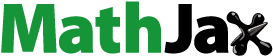
Abstract
The urbanization process has significantly intensified surface urban heat island (SUHI) effects in the Bangkok Metropolitan Region (BMR). However, understanding the evolution of the urban thermal environment is challenging due to the difficulty in obtaining consistent remote sensing data of the cloud-prone landscape in the BMR. In this study, the data fusion algorithm was utilized to fill cloud-induced data gap and create high spatiotemporal-resolution data by blending Landsat and MODIS remote sensing images. The fused data was used to retrieve land surface temperature (LST) for winter months from 2000 to 2020. The spatiotemporal variations in SUHI were then captured using spatial cluster analysis. Finally, gradient analysis and geographically weighted regression (GWR) were employed to analyse the effects of land cover composition on LST. The SUHI intensity in winter increased from 4.40 °C in 2000 to 5.76 °C in 2020. The areal percentage of SUHI hot spots increased from 24.86% to 29.13%. The gradient analysis results indicated that vegetation with a density higher than 0.3 had a significant effect on LST compared to low-density areas. The woody lands were more effective in lowering LST than cultivated lands. These results provide useful information for developing heat mitigation strategies in the metropolitan regions.
1. Introduction
As a complex socio-economic process, urbanization shifts the population distribution from dispersed rural areas to dense urban areas. The urbanization process converts natural land to impervious surfaces, which changes the thermal properties and energy budget of land surface. These changes further lead to changes in the thermal environment of urban areas and the urban heat island(UHI) effect (Zhao et al. Citation2014; Manoli et al. Citation2019). The UHI effect can exacerbate the intensity and frequency of extreme heat events (Marcotullio et al. Citation2021; Mentaschi et al. Citation2022), cause higher human health risks (Estoque et al. Citation2020), enhance environmental pollution (Henao et al. Citation2020), and increase energy consumption (Lu et al. Citation2019a). The investigation of UHI is of great importance for developing thermal mitigation strategies and enhancing thermal resilience of urban settlements, thereby contributing to the achievement of United Nation’s sustainable development goals in urban areas (United Nations Citation2015).
The UHI effect can be represented using the boundary layer heat island, canopy layer heat island, and surface urban heat island (SUHI) (Stewart and Oke Citation2012; Venter et al. Citation2021). The former two are also known as atmospheric heat islands, which are measured based on the temperature acquired from weather stations. SUHI is described using land surface temperature (LST) differences between urban and neighbouring areas based on remote sensing data (Zhou et al. Citation2019). With the development of thermal infrared remote sensing technologies, LST obtained from satellite sensors has been widely used in SUHI studies (Weng et al. Citation2018). In the 1990s, Landsat TM images were applied to reveal the LST differences among different land cover types (Xian et al. Citation2022). In the 2000s, the MODIS Terra sensor was used to retrieve LST at a ∼1 km resolution, but imagery with such a low spatial resolution may fail to capture urban structural details (Sobrino et al. Citation2012; Cheval et al. Citation2022). Remote sensing data with a spatial resolution lower than 50 m tend to insufficiently distinguish the thermal differences within cities (Stewart and Oke Citation2012; Ezimand et al. Citation2021). To obtain remote sensing data with both high spatial and temporal resolution, spatiotemporal data fusion models have been developed (Weng et al. Citation2014; Ghamisi et al. Citation2019). These models blend the frequent temporal information and high-resolution spatial information from different sensors. The spatial and temporal adaptive reflectance fusion model (STARFM) proposed by Feng et al. (Citation2006) has been a widely adopted approach due to its high efficiency. The spatiotemporal adaptive data fusion algorithm for temperature mapping (SADFAT) was developed by introducing a conversion coefficient (Weng et al. Citation2014). Compared with these two methods, the enhanced spatiotemporal adaptive reflectance fusion model (ESTARFM) can further improve the fusion accuracy for complex and heterogeneous surfaces (Zhu et al. Citation2010). The ESTARFM model has been used to fuse Landsat and MODIS satellite imagery and described the spatial details of high-resolution LST data clearly (Liu et al. Citation2020). Using Landsat-like images produced by blending satellite data from different sensors, fine-scale spatiotemporal variations of surface urban heat islands and their relationship with geophysical parameters were revealed in Rasht city, Iran (Ezimand et al. Citation2021).
Since land cover categories such as impervious surfaces and vegetation can affect land surface temperature, the spatial distribution of LST shows high variability in urbanized regions (Lu et al. Citation2019b; Kedia et al. Citation2021). The urban-rural gradient approach has been used to explore urban growth patterns and its linkage with LST in Jakarta, Bangkok, Manila (Estoque et al. Citation2017), and the Greater Bay Area, China (Xie et al. Citation2021). The influence of land cover features on LST and UHI intensity has been explored in numerous studies (Lu et al. Citation2020; Dilawar et al. Citation2021; Singh et al. Citation2022). LST was found to be closely related to the density of impervious surface (positive) and vegetation (negative) areas (Tariq et al. Citation2020; Mondal et al. Citation2021). Despite the importance of understanding urban climate in the tropical zones, the knowledge on the impact of urban characteristics on urban heat island lags behind that of temperate climate (Giridharan and Emmanuel Citation2018). Although the association between land cover and LST has been reported in multiple tropical cities, such a relationship varies with different city sizes and different climatic characteristics (Chakraborty and Lee Citation2019; Cao et al. Citation2022). Comparing 150 major cities in India, Mohammad and Goswami (Citation2021) reported that different variables such as vegetation, evapotranspiration and thermal inertia were responsible for the daytime SUHI intensity variations during summer/winter season in cities in different climatic zones. Climatic factors such as rainfall and solar radiation affect the shading and evapotranspiration intensity of urban vegetation, and their cooling effects on thermal environment are different in different regions (Cheung et al. Citation2021).
As the capital of Thailand, Bangkok has experienced severe SUHI effects due to extensive urban development in recent decades (Xu et al. Citation2019; Pimonsree et al. Citation2022). Air temperature records from meteorological stations revealed that the maximum SUHI intensity could reach up to 6-7 °C during the dry season in Bangkok (Arifwidodo and Tanaka Citation2015). The enhanced urban temperature, urban heat island, and global warming exacerbated the heat stress effects and brought negative impacts on the health and well-being of urban residents in Bangkok in recent years (Arifwidodo and Chandrasiri Citation2020a). The majority of UHI studies for the Bangkok Metropolitan Region (BMR) have used in situ meteorological data, since frequent cloud coverage makes it difficult to obtain consistent and continuous remote sensing data (Pakarnseree et al. Citation2018). Due to the uneven and sparsely distribution of weather stations, the meteorological data cannot reflect the spatiotemporal variation of the UHI phenomenon in this region accurately (Pakarnseree et al. Citation2018; Kim and Brown Citation2021). MODIS data has been used to explore SUHI trends in six major cities in Thailand (Keeratikasikorn and Bonafoni Citation2018a). However, temporal and spatial changes in SUHI were not clearly captured due to the low spatial resolution(1 km) of the MODIS satellite sensors. Therefore, a comprehensive investigation of SUHI using remotely sensed land surface temperature data with high spatial and temporal resolution is crucial to fill the knowledge gap for the development of heat mitigation and adaptation strategies in the BMR.
This study aimed to reveal the detailed spatiotemporal evolution of SUHI using an enhanced LST dataset in the BMR in last two decades. The second objective of this study is to examine the relationship between LST and land cover composition. The Landsat and MODIS remote sensing data were blended using the ESTARFM algorithm to create an enhanced LST dataset of the study area for the 2000–2020 period with a 5-year interval. Maps of SUHI were then obtained, and spatial cluster analysis was used to examine the spatiotemporal variation in SUHI intensity (SUHII). The relationships between LST and the densities of the built-up and vegetation areas were explored using gradient analysis and geographically weighted regression.
2. Study area and data
2.1. Study area
Bangkok is the largest metropolis on the Indo-China Peninsula and Southeast Asia’s second-largest city (). It is located in the Chao Phraya River delta in Thailand’s middle plain with an average elevation of less than 2 m (Keeratikasikorn and Bonafoni Citation2018b). The built-up areas are concentrated in Bangkok’s central and eastern areas along the Chao Phraya River. There are six industrial and commercial districts in the BMR. Among them, the most prosperous is Bangkok Grand Kyoto, which is the political centre of Thailand. Bangkok has a tropical monsoon climate which is hot and rainy all year round. The annual average temperature is 30 °C, and the annual average precipitation is 1,500 mm. The year can be divided into three distinct seasons: summer (March to April), the rainy season (May to October), and winter (November to February).
Figure 1. Geographical location of the Bangkok Metropolitan Region. The land use map of BMR(c) is obtained from Department of Public Works and Town & Country Planning, Bangkok (https://dpt.go.th/en/).

2.2. Data source and pre-processing
In the study, the analysis was set in winter season, since more data were available and cloud-free remote sensing images cannot be obtained in the rainy season. Cloud-free Landsat 5/8, MOD11 A2, and MOD09 A1 images were used for LST retrieval in winter during the 2000–2020 period with a 5-year time interval. Landsat 8 Collection 2 level-2 surface temperature products were used to evaluate accuracy of LST prediction results. Satellite imagery from 2008 was used to supplement the imagery from 2010 and from 2014 supplemented 2015 due to the high cloud coverage in 2010 and 2015. Landsat 5 TM and Landsat 8 OLI/TIRS Collection 1 level-1 images were downloaded from the USGS Earth Explorer (http://earthexplorer.usgs.gov/). The MOD11 A2 and MOD09 A1 data products were obtained from NASA's Earth Observation Data and Information Systems (https://earthdata.nasa.gov/).
The remote sensing images were radiometrically calibrated and atmospheric corrected, and then was projected to UTM-WGS84 coordinate system and resampled to 30 m spatial resolution. For Landsat 5/8 satellite imagery, the surface reflectance data in the red and near-infrared bands corresponded to bands 1 and 2 in MOD09A1 imagery. Using the radiative transfer equation (RTE) based method (Chatterjee et al. Citation2017), spectral radiance was derived from the LST and emissivity data of MOD11A2 products. It corresponds to the spectral radiance in the Landsat 5/8 thermal infrared band. Using the ESTARFM model, Landsat-like LST data were generated from November to January in each time period ().
Table 1. Remote sensing datasets used in this study.
3. Methods
shows the data processing workflow used in this study. The enhanced LSTs were first retrieved through the fusion of Landsat and MODIS data from the winter months of 2000, 2005, 2008, 2015, and 2020. The study then analyzed the temporal and spatial changes in SUHII in the BMR from 2000 to 2020. Finally, the relationship between SUHI and land cover was explored using gradient analysis and regression methods.
3.1. Land cover classification
A support vector machine (SVM) classifier was employed to map the study area into five categories, including built-up areas, cultivated lands, woodlands, water bodies, and bare lands (Deliry et al. Citation2021). A stratified random sampling strategy was adopted to select reference samples. More than 150 samples were selected for land cover classification and accuracy evaluation for each land cover type. Among the samples collected for each land cover, 60% were training samples, and 40% were used for validation. The quantitative metrics such as users’ accuracy, producers’ accuracy, overall accuracy, and the kappa coefficient were used to evaluate classification accuracy. Finally, the area of each land cover type was extracted from the classified imagery.
3.2. Land surface temperature retrieval
The ESTARFM algorithm is specifically tailored for data fusion of complex terrain regions and includes four main steps (). First, two Landsat images at and
were used to find pixels within a sliding window that are spectrally similar to the centre pixel. ESTARFM uses the threshold approach to find similar neighbourhood pixels based on EquationEquation (1)
(1)
(1) (Zhu et al. Citation2016).
(1)
(1)
where
defines the ith pixel;
is the centre pixel of the sliding window;
is the sliding window size;
and
respectively represent the ith pixel and centre pixel at
high spatial resolution images on band B;
represents the standard deviation of band B at
time; and
refers to the estimated feature category.
The conversion coefficient was obtained using a linear regression between Landsat and MODIS images at and
The weight of a spectrally similar pixel was computed using the location, reflectance, and thermal similarity between Landsat and MODIS data. Subsequently, two approximated Landsat-like images were created at the prediction time
Finally, the 30 m resolution image was estimated using a weighted combination of the two Landsat-like images.
This study used fused Landsat-like data and the radiative transfer equation (RTE) method to retrieve land surface temperatures. The RTE method is a widely used LST retrieval algorithm with high accuracy (Malakar et al. Citation2018). It is described using EquationEquation (2)(2)
(2) (Sekertekin and Bonafoni Citation2020):
(2)
(2)
where
represents the radiation received by the sensor;
is the blackbody radiance given by Plank’s law;
is LST;
and
represents land surface emissivity and atmospheric transmissivity, respectively; and
and
represent upwelling and downwelling atmospheric radiance, respectively. The Normalized differential vegetation index (NDVI) threshold method was used to calculate land surface emissivity. The land surface temperature can be calculated by EquationEquation (3)
(3)
(3) .
(3)
(3)
where
represent LST in Kelvin.
and
are the calibration constants for the sensors.
By comparing the predicted Landsat-like LST with observed Landsat data on the same date, the accuracy of the predicted LSTs was evaluated. The root mean square error (RMSE), mean absolute difference (MAD), and correlation coefficient (CC) between estimated and observed LST were calculated to quantitatively evaluate the estimation error of the fused LST data.
3.3. Surface urban heat island intensity quantification
Following the local climate zone framework (Stewart and Oke Citation2012), the difference in LST between urban and dense trees was used to calculate the surface urban heat island intensity (SUHII). This LST difference was measured using EquationEquation (4)(4)
(4) (Schwaab et al. Citation2021).
(4)
(4)
where
represents the land surface temperature at pixel i and
represents the average temperature of green space with dense trees. Based on land cover classification results, woodland pixels with NDVI > 0.5 were regarded as densely-vegetated green space. SUHII was further classified using combinations of the mean value and standard deviations of μ (mean), μ ± 0.5 std (standard deviation), and μ ± 1 std, yielding five grades ().
Table 2. Classification of SUHII.
3.4. Spatial cluster analysis
Multi-scale segmentation was used to create image objects to analyze the spatial pattern of LST in the study area. The segmentation of LST images was performed using eCognition Developer software (http://www.ecognition.com/). A scale parameter of 20 was used since it produced meaningful objects with 'natural’ geographic borders (Guo et al. Citation2015). The study area was divided into more than 7,500 objects using Landsat data. Getis-ord is a spatial cluster analysis method. Its basic principle is to calculate each element in the data set with EquationEquation (5)
(5)
(5) (Lemus-Canovas et al. Citation2020) and obtain the Z-score and p-value.
(5)
(5)
where
is the spatial correlation index;
is the spatial weights matrix; and
is the observation
A feature with a high Z-score and modest p-value implies a substantial hot spot. A substantial cold spot is indicated by a low negative Z-score and a small p-value. This method can be used to evaluate the spatial characteristics of LST at fine spatial scales.
3.5. Relationship analysis of land surface temperature and land cover
Based on the SVM classification results, the built-up land and vegetation areas (woodland and cultivated land) were selected to explore the relationship between LST and land cover on the urban-rural gradient. Following the previous urban-rural gradient analysis studies (Xu et al. Citation2019; Kaminski et al. Citation2021) and considering the spatial resolution of Landsat thermal images, this study created a total of 45 concentric buffer zones with 1 km intervals around the city center of Bangkok (13°45'7"N, 100°31'48"E) (Bonafoni and Keeratikasikorn Citation2018). The areal proportions of the built-up and vegetation areas to the total area within each buffer were calculated as the built-up and vegetation density, respectively.
The geographically weighted regression (GWR) method, which considers the spatial heterogeneity of the correlation relationship, was used to explore the spatial association between LST and land cover (Fotheringham et al. Citation2017). Firstly, the study area was divided into 6,737 grid cells with a size of 1 km × 1 km. Built-up area, cultivated land and woodland accounted for a large proportion in the study area. The density of three land cover types in each grid cell were calculated and selected as explanatory variables of LST. The EquationEquation (6)(6)
(6) was used for GWR analysis (Fotheringham et al. Citation2017).
(6)
(6)
where
is the response variable,
and
are the fitting parameters,
is the kth explanatory variable in region
is the geospatial location, and
is the random error in region
4. Results
4.1. Land cover changes
shows the land cover classification results for the BMR in 2000, 2005, 2008, 2014, and 2020. Most of the built-up area was located in the central part of the metropolitan area, while cultivated lands and woodlands were mainly distributed in the surrounding areas. Water bodies were scattered in the southern coastal area. Most of the built-up area increase was converted from vegetated areas.
shows the accuracy assessment results (producer’s accuracy (PA) and user’s accuracy (UA)) for the land cover classification maps. The overall accuracy of the land cover maps ranges from 90.45% to 93.90%. The kappa values range from 0.87 to 0.92. The overall classification accuracy was above 90% for each year. The land cover change analysis indicates that the built-up and bare land areas increased, while cultivated lands, woodlands, and water bodies decreased from 2000 to 2020. Among the different land cover types, the built-up areas exhibited the maximum areal increase, with 71.97% of the area converted from cultivated land.
Table 3. Accuracy assessment results for the land cover classification maps.
4.2. Land surface temperature retrieval results
The fused Landsat-MODIS images were used for LST retrieval in the study area from November to January in each time period. shows the predicted Landsat-like LST in the study area. The fused LST more clearly reflected the spatial distribution of rivers, roads, and urban gatherings in comparison to MODIS. The texture features of the spatiotemporal fusion data were clearly observable.
Figure 4. Observed Landsat on 19/12/2020 (a), predicted Landsat-like LST on 09/01/2021-16/01/2021(b), and MODIS LST on 09/01/2021-16/01/2021(c).

shows the comparison between two Landsat 8 LST products and the concurrent fusion results. Through visual comparison, the spatial distribution of predicted LST and Landsat 8 LST products are highly consistent. The RMSE between the two datasets was 1.73 °C and 1.64 °C, MAD was 0.86 °C and 0.79 °C, and CC was 0.82 and 0.89, respectively. It indicates that there was a strong correlation between the predicted LST and the Landsat LST product in most regions. The predicted LST on both dates are slightly higher than that of Landsat 8 products.
4.3. Spatiotemporal variation of the surface urban heat island
shows the spatial pattern of the average winter SUHII from 2000 to 2020. SUHII was divided into five grades based on the mean standard deviation. The SUHII generally increased across time as built-up areas expanded except in 2008. In 2008, Bangkok showed the lowest value of SUHII. The enhanced precipitation may result in the low overall SUHII and large uncertainty in LST retrieval results in 2008 (Limsakul and Singhruck Citation2016; Pimonsree et al. Citation2022). The very high-level areas gradually expanded and the most noticeable SUHII increases occurred in the eastern part of the study area. In 2000, very low-level SUHII areas were in Bangkok’s urban core. In 2020, the urban core exhibited moderate-level and high-level SUHII values, while large areas with high and very high-level SUHII values were observed in the eastern BMR.
The areal proportion of SUHII in different grades during the study period is shown in . Aside from 2008, the proportion of high-level and very high-level SUHII areas increased from 24.89% in 2000 to 46.78% in 2020, with an annual average growth rate of 1.10%. The proportion of low-level and very low-level SUHII areas decreased from 56.36% to 19.94%. The moderate-level SUHII areas accounted for the largest proportion of the study area with little temporal change. The proportion of very low-level SUHII is the highest in year 2008, while the areal percentage of high-level SUHII is the lowest.
Figure 7. Areal percentage of different SUHII grades in the Bangkok Metropolitan Region from 2000 to 2020.

shows the LST spatial cluster results for the study area. Hot spots were mostly concentrated in the urban core, and cold spots were scattered in the urban fringe and southern coastal areas. The hot spot areas were observed to spread from the urban centre to the surrounding areas over time. In addition, the hot spots in the city centre have decreased slightly. One of these areas is Bang Kachao park which area has increased during the study period. This indicates that the government has made some efforts to improve public parks and green spaces in the central area of the city and the SUHI intensity was reduced in these areas. The spatial variation of cold and hot spots captured the changes in the thermal environment of the study area over time effectively.
The proportion of hot and cold spot cluster areas showed an increasing trend over time (). Overall, the area of hot spot clusters was larger than cold spot clusters in the BMR. The areal percentage of LST hot spots increased from 24.86% to 29.13% during the study period. The average LST of cold spot clusters increased from 28.38 °C in 2000 to 31.56 °C in 2020. The average LST of hot spot clusters increased from 34.61 °C to 38.79 °C.
Table 4. Cold and hot spot clusters identified in the study area from 2000 to 2020.
4.4. Relationship between land cover and land surface temperature
The relationship between LST and land cover types was analysed in five time periods (). Generally, the LST in built-up areas was much higher than in other land cover types, and LST was lowest in water bodies. The LST of the four land cover types showed an increasing trend from 2000 to 2020 except for 2008. Among them, the LST in built-up areas showed the greatest change, increasing by about 6.20 °C from 2000 to 2020.
Figure 9. Box plots of land surface temperatures for four land-cover types: (a) built-up areas; (b) cultivated lands; (c) woodlands; and (d) water bodies.

Gradient analysis shows that the density of built-up areas decreased while the vegetation density increased from the city centre to the surrounding suburbs and rural areas (). High LST values with little variation were observed in the core region with high built-up density, which is within 15 km from city center. The built-up area density increased over time. Intensive built-up area sprawl in the transitional areas between urban and outskirt areas was observed from 2000 to 2020 (). The mitigation effect on LST was stronger in the area with vegetation density ranging from 0.30 to 0.80 than in low-density areas (). The relationship between built-up density, vegetation density, and LST was analysed within each buffer (). There were significant correlations between land cover densities and LST, with R2 values above 0.87. The density of built-up areas had a significant positive relationship with LST, while vegetation density had a negative relationship with LST. The slope value of the vegetation density-LST relationship was greater than in the built-up density-LST relationship. This indicates that the effect of vegetation density on LST was greater than that of built-up land. The results are in line with those obtained in previous studies (Adulkongkaew et al. Citation2020).
Figure 10. The mean LST vs. built-up density with the distance from the city center (a), the mean LST vs. vegetation density with the distance from the city center (b), relationship between built-up density and LST from 2000 to 2020 (c), and relationship between vegetation density and LST from 2000 to 2020 (d).

In 2020, the GWR results spatially varied for different land cover types (). In the core regions, the built-up density was close to 1, and the correlation between LST and urban built-up density was low. In the urban-rural fringe, the density of built-up areas greatly changed across space and featured a high correlation with LST. Cultivated land was scattered in patches in the core urban area and was contiguously distributed in the suburbs. Woodlands had a wider distribution of low regression coefficients compared to cultivated land. The land cover composition had an insignificant relationship with LST in regions with a high proportion of mixed land use.
5. Discussion
5.1. Implications for urban heat island mitigation
Our analysis showed that 71.97% of the newly increased built-up areas in the BMR were converted from agriculture land between 2000 and 2020. The land in the urban core was dense and compact; however, there were many fragmented built-up areas in the outskirts and hinterland (Xu et al. Citation2019; Adulkongkaew et al. Citation2020). The built-up area became more aggregated across time. During the study period, urban expansion grew radially from the urban core to the surrounding areas along the Chao Phraya River. The gradient analysis results indicate that urban land density showed an inverse S-shaped function trend based on the distance from the urban center.
The temporal changes of LST showed an increasing trend in the four land cover categories. The average LST of built-up land increased most significantly from 31.03 °C in 2000 to 37.23 °C in 2020. The intensified SUHI have been revealed in a number of cities in developing countries (Mathew et al. Citation2018; Sultana and Satyanarayana Citation2020). The intensities of UHI were observed to be increasing during winter season and the hotspots with high LSTs were identified in high-density constructed region or dry barren lands in Indian cities such as Kolkata, Mumbai and Hyderabad (Sultana and Satyanarayana Citation2020). Bankgkok has experienced wetter conditions and a concomitant increase in the frequency and magnitude of heavy precipitation events from 1955 to 2014 (Limsakul and Singhruck Citation2016; Pimonsree et al. Citation2022). Located at the mouth of the Chao Phraya River, serious floods (such as the 2011 flood) usually take place in October and last for one or two months when the upstream flood and local rainstorm occur at the same time (Worawiwat et al. Citation2021). The low SUHII in Bangkok in 2008 might be caused by the heavy precipitation and flooding during the study period (Arifwidodo and Tanaka Citation2015).
The spatial clustering analysis results of Bangkok show that the high LST clusters are mainly distributed in the central urban construction land intensive areas, while the low LST clusters are mainly scattered in the surrounding areas of the city. The area of hot spots increased from 24.86% in 2000 to 29.13% in 2020, showing an obvious growth trend. Previous studies found that the built-up density greatly impacted SUHI in Bangkok, and the building coverage and floor area ratio were significantly linked with SUHI (Pakarnseree et al. Citation2018). Despite the continuous expansion of the high SUHI intensity area in the mostly urbanized central area, few SUHI hotspots were identified in the newly developed areas with proper planning and green space in Kolkata, India (Halder et al. Citation2021). With the improvement of road transportation facilities such as subway and light rail in Bangkok in recent years, residents tend to settle in Nonthaburi and Pathum Thani areas, which intensifies the LST in northern Bangkok. Public green spaces such as Bang Kachao Park should be built to alleviate heat stress in the newly developed settlements in the north (Arifwidodo and Chandragiri Citation2020b).
The effects of land cover composition on LST have been studied extensively (Mondal et al. Citation2021). Keeratikasikorn and Bonafoni (Citation2018b) found that the average SUHI was lowest in rural and agricultural areas and highest in commercial areas in the BMR. This was related to the concentration of commercial land use in the city center. The association between LST and vegetation density indicates that dense and aggregated vegetation areas were very effective at reducing the temperatures in the BMR. An analysis of 44 major cities in India found that the SUHII was intense during daytime in cities in tropical climatic zones such as Chennai and Kolkata and the impact of vegetation on the determination of SUHII is obvious (Raj et al. Citation2020). A recent study in Delhi, India also reported a high degree of positive correlation between LST and Normalized difference built-up index(NDBI) (R2 = 0.8369) and negative correlation between LST and NDVI (R2 = −0.8872) (Singh et al. Citation2022). This is consistent with the results that the mitigation effect of vegetation on SUHI is stronger than the warming effect of buildings in this study. The GRW analysis further reveals that woody vegetation is more effective in lowing LST than agricultural lands. Therefore, urban green space and forests should be planned and provided to mitigate SUHI in BMR. According to Adulkongkaew et al. (Citation2020), the LST in the BMR can be greatly reduced by expanding the tree canopy to around 20%, water bodies to around 30%, or green yards to about 40% in urban areas. As reported by Estoque et al. (Citation2017), urban planning and management should be strengthened in the areas 2–12 km away from the city center. The mitigation strategies of UHI effect can be classified into three types: roof strategies (high reflectivity roofs, vegetation roofs), non-roof strategies (shading structures with energy generation/architectural devices, high reflectance paving, plantation, and water bodies), and covered parking strategies (Khare et al. Citation2021). The UHI effect can be considerably minimized by employing these measures.
5.2. Limitations and future perspectives
The spatiotemporal characteristics of SUHI provide valuable information for land use planning and management to mitigate SUHI in the study area. However, this study has several limitations. First, the study only investigated the daytime SUHII in winter due to the lack of remote sensing data. SUHI effects showed significant diurnal and seasonal variability (Mohammad and Goswami Citation2021). Multi-source remote sensing data can be applied to explore the diurnal and nocturnal LST changes in different seasons. Secondly, detailed land use types can be classified to examine the impact of land use changes on SUHI using remote sensing imagery with high spatial resolution (Keeratikasikorn and Bonafoni Citation2018b). Finally, SUHI patterns can be influenced by different elements, such as urban morphology, vegetation cooling, and background climate. Fine-scale datasets such as three dimensional morphology data of buildings and field measurements can facilitate the exploration of SUHI influencing factors and the implementation of practical heat mitigation measures (Pakarnseree et al. Citation2018; Arifwidodo and Chandrasiri Citation2021).
6. Conclusions
In this study, high spatiotemporal resolution remote sensing data were used to explore the variations in the thermal environment and their interactions with land cover features in the Bangkok Metropolitan Region. Monthly LST data were estimated using Landsat-like imagery from 2000 to 2020 with a five-year interval in the winter months. The rise in mean SUHII from 4.40 °C in 2000 to 5.76 °C in 2020 demonstrated an overall surface warming trend in the study area. The areas with high-level and very high-level SUHII values were mainly distributed in the urban core and eastern BMR. Low-level and very low-level SUHII areas were located on the urban fringe and coastal regions. The areal percentage of LST hot spots increased from 24.86% in 2000 to 29.13% in 2020. The gradient analysis results indicate that vegetation had a stronger effect on LST than the built-up area, while the effects of built-up land increased from 2000 to 2020. Woodlands had a more widely distributed cooling effect compared to agricultural lands. The increase in medium and high-density woody vegetation and green space would effectively mitigate the negative effects of SUHI in the BMR, especially in suburban and newly developed areas.
Although the present study provides insight for SUHI mitigation in the study area, the seasonal and nocturnal variations of urban thermal environment can be investigated in future studies. The application of more detailed data such as high-resolution remote sensing data, building morphology and field measurements can enable better understanding of the drivers of SUHI and the development of practical thermal mitigation strategies.
Disclosure statement
No potential conflict of interest was reported by the authors.
Data availability statement
The datasets used in this study is available from the corresponding author (Linlin Lu) upon reasonable request.
Additional information
Funding
References
- Adulkongkaew T, Satapanajaru T, Charoenhirunyingyos S, Singhirunnusorn W. 2020. Effect of land cover composition and building configuration on land surface temperature in an urban-sprawl city, case study in Bangkok Metropolitan Area, Thailand. Heliyon. 6(8):e04485.
- Arifwidodo S, Chandrasiri O. 2021. The effects of park improvement on park use and park-based physical activity. J Archit Urbanism. 45:73–79.
- Arifwidodo SD, Chandrasiri O. 2020a. Urban heat stress and human health in Bangkok, Thailand. Environ Res. 185:109398.
- Arifwidodo SD, Chandrasiri O. 2020b. Association between park characteristics and park-based physical activity using systematic observation: insights from Bangkok, Thailand. Sustainability. 12(6):2559.
- Arifwidodo SD, Tanaka T. 2015. The characteristics of urban heat island in Bangkok, Thailand. Proc-Soc Behav Sci. 195:423–428.
- Bonafoni S, Keeratikasikorn C. 2018. Land surface temperature and urban density: multiyear modeling and relationship analysis using MODIS and Landsat data. Remote Sensing. 10(9):1471.
- Cao S, Cai Y, Du M, Weng Q, Lu L. 2022. Seasonal and diurnal surface urban heat islands in China: an investigation of driving factors with three-dimensional urban morphological parameters. GISci Remote Sensing. 59(1):1121–1142.
- Chakraborty T, Lee X. 2019. A simplified urban-extent algorithm to characterize surface urban heat islands on a global scale and examine vegetation control on their spatiotemporal variability. Int J Appl Earth Obs Geoinf. 74:269–280.
- Chatterjee RS, Singh N, Thapa S, Sharma D, Kumar D. 2017. Retrieval of land surface temperature (LST) from Landsat TM6 and TIRS data by single channel radiative transfer algorithm using satellite and ground-based inputs. Int J Appl Earth Obs Geoinf. 58:264–277.
- Cheung PK, Livesley SJ, Nice KA. 2021. Estimating the cooling potential of irrigating green spaces in 100 global cities with arid, temperate or continental climates. Sustainable Cities Soc. 71:102974.
- Cheval S, Dumitrescu A, Irașoc A, Paraschiv M-G, Perry M, Ghent D. 2022. MODIS-based climatology of the Surface Urban Heat Island at country scale (Romania). Urban Clim. 41:101056.
- Deliry SI, Avdan ZY, Avdan U. 2021. Extracting urban impervious surfaces from Sentinel-2 and Landsat-8 satellite data for urban planning and environmental management. Environ Sci Pollut Res Int. 28(6):6572–6586.
- Dilawar A, Chen B, Trisurat Y, Tuankrua V, Arshad A, Hussain Y, Measho S, Guo L, Kayiranga A, Zhang H, et al. 2021. Spatiotemporal shifts in thermal climate in responses to urban cover changes: a-case analysis of major cities in Punjab, Pakistan. Geomatics Nat Hazards Risk. 12(1):763–793.
- Estoque RC, Murayama Y, Myint SW. 2017. Effects of landscape composition and pattern on land surface temperature: an urban heat island study in the megacities of Southeast Asia. Sci Total Environ. 577:349–359.
- Estoque RC, Ooba M, Seposo X, Togawa T, Hijioka Y, Takahashi K, Nakamura S. 2020. Heat health risk assessment in Philippine cities using remotely sensed data and social-ecological indicators. Nat Commun. 11(1):1581.
- Ezimand K, Chahardoli M, Azadbakht M, Matkan AA. 2021. Spatiotemporal analysis of land surface temperature using multi-temporal and multi-sensor image fusion techniques. Sustainable Cities Soc. 64:102508.
- Feng G, Masek JG, Schwaller MR, Hall FF. 2006. On the blending of the Landsat and MODIS surface reflectance: predicting daily Landsat surface reflectance. IEEE Trans Geosci Remote Sensing. 44(8):2207–2218.
- Fotheringham AS, Yang W, Kang W. 2017. Multiscale geographically weighted regression (MGWR). Ann Am Assoc Geogr. 107(6):1247–1265.
- Ghamisi P, Rasti B, Yokoya N, Wang Q, Hofle B, Bruzzone L, Bovolo F, Chi M, Anders K, Gloaguen R, et al. 2019. Multisource and multitemporal data fusion in remote sensing: a comprehensive review of the state of the art. IEEE Geosci Remote Sens Mag (Replaces Newsletter). 7(1):6–39.
- Giridharan R, Emmanuel R. 2018. The impact of urban compactness, comfort strategies and energy consumption on tropical urban heat island intensity: a review. Sustainable Cities Soc. 40:677–687.
- Guo G, Wu Z, Xiao R, Chen Y, Liu X, Zhang X. 2015. Impacts of urban biophysical composition on land surface temperature in urban heat island clusters. Landscape Urban Plann. 135:1–10.
- Halder B, Bandyopadhyay J, Banik P. 2021. Monitoring the effect of urban development on urban heat island based on remote sensing and geo-spatial approach in Kolkata and adjacent areas, India. Sustainable Cities Soc. 74:103186.
- Henao JJ, Rendón AM, Salazar JF. 2020. Trade-off between urban heat island mitigation and air quality in urban valleys. Urban Clim. 31:100542.
- Kaminski A, Bauer D, Bell K, Loftin C, Nelson E. 2021. Using landscape metrics to characterize towns along an urban-rural gradient. Landscape Ecol. 36:1–20.
- Kedia S, Bhakare SP, Dwivedi AK, Islam S, Kaginalkar A. 2021. Estimates of change in surface meteorology and urban heat island over northwest India: impact of urbanization. Urban Clim. 36(34):100782.
- Keeratikasikorn C, Bonafoni S. 2018a. Satellite images and gaussian parameterization for an extensive analysis of urban heat islands in Thailand. Remote Sensing. 10(5):665.
- Keeratikasikorn C, Bonafoni S. 2018b. Urban Heat Island Analysis over the Land Use Zoning Plan of Bangkok by Means of Landsat 8 Imagery. Remote Sensing. 10(3):440.
- Khare V, Vajpai A, Gupta D. 2021. A big picture of urban heat island mitigation strategies and recommendation for India. Urban Clim. 37:100845.
- Kim SW, Brown RD. 2021. Urban heat island (UHI) intensity and magnitude estimations: a systematic literature review. Sci Total Environ. 779:146389.
- Lemus-Canovas M, Martin-Vide J, Moreno-Garcia MC, Lopez-Bustins JA. 2020. Estimating Barcelona’s metropolitan daytime hot and cold poles using Landsat-8 Land Surface Temperature. Sci Total Environ. 699:134307.
- Limsakul A, Singhruck P. 2016. Long-term trends and variability of total and extreme precipitation in Thailand. Atmos Res. 169:301–317.
- Liu X, Zhou Y, Yue W, Li X, Liu Y, Lu D. 2020. Spatiotemporal patterns of summer urban heat island in Beijing, China using an improved land surface temperature. J Cleaner Prod. 257:120529.
- Lu L, Weng Q, Guo H, Feng S, Li Q. 2019b. Assessment of urban environmental change using multi-source remote sensing time series (2000–2016): a comparative analysis in selected megacities in Eurasia. Sci Total Environ. 684:567–577.
- Lu L, Weng Q, Xiao D, Guo H, Hui W. 2020. Spatiotemporal variation of surface urban heat islands in relation to land cover composition and configuration: a multi-scale case study of Xi’an, China. Remote Sensing. 12(17):2713.
- Lu L, Weng Q, Xie Y, Guo H, Li Q. 2019a. An assessment of global electric power consumption using the Defense Meteorological Satellite Program-Operational Linescan System nighttime light imagery. Energy. 189:116351.
- Malakar N, Hulley G, Hook S, Laraby K, Cook M, Schott J. 2018. An operational land surface temperature product for Landsat thermal data: methodology and validation. IEEE Trans Geosci Remote Sens. 56(10):5717–5735.
- Manoli G, Fatichi S, Schläpfer M, Yu K, Crowther TW, Meili N, Burlando P, Katul GG, Bou-Zeid E. 2019. Magnitude of urban heat islands largely explained by climate and population. Nature. 573(7772):55–60.
- Marcotullio PJ, Keßler C, Fekete BM. 2021. The future urban heat-wave challenge in Africa: exploratory analysis. Global Environ Change. 66:102190.
- Mathew A, Khandelwal S, Kaul N. 2018. Analysis of diurnal surface temperature variations for the assessment of surface urban heat island effect over Indian cities. Energy Build. 159:271–295.
- Mentaschi L, Duveiller G, Zulian G, Corbane C, Pesaresi M, Maes J, Stocchino A, Feyen L. 2022. Global long-term mapping of surface temperature shows intensified intra-city urban heat island extremes. Global Environ Change. 72:102441.
- Mohammad P, Goswami A. 2021. Quantifying diurnal and seasonal variation of surface urban heat island intensity and its associated determinants across different climatic zones over Indian cities. GISci Remote Sensing. 58(7):955–981.
- Mondal A, Guha S, Kundu S. 2021. Dynamic status of land surface temperature and spectral indices in Imphal city, India from 1991 to 2021. Geomatics Nat Hazards Risk. 12(1):3265–3286.
- Pakarnseree R, Chunkao K, Bualert S. 2018. Physical characteristics of Bangkok and its urban heat island phenomenon. Build Environ. 143:561–569.
- Pimonsree S, Limsakul A, Kammuang A, Kachenchart B, Kamlangkla C. 2022. Urbanization-induced changes in extreme climate indices in Thailand during 1970–2019. Atmos Res. 265:105882.
- Raj S, Paul S, Chakraborty A, Kuttippurath J. 2020. Anthropogenic forcing exacerbating the urban heat islands in India. J Environ Manage. 257:110006.
- Schwaab J, Meier R, Mussetti G, Seneviratne S, Bürgi C, Davin EL. 2021. The role of urban trees in reducing land surface temperatures in European cities. Nat Commun. 12(1):6763.
- Sekertekin A, Bonafoni S. 2020. Land surface temperature retrieval from Landsat 5, 7, and 8 over rural areas: assessment of different retrieval algorithms and emissivity models and toolbox implementation. Remote Sensing. 12(2):294.
- Singh CA, Verma P, Singh V, Meena S. 2022. Earth observation data sets in monitoring of urbanization and urban heat island of Delhi, India. Geomatics Nat Hazards Risk. 13:1762–1779.
- Sobrino JA, Oltra-Carrió R, Sòria G, Bianchi R, Paganini M. 2012. Impact of spatial resolution and satellite overpass time on evaluation of the surface urban heat island effects. Remote Sens Environ. 117:50–56.
- Stewart D, Oke TR. 2012. Local climate zones for urban temperature studies. Bull Amer Meterol Soc. 93(12):1879–1900.
- Sultana S, Satyanarayana ANV. 2020. Impact of urbanisation on urban heat island intensity during summer and winter over Indian metropolitan cities. Environ Monit Assess. 191(3):789.
- Tariq A, Riaz I, Ahmad Z, Yang BS, Amin M, Kausar R, Andleeb S, Farooqi MA, Rafiq M. 2020. Land surface temperature relation with normalized satellite indices for the estimation of spatio-temporal trends in temperature among various land use land cover classes of an arid Potohar region using Landsat data. Environ Earth Sci. 79(1):40.
- United Nations. 2015. Transforming our world: the 2030 Agenda for Sustainable Development. New York (NY).
- Venter ZX, Chakraborty T, Lee X. 2021. Crowdsourced air temperatures contrast satellite measures of the urban heat island and its mechanisms. Sci Adv. 7(22):eabb9569.
- Weng Q, Firozjaei MK, Sedighi A, Kiavarz M, Alavipanah SK. 2018. Statistical analysis of surface urban heat island intensity variations: A case study of Babol city, Iran. GISci Remote Sensing. 56(4):576–604.
- Weng Q, Fu P, Gao F. 2014. Generating daily land surface temperature at Landsat resolution by fusing Landsat and MODIS data. Remote Sens Environ. 145:55–67.
- Worawiwat A, Chaleeraktrakoon C, Sharma A. 2021. Is increased flooding in Bangkok a result of rising local temperatures? J Hydrol X. 13:100095.
- Xian G, Shi H, Zhou Q, Auch R, Gallo K, Wu Z, Kolian M. 2022. Monitoring and characterizing multi-decadal variations of urban thermal condition using time-series thermal remote sensing and dynamic land cover data. Remote Sens Environ. 269:112803.
- Xie J, Ren C, Li X, Chung L. 2021. Investigate the urban growth and urban-rural gradients based on local climate zones (1999–2019) in the Greater Bay Area, China. Remote Sens Appl: Soc Environ. 25:100669.
- Xu G, Jiao L, Liu J, Shi Z, Zeng C, Liu Y. 2019. Understanding urban expansion combining macro patterns and micro dynamics in three Southeast Asian megacities. Sci Total Environ. 660:375–383.
- Zhao L, Lee X, Smith RB, Oleson K. 2014. Strong contributions of local background climate to urban heat islands. Nature. 511(7508):216–219.
- Zhou D, Xiao J, Bonafoni S, Berger C, Deilami K, Zhou Y, Frolking S, Yao R, Qiao Z, Sobrino JA. 2019. Satellite remote sensing of surface urban heat islands: progress, challenges, and perspectives. Remote Sensing. 11(1):48.
- Zhu X, Chen J, Gao F, Chen X, Masek JG. 2010. An enhanced spatial and temporal adaptive reflectance fusion model for complex heterogeneous regions. Remote Sens Environ. 114(11):2610–2623.
- Zhu X, Helmer EH, Gao F, Liu D, Chen J, Lefsky MA. 2016. A flexible spatiotemporal method for fusing satellite images with different resolutions. Remote Sens Environ. 172:165–177.