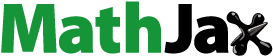
Abstract
The increase in the number and severity of seismic disasters has put communities in danger, especially in rapidly developing and densely populated areas. Traditional seismic vulnerability and risk assessment methods, including field investigation, are accurate at the building scale; however, their low-efficiency and high-cost characteristics limit the application of these methods in urban-scale regions with high-speed development and risk exposure. To address this issue, multisource remote sensing interpretation, support vector machine (SVM) and geographic information science (GIScience) technologies are combined to test the performance and efficiency of a urban-scale macroscopic seismic vulnerability and risk assessment method in the Lixia District of Jinan City, Shandong Province, China, which is characterized by rapid development, a variety of building types, and moderate-to-low seismic risk. First, a traditional field survey was conducted in Lixia District, and a building attribute information database was constructed. Second, the vulnerability proxies of building attribute information and building seismic vulnerability were estimated based on the EMS-98 standard and the SVM. Finally, vulnerability proxies established based on the RISK-UE model were applied to the Lixia database, and the vulnerability and risk assessment under different seismic intensities were estimated with the experimental accuracy verified. The results showed that the SVM method can obtain stable and accurate results in urban scale vulnerability assessment. The mean building vulnerability index in Lixia District is 0.43, which indicates that the overall seismic performance is good. Most of the area falls within the seismic intensity range of VII-X degrees and would experience slight to moderate damage. The results of the study contribute to enhancing the precision and efficacy of large-scale seismic risk assessment, and they can be used by relevant departments to create tailored emergency plans and reduce seismic hazard losses. Additionally, these results can aid in achieving the climate action goal (SDG13) of the United Nations Sustainable Development Goals (SDGs).
1. Introduction
As a natural phenomenon, earthquakes are unpredictable, highly destructive and broadly distributed compared with other natural disaster types, and they seriously threaten the sustainable development of humans and market economies (Thomalla et al. Citation2006; Change IPOCJGI Citation2007; Li et al. Citation2018; Liu et al. Citation2021). The complex geological structure, tectonics, active crustal deformation, and strong seismicity in China have long been threatening to societal development and the safety of the masses (Wang et al. Citation2001; Huang and Zhao Citation2006). At present, earthquakes in China account for approximately 1/3 of all earthquakes globally, making China one of the world’s most earthquake-stricken areas. More than 50% of the total cities and 70% of the large and moderate-size cities in China are located in areas with seismic intensity levels greater than VII (Nie et al. Citation2002). For instance, the Ms 8.0 earthquake in Wenchuan in 2008 damaged more than 5 million houses and killed approximately 69,000 people (Tesfamariam and Liu Citation2010). In 2010, an Ms 7.1 earthquake in Yushu damaged approximately 90% of homesteads and killed 2,698 people (Zhai and Huang Citation2016). In 2013, an Ms 7.0 earthquake hit Ya’an city, killing 196 people and injuring 11,826 others (Jia et al. Citation2014). In 2013, more than 99% of the wooden-structured houses were damaged at the epicenter of the Ms 7.0 earthquake in Lushan County (Xu et al. Citation2013). In 2014, an Ms 6.5 earthquake struck Ludian County in Yunnan Province, collapsing more than 80,000 houses, killing 617 people, and injuring 3,143 people (Lin et al. Citation2015). Still, over time, China has experienced rapid and massive urban expansion (Huang et al. Citation2019). With the tremendous development of the economy and culture, the potential seismic risk has increased to a uniquely new level (Liu, Li, et al. Citation2019). However, it is still impossible to accurately predict the occurrence of earthquakes at present (Maya and Yu Citation2019). Moreover, achieving the Sustainable Development Goals (SDGs) proposed by the United Nations is a common goal for all governments. Therefore, regional seismic vulnerability assessment is an essential task for optimally allocating resources and mitigating the consequences of earthquakes; thus, it should be a focus of governments and decision-makers (Cockburn and Tesfamariam Citation2012, Tesfamariam and Saatcioglu Citation2010).
Seismic vulnerability refers to the sensitivity of a building group to damage due to seismic ground motion (Hill and Rossetto Citation2008; Alam et al. Citation2012). Accurate building data are needed to establish regional-scale assessment models for evaluating seismic hazard risk. Currently, the prevailing treatment vulnerability assessment models are performed by directly deriving the relationship between seismic intensity and damage losses, such as empirical and mechanical methods (Silva et al. Citation2019). The empirical method classifies the damaged buildings after the earthquakes occurred according to a building taxonomy, then evaluates the probability of a certain damage state when the earthquake intensity increases according to the damage data processing results (Ruggieri et al. Citation2022). Different building taxonomy methods, such as FEMA 310 (Citation1998), with different vulnerability factors, such as the quantity of soft stories, heavy overhangs, or short columns, the pounding possibility, the age of the structure, the building height, and visible ground settlement, are used. The Eurocode 8 method (Code PJBECfS Citation2005) includes factors such as the age of the structure, building height, and uncertainty within the model. The EMS-98 method considers vulnerability factors such as the type of structure, roof type, number of floors, and period of construction. Mechanical methods derive vulnerability functions from analytical estimates of seismic behaviour based on numerical models representing various building types (Ruggieri et al. Citation2021). Different seismic vulnerability and risk assessment analysis methods can be fine-tuned based on detailed building inventories to reflect the characteristics of regional building vulnerability (Liu et al. Citation2020; Leggieri et al. Citation2021). The process of creating a detailed inventory of building characteristics based on field surveys is costly and complex, and it is not practical to characterize a single building for a large area in a short time, especially in moderate-to-low seismic-prone regions (Guettiche et al. Citation2017), such as the Lixia District of Jinan City, China.
Recently, simplified macroscale methods have been applied to reduce the inherent cost of in situ building surveys and improve the efficiency of seismic vulnerability at the urban scale (Guéguen et al. Citation2007). Such initiatives consist of simplifying the visual screening stage by only considering the key parameters of seismic vulnerability or by using remote sensing and GIScience methods (French and Muthukumar Citation2006; Mueller et al. Citation2006; Borfecchia et al. Citation2010; Borzi et al. Citation2011; Geiß and Taubenböck Citation2013; Kaushik and Dasgupta Citation2013; Geiß et al. Citation2014; Costanzo et al. Citation2016; Guettiche et al. Citation2017; Li et al. Citation2020; Geiß et al. Citation2022). GIS spatial analysis provides an effective method for predicting the appropriate geographic distribution of data, obtaining complete information on missing data, and establishing the dense distribution of data using small observational data sets (Hamdy et al. Citation2022). In urban-scale seismic risk assessment, GIS management of cadastral databases is useful for analyzing and evaluating the damaging impact of various scenarios. Ródenas et al. (Citation2018) employed GIS to graphically explain the building vulnerability index and predicted damage, as well as to establish the city’s most vulnerable location based on detailed land parcel features. Frigerio et al. (Citation2016) used the qualitative and quantitative methods of spatial analysis and the statistical model of GIS to incorporate social vulnerability into seismic risk analysis in Italy and drew a qualitative social vulnerability exposure map for seismic disasters. Rahman et al. (Citation2015) produced vulnerability maps based on GIS with the FEMA-RVS method, which is useful for relocating people living in vulnerable buildings but only applies to a small portion of the research region.
Machine learning methods (ML) develop rapidly and are widely used in the seismic field. Xie et al. (Citation2020) put forward a comprehensive review based on the research of ML in seismic engineering in recent years. They discussed the application of seven ML methods in seismic hazard analysis, system identification and damage detection, seismic vulnerability assessment, and seismic structure control. Seven ML methods include ANN, support vector machine (SVM), response surface model (RSM), logistic regression (LR), decision tree (DT) and random forest (RF), hybrid methods that couple two or more soft computing algorithms, and all other methods. In seismic vulnerability assessment, the relationship between predictive factors and structural parameters can be effectively quantified by the ML method. RMS and SVM algorithms are commonly used in the development of multi-predictive probabilistic seismic demand models (PSDMs) (de Felice and Giannini Citation2010; Ghosh et al. Citation2018; Sichani et al. Citation2018). SVM is a binary classification algorithm that uses kernel functions to map data into high-dimensional feature spaces. These features can be used to obtain the best proxies for building characteristics, which can be easily evaluated using existing databases to investigate the seismic vulnerability of buildings (Geiß et al. Citation2015; Ramírez Eudave and Ferreira Citation2021). A vulnerability proxy can reflect the urban scale based on targeted small-scale field data. Although rather accurate, seismic risk assessments of individual buildings are ineffective. However, the neighbouring structures with the same attribute features can be split into blocks for analysis, which increases the speed and accuracy of data processing.
Here, a comprehensive method using ML, remote sensing interpretation, and GIS spatial analysis is employed to assess the macroscale seismic vulnerability of the Lixia District, Jinan. In this study, building attribute information is gathered from a variety of sources, including street view pictures, field survey, urban planning, traffic planning, and remote sensing techniques. The building vulnerability in the field survey area was categorized using EMS-98 classifications. Then, using an SVM model, vulnerability proxies were developed to establish connections between various building attribute combinations and their most likely EMS-98 vulnerability classifications. Additionally, the proxy approach was applied to all of Lixia District. Finally, several seismic scenarios in Lixia District were explored considering a range of probable seismic intensities, and the results were displayed with a GIS platform. The results of this study will help relevant departments formulate emergency plans in a targeted manner and provide excellent practical value for reducing the risk of seismic hazards.
2. Study area and data
2.1. Study area
Lixia District, which is located in eastern Jinan city, has experienced considerable changes to its urban development over the last 10 years and is the scientific, cultural, commercial, political, and tourist center of Jinan. According to the data from the seventh census, the permanent population of Lixia District is 819,700, the population density is 8,020 and the total GDP reached 212.42 billion RMB by the end of 2021. As presented in the north and south of Lixia District are undulating. Lixia District is on the north side of the Taishan uplift in the Shandong massif, adjacent to the Jiyang Depression in the north, and contains the Qianfoshan fault layer. From the perspective of historical disasters, there were 42 earthquakes with seismic intensities above IV recorded within 100 km of Lixia District, with a high frequency of earthquake occurrence. However, to the best of our knowledge, no detailed quantitative assessments have been conducted within this city to date.
2.2. Data collection and preprocessing
Obtaining detailed building data is the most important requirement of seismic vulnerability assessment in urban areas (French and Muthukumar Citation2006). The data used in this study are composed of basic census data from Jinan city, visually interpreted data from multisource remote sensing images, and field survey data from Lixia District.
2.2.1. Building data of the Lixia Districts
Both rural revitalization and urban expansion are largely influenced by government regulation. Recent years have witnessed an increasing number of rural areas being transformed into new communities, and residential communities generally refer to relatively independent residential areas (Liu, Liu, et al. Citation2019). Jinan city database can be obtained in Jinan urban contour data, districts and counties planning data, as well as road planning data. Based on data from the Jinan database, the outlines of residential buildings in the study area were extracted through visual interpretation combined with high-resolution remote sensing images, street maps, and internet data. Combined with the road traffic data, the buildings are divided into different residential blocks. Since this study focuses on the urban-level seismic risk assessment in the moderate-to-low seismic hazard region, it is assumed that the buildings in the same block have the same building age, structure type, and other attribute information (Liu, Li, et al. Citation2019). The residential plots were recognized as being uniform, meaning that both the population densities in different residential plots and the building structure in a certain residential plot were consistent. Finally, Lixia District was divided into 359 residential blocks, and the vulnerability of buildings in Lixia District was evaluated by treating each building block as a unit. The main structure types considered in the study area are shown in , and they include adobe, wooden slabs, reinforced/confined masonry walls, concrete moment frames, and concrete shear walls. The characteristics of various structures are as follows.
(1) Adobe (earthen bricks)
Adobe houses in rural China are mostly adobe masonry buildings made of natural soil, sand, and hydraulic adhesives. Such adobe structures have the advantages of high insulation, humidity regulation, environmental friendliness, and sustainability, and are generally considered to have poor resistance to change and cracking, and a high vulnerability coefficient (Preciado et al. Citation2020). Wu et al. (Wu et al. Citation2017) studied the in-plane seismic performance of traditional adobe walls. The results show that under cyclic load, traditional adobe structures produce residual deformation to improve drift limit and displacement ductility, and have similar failure modes as unreinforced masonry structures. Limiting the vulnerability of adobe structures is the weak vertical shear strength of the structure and the low strength of masonry mortar used for walls. The building construction materials and structure are randomly established and applied, without complete seismic fortification measures. The adobe buildings in Lixia District are mainly located along Quancheng Road and Baotuquan North Road in the Quancheng Street area, Yaotou Road and Jiefang East Road in the Yaojia Street area, and Zhongjingzhuang Village Road in the Longdong Street area.
(2) Wooden slabs (hardwood)
A wooden slab structure includes a vertical load-bearing structure with a brick or block masonry wall and a transverse load-bearing structure with a reinforced concrete beam, floor panels, and roof. No ring beams, structural columns, and other seismic mitigation measures are used in these structures. Brick and concrete structures in Lixia District are mainly distributed along Dongguan Street, Yaojia Street, and Zhiyuan Street. The passages between two adjacent houses are very narrow and can only accommodate bicycles. The houses are densely distributed, and the population flows are large, resulting in high seismic safety risks.
(3) Reinforced/confined masonry walls
Composite steel structures are brick and concrete structures with improved ring beams, structural columns, and other seismic measures, including in the walls and between the walls. The overall structure seismic performance of these structures is good (Xue et al. Citation2019). In Lixia District, some buildings in high-level residential areas are built from seismic-proof brick and concrete, and most of these buildings are relatively broadly distributed.
(4) Concrete moment frames
A concrete moment frame structure has horizontal and vertical load capacities and can provide good overall seismic performance. These structures are among the most commonly used in urban buildings. Columns and beams form the building’s load-bearing system. Notably, columns, link beams, and the main beam form a plane frame system (Yi et al. Citation2008). Lixia District has a large number of houses with frame structures, and all streets have frame structure buildings, which are mainly distributed in the early developed areas, such as the western and central parts of Lixia District.
(5) Concrete shear walls
Shear walls are a primary type of construction that limits lateral movement; these structures are characterized by high stiffness and very strong seismic stability. A concrete shear wall structure refers to the use of reinforced concrete wall panels to bear the horizontal and vertical forces of the structure. New buildings have widely adopted this structure, especially high-rise buildings (Hidalgo et al. Citation2002). This construction, which is mostly associated with high-rise and super-high-rise structures in the recently developed areas in eastern Lixia District, significantly increases the efficiency of land use and relieves the housing pressure associated with the growing population.
2.2.2. Field data
The factors that may affect the seismic performance of buildings, such as the building structure, characteristics, quality, age, and preservation state, are considered. The building data required in this study include the type of structure, roof type, number of floors, period of construction, and preservation state (Riedel et al. Citation2014). According to the urban planning data from Lixia District and Jinan city, the building period was divided into four subperiods: 2002-present, 1982-2002, 1960-1981, and before 1960. According to the RISK-UE seismic assessment method, the number of floors was divided into three categories: low-rise buildings with two floors or less, moderate-rise buildings with three to five floors (including five floors), and high-rise buildings with more than six floors (Lestuzzi et al. Citation2016). The roof types were divided into two categories: flat roofs and sloped roofs. The effects of site factors and topography on seismic vulnerability were not considered in this study.
The building structure type, building age, roof type, number of floors, and preservation state were obtained from multisource remote sensing data and field surveys. The corresponding statistical results were as follows: there were 219 buildings in RC1 (61%), 115 buildings in RC2 (32%), 12 buildings in M4 (3.3%), 7 buildings in M3.3 (2%), and only 6 buildings in M3.1 (1.7%). There were no buildings constructed before 1960, 14 buildings constructed from 1960-1981 (3.9%), 142 buildings constructed from 1982-2002 (39.6%), and 203 buildings constructed since 2002 (56.5%). Only 25 (7.0%) of the buildings were low rise (less than 2 stories), 218 (60.7%) were moderate rise (3-6 stories), and 116 (32.3%) were high rise (7 stories or more). Through the interpretation of remote sensing images and Baidu Street Map view images, 258 buildings were identified as having flat roofs, and 101 were identified as having sloped roofs. The results are shown in .
Figure 3. Spatial distributions and proportions of different attributes in the in situ building database.

The detailed definitions of various terms considering the building type, the extent of the damage, and the number of buildings distinguish EMS-98 from other strength codes. The EMS-98 standard classifies buildings with different structural types into six grades, ranging from A (the highest) to F (the lowest). The seismic damage grades of buildings as were divided into DG1 (slight damage), DG2 (moderate damage), DG3 (substantial to heavy damage), DG4 (severe damage), and DG5 (destruction).
After statistics were obtained for building information at the block scale, a small area (approximately 800*1200 ) in Lixia District was selected for detailed field investigation. The selected field survey area shown in contained a variety of building structure types and numerous apartment complexes, which are representative and can reflect the architectural characteristics of Lixia District. In addition to recording the basic building attribute information for each block, the vulnerability level of each block was assessed according to the EMS-98 standard (Grünthal Citation1998).
3. Method
3.1. Support vector machine (SVM)
ML is a growing field of computational analysis that aims to mimic human intelligence by learning from the available information (El Naqa and Murphy Citation2015). Notably, ML refers to the analysis of (often large) observational data sets to discover unanticipated relationships and summarize the data in novel ways that are both understandable and useful to data users (Ruggieri et al. Citation2021; Sun et al. Citation2021; Ruggieri et al. Citation2022). ML algorithms can yield high-accuracy results in a short period. SVMs are relatively new ML models used to solve two-class classification problems (Cortes and Vapnik Citation1995); they are trained using current data, and several support vectors are selected from the training data to represent all the data. Notably, linearly inseparable problems can be solved with SVMs, and thus, they are widely used in decision-making and prediction in various fields (Chen Citation2020). The SVM principle involves building a hyperplane as a decision surface, maximizing the isolation edge between two classes, and obtaining the maximum geometric distance by controlling the function-based distance. shows the three phases of this study: data processing, ML, and application. Training and testing are the two parts of the SVM approach used in this study to develop a vulnerability proxy. The data are split into training and test sets in the first phase, and a proxy is created that corresponds to the fundamental structural characteristics of buildings for vulnerability categorization. The created proxy is applied to a target region with certain building property attributes in the second phase. This approach may significantly lower the cost of urban-scale building vulnerability analysis.
A set of training samples is considered: where
If a vector w and a scalar b exist such that each element satisfies the following inequality,
(1)
(1)
(2)
(2)
the functional distance of a point from the hyperplane is:
(3)
(3)
The optimal hyperplane can be defined as a classification surface for which the two categories are correctly classified and the classification interval is maximized. The optimal parameters are set as and
Then, the optimal hyperplane is:
(4)
(4)
The classification interval is:
(5)
(5)
The maximum geometric distance between the projections of two training vectors of different classes is:
(6)
(6)
For convenience, the maximum geometric distance can also be written as:
(7)
(7)
SVMs use four types of kernel functions. The radial basis function (RBF) is selected in this study, and its mathematical representation is as follows (Pourghasemi et al. Citation2013):
(8)
(8)
where
is the kernel function and
is the kernel function parameter entered manually during the calculation. The class labels of the training set are the vulnerability classification results, and the other four attributes are the type of structure, roof type, number of floors, and period of construction. Under a supervised classification framework, the SVM algorithm is applied to find the best hyperplane for building vulnerability classification using the training set, and the accuracy is evaluated by calculating the ratio of correct classification to overall classification according to a confusion matrix.
3.2. Vulnerability index method
The European RISK-UE project was launched at the end of the International Decade for Natural Disaster Reduction (IDNDR) in 1999; it is an advanced methodology for large-scale seismic risk scenarios. The project was initially established in seven Eastern and Western European cities, and a method for modelling earthquake scenarios was created (Mouroux and Le Brun Citation2008). This method is highly compatible with the EMS-98 standard; thus, the RISK-UE method has been widely used around the world. The damage status of a building is usually described by four or five grades. The LM1 method (based on EMS-98) classifies the damage status as none, slight, moderate, substantial to heavy, very heavy, and destruction; the LM2 method (based on FEMA/NIBS) classifies it as none, slight, moderate, severe, and collapse. The main difference between LM1 and LM2 is the upper-limit damage grade. The LM1 method in the RISK-UE risk assessment is employed in the study, and the calculation can be divided into the following three processes.
1. Estimate the vulnerability index ()
The vulnerability index () is used to indicate the category of vulnerability to which a building belongs and quantify this vulnerability. The range is (0-1), where a value of 0 indicates the lowest vulnerability and a value of 1 indicates the highest vulnerability. The vulnerability index is classified according to the type of building structure based on the median (
), the minimum limit of the reasonable range (
), the maximum limit of the reasonable range (
), the upper limit of possible values (
), and the lower limit of possible values (
) corresponding to the most likely value of the vulnerability index. The building structure types in the study area and the corresponding index values in the RISK-UE method are shown in .
Table 1. Vulnerability index values for various buildings in Lixia District based on RISK-UE.
The factors affecting vulnerability, such as the geographical location, height, and irregularity of a complex building, are added, and is adjusted to obtain a more accurate value of the vulnerability index, as shown in formula (9). Here,
represents the different vulnerability indexes corresponding to different building structure types.
is a regional vulnerability factor that considers the particular quality of some building types at a regional level.
is the seismic behaviour modifier, which encompasses all other factors of seismic performance. Regional vulnerability factors and seismic correction factors are not considered in this study.
(9)
(9)
2. Estimate the mean seismic damage grade
The mean seismic damage grade is estimated according to the vulnerability index
and the corresponding seismic intensity
The corresponding formula is as follows:
(10)
(10)
where
represents the seismic intensity and
represents the ductility index, which is determined after considering the building type. For buildings, the value
is 2.3. The mean seismic damage index
is calculated by the following formula:
(11)
(11)
where k represents the seismic damage grade, ranging from 0 to 5, and
represents the corresponding probability for each seismic damage grade.
3. Estimate the damage distribution.
It is assumed that for a given seismic intensity, the probability of predicting the damage grade follows a binomial distribution, and the probability calculation for each grade is as follows:
(12)
(12)
4. Results
4.1. SVM applications
After classifying the buildings in the selected small area according to the EMS-98 standard for vulnerability, the database was divided into an independent training set and a test set. To ensure the robustness of the results, the data were randomly selected from the database before each epoch to generate the training set, and the experiment was repeated 1000 times independently to generate 1000 independent training and test sets.
Determining the optimal amount of data in the training set is the key to the experiment. The data for 10%, 20%, 30%, 40%, 50%, 60%, and 70% of the total number of buildings were randomly taken from the database of the vulnerability levels of buildings and the four building attributes as the training set for the experiment. The statistical accuracy results are shown in . As the percentage of the training set used increases, the accuracy gradually improves, reaching a peak at 60%; then, it starts to decline, and dispersion first gradually decreases and then increases after reaching a minimum at 40%. The accuracy at 40% is not different from that at 60%, and the dispersion is comparatively limited. Thus, 40% is selected as the best training set proportion in the experiment.
Different combinations of building attributes affect the accuracy of classification. The sample size based on a training set ratio of 40% was determined, and a process with 1000 iterations involving the different combinations of building attributes was implemented. Then, the average accuracy of each group was recorded, as shown in .
shows the combination with the period of construction and the number of floors, with an average accuracy of 75.69%; shows the combination with the roof type and the number of floors, with an average accuracy of 67.72%. the average accuracy is 75.07% after considering three attributes: roof type, period of construction, and the number of floors. after changing the roof type to the structure type, the classification accuracy increased to 75.48%. It is confirmed that the type of structure has a considerable influence on the determination of building vulnerability.
The confusion matrix was calculated using the vulnerability from the field survey data set, the five classifications obtained using the SVM method, and the combination of the four attributes. The calculation results are shown in . The classification accuracy of Classes A and E is relatively high. A total of 66.7% of Class B buildings were incorrectly classified as Class A, and 91% of Class C buildings were incorrectly classified as Class D. The diagonal values in the table are the percentages of buildings that were correctly classified, and the overall classification accuracy is 76.90%. According to the EMS-98 standard, A and B display similar vulnerabilities and are difficult to distinguish. Moreover, due to the limitation of the SVM classifier in the discrimination of neighbouring classes, five classes are combined into three classes for the test, namely, A and B are one class, C and D are the second class, and E is the third class (Riedel et al. Citation2015). when four attributes (number of floors, period of construction, type of structure, and roof type) and five EMS-98 categories are considered, the average accuracy increases to 75.52%. As shown in , the average accuracy is directly increased to 86.33% when four attributes and three categories are considered.
Table 2. Example of a confusion matrix obtained using the SVM method with the in situ database.
The vulnerability proxy obtained by SVM training in a small area is then applied to the whole building database for Lixia District, and the obtained spatial distribution of vulnerability and the corresponding proportions are shown in .
4.2. Seismic risk assessment in Lixia District
4.2.1. Vulnerability analysis
According to formula (9), the vulnerability index in Lixia District varies from 0.35 to 0.75, with an average value of 0.43. The spatial distribution of the mean vulnerability index is shown in . In general, the overall buildings in Lixia District display low fragility and good seismic performance. Yaojia Street in the middle of Lixia District, Longdong Street in the south, Shunhua Street in the east, and Zhiyuan Street display the lowest building vulnerability, with a value of less than 0.45. In contrast, the areas of Lixia District and villages in the city region that were built earlier, the buildings with brick and wood structures and the buildings with brick and concrete structures are more vulnerable, with vulnerability index values of more than 0.7. The buildings in these areas are generally single-story self-built houses with partially damaged structures and a lack of anti-seismic fortification measures.
4.2.2. Seismic risk analysis of buildings
After obtaining the seismic vulnerability index, formula (10) was used to estimate the mean seismic damage index for buildings based on different seismic intensities, and the mean seismic damage degree was then obtained. In this study, the seismic damage degree of buildings was evaluated under intensities VII, VIII, IX, and X. According to the RISK-UE method, the seismic damage degree of buildings was divided into six grades: ‘none’, ‘slight’, ‘moderate’, ‘substantial to heavy’, ‘very heavy’, and ‘destruction’. The mean seismic damage index and the classification standard for different seismic damage degrees are shown in . Many factors affect the seismic risk, such as the vibration intensity, side effects, and geological conditions. In this study, the vibration intensity factor was mainly considered. According to the calculation results, there are no damaged buildings in Lixia District at seismic intensity X and below.
Table 3. Mean damage index values and the corresponding damage grades.
The spatial distribution of seismic damage grade is shown in . For intensity VII, the seismic damage coefficient ranges from 0.260 to 1.086, and the weighted average damage index is approximately 0.036, corresponding to the ‘none’ grade. shows that the seismic damage degree of buildings in Lixia District is categorized as ‘none’ or ‘slight’ damage. Buildings are intact in most areas, with a basically uniform distribution throughout the whole district. The lightly damaged houses are mainly distributed in the mountainous areas in the northwestern, eastern, and southern parts of the district and in some parts of the district that were developed earlier than others. For intensity VIII, the seismic damage index ranges from 0.441 to 1.991, and the weighted mean seismic damage index is approximately 0.716, which corresponds to ‘slight’ damage. shows that the buildings in Lixia District generally experience slight damage, and the buildings without damage are high-rise and super-high-rise buildings with the shear-frame wall structures. Additionally, the buildings with moderate damage are mainly brick and wood structures in the old city or countryside areas scattered along the edges of the Lixia District. For seismic intensity IX, the damage index ranges from 0.937 to 3.061, and the weighted mean damage index is approximately 1.072, which corresponds to ‘slight’ damage. As shown in , all the buildings in Jinan city are slightly damaged, none of them are intact, and moderately to severely damaged buildings are scattered along the edges and central areas of the district. For intensity X, the damage coefficient ranges from 1.774-3.951, and the weighted average damage index is approximately 2.072, which corresponds to a ‘moderate’ grade. In , the seismic influence on buildings in Lixia District is relatively serious, and most of the buildings are moderately damaged. Notably, the severely damaged buildings are brick and wood structures with the relatively scattered distribution. The results of different damage grades under different seismic intensities were shown in .
Figure 11. The spatial distribution of seismic damage grade under different seismic intensities. (a) Intensity VII, (b) intensity VIII, (c) intensity IX, and (d) intensity X.

Table 4. The proportions of damage grades under different seismic intensities.
5. Discussion
This study focuses on implementing seismic vulnerability and risk assessment of large-scale urban areas in low-risk locations with high efficiency and cheap cost and selects Lixia District as the study area. Since most residential buildings in the study area are distributed in groups and property information for buildings in the same community is consistent, the vulnerability of single buildings is extended to the vulnerability of block buildings. This not only increases efficiency but also ensures accuracy. To extract building attribute information, this study employs multisource remote sensing interpretation. Within the study area, only a small area (about 800*1200 m2) with architectural representation be selected for detailed field investigation, and the vulnerability grade is assessed using the EMS-98 standard. In ArcGIS, we outline the building block with the same attribute information and establish the corresponding database. The ML method is used to train data with vulnerability to build a proxy with consistent accuracy and reliability that can be applied throughout the entire study region. The results show that SVM can obtain a stable and accurate evaluation in vulnerability assessment. In this way, accurate vulnerability assessment can be made even in the case of insufficient data.
However, building information estimation based on multisource remote sensing interpretation also relies on manual visual interpretation, which has considerable accuracy but limited efficiency. As a result, future efforts will be directed towards the achievement of intelligent extraction of building information. Furthermore, while ML is a relatively established technology, the quality of the resulting model is strongly dependent on the training data, which has certain limitations. To fix this, the next move would be trying to merge artificial intelligence neural networks with the seismic vulnerability assessment.
6. Conclusion
Regional seismic risk assessments of buildings are important for urban planning, as well as for formulating emergency management plans and minimizing seismic risks. Additionally, the results of these assessments can be used to raise public awareness regarding possible disaster prevention measures, enhance people’s capacity for self-rescue and decrease the number of potential casualties. In this study, a comprehensive method combining multisource remote sensing interpretation, ML, and GIS technology was introduced to assess the macroscale seismic vulnerability of buildings in Lixia District, Jinan City, China. We first obtained building attribute information based on multisource data, such as land and traffic planning data, street view images, and multitemporal remote sensing images. Then, a small area was selected for field research, the EMS-98 standard was used to determine the vulnerability of buildings in this area, and the SVM model was used to establish the corresponding relationship between building attributes and vulnerability. After the SVM was adopted, the accuracy and stability of the results were evaluated. Finally, the damage degrees of buildings in Lixia District under seismic intensities VII, VIII, IX, and X were calculated with the RISK-UE vulnerability index method and presented in the ArcGIS environment. The main conclusions of this study are as follows.
The mean vulnerability index of buildings in Lixia District is 0.43, and the seismic performance of buildings is generally good;
When the seismic intensity reaches VII and VIII, the buildings in Lixia District are generally slightly or not damaged, and the requirements for the seismic fortification of buildings are met. When the seismic intensity reaches IX and X, the damage degrees of buildings with brick-concrete and single-story brick-wood structures are the greatest. The mountainous areas in southern Lixia District and urban villages experience severe damage;
At the same seismic intensity, the damage degrees of low-rise brick and wood structures and brick and concrete structures located along northern Zhiyuan Street, the middle of Yaojia Street, the western part of Longdong Street, the southern part of Dongguan Street, the eastern part of Quancheng Road Street and the western part of Daming Lake Street are the highest. Therefore, it is necessary to strengthen the seismic fortification of buildings in these areas and improve public awareness regarding disaster risk.
In conclusion, this study demonstrated that a seismic vulnerability and risk assessment approach based on multisource remote sensing, ML, and GIScience technology can provide an accurate evaluation of seismic damage. This study provides a scientific foundation for seismic risk investigation and risk assessments, as well as a foundation for the government to formulate policies and implement disaster prevention and mitigation measures. The proposed approach can be applied in regional development and planning, land and space planning, seismic disaster prevention, and other fields. It is important to reduce the direct economic losses caused by disasters and achieve the sustainable development goals of the United Nations.
Acknowledgements
We sincerely thank the anonymous reviewers and associated editors for their valuable and constructive comments and suggestions that helped to improve this paper.
Disclosure statement
The authors report there are no competing interests to declare.
Data availability statement
The data that support the findings of this study are available from the author Yaohui Liu upon reasonable request.
Additional information
Funding
References
- Alam N, Alam MS, Tesfamariam S. 2012. Buildings’ seismic vulnerability assessment methods: a comparative study. Nat Hazards. 62(2):405–424.
- Borfecchia F, De Cecco L, Pollino M, La Porta L, Lugari A, Martini S, Ristoratore E, Pascale C. 2010. Active and passive remote sensing for supporting the evaluation of the urban seismic vulnerability. ItJRS. 42:129–141.
- Borzi B, Dell’Acqua F, Faravelli M, Gamba P, Lisini G, Onida M, Polli D. 2011. Vulnerability study on a large industrial area using satellite remotely sensed images. Bull Earthquake Eng. 9(2):675–690.
- Change IPOCJGI. 2007. Climate change 2007: the physical science basis: summary for policymakers.104–116.
- Chen C-S. 2020. Seismic performance assessments of school buildings in Taiwan using artificial intelligence theories. EC. 37(9):3321–3343.
- Cockburn G, Tesfamariam S. 2012. Earthquake disaster risk index for Canadian cities using Bayesian belief networks. Georisk Assess Manag Risk Eng Syst Geohazards. 6(2):128–140.
- Code PJBECfS. 2005. Eurocode 8: design of structures for earthquake resistance-part 1: general rules, seismic actions and rules for buildings.Brussels: European Committee for Standardization.
- Cortes C, Vapnik V. 1995. Support-vector networks. Mach Learn. 20(3):273–297.
- Costanzo A, Montuori A, Silva JP, Silvestri M, Musacchio M, Doumaz F, Stramondo S, Buongiorno M. 2016. The combined use of airborne remote sensing techniques within a GIS environment for the seismic vulnerability assessment of urban areas: an operational application. Remote Sens. 8(2):146.
- de Felice G, Giannini R. 2010. An efficient approach for seismic fragility assessment with application to old reinforced concrete bridges. J Earthquake Eng. 14(2):231–251.
- El Naqa I, Murphy MJ. 2015. What is machine learning?. In Machine learning in radiation oncology. Cham: Springer.
- FEMA 310. 1998. Handbook for the seismic evaluation of buildings—A pre-standard. Washington, DC: Federal Emergency Management Agency.
- French SP, Muthukumar S. 2006. Advanced technologies for earthquake risk. J Earthquake Eng. 10(2):207–236. inventories.10:207-236.
- Frigerio I, Ventura S, Strigaro D, Mattavelli M, De Amicis M, Mugnano S, Boffi M. 2016. A GIS-based approach to identify the spatial variability of social vulnerability to seismic hazard in Italy. Appl Geogr. 74:12–22.
- Geiß C, Aravena Pelizari P, Marconcini M, Sengara W, Edwards M, Lakes T, Taubenböck H. 2015. Estimation of seismic building structural types using multi-sensor remote sensing and machine learning techniques. ISPRS J Photogramm Remote Sens. 104:175–188.
- Geiß C, Priesmeier P, Aravena Pelizari P, Soto Calderon AR, Schoepfer E, Riedlinger T, Villar Vega M, Santa María H, Gómez Zapata JC, Pittore M, et al. 2022. Benefits of global earth observation missions for disaggregation of exposure data and earthquake loss modeling: evidence from Santiago de Chile. Nat Hazards.1–26.
- Geiß C, Taubenböck HJNH. 2013. Remote sensing contributing to assess earthquake risk: from a literature review towards a roadmap. Nat Hazards. 68(1):7–48.
- Geiß C, Taubenböck H, Tyagunov S, Tisch A, Post J, Lakes TJES. 2014. Assessment of seismic building vulnerability from space. Earthquake Spectra. 30(4):1553–1583.
- Ghosh S, Roy A, Chakraborty S. 2018. Support vector regression based metamodeling for seismic reliability analysis of structures. Appl Math Modell. 64:584–602.
- Grünthal G. 1998. European macroseismic scale 1998.
- Guéguen P, Michel C, LeCorre L. 2007. A simplified approach for vulnerability assessment in moderate-to-low seismic hazard regions: application to Grenoble (France). Bull Earthquake Eng. 5(3):467–490.
- Guettiche A, Guéguen P, Mimoune M. 2017. Seismic vulnerability assessment using association rule learning: application to the city of Constantine, Algeria. Nat Hazards. 86(3):1223–1245.
- Hamdy O, Gaber H, Abdalzaher MS, Elhadidy M. 2022. Identifying exposure of urban area to certain seismic hazard using machine learning and GIS: a case study of greater Cairo. Sustainability. 14(17):10722.
- Hidalgo PA, Ledezma CA, Jordan R. 2002. Seismic behavior of squat reinforced concrete shear walls. Earthquake Spectra. 18(2):287–308.
- Hill M, Rossetto T. 2008. Comparison of building damage scales and damage descriptions for use in earthquake loss modelling in Europe. Bull Earthquake Eng. 6(2):335–365.
- Huang X, Xia J, Xiao R, He T. 2019. Urban expansion patterns of 291 Chinese cities, 1990–2015. Int J Digital Earth. 12(1):62–77.
- Huang J, Zhao D. 2006. High-resolution mantle tomography of China and surrounding regions. Solid Earth. 111(B9).
- Jia K, Zhou S, Zhuang J, Jiang C. 2014. Possibility of the independence between the 2013 Lushan earthquake and the 2008 Wenchuan earthquake on Longmen Shan fault, Sichuan, China. Seismol Res Lett. 85(1):60–67.
- Kaushik HB, Dasgupta K. 2013. Assessment of seismic vulnerability of structures in Sikkim, India, based on damage observation during two recent earthquakes. J Perform Constr Facil. 27(6):697–720.
- Leggieri V, Ruggieri S, Zagari G, Uva G. 2021. Appraising seismic vulnerability of masonry aggregates through an automated mechanical-typological approach. Autom Constr. 132:103972.
- Lestuzzi P, Podestà S, Luchini C, Garofano A, Kazantzidou-Firtinidou D, Bozzano C, Bischof P, Haffter A, Rouiller J-D. 2016. Seismic vulnerability assessment at urban scale for two typical Swiss cities using Risk-UE methodology. Nat Hazards. 84(1):249–269.
- Li X, Li Z, Yang J, Li H, Liu Y, Fu B, Yang F. 2020. Seismic vulnerability comparison between rural Weinan and other rural areas in Western China. Int J Disaster Risk Reduct. 48:101576.
- Li X, Li Z, Yang J, Liu Y, Fu B, Qi W, Fan XJNH. 2018. Spatiotemporal characteristics of earthquake disaster losses in China from 1993 to 2016. Nat Hazards. 94(2):843–865.
- Lin X, Zhang H, Chen H, Chen H, Lin JJEE. 2015. Field investigation on severely damaged aseismic buildings in 2014 Ludian earthquake. Earthq Eng Eng Vib. 14(1):169–176.
- Liu P, Liu X, Liu M, Shi Q, Yang J, Xu X, Zhang YJRS. 2019. Building footprint extraction from high-resolution images via spatial residual inception convolutional neural network. Remote Sens. 11(7):830.
- Liu Y, Li Z, Wei B, Li X, Fu B. 2019. Seismic vulnerability assessment at urban scale using data mining and GIScience technology: application to Urumqi (China). Geomatics Nat Hazards Risk. 10(1):958–985.
- Liu Y, So E, Li Z, Su G, Gross L, Li X, Qi W, Yang F, Fu B, Yalikun A, et al. 2020. Scenario-based seismic vulnerability and hazard analyses to help direct disaster risk reduction in rural Weinan, China. Int J Disaster Risk Reduct. 48:101577.
- Liu Y, Zhang W, Chen X, Yu M, Sun Y, Meng F, Fan X. 2021. Landslide Detection of High-Resolution Satellite Images using Asymmetric Dual-Channel Network. 2021 IEEE International Geoscience and Remote Sensing Symposium IGARSS.
- Maya M, Yu W. 2019. Short-term prediction of the earthquake through neural networks and meta-learning. Proceedings of the. 2019 16th International Conference on Electrical Engineering, Computing Science and Automatic Control (CCE); IEEE.
- Mouroux P, Le Brun B. 2008. Risk-UE project: an advanced approach to earthquake risk scenarios with application to different European towns. In: Assessing and Managing Earthquake Risk. Springer; p. 479–508.
- Mueller M, Segl K, Heiden U, Kaufmann H. 2006. Potential of high-resolution satellite data in the context of vulnerability of buildings. Nat Hazards. 38(1-2):247–258.
- Nie G-z, Gao J-g, Ma Z-j 2002. On the risk of earthquake disaster in China in the coming 10 ∼ 15 years.11:68–73.
- Pourghasemi HR, Jirandeh AG, Pradhan B, Xu C, Gokceoglu C. 2013. Landslide susceptibility mapping using support vector machine and GIS at the Golestan Province, Iran. J Earth Syst Sci. 122(2):349–369.
- Preciado A, Ramirez-Gaytan A, Santos JC, Rodriguez O. 2020. Seismic vulnerability assessment and reduction at a territorial scale on masonry and adobe housing by rapid vulnerability indicators: the case of Tlajomulco, Mexico. Int J Disaster Risk Reduct. 44:101425.
- Rahman N, Ansary MA, Islam I. 2015. GIS based mapping of vulnerability to earthquake and fire hazard in Dhaka city, Bangladesh. Int J Disaster Risk Reduct. 13:291–300.
- Ramírez Eudave R, Ferreira T. 2021. On the potential of using the Mexican National Catalogue of Historical Monuments for assessing the seismic vulnerability of existing buildings: a proof-of-concept study. Bull Earthquake Eng. 19(12):4945–4978.
- Riedel I, Guéguen P, Dalla Mura M, Pathier E, Leduc T, Chanussot J. 2015. Seismic vulnerability assessment of urban environments in moderate-to-low seismic hazard regions using association rule learning and support vector machine methods. Nat Hazards. 76(2):1111–1141.
- Riedel I, Gueguen P, Dunand F, Cottaz S. 2014. Macroscale vulnerability assessment of cities using association rule learning. Seismol Res Lett. 85(2):295–305.
- Ródenas JL, García-Ayllón S, Tomás A. 2018. Estimation of the buildings seismic vulnerability: a methodological proposal for planning ante-earthquake scenarios in urban areas. Appl Sci. 8(7):1208.
- Ruggieri S, Calò M, Cardellicchio A, Uva G. 2022. Analytical-mechanical based framework for seismic overall fragility analysis of existing RC buildings in town compartments. Bull Earthquake Eng. 20(15):8179–8216.
- Ruggieri S, Cardellicchio A, Leggieri V, Uva G. 2021. Machine-learning based vulnerability analysis of existing buildings. Autom Constr. 132:103936.
- Sichani ME, Padgett JE, Bisadi V. 2018. Probabilistic seismic analysis of concrete dry cask structures. Struct Saf. 73:87–98.
- Silva V, Akkar S, Baker J, Bazzurro P, Castro JM, Crowley H, Dolsek M, Galasso C, Lagomarsino S, Monteiro R, et al. 2019. Current challenges and future trends in analytical fragility and vulnerability modeling. Earthquake Spectra. 35(4):1927–1952.
- Sun H, Burton HV, Huang H. 2021. Machine learning applications for building structural design and performance assessment: state-of-the-art review. J Build Eng. 33:101816.
- Tesfamariam S, Liu Z. 2010. Earthquake induced damage classification for reinforced concrete buildings. Struct Saf. 32(2):154–164.
- Tesfamariam S, Saatcioglu MJES. 2010. Seismic vulnerability assessment of reinforced concrete buildings using hierarchical fuzzy rule base modeling. Earthquake Spectra. 26(1):235–256.
- Thomalla F, Downing T, Spanger-Siegfried E, Han G, Rockström JJD. 2006. Reducing hazard vulnerability: towards a common approach between disaster risk reduction and climate adaptation. Disasters. 30(1):39–48.
- Wang Q, Zhang PZ, Freymueller JT, Bilham R, Larson KM, Lai X, You X, Niu Z, Wu J, Li Y, et al. 2001. Present-day crustal deformation in China constrained by global positioning system measurements. Science. 294(5542):574–577.
- Wu F, Wang H-T, Li G, Jia J-Q, Li H-N. 2017. Seismic performance of traditional adobe masonry walls subjected to in-plane cyclic loading. Mater Struct. 50(1):14.
- Xie Y, Ebad Sichani M, Padgett JE, DesRoches R. 2020. The promise of implementing machine learning in earthquake engineering: a state-of-the-art review. Earthquake Spectra. 36(4):1769–1801.
- Xu X, Wen X, Han Z, Chen G, Li C, Zheng W, Zhnag S, Ren Z, Xu C, Tan X, et al. 2013. Lushan M S7. 0 earthquake: a blind reserve-fault event. Chin Sci Bull. 58(28-29):3437–3443.
- Xue J, Xu D, Qi L. 2019. Experimental seismic response of a column-and-tie wooden structure. Adv Struct Eng. 22(8):1909–1922.
- Yi W-J, He Q-F, Xiao Y, Kunnath S. 2008. Experimental study on progressive collapse-resistant behavior of reinforced concrete frame structures. ACI Struct J. 105(4):433.
- Zhai W, Huang CJE. 2016. Fast building damage mapping using a single post-earthquake PolSAR image: a case study of the 2010 Yushu earthquake. Earth Planet Sp. 68:1–12.