model (Abbaspour Citation2015).
3.4.3. Model calibration
Following adjusting the calibration inputs, observation, extraction, and objective functions in the SWAT Cup, the SWAT model was calibrated for streamflow for the Akaki catchment using the most sensitive parameters for 6 years (1990–1995) using the first 2 years as a warm-up period in Sufi-2 algorithm. Because the Arc SWAT calibration provided an unsatisfactory result, it was necessary to calibrate the results of the Arc SWAT simulations using the SWAT Cup interface. Accordingly, 100 simulations with 10 iterations were performed in the calibration process to get the best calibration outputs. The LULC map of 1993 was used to calibrate the daily streamflow data. Graphical comparison and statistical indices such as Nash Sutcliff Efficiency (NSE), R-Square Coefficient (R2), and Percentage Bias (PBIAS) to the standard deviation of measured data were used to evaluate the performance of the calibrated parameters. The observed and simulated streamflow calibration output coefficients were evaluated using the NSE, R2, and PBIAS. The NSE, R2, and PBIAS for the observed and simulated streamflow for the calibration period were 0.81, 0.83, and −0.54, respectively.
As presented in , based on the statistical model evaluator calibration result, the daily observed and simulated streamflow agreed as predicted by the selected parameters. The SWAT model overestimated the daily high streamflow and underestimated the daily low streamflow. In the medium flow cases, the observed and simulated flows fit better. Abebe and Gebremariam (Citation2019), in their SWAT-based streamflow and sediment yield study in the Kesem watershed of the Awash basin, indicated that SWAT overestimated the streamflow for some months, which had a higher record due to the rainy season. The reason is that SWAT streamflow simulation is highly sensitive to the high streamflow record period during the days of the Kiremt (JJA) season (Spruill et al. Citation2000). This indicates that the SWAT Cup model best simulated medium flow. Precise parameter adjustments and frequent iterations are needed to best simulate high and low flow. The calibration results suggest that using the SWAT model to simulate streamflow in the Akaki catchment is recommended, with a precise selection of sensitive streamflow parameters.
3.4.4. Model validation
Validation is the process of executing a model with parameters selected during the calibration stage and comparing the simulations to data not utilized in the calibration process (Arnold et al. Citation2012). SWAT model validation was conducted for 5 years (2012–2016) for the selected daily streamflow parameters. Due to the bulk nature of the daily streamflow data in a relatively larger study area, we preferred to calibrate and validate the SWAT model for limited years. The LULC map of 2016 was used to validate the streamflow. The model validation’s R2, NSE, and PBIAS were 0.80, 0.79, and −0.24, respectively. As shown in , the observed and simulated daily streamflow of the Akaki catchment depicted a good agreement, though the model overestimated the streamflow during Kiremt (JJA) time. This was due to the high variations in daily streamflow of the catchment during the high precipitation time (June to August).
The daily streamflow simulation between 2012 and 2016 also yielded a peak flow. According to the simulation, the peak flow of the observed and simulated daily streamflow in the Akaki catchment was 469.92 mm and 476.93 mm, respectively. The slight increment of the simulated flow compared to the observed agrees with the previously conducted studies in the Awash basin (Abebe and Gebremariam Citation2019; Tessema et al. Citation2021; Abdulahi et al. Citation2022).
3.5. Climatic water balance model results
The seasonal PET of the catchment was computed based on the 27 years (1990–2016) time series precipitation and temperature data. The reason behind the computation of the seasonal PET is that the water balance gives direct meaning when it is calculated, which directly correlates with the crop growing season. Hence, the PET was computed for the four seasons: Tsedey (SON), Bega (DJF), Belg (MAM), and Kiremt (JJA).
As presented in , the PET was more than 140 mm/day for most years in the Belg (MAM) season of the Akaki catchment. The highest PET was 176 mm/day observed in the Belg (MAM) season. The Bega (DJF) recorded a relatively higher amount of PET than the Tsedey (SON) and Kiremt (JJA) seasons. Higher inter-seasonal variabilities of PET results were observed in the Kiremt (JJA) and Tsedey (SON) seasons. Evapotranspiration is an element of the hydrological process that can influence the amount and quality of surface water (Yadeta et al. Citation2020). Although increasing streamflow trends were observed in the Akaki catchment, the higher amount of calculated PET has influenced surface water availability.
The Mann-Kendall trend analysis results also indicated a significant (95% confidence level) increase in PET trends for the Belg (MAM) and Kiremt (JJA) seasons. The Bega (DJF) season’s Pet al.so showed a significant (at 90% confidence level) increasing trend during the study period (). In the Awash basin, the temperature of Belg (MAM) and Kiremt (JJA) is relatively high (Shawul and Chakma Citation2019) due to the movement of the Intertropical Convergence Zone (ITCZ) to the northern hemisphere (Marriner et al. Citation2012). Belg (MAM) and Kiremt (JJA) are the main rainy seasons in the basin and Ethiopia when farmers fully engage in agricultural activities, especially in the rainfed farming system (Belihu et al. Citation2020).
Table 5. Mann Kendall and Sen’s Slope seasonal trend analysis for PET.
The climatic water balance of the Akaki catchment showed variations between the four seasons. This concerns the precipitation and temperature variations across the seasons. As shown in , the climatic water balance of the Bega (DJF) seasons was negative for 27 years, indicating the season’s dryness. Winters are dry in the catchment, so the water balance deficit is high. Positive and negative CWB were observed in the Tsedey (SON) and Belg (MAM) seasons, with the relative positives dominating. Tsedey (SON) is the transitional season from cultivation to harvest of the major crops, while Belg (MAM) is preparing the land for rainfed agriculture in most parts of the catchment.
In most parts of the Akaki catchment, Kiremt (JJA) is the main rain season. Hence, as shown in , the CWB is high and positive except for three years compared to the other seasons. The highest CWB, more than 700 mm, was also registered this season. The water availability during this season is crucial for rainfed farming in the catchment (Gummadi et al. Citation2018). Most crops are planted during this season, and the absence of water during the Bega (DJF) is an indicator of drought in the coming harvesting seasons (Temam et al. Citation2019).
The recent years (2014–2016), CWB showed a negative value for all four seasons (). This is due to the increment in PET and the reduction in rainfall. Maru et al. (Citation2022) also indicated that recent years had been characterized by meteorological drought in the Awash basin. Dryness is being expanded in previously wet areas, and drought occurred in the basin’s previously non-drought agroecological zones.
The seasonal CWB Mann Kendall t-test results supported the above results. As presented in , except for the Tsedey (SON) season, statistically significant decreasing trends of CWB were observed in the three seasons. The Bega (DJF) CWB trend was significant at 95%, while the Belg (MAM) and Kiremt (JJA) trends were significant at 90%. The higher PET trends in the Bega (DJF) and Belg (MAM) seasons might have also contributed to the relatively higher decreasing trends in CWB. The results indicate that the seasonal CWB of the Akaki catchment showed a significant decrease in the 27 years. In relation to these results, the water availability in the catchment decreased from 1990 to 2016, implying the meteorological drought dominating the recent years.
Table 6. Mann Kendall and Sen’s Slope seasonal trend analysis for CWB.
3.6. Implications of LULC change on surface water availability
One of the capabilities of the SWAT model is it can quantify the surface water using different parameters. Using LULC 1993 and LULC 2016 scenarios, the outputs of the SWAT model for the Akaki catchment were compared based on some parameters. These parameters can estimate the water availability in the catchment. The average surface runoff increased from 236.01 mm to 272.39 mm between 1993 and 2016 (). Between these years, the two major LULC classes were built-up areas and barren land. In the study period, the built-up area and barren land increased by 5.3% and 3.8%, respectively (). Addis Ababa is located in the study area, and its expansion at the expense of the surrounding rural areas is ever-increasing. The expansion of both land uses contributed positively to the lateral movement of water on the surface, increasing the runoff. According to Eshtawi et al. (Citation2015), built-up and urban expansions yield a rapid lateral surface water flow and increase surface runoff. The increase in surface runoff was attributed mainly to the LULC change because other surface runoff providers, like total aquifer recharge, lateral soil flow, and percolation out of the soil, decreased in the catchment during the study period ().
Table 7. Water availability parameters with the 1993 and 2016 LULC scenarios in the Akaki catchment.
The total water yield of the catchment also increased during the study period. The difference between the 1993 and 2016 LULC scenarios in total water yield is 15.31 mm (). The combined effect of the increasing surface runoff and total water yield in the catchment can be associated with an extreme climate event – flooding. In recent years, flooding has become one of the extreme climate events in the Akaki catchment (Zeberie Citation2019). For instance, Addis Ababa has expanded from 80.1 km2 to 287.9 km2 between 1984 and 2020 (Beshir and Song Citation2021), implying an increasing flood risk in the city. According to this study, the long-term precipitation trend of the city was not significant, and the increased surface runoff was the major contributor to the increasing flooding event.
4. Conclusions
The current study analyzed the impacts of LULC change on the streamflow of the Akaki catchment and the availability of surface water in the catchment using SWAT and Climatic Water Balance models. The study’s results indicated that the 27 years of Mann Kendall and Sen’s Slope trend analysis indicated that daily streamflow and maximum and minimum temperature increased in the catchment. On the other hand, between 1993 and 2016, the LULC of the catchment showed different changes. The major LULC changes during the study period were the increment of built-up area and barren land; and the decrement of grasslands. SWAT slightly over-simulated daily peak flows and best simulated the daily medium and low flows in the catchment. The over-simulated of the peak flows is related to the high variation in daily streamflow during dry and wet days. The results indicated the shift of the rainy season in the catchment from Belg (MAM) to Tsedey (SON).
One of the impacts of the LULC change on streamflow in the Akaki catchment is that surface water increased from 1993 to 2016. The increase in the surface runoff and total water yield could also lead to a climate extreme event – flooding in the catchment. The study also shows that surface water is enhanced in the catchment. There could be two options to manage the excess surface water: utilizing the water for productive purpose and draining it to its final destination properly. In the case of using the water for productive purposes, surface water harvesting and utilizing it through urban agriculture in terms of hydroponics and hydroponics could have a double benefit in utilizing the extra surface water before it causes the problem aiding urban food production. Enhancing the urban areas’ drainage and water disposal system to safely dispose of the generated surface flow to the appropriate area could be another consideration. Unless the surface water is utilized for productive purposes, it could cause flooding, especially in urban areas. In this case, proactive flood management is important to reduce the impacts of flooding on life and property.
Abbreviations | ||
CWB | = | Climatic water balance |
DJF | = | December January February |
ETM | = | Enhanced thematic mapper |
GPS | = | Global positioning system |
HRU | = | Hydrological response unit |
JJA | = | June July August |
LULC | = | Land use land cover |
MAM | = | March April May |
MoWE | = | Ministry of water and energy |
NMA | = | National Meteorology Agency |
NSE | = | Nash–Sutcliffe Efficiency |
SON | = | September October November |
SWAT | = | Soil and Water Assessment Tool |
USGS | = | United States Geological Survey |
Acknowledgments
The authors are grateful and appreciate the provision of the gridded temperature and precipitation data from the National Meteorological Agency (NMA) of Ethiopia and its staff. We also would like to thank Water Land and Ecosystem (WLE), Addis Ababa University, and Wolaita Sodo University for their financial support for the corresponding author to conduct this study.
Data availability statement
The datasets supporting this study are available from the corresponding author upon reasonable request.
Disclosure statement
On behalf of all authors, the corresponding author declares that there is no conflict of interest.
References
- Abbaspour KC. 2015. SWAT-CUP: SWAT calibration and uncertainty programs—a user manual. Dübendorf: Eawag, p. 16–70.
- Abdulahi SD, Abate B, Harka AE, Husen SB. 2022. Response of climate change impact on streamflow: the case of the Upper Awash sub-basin, Ethiopia. J Water Clim Chang. 13(2):607–628.
- Abebe T, Gebremariam B. 2019. Modeling runoff and sediment yield of Kesem dam watershed, Awash Basin, Ethiopia. SN Appl Sci. 1:1–13.
- Acebes P, Iglesias-González Z, Muñoz-Galvez FJ. 2021. Do traditional livestock systems fit into contemporary landscapes? Integrating social perceptions and values on landscape change. Agriculture. 11(11):1107.
- Adnan NA, Atkinson PM. 2011. Exploring the impact of climate and land use changes on streamflow trends in a monsoon catchment. Int J Climatol. 31(6):815–831.
- Affessa GM, Belew AZ, Tenagashaw DY, Tamirat DM. 2022. Land use/cover change impacts on hydrology using SWAT model on Borkena Watershed, Ethiopia. Water Conserv Sci Eng. 7(1):55–63.
- Aghsaei H, Dinan NM, Moridi A, Asadolahi Z, Delavar M, Fohrer N, Wagner PD. 2020. Effects of dynamic land use/land cover change on water resources and sediment yield in the Anzali wetland catchment, Gilan, Iran. Sci Total Environ. 712:136449.
- Ahmed B, Ahmed R. 2012. Modeling urban land cover growth dynamics using multi‑temporal satellite images: a case study of Dhaka, Bangladesh. ISPRS Int J Geoinf. 1(1):3–31.
- Al-Sudani HIZ. 2019. Temperature – potential evapotranspiration relationship in Iraq using thornthwaite method. J Univ Babylon Eng Sci. 27(1):16–25.
- Anand J, Gosain AK, Khosa R. 2018. Prediction of land use changes based on land change modeler and attribution of changes in the water balance of Ganga basin to land use change using the SWAT model. Sci Total Environ. 644:503–519.
- Aragaw HM, Goel MK, Mishra SK. 2021. Hydrological responses to human-induced land use/land cover changes in the Gidabo River basin, Ethiopia. Hydrol Sci J. 66(4):640–655.
- Arnold JG, Moriasi DN, Gassman PW, Abbaspour KC, White MJ, Srinivasan R, Santhi C, Harmel R, Van Griensven A, Van Liew MW. 2012. SWAT: model use, calibration, and validation. Trans ASABE. 55:1491–1508.
- Belay A, Recha JW, Woldeamanuel T, Morton JF. 2017. Smallholder farmers’ adaptation to climate change and determinants of their adaptation decisions in the Central Rift Valley of Ethiopia. Agric & Food Secur. 6(1):13.
- Belihu M, Tekleab S, Abate B, Bewket W. 2020. Hydrologic response to land use land cover change in the Upper Gidabo Watershed, Rift Valley Lakes Basin, Ethiopia. HydroResearch. 3:85–94.
- Bennour A, Jia L, Menenti M, Zheng C, Zeng Y, Asenso Barnieh B, Jiang M. 2022. Calibration and validation of SWAT model by using hydrological remote sensing observables in the Lake Chad Basin. Remote Sensing. 14(6):1511.
- Beshir AA, Song J. 2021. Urbanization and its impact on flood hazard: the case of Addis Ababa, Ethiopia. Nat Hazards. 109(1):1167–1190.
- Betru T, Tolera M, Sahle K, Kassa H. 2019. Trends and drivers of land use/land cover change in Western Ethiopia. Appl Geogr. 104:83–93.
- Birhanu A, Masih I, van der Zaag P, Nyssen J, Cai X. 2019. Impacts of land use and land cover changes on hydrology of the Gumara catchment, Ethiopia. Phys Chem Earth, Parts a/b/c. 112:165–174.
- Bulti DT, Abebe BG. 2020. Analyzing the impacts of urbanization on runoff characteristics in Adama city, Ethiopia. SN Appl Sci. 2:1–13.
- Caballero CB, Ruhoff A, Biggs T. 2022. Land use and land cover changes and their impacts on surface-atmosphere interactions in Brazil: a systematic review. Sci Total Environ. 808:152134.
- Chaemiso SE, Kartha SA, Pingale SM. 2021. Effect of land use/land cover changes on surface water availability in the Omo-Gibe basin, Ethiopia. Hydrol Sci J. 66(13):1936–1962.
- Chanapathi T, Thatikonda S. 2020. Investigating the impact of climate and land-use land cover changes on hydrological predictions over the Krishna river basin under present and future scenarios. Sci Total Environ. 721:137736.
- Chilagane NA, Kashaigili JJ, Mutayoba E, Lyimo P, Munishi P, Tam C, Burgess N. 2021. Impact of land use and land cover changes on surface runoff and sediment yield in the Little Ruaha River Catchment. Open J Modern Hydrol. 11(03):54–74.
- Cong Z, Shahid M, Zhang D, Lei H, Yang D. 2017. Attribution of runoff change in the alpine basin: a case study of the Heihe Upstream Basin, China. Hydrol Sci J. 62(6):1013–1028.
- Congalton RG. 1991. A review of assessing the accuracy of classifications of remotely sensed data. Remote Sens Environ. 37(1):35–46.
- Daba MH, You S. 2020. Assessment of climate change impacts on river flow regimes in the upstream of Awash Basin, Ethiopia: based on IPCC fifth assessment report (AR5) climate change scenarios. Hydrology. 7(4):98.
- Daba MH, You S. 2022. Quantitatively assessing the future land-use/land-cover changes and their driving factors in the upper stream of the Awash River based on the C.A.–Markov model and their implications for water resources management. Sustainability. 14(3):1538.
- Damtew A, Teferi E, Ongoma V. 2022. Farmers’ perceptions and spatial statistical modeling of most systematic LULC transitions: drivers and livelihood implications in Awash Basin, Ethiopia. Remote Sens. Appl.: Soc. Environ. 25:100661.
- Degefu MA, Argaw M, Feyisa GL, Degefa S. 2021. Impact of landscape dynamics and intensities on the ecological land of major cities in Ethiopia. Environ Syst Res. 10(1):19.
- Demissie TA. 2022. Land use and land cover change dynamics and its impact on watershed hydrological parameters: the case of Awetu watershed, Ethiopia. J Sediment Environ. 7(1):79–94.
- Dibaba WT, Demissie TA, Miegel K. 2020a. Watershed hydrological response to combined land use/land cover and climate change in highland Ethiopia: finchaa catchment. Water. 12(6):1801.
- Dibaba WT, Demissie TA, Miegel K. 2020b. Drivers and implications of land use/land cover dynamics in Finchaa catchment, Northwestern Ethiopia. Land. 9(4):113.
- Dinka MO, Klik A. 2019. Effect of land use–land cover change on the regimes of surface runoff—the case of Lake Basaka catchment (Ethiopia). Environ Monit Assess. 191(5):1–13.
- Elias E, Seifu W, Tesfaye B, Girmay W. 2019. Impact of land use/cover changes on lake ecosystem of Ethiopia central rift valley. Cogent Food Agricul. 5(1):1595876.
- Emiru NC, Recha JW, Thompson JR, Belay A, Aynekulu E, Manyevere A, Demissie TD, Osano PM, Hussein J, Molla MB, et al. 2021. Impact of climate change on the hydrology of the Upper Awash River Basin, Ethiopia. Hydrology. 9(1):3.
- Esayas B, Simane B, Teferi E, Ongoma V, Tefera N. 2018. Trends in extreme climate events over three agroecological zones of Southern Ethiopia. Adv Meteorol. 2018:1–17.
- Eshtawi T, Evers M, Tischbein B. 2015. Quantifying the impact of urban area expansion on groundwater recharge and surface runoff. Hydrol Sci J. 61:826–843.
- Fahsi A, Tsegaye T, Tadesse W, Coleman T. 2000. Incorporation of digital elevation models with Landsat-TM data to improve land cover classification accuracy. For Ecol Manage. 128(1-2):57–64.
- Fugara A, Mohammed A, Pradhan B, Ahmed Mohamed T. 2009. Improvement of land-use classification using object-oriented and fuzzy logic approach. Appl Geomat. 1(4):111–120.
- Gashaw T, Tulu T, Argaw M, Worqlul AW. 2018. Modeling the hydrological impacts of land use/land cover changes in the Andassa watershed, Blue Nile Basin, Ethiopia. Sci Total Environ. 619-620:1394–1408.
- Gebremicael TG, Mohamed YA, V. Zaag P, Hagos EY. 2017. Temporal and spatial changes of rainfall and streamflow in the Upper Tekezē–Atbara river basin, Ethiopia. Hydrol Earth Syst Sci. 21(4):2127–2142.
- Gebremicael T, Mohamed Y, van Der Zaag P, Hagos E. 2018. Quantifying longitudinal land use change from land degradation to rehabilitation in the headwaters of Tekeze-Atbara Basin, Ethiopia. Sci Total Environ. 622-623:1581–1589.
- Gedefaw M, Wang H, Yan D, Song X, Yan D, Dong G, Wang J, Girma A, Ali B, Batsuren D, et al. 2018. Trend analysis of climatic and hydrological variables in the Awash river basin, Ethiopia. Water. 10(11):1554.
- Goshime DW, Absi R, Ledésert B. 2019. Evaluation and bias correction of CHIRP rainfall estimate for rainfall-runoff simulation over Lake Ziway watershed, Ethiopia. Hydrology. 6(3):68.
- Gummadi S, Rao K, Seid J, Legesse G, Kadiyala M, Takele R, Amede T, Whitbread A. 2018. Spatio-temporal variability and trends of precipitation and extreme rainfall events in Ethiopia in 1980–2010. Theor Appl Climatol. 134(3-4):1315–1328.
- Gurara MA, Jilo NB, Tolche AD. 2021. Modelling climate change impact on the streamflow in the Upper Wabe Bridge watershed in Wabe Shebele River Basin, Ethiopia. Int J River Basin Manage. :1–13.
- Hargreaves GH, Samani ZA. 1982. Estimating potential evapotranspiration. J Irrig and Drain Div. 108(3):225–230.
- Hassen JM. 2022. Understanding the impact of land use and land cover change on local hydrology: implications for long-term planning in the sore and Geba Watersheds, Southwestern Ethiopia. Open Access Library J. 09(02):1–16.
- Hyandye CB, Worqul A, Martz LW, Muzuka AN. 2018. The impact of future climate and land use/cover change on water resources in the Ndembera watershed and their mitigation and adaptation strategies. Environ Syst Res. 7(1):24.
- Karakuş CB. 2019. The impact of land use/land cover (LULC) changes on land surface temperature in Sivas City Center and its surroundings and assessment of Urban Heat Island. Asia-Pacific J Atmos Sci. 55(4):669–684.
- Kassie BT, Hengsdijk H, Rötter R, Kahiluoto H, Asseng S, Van Ittersum M. 2013. Adapting to climate variability and change: experiences from cereal-based farming in the Central Rift and Kobo Valleys, Ethiopia. Environ Manage. 52(5):1115–1131.
- Kendall MG. 1948. Rank correlation methods, 2nd ed. New York: Hafner.
- Khalid K, Ali MF, Abd Rahman NF, Mispan MR, Haron SH, Othman Z, Bachok MF. 2016. Sensitivity analysis in watershed model using SUFI-2 algorithm. Procedia Eng. 162:441–447.
- Kiprotich P, Wei X, Zhang Z, Ngigi T, Qiu F, Wang L. 2021. Assessing the impact of land use and climate change on surface runoff response using gridded observations and swat+. Hydrology. 8(1):48.
- Koroso NH, Zevenbergen JA, Lengoiboni M. 2020. Urban land use efficiency in Ethiopia: an assessment of urban land use sustainability in Addis Ababa. Land Use Policy. 99:105081.
- Kumar N, Singh SK, Singh VG, Dzwairo B. 2018. Investigation of impacts of land use/land cover change on water availability of Tons River Basin, Madhya Pradesh, India. Model Earth Syst Environ. 4(1):295–310.
- Leta MK, Demissie TA, Tränckner J. 2021. Hydrological responses of watershed to historical and future land use land cover change dynamics of Nashe watershed, Ethiopia. Water. 13(17):2372.
- Malede DA, Agumassie TA, Kosgei JR, Linh NTT, Andualem TG. 2022. Analysis of rainfall and streamflow trend and variability over Birr River watershed, Abbay basin, Ethiopia. Environ Chall. 7:100528.
- Mann HB. 1945. Non-parametric tests against trend. Econometrica. 13(3):245–259.
- Mapes KL, Pricope NG. 2020. Evaluating SWAT model performance for runoff, percolation, and sediment loss estimation in low-gradient watersheds of the Atlantic coastal plain. Hydrology. 7(2):21.
- Marriner N, Flaux C, Kaniewski D, Morhange C, Leduc G, Moron V, Chen Z, Gasse F, Empereur J-Y, Stanley J-D. 2012. ITCZ and ENSO-like pacing of Nile delta hydro-geomorphology during the Holocene. Quat Sci Rev. 45:73–84.
- Maru H, Haileslassie A, Zeleke T, Esayas B. 2021. Analysis of smallholders’ livelihood vulnerability to drought across agroecology and farm typology in the Upper Awash Sub-Basin, Ethiopia. Sustainability. 13(17):9764.
- Maru H, Haileslassie A, Zeleke T, Esayas B. 2022. Agroecology-based analysis of meteorological drought and mapping its hotspot areas in Awash Basin, Ethiopia. Model Earth Syst Environ. 8(1):339–360.
- Measho S, Chen B, Pellikka P, Trisurat Y, Guo L, Sun S, Zhang H. 2020. Land use/land cover changes and associated impacts on water yield availability and variations in the Mereb‐Gash River Basin in the Horn of Africa. J Geophys Res Biogeosci. 125(7):e2020JG005632.
- Mekonnen DF, Duan Z, Rientjes T, Disse M. 2018. Analysis of combined and isolated effects of land-use and land-cover changes and climate change on the upper Blue Nile River basin’s streamflow. Hydrol Earth Syst Sci. 22(12):6187–6207.
- Mekonnen A, Tessema A, Ganewo Z, Haile A. 2021. Climate change impacts on household food security and adaptation strategies in southern Ethiopia. Food Energy Secur. 10(1):e266.
- Mekore G, Yaekob T. 2018. Determinants and its extent of rural poverty in Ethiopia: evidence from Doyogena District, Southern part of Ethiopia. J Econ Int Finance. 10:22–29.
- Ministry of Agriculture (MoA) Ethiopia. 1998. Agro ecological Zones. Natural Resources Management and Regulatory Department in collaboration with German Agency for Technical Cooperation (GTZ). March 1998, Addis Ababa
- Muhammed HH, Mustafa AM, Kolerski T. 2021. Hydrological responses to large-scale changes in land cover of river watershed. J Water Land Dev. 2021(50):108–121.
- Mulugeta S, Fedler C, Ayana M. 2019. Analysis of long-term trends of annual and seasonal rainfall in the Awash River Basin, Ethiopia. Water. 11(7):1498.
- Nannawo AS, Lohani TK, Eshete AA. 2021. Exemplifying the effects using WetSpass model depicting the landscape modifications on long-term surface and subsurface hydrological water balance in bilate basin, Ethiopia. Adv Civ Eng. 2021:1–20.
- Neitsch SL, Arnold Jg Kiniry JR, Williams JR. 2011. Soil and water assessment tool theoretical documentation version 2009. Texas Water Resources Institute.
- Orke YA, Li M-H. 2021. Hydroclimatic variability in the Bilate Watershed, Ethiopia. Climate. 9(6):98.
- Regasa MS, Nones M, Adeba D. 2021. A review on land use and land cover change in Ethiopian basins. Land. 10(6):585.
- Rwanga SS, Ndambuki JM. 2017. Accuracy assessment of land use/land cover classification using remote sensing and GIS. IJG. 08(04):611–622.
- Schuol J, Abbaspour K. 2007. Using monthly weather statistics to generate daily data in a SWAT model application to West Africa. Ecol Modell. 201(3–4):301–311.
- Senbore S, Oke S. 2021. Urban development impact on climate variability and surface water quality in part of Mangaung metropolis of South Africa. Dev Southern Africa. 1–20.
- Shahid M, Cong Z, Zhang D. 2018. Understanding the impacts of climate change and human activities on streamflow: a case study of the Soan River basin, Pakistan. Theor Appl Climatol. 134(1-2):205–219.
- Shawul AA, Chakma S. 2019. Spatiotemporal detection of land use/land cover change in the large basin using integrated approaches of remote sensing and GIS in the Upper Awash basin, Ethiopia. Environ Earth Sci. 78(5):1–13.
- Spruill CA, Workman SR, Taraba JL. 2000. Simulation of daily and monthly stream discharge from small watersheds using the SWAT model. Trans ASAE. 43:1431.
- Story M, Congalton RG. 1986. Accuracy assessment: a user’s perspective. Photogramm Eng Remote Sens. 52:397–399.
- Tadese M, Kumar L, Koech R. 2020b. Long-term variability in potential evapotranspiration, water availability and drought under climate change scenarios in the Awash River Basin, Ethiopia. Atmosphere. 11(9):883.
- Tadese M, Kumar L, Koech R, Kogo BK. 2020a. Mapping of land-use/land-cover changes and its dynamics in Awash River Basin using remote sensing and GIS. Remote Sens Appl: Soc Environ. 19:100352.
- Tadese MT, Kumar L, Koech R, Zemadim B. 2019. Hydro-climatic variability: a characterisation and trend study of the Awash River Basin, Ethiopia. Hydrology. 6(2):35.
- Temam D, Uddameri V, Mohammadi G, Hernandez EA, Ekwaro-Osire S. 2019. Long-term drought trends in Ethiopia with implications for dryland agriculture. Water. 11(12):2571.
- Tessema N, Kebede A, Yadeta D. 2020. Modeling land use dynamics in the Kesem sub-basin, Awash River basin, Ethiopia. Cogent Environ Sci. 6(1):1782006.
- Tessema N, Kebede A, Yadeta D. 2021. Modelling the effects of climate change on streamflow using climate and hydrological models: the case of the Kesem sub-basin of the Awash River basin, Ethiopia. Int J River Basin Manage. 19(4):469–480.
- Tessema SM, Setegn SG, Mörtberg U. 2015. Watershed modeling as a tool for sustainable water resources management: SWAT model application in the Awash River basin, Ethiopia. In: Setegn SG, Donoso MC, editors. Sustainability of integrated water resources management. Cham: Springer. p. 579–606.
- Thapa RB, Murayama Y. 2009. Urban mapping, accuracy, & image classification: a comparison of multiple approaches in Tsukuba City, Japan. Appl Geogr. 29(1):135–144.
- Tolera MB, Chung I-M. 2021. Integrated hydrological analysis of Little Akaki Watershed using SWAT-MODFLOW, Ethiopia. Appl Sci. 11(13):6011.
- Tufa F, Amsalu A, Zoomers E. 2018. Failed promises: governance regimes and conflict transformation related to Jatropha cultivation in Ethiopia. Ecol Soc. 23(4):1–11.
- Wang J, Huo A, Zhang X, Lu Y. 2020. Prediction of the response of groundwater recharge to climate changes in Heihe River basin, China. Environ Earth Sci. 79(1):1–16.
- Wang D, Yu X, Jia G, Wang H. 2019. Sensitivity analysis of runoff to climate variability and land-use changes in the Haihe Basin mountainous area of north China. Agric Ecosyst Environ. 269:193–203.
- Wendimu MA, Henningsen A, Gibbon P. 2016. Sugarcane outgrowers in Ethiopia: "Forced" to remain poor? World Dev. 83:84–97.
- Woldesenbet TA, Elagib NA, Ribbe L, Heinrich J. 2017. Hydrological responses to land use/cover changes in the source region of the Upper Blue Nile Basin, Ethiopia. Sci Total Environ. 575:724–741.
- Yadeta D, Kebede A, Tessema N. 2020. Potential evapotranspiration models evaluation, modelling, and projection under climate scenarios, Kesem sub-basin, Awash River basin, Ethiopia. Model Earth Syst Environ. 6(4):2165–2176.
- Yang D, Yang Y, Xia J. 2021. Hydrological cycle and water resources in a changing world: a review. Geogr Sustain. 2(2):115–122.
- Zeberie W. 2019. Modelling of rainfall-runoff relationship in Big-Akaki watershed, upper Awash Basin, Ethiopia. World News Nat Sci. 27:108–120.