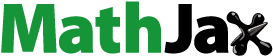
Abstract
Soil erosion and sediment yields are the current limitations and future threats to agriculture, water resources and hydropower projects particularly in developing countries. Estimating the extent and comprehending the spatial distribution of hotspot area is crucial to implement evidence-based soil and water conservation (SWC) measures with limited resources. The study used RUSLE and SDR models in ArcGIS 10.8 environment. The RUSLE model was found to be highly sensitive to C factor followed by LS factor. The result indicated that the annual soil loss varies from 0 to 359.99 t ha−1 yr−1 with 22.31 t ha−1 yr−1 as a mean annual. Besides, the estimated sediment yield ranged from 0 to 42.5 t ha−1 yr−1 with a mean value of 12.02 t ha−1 yr−1. The finding revealed that the central west (SW_5) and northeast (SW_4) parts of the watershed yield higher sediment. The result also signified that about 52.9% of the eroded materials including soil and nutrients are transferred to the outlet. The outcome of our finding undoubtedly aids in the identification of hotspot areas for the adoption of appropriate SWC measures. Hence, adopting RUSLE and SDR for Gununo watershed and another watershed having similar biophysical and environmental factors is suggested.
1. Introduction
Soil erosion and subsequent sediment from watersheds are the main limitations to attain sustainable land use and preserve water quality in streams and lakes (Mutua and Klik Citation2006). Sediment yield (SY) is the sediment load at the outlet of the terrace diversion channels, at the end of the slope length, or sediment from the basins that are considered by the RUSLE model (Gelagay Citation2016). The sediment patterns and transport rates depend on factors like topographic characteristics, land use, soil texture, location of sediment sources and catchment area (Vanmaercke et al. Citation2014). The shearing force of flowing water and raindrop impact is the main driving force for the detachment and transportation of sediment.
Sediment deposition can reduce the reservoir capacity and availability of water for industrial and domestic uses and affect hydropower generation (Kothyari et al. Citation2002). A study conducted by Baral et al. (Citation2014) reported that soil erosion is the reason for the siltation of the reservoir and this process potentially affects the hydropower dam. Aga et al. (Citation2019) also reported that sedimentation causes reservoirs to lose 2% of their designed storage volume each year. Similar studies conducted in different sub-catchments of Omo Gibe basin revealed the vulnerability of the basin to erosion and downstream effect of sediment exported (Devi et al. Citation2008; Aneseyee et al. Citation2020; Chaemiso et al. Citation2021; Alemayehu et al. Citation2022). Hence, this study contributes to understand the extent and sources of soil erosion and sediment yield from Gununo watershed.
In Gununo watershed, soil and water conservation practices have been implemented since the 1980s due to launching and mobilization of the Soil Conservation Research Program (SCRP) (Shiferaw et al. Citation2013). However, the impact of this effort was far less than expected, and soil erosion continued to be a serious issue (Madalcho and Tefera Citation2016). This is mainly due to the high population density of over 450 people per square kilometer and consequent change in land use/land cover, inappropriate conservation measures, and lack of information on the spatial pattern of erosion risk areas (Madalcho and Tefera Citation2016; Tsegaye and Awdenegest Citation2018; WZFEDD Citation2008). The widespread sheet, rill and gully erosion observed in the study watershed are the most visible evidence indicated the severity of erosion problem. According to Benjamin (Citation2018) and Estifanos and Gebremariam (Citation2019), the middle streams of the Omo-Gibe basin have significant impact on downstream environment and contribute a high amount of sediment to Gibe III hydropower dam thereby affecting its sustainability. The study area is situated in the middle stream of the Omo-Gibe basin and it is one of the erosion-prone catchments releasing a considerable amount of erosion and sediment. Though its substantial contribution, no attention was given to understand the hydrology and sediment yield.
Generating information on the sources of sediment within the watershed can be used as an indication of the amount of erosion occurring within the watershed (Jain and Kothyari Citation2000). Besides, estimating sediment yield is crucial for many reasons such as planning appropriate soil and water conservation measures, river morphological studies and estimation of chemical concentrations absorbed to sediment particles. Although estimating sediment yield was first industrialized to study the impact of agricultural practices, the data is essentially vital for managing and developing water resource developments. However, estimating sediment yield at the watershed level is very difficult due to huge data requirements and inadequate knowledge of the complex processes involved (De Vente et al. Citation2006). To overcome those problems, different empirical equations have been developed by various scholars such as Sediment Distributed Delivery (SEDD), SWAT (Soil and Water Assessment Tool), Sediment Delivery Ratio (SDR), and others. In Omo-Gibe basin, considerable studies used SWAT to estimate sediment yield (Chaemiso et al. Citation2021; Choto and Fetene Citation2019; Estifanos and Gebremariam Citation2019). However, research conducted to predict soil erosion and sediment yield by relating RUSLE and SDR model is found to be insufficient.
According to Ouyang and Bartholic (Citation1997), accurate prediction of SDR is a significant and effective approach to predict sediment yield. Thus, this study used the SRD model to determine sediment yield from the study watershed. According to Ferro and Minacapilli (Citation1995), SDR is a probability that eroded particles from a given upland area will reach the adjoining stream channel. Its values fall between 0 and 1 that signifies the percentage of gross soil loss that is delivered to the stream network and ultimately to the outlet of the watershed since it neglects deposition in the channel. The values of SDR for a catchment can be affected by transport system, sediment source, catchment physiology, land cover and texture of eroded material (Richard Citation1993; Walling Citation1988).
The RUSLE model (Renard et al. Citation1997), integrated with Geographic Information System (GIS) is widely used for assessing soil loss from rill, inter-rill, and sheet erosion due to less and simple computational input data, less time required to run, and its convenience with GIS environment (Gashaw et al. Citation2018; Yesuph and Dagnew Citation2019). The improvement in GIS and RS technologies assist to achieve the RUSLE input parameters at minimal cost and with reasonable accuracies (Phinzi and Ngetar Citation2019). Moreover, the GIS provides a detailed analysis of single factors by dividing an area into small grid cells and it permits the quantification of individual factors to the overall result. However, the state-of-the-art of the RUSLE and SDR models for predicting soil erosion and sediment yield has some limitations like diverse sediment sources (massive erosion) and quality or resolution of the digital elevation model (Zhou et al. Citation2019). Aside from limitations, in recent studies the integrated application of RUSLE and SDR in data scarce regions like Gununo watershed was found to be significant approach to predict soil erosion and sediment yield (Ahmad et al. Citation2022; Bhattacharya et al. Citation2020; Colman et al. Citation2018; Rajbanshi and Bhattacharya Citation2020). Therefore, due to aforementioned reasons, this study used the RUSLE and SDR models to determine the values of factors in Gununo watershed.
The goals of this study are (1) to identify sediment hotspot areas by quantifying sediment yield and sediment delivery ratio for targeted soil and water conservation intervention; (2) to calibrate and validate the models against measured sediment yield. Thus, the approach and method used in this study is expected to be applicable to other watersheds having similar biophysical and environmental factors within the Omo-Gibe basin and other regions.
2. Materials and methods
2.1. Description of the study area
Gununo watershed is located in Damot Sore woreda of Wolaita Zone in Southern Ethiopia. It was one of the benchmark sites of the African Highlands Initiative (AHI) eco-regional program which works in collaboration with Areka Agricultural Research Centre (Mazengia and Mowo Citation2013). It is located in the mid-stream of the Omo-Gibe basin with a total area of 20,440.89 ha and is geographically located between 6°44’24.0′' and 6°55’33.6′’ N and 37°34’48.0′’ to 37° 46′40.8′’ E (). The watershed is located at about 22 km from Sodo town and 330 km from Addis Ababa (Madalcho and Tefera Citation2016). The topographic configuration of the watershed is characterized by plain lands, plateaus, hills, and rugged mountains.
According to Hurni et al. (Citation2016) classification system, the agroecological zone of the study area is classified as Moist Dega and Moist Weyna Dega; however, the dominant agroecological zone of the study area is Moist Weyna Dega. The study area has a prolonged period of long rains with a peak in the April/May and July/August months () with bimodal rainfall distribution. The study area has mean annual temperature of 19 °C with the mean annual maximum and minimum of 26.1 °C and 11.9 °C, respectively. Its mean annual rainfall ranges from 756 to 1394 mm with 1184 mm. The area experiences the coldest month in September and the warmest month in March.
The soil of the study area is classified as Humic Nitisols, Chromic Luvisols, Eutric Vertisols, and Humic Alisols as shown in . The dominant soil type in the watershed is found to be Humic Nitisols which covers an area of about 17028.08 ha (83.3%) of the total landmass of the watershed followed by Eutric Vertisols which covers an area of about 1651.48 ha (8.08%).
2.2. Methods
2.2.1. Data sources and processing
Both primary and secondary data was generated using field observation, digital soil map, meteorological data, digital elevation model (DEM) and Landsat images () from respective governmental and non-governmental organizations to implement this research. The digital soil map was obtained from the Ethiopian Ministry of Water, Irrigation, and Energy (MoWIE), Landsat-8 OLI/TIRS (Operational Land Imager/Thermal Infrared Sensor) 30 m resolution was obtained from the website of the United States Geographical Survey (USGS) and Shuttle Radar Topographic Mission (SRTM) DEM of 30 m resolution was acquired from NASA earth explorer website. Besides, meteorological data for 32 years (1989–2020) were obtained from Ethiopian National Meteorological Agency (ENMA) and Water and Land Resource Centre of Addis Ababa University (WLRC-AAU) for within (Gununo) and adjacent meteorological stations (Sod Zuria, Areka and Boditi) (). Similarly, ten-year (20112020) measured sediment yield data were collected from WLRC-AAU to calibrate and validate the sediment yield estimated using RUSLE model.
Table 1. Description of Landsat images used in LULC classification.
Data processing and analysis was made by computing and classifying the necessary information of each thematic layer using the ArcGIS 10.8 environment. The ERDAS Imagine 2014 was used for satellite image processing. To predict mean annual soil loss and sediment yield in a spatial domain in ton per hectare per year, factor layers were spatially overlaid and combined cell by cell grid modeling procedure in ArcGIS 10.8 environment. Before being processed together, the various layers of data were re-projected to geographic projection UTM (Universal Transverse Mercator) zone 37° N with WGS 1984 datum. Furthermore, Microsoft office packages were employed to make a chart and run some statistical analyses such as coefficient of determination (R2) and consistency analysis. Meanwhile, XLSTAT 2021 was used to test the homogeneity of annual rainfall data for all stations from the year 1989–2020 and to fill missing datasets.
2.2.2. Estimation of soil loss
The RUSLE method (Renard et al. Citation1997) (EquationEquation 1(1)
(1) ) was used for estimating annual soil loss caused by raindrop impact and associated overland flow from the study area.
(1)
(1)
Each factor layers were developed in raster format as an input for the RUSLE model Accordingly, to map R factor an EquationEquation (2)(2)
(2) developed by Hurni (Citation1985) for the Ethiopian-Eritrean highland conditions that was based on the convenient annual average rainfall data was used. Then, the K factor was estimated using soil type-color approach. Similarly, the LS factor was mapped using an equation developed by Moore and Wilson (Citation1992). The P and C factors were obtained from land use/land cover map and reclassified land use/land cover and slope map respectively.
(2)
(2)
2.2.3. Determination of sediment yield and sediment delivery ratio
Identifying critical sediment source area is often dictated as an effective approach for directing the limited resources to areas of high risk rather than spreading them equally across the entire watershed during implementation of SWC practices (Haregeweyn et al. Citation2013). However, it is not common to directly estimate SY reaching the outlet of the watershed unless additional data exist. The precise estimation of SDR, the capacity to transport a portion of gross erosion that is expected from a given area in a given time interval, was found to be an effective approach to estimate SY (Ouyang and Bartholic Citation1997). Thus, for this study, sediment yield from the entire watershed was computed by multiplying the annual soil loss (A) of the watershed by the value of mean SDR ().
Several SDR determination methods have been developed on the basis of combinations of different physical characteristics of the watershed. However, Williams and Berndt’s (Citation1972) found that the mean stream channel slope (SLP) is more convenient in estimating SDR. This technique was further confirmed by Onyando et al. (Citation2005). Therefore, the sediment delivery ratio map of the watershed was derived from the average channel slope (SLP) in percent for each cell in the flow path by using the following empirical equation (EquationEquation 3(3)
(3) ) which is developed by Williams and Berndt’s (Citation1972). HEC-GeoHMS 10.8 extension in ArcGIS 10.8 environment was used to generate the SLP map of the watershed.
(3)
(3)
2.3. Sensitivity analysis
The RUSLE model has the capability to respond in an unexpected way to input modifications (Renard et al. Citation1997). Thus, a methodological study of the response of selected output RUSLE variables to variation in input parameters using one at a time sensitivity analysis is essential. Sensitivity analysis is an evaluation of the relative changes in the model’s output as a function of relative changes in the values of model input parameters (Gete Citation2000). The sensitivity analysis was carried out to select the most sensitive parameters that direct targeted and selective interventions (Diwediga et al. Citation2018). Accordingly, changes in estimated mean soil loss for the study area by changing one input variable at a time was conducted.
In this study, sensitivity analysis was demonstrated by employing the model to see how much it was sensitive with respect to input changes and thereby to decide which parameter should be subjected to calibration. The five RUSLE parameter mean values were used as a baseline to employ the sensitivity analysis. A widely implemented way of model sensitivity analysis is a one-at-a-time technique, in which a single factor per scenario is increased or decreased (Van Griensven and Meixner Citation2006). Thus, in this study RUSLE model parameters were evaluated for their impact on the output of the model by changing the parameter’s simulated base value by ± 20% turn by turn (Kleijnen Citation2005). Finally, the relative importance of each parameter was evaluated with the help of sensitivity index analysis (EquationEquation 4(4)
(4) ) developed by Gete (Citation2000) as follows;
(4)
(4)
where SI = sensitivity index, PX = model output with X% increase of the variable, MX = model output with X% decrease of the variable
2.4. Model calibration and validation
Model calibration is parameterizing a model to a given set of local conditions. It is aimed to choose the best set of parameters to increase the accuracy and predictability of the actual conditions and eventually to achieve a good fit between predicted and measured values. The RUSLE model was calibrated for the study area following the sensitivity analysis. Manual calibration method was employed to get the best result and it was performed until parameters reach an unchanged level based on suggestion given by Eckhardt and Arnold (Citation2001). To undertake calibration efforts, six-year (2011 – 2016) measured sediment yield data were used.
Model validation is the process of demonstrating that a given site-specific model is capable of making sufficiently accurate predictions. After calibration of uncertain parameters, the model has undergone a validation process. The data used during calibration were not used for model validation. The remaining measured data from the calibration stage (2017–2020) were used for model validation. It was aimed to ensure that the calibrated parameter set performs soundly well under independent dataset.
2.5. Model performance evaluation
To evaluate the precision of model calibration and validation, several statistical measures like coefficient of determination (R2) and percent bias (PBIAS) have been used by several scholars. Accordingly, for this study, both methods were used and made to establish the relationship between observed and estimated data. The value of R2 ranges from 0 to 1 and the more the R2 approaches 1, the better is the performance of the model, while R2 less than 0.5 indicates the poor performance of the model (Gupta et al. Citation1999). The association between the dependent and independent variables was used in describing the model performance relative to the observed data. The coefficient of determination (R2) was employed to determine the goodness of fit between the observed and the final best simulations (EquationEquation 5(5)
(5) ). It was computed as:
(5)
(5)
where Xi is the measured value, Xav is the average measured value, Yi is the simulated value, and Yav is the average simulated value.
The percent bias (PBIAS) measures the average tendency of the predicted data to be larger or smaller than their measured counterparts (EquationEquation 6(6)
(6) ). According to Gupta et al. (Citation1999), the optimum value is zero; positive values indicate a model bias toward underestimation, whereas a negative values indicate a bias toward overestimation. It was calculated as;
(6)
(6)
where SYob is the observed sediment yield and SYpr is the predicted sediment yield
3. Result and discussion
3.1. Computed RUSLE factors
This study resulted in the mean values of individual factor maps as 734.97 MJ mm ha−1 hr−1 yr−1, 0.156 t hr MJ−1 mm−1, 2.13, 0.163, and 0.55, which were rainfall-runoff erosivity, soil erodibility, slope length and gradient, surface cover, and management practice factors respectively. Based on this research finding the annual soil loss rate of the study watershed varies from 0 to 359.99 t ha−1yr−1 with a mean of 22.31 t ha−1yr−1.
3.2. RUSLE sensitive parameter analysis
The effect of each input variable on the sensitivity index in relation to base values of input variables is presented in . The result signified that for this study, the RUSLE model was relatively less sensitive to soil erodibility (K) factor, but it is equally sensitive to rainfall erosivity and management practice factors. The result depicted that the RUSLE model is most sensitive to the cover (C) factor followed by the topographic (LS) factor. This indicates that a small change in estimating the C and LS value may lead to a huge mistake in estimating mean annual soil loss in Gununo watershed. This study is in reasonable agreement with the research finding conducted by Bobe (Citation2004) who documented that change in surface cover and topography brought about the largest reduction in mean soil loss than other input variables in the Hararge region of Ethiopia. The sensitivity result showed that a 20% increase in vegetation cover can reduce the C value from 0.163 to 0.13 and thereby reduce mean annual soil loss by 21.69% (17.46 t ha−1 yr−1). Besides, decreasing the LS factor by 20% (from 2.13 to 1.7) through appropriate land management practice can reduce the mean annual soil loss by 21.69% (17.47 t ha−1 yr−1). In general, the RUSLE model parameters sensitivity is site-specific and can vary across different locations.
Table 2. Sensitivity analysis and change in mean soil loss for the watershed.
3.3. Estimated sediment delivery ratio
The mean SDR of the study watershed was computed as 0.529513 ranging from 0.459 to 0.555 (). This result is in reasonable agreement with the finding of Mengistu et al. (Citation2015) who reported 0.5 mean SDR for the Blue Nile River basin, Ethiopia.
The result signifies that in Gununo watershed, 45.9% to 55.5% of the eroded soil particles passing through the channel system contribute to sediment yield. The SDR value increases with an increase in SLP of the stream (). indicates that from the lower, upper right, and central parts of the watershed, the highest eroded materials pass to the channel and be transported to the outlet of the study watershed. This implies that those streams in this area have a higher capacity to transport eroded material, but they have low storage capacity. In contrary, the central right and lower right parts of the watershed have relatively good storage capacity of eroded soil and has less sediment delivery capacity. Besides, on average, the watershed has about 52.9% of the sediment transport capacity. This shows that 52.9% of the eroded materials including soil and nutrient are transferred to the outlet of the Gununo watershed thereby contributing high sediment yield to Gibe III hydroelectric dam; whereas, the remaining eroded materials of 47.1% are redeposited within the watershed. This may accelerate soil and nutrient loss from productive areas and consequently can affect the productivity of agricultural land. This study revealed that the downstream effect of sediment exported from Gununo watershed is a threat to Gibe III hydropower dam. The silting of dams was also reported from different regions of the world due to accelerated soil erosion in the watersheds ( Amasi et al. Citation2021; Condé et al. Citation2019; Karki et al. Citation2018).
3.4. Spatial distribution of sediment yield
The estimated sediment yield of the watershed varies from 0 mostly in lower parts to 42.5 t ha−1 yr−1 in the central west and northeast parts of the watershed with the mean value of 12.02 t ha−1 yr−1. The overall sediment yield of the watershed was found to be 755839.8 t yr−1. The sediment yield map of the study area () has a similar spatial distribution with the map of sediment delivery ratio (). However, these maps have slight variation in lower parts and it might be attributed to the dominance of lower soil loss rate in lower parts of the study area. This implies that particular combination of high soil loss rate and high SDR produces relatively higher sediment yield.
The estimated sediment yield values are comparable with various previous research reports. For instance, Balthazar et al. (Citation2013) measured regional sediment yield from Atbara and reported 4 to 49 t ha−1 yr−1. Gelagay (Citation2016) also estimated the annual sediment yield rate of 0 to 51 t ha−1 yr−1 for the entire Koga watershed. Haregeweyn et al. (Citation2017) estimated a mean sediment yield of 7.34 t ha−1 yr−1 and annual sediment yield ranged from 0 to 40 t ha−1 yr−1 in the Upper Blue Nile basin.
In this study, subwatershed prioritization was done using mean annual sediment yield to implement appropriate SWC measures (). Given the mean annual sediment delivered SW_4, SW_5, SW_7, SW_8, SW_3, SW_9, SW_6, SW_10, SW_2, and SW_1 got 1 to 10 priority levels in order of their arrangement (). Relatively higher sediment yield was associated with a steep slope in the central west and northeast borders of the watershed (SW_4 and SW_5) and the lower values were recorded from the central east and southeast parts of the watershed (SW_1 and SW_2) (). The subwatersheds (SW_4 and SW_5) which cover an area of about 21.1% of the study area have a mean sediment yield of 38.46 t ha−1 yr−1 and a total of 608081.96 (80.45%) tons per annum. However, irrespective of their area coverage (20.59%) SW_1 and SW_2 contribute a mean of 17.53 t ha−1 yr−1 and a total of 25508.19 (3.38%) tons of sediment yield per annum. This result indicates that the central west and northeast borders are the most sediment delivering subwatershed that require prior soil and water conservation interventions.
Table 3. Contribution of subwatersheds and priority level.
3.5. Model calibration
Out of the ten (2011–2020) years annually measured sediment yield data, two-third (2011–2016) of the data were used for calibration ().
Table 4. Predicted and measured sediment yield data for calibration.
The coefficient of determination (R2) and percent bias measures (PBIAS) were used to evaluate the strength of association between predicted and measured values and the average tendency of the measured and predicted data. Accordingly, the value of R2 was computed as 0.7579. This signified that there is a strong linear association between measured and predicted sediment yield. The PBIAS value ± 25 is rated as satisfactory (Gupta et al. Citation1999). Since the value of PBIAS for this study was found to be −5.24, indicating that the measured and predicted data matched well with a definite tendency to overestimation. below indicated that the measured and predicted sediment yield have a similar trend.
3.6. Model validation
After the calibration of RUSLE input parameters, the outcomes were evaluated whether they symbolize the real soil loss of the watershed through a validation process. It is to determine the quality of outcomes and to check the precise prediction of the model. During validation, the parameter values of calibrated model were kept unchanged, while simulating the input parameters for the years other than the calibration period.
Validation for sediment yield was conducted on annual basis to compare the predicted sediment yield with the measured one. Sediment yield data of an independent validation period (2017 – 2020) was used for validation of the RUSLE model (). Accordingly, the value of R2 resulted in 0.845 (). This value signifies that the predicted sediment has linear association with the measured ones since the R2 value closer to 1 is considered as a good association between measured and predicted values. The PBIAS value 1.06 also signifies that the measured data and its counterpart matched well with definite tendency to model under estimation. Thus, it is possible to use RUSLE for soil loss estimation in Gununo watershed. and show that the sediment yield predicted is almost similar to the measured values.
Table 5. Predicted and measured sediment yield data for validation.
4. Conclusion
This study used primary and secondary data (attribute and spatial) collected from governmental organizations, non-governmental organizations and field visits. Rainfall, 30 m Landsat images, 30 m DEM and soil map were the datasets used to create each RUSLE factor layer in the ArcGIS 10.8 environment. XLSTAT 2021 was used to perform a homogeneity test and fill in missing rainfall data (1989–2020) for all stations. Then, individual factor layers were analyzed separately, resampled to 30 m resolution, and projected to UTM Zone 37 N with WGS 1984 datum in the ArcGIS 10.8 environment before overlaying all raster layers of the RUSLE input parameters. The sediment yield for the study watershed was computed by multiplying annual soil loss with the mean sediment delivery ratio of the watershed which was obtained using the mean main stream channel slope method. Finally, the performance of the model was tested using coefficient of determination and percent bias statistical goodness-of-fit criteria.
Using factors mean value as a base, parameter sensitivity analysis was undertaken and using the sensitive parameters, model calibration was employed. The model was found to be most sensitive to C factor followed by the LS factor; whereas, in this study, the K factor was found to be less sensitive and both the R and P factors were found to be equally sensitive to the model. Besides, the mean sediment delivery ratio of the entire watershed was estimated and found to be 0.529513. This implies that about 52.9% of eroded material transport to the outlet of the watershed. In the study area, the highest sediment yield was recorded from areas having high SDR, which might be affected by the average main channel slope of each cell in the flow path. In the study area those streams located in high SDR area experience high sediment transport capacity and are dominantly situated at the lower, upper and central parts. These areas could be disastrous due to the fact that they carry a huge amount of sediment to the outlet of the watershed and finally to Gibe III hydropower reservoir.
The result of this finding shows that the mean soil loss rate of the watershed was found to be 22.31 t ha−1 yr−1. The result also revealed that the annual sediment yield from the entire watershed ranged from 0 to 42.5 t ha−1 yr−1 with a mean of 12.02 t ha−1 yr−1. From the entire watershed, annually about 755,839.8 tons of sediment were delivered to the outlet of Gununo watershed. The result of this finding shows that the sediment yield prevails in the northeast (SW_4) and central west (SW_5) parts of the watershed. These areas are the most sediment yielding hotspot areas of the watershed which contribute a substantial amount of sediment yield to the streams. The outcome of this study will undoubtedly aid to achieve the sustainability of Gibe III hydropower and natural resources in the study area. Therefore, soil and water intervention measures and stream bank stabilization should be forwarded to maintain sustainable agricultural production system and reduce siltation of Gibe III reservoir. In general, the RUSLE and SDR models predicted the soil loss and sediment yield in the study area well and hence may be used in watersheds having similar biophysical and environmental factors to estimate soil loss, sediment yield and identify hotspot areas to plan and implement evidence-based soil and water conservation measures.
Acknowledgement
The first author appreciates the Southern Agricultural Research Institute for the financial support to cover the research expenses. Authors are very thankful to Ethiopian National Meteorological Agency, Ethiopian Ministry of Water, Irrigation and Energy, and AU-WLRC for providing sediment, soil and climatic data. We also acknowledge the USGS for allowing us to access satellite imageries and SRTM DEM.
Disclosure statement
The authors declare that they have no competing interests to declare.
Data availability statement
The required datasets used for this study are available from the corresponding author on reasonable request.
References
- Abreham BA, Eyasu E, Teshome S, Gudina LF. 2018. Land use/land cover change effect on soil erosion and sediment delivery in the Winike watershed, Omo Gibe Basin, Ethiopia. Sci Total Environ. 728:138776
- Aga A, Melesse A, Chane B. 2019. Estimating the sediment flux and budget for a data limited Rift Valley Lake in Ethiopia. Hydrology. 6(1):1.
- Ahmad WS, Jamal S, Taqi M, El-Hamid HTA, Norboo J. 2022. Estimation of soil erosion and sediment yield concentrations in Dudhganga watershed of Kashmir Valley using RUSLE and SDR model. Env Dev Sustain. 1–24.
- Alemayehu GW, Fufa FA, Mentsiro A. 2022. Assessment of spatial soil erosion using RUSLE model integration with GIS and RS tools: the case of Gilgel Gibe-I Catchment, South West Ethiopia. Amer J Biosci Bioeng. 10(1):10.
- Amasi A,Wynants M,Blake W,Mtei K. 2021. Drivers, impacts and mitigation of increased sedimentation in the hydropower reservoirs of east Africa. Land. 10(6):638.
- Aneseyee AB, Elias E, Soromessa T, Feyisa GL. 2020. Land use/land cover change effect on soil erosion and sediment delivery in the Winike watershed, Omo Gibe Basin, Ethiopia. Sci Total Environ. 728:138776.
- Avery S, Eng C. 2012. Lake Turkana and the Lower Omo: hydrological impacts of major dam and irrigation developments. Oxford, England: African Studies Centre, the University of Oxford.
- Balthazar V, Vanacker V, Girma A, Poesen J, Golla G. 2013. Human impact on sediment fluxes within the Blue Nile and Atbara River basins. Geomorphology. 180:231–241.
- Baral H, Keenan RJ, Sharma SK, Stork NE, Kasel S. 2014. Spatial assessment and mapping of biodiversity and conservation priorities in a heavily modified and fragmented production landscape in north-central Victoria, Australia. Ecol Indic. 36:552–562.
- Benjamin M. 2018. The power of hydrology in the Omo-Gibe River Basin: gibe III and Flood Retreat Agriculture on the River Omo. Lund, Sweden: Lund University.
- Bhattacharya RK, Chatterjee ND, Das K. 2020. Estimation of erosion susceptibility and sediment yield in ephemeral channel using RUSLE and SDR model: tropical plateau fringe region, India. Gully erosion studies from India and surrounding regions (p. 163–185). Cham: Springer.
- Bobe B. 2004. Evaluation of soil erosion in the Harerge region of Ethiopia using soil loss models, rainfall simulation and field trials. Pretoria, South Africa: University of Pretoria.
- Chaemiso SE, Kartha SA, Pingale SM. 2021. Effect of land use/land cover changes on surface water availability in the Omo-Gibe basin, Ethiopia. Hydrol Sci J. 66(13):1936–1962.
- Choto M, Fetene A. 2019. Impacts of land use/land cover change on stream flow and sediment yield of Gojeb watershed, Omo-Gibe basin, Ethiopia. Remote Sens App soc Env. 14:84–99.
- Colman CB, Garcia KMP, Pereira RB, Shinma EA, Lima FE, Gomes AO, Oliveira PTS. 2018. Different approaches to estimate the sediment yield in a tropical watershed. Revista Brasileira de Recursos Hidricos. 23:1–9.
- Condé R,Martinez JM,Pessotto M,Villar R,Cochonneau G,Henry R,Lopes W,Nogueira M. 2019. Indirect assessment of sedimentation in hydropower dams using modis remote sensing images. Remote Sens. 11(3):314.
- De Vente J, Poesen J, Bazzoffi P, Van Rompaey AGV. 2006. Predicting catchment sediment yield in Mediterranean environments: the importance of sediment sources and connectivity in Italian drainage basins. Earth Surf Proc Land. 31(8):1017–1034.
- Devi R, Tesfahune E, Legesse W, Deboch B, Beyene A. 2008. Assessment of siltation and nutrient enrichment of Gilgel Gibe dam, Southwest Ethiopia. Bioresour Technol. 99(5):975–979.
- Diwediga B, Bao L, Agodzo S, Tamene L, Wala K. 2018. Modelling soil erosion response to sustainable landscape management scenarios in the Mo River Basin (Togo, West Africa). Sci Total Environ. 625:1309–1320.
- Eckhardt K, Arnold JG. 2001. Automatic calibration of a distributed catchment model. J Hydrol. 251(1–2):103–109.
- Estifanos TH, Gebremariam B. 2019. Modeling-impact of Land use/cover change on sediment yield (Case Study on Omo-gibe Basin, Gilgel Gibe III Watershed, Ethiopia). American Journal of Modern Energy. 5(6):84–93.
- Ferro V, Minacapilli M. 1995. Sediment delivery processes at basin scale. Hydrol Sci J. 40:703–716.
- Gashaw T, Tulu T, Argaw M. 2018. Erosion risk assessment for prioritization of conservation measures in Geleda watershed, Blue Nile basin, Ethiopia. Environ Syst Res. 6(1):1–14.
- Gelagay H. 2016. RUSLE and SDR model based sediment yield assessment in a GIS and remote sensing environment; A case study of Koga Watershed, Upper, Ethiopia. Hydrol Current Res. 7:239.
- Gete Z. 2000. Landscape dynamics and soil erosion process modeling in the northwestern Ethiopia highlands, African studies series A16. Geographical Bernensia, Berne.
- Gupta H, Sorooshian S, Yapo P. 1999. Status of automatic calibration for hydrologic models: comparison with multilevel expert calibration. J. Hydrol. Eng. 4:135–143.
- Haregeweyn N, Poesen J, Verstraeten G, Govers G, Vente JDE, Nyssen J, Deckers J, Moeyersons J. 2013. Assessing the performance of a spatially distributed soil erosion and sediment delivery model (WATEM/SEDEM) in Northern Ethiopia. Land Degrad. Develop. 24:188–204.
- Haregeweyn N, Tsunekawa A, Poesen J, Tsubo M, Meshesha D, Fenta A, Nyssen J, Adgo E. 2017. Comprehensive assessment of soil erosion risk for better land use planning in river basins: case study of Upper Blue Nile River. Sci Total Environ. 574:95–108.
- Hurni H. 1985. Erosion-productivity-conservation systems in Ethiopia. pp. 654–674. In: Proceeding 4th international conference on soil conservation, 3–9 November 1985. Soil Conservation and Productivity. Maracacy, Venezuela.
- Hurni K, Zeleke G, Kassie M, Tegegne B, Kassawmar T, Teferi E, Moges A, Tadesse D, Ahmed M, Degu Y, et al. 2016. Soil degradation and sustainable land management in the rainfed agricultural areas of Ethiopia: An assessment of the Economic Implications. Economics of Land Degradation (ELD) Ethiopia case study report for the Economics of Land Degradation Initiative.
- Jain MK, Kothyari UC. 2000. Estimation of soil erosion and sediment yield using GIS. Hydrol Sci J. 45(5):771–786.
- Karki S,Thandar AM,Uddin K,Tun S,Aye WM,Aryal K,Kandel P,Chettri N. 2018. Impact of land use land cover change on ecosystem services: a comparative analysis on observed data and people’s perception in Inle Lake, Myanmar. Environ Syst Res. 7(1):1–15.
- Kleijnen JP. 2005. An overview of the design and analysis of simulation experiments for sensitivity analysis. Eur J Oper Res. 164:287–300.
- Kothyari UC, Jain MK, Ranga Raju, KG. 2002. Estimation of temporal variation of sediment yield using GIS/Estimation de la variation temporelle de l’exportation sédimentaire grǎce à un SIG. Hydrol Sci J. 47(5):693–706.
- Madalcho A, Tefera M. 2016. Management of traditional agroforestry practices in Gununo Watershed in Wolaita Zone, Ethiopia. Forest Res. 5:1–6.
- Mengistu D, Bewket W, Lal R. 2015. Soil erosion hazard under the current and potential climate change induced loss of soil organic matter in the Upper Blue Nile (Abay) River Basin, Ethiopia. In Sustainable intensification to advance food security and enhance climate resilience in Africa (p. 137–163. Cham: Springer.
- Moore D, Wilson J. 1992. Length slope factor for the revised universal soil loss equation: simplifed method of solution. J Soil Water Conserv. 47(5):423–428.
- Mutua BM, Klik A. 2006. Estimating spatial sediment delivery ratio on a large Rural Catchment. J Spat Hydrol. 6:64–80.
- Onyando J, Kisoyan K, Chemelil E. 2005. Estimation of potential soil erosion for River Perkerra Catchment in Kenya. Water Resour Manage. 19:133–143.
- Ouyang D, Bartholic J. 1997. Predicting sediment delivery ratio in Saginaw Bay Watershed. The 22nd National Association of Environmental Professionals Conference Proceedings, p. 659–671.
- Phinzi K, Ngetar N. 2019. The Assessment of water-borne erosion at catch ment level using GIS-based RUSLE and remote sensing: a review. Int Soil Water Conserv. 7:27–46.
- Rajbanshi J, Bhattacharya S. 2020. Assessment of soil erosion, sediment yield and basin specific controlling factors using RUSLE-SDR and PLSR approach in Konar river basin, India. Journal of Hydrology. 587:124935.
- Renard KG, Foster GR, Weesies GA, Porter JP. 1997. RUSLE: revised universal soil loss equation. J. Soil Water Conserv. 46:30–33.
- Richard K. 1993. Sedimet delivery and the drainage network. In: K Beven and MJ Kirkby, editors. Channel Network Hydrology (p. 225–254). Chichester, West Sussex, UK: John Wiley and Sons.
- Shiferaw A, Hurni H, Zeleke G. 2013. Long-term changes in soil-based ecological services at three sites in Ethiopia. J Env Microbiol. 1(1):136–144.
- Tsegaye B, Awdenegest M. 2018. Technical evaluation of selected soil conservation practices and farmers’ perception about soil erosion in Donbi Watershed, Wolaita Zone, Southern Ethiopia. Int J Agric Sc Food Technol. 4(2):16–23.
- Van Griensven A, Meixner T. 2006. Methods to quantify and identify the sources of uncertainty for river basin water quality models. Water Sci Technol. 53(1):51–59.
- Vanmaercke M, Poesen J, Broeckx J, Nyssen J. 2014. Sediment yield in Africa. Earth Sci. Rev. 136:350–368.
- Mazengia W, Mowo J. 2013. Role of collective actions in integrated soil and water conservation: the case of Gununo Watershed, Southern Ethiopia. Afr J Water Conserv Sustain. 1(5):81–93.
- Walling DE. 1988. Erosion and sediment yield research-some recent perspectives. J Hydrol. 100:113–141.
- Williams JR, Berndt’s HD. 1972. Slope of the main stream channel to predict sediment delivery ratio.
- WZFEDD. 2008. Zonal basic socio-economic and demographic information. Wolaita Soddo.
- Yesuph AY, Dagnew AB. 2019. Soil erosion mapping and severity analysis based on RUSLE model and local perception in the Beshillo Catchment of the Blue Nile Basin, Ethiopia. Env Syst Res. 8:1–21.
- Zhou M, Deng J, Lin Y, Belete M, Wang K, Comber A, Huang L, Gan M. 2019. Identifying the effects of land use change on sediment export: integrating sediment source and sediment delivery in the Qiantang River Basin, China. Sci Total Environ. 686:38–49.