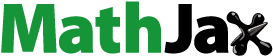
Abstract
InSAR technology provides a powerful tool for detecting large-scale surface deformation. In particular, the newly developed DS-InSAR method fused PS points has obvious advantages in monitoring bare land and vegetation covered areas, but at present, there is a lack of effectiveness evaluation of this method. Therefore, this paper takes Hongta District of Yuxi City as an example. The 29 scenes sentinel-1A data from January 2019 to December 2019 were processed and analyzed using the DS-InSAR method fused PS points. The research results show that the position and deformation trend of the inversion results of the three methods are highly consistent, the correlation between DS-InSAR method fused PS points and PS- InSAR and SBAS-InSAR methods is 0.9473 and 0.8583, respectively. But the spatial density of the measurement points (MPs) obtained by the DS-InSAR method of PS points is 11 times and 4 times that of the PS-InSAR method and SBAS-InSAR method respectively. It clearly shows that DS-InSAR method fused PS points has greater advantages than PS-InSAR and SBAS-InSAR methods in deformation mapping displacement, because the MPs density is higher. It is conducive to the detailed analysis of the spatio-temporal characteristics and deformation mechanism of deformation.
1. Introduction
Surface subsidence is an environmental geological phenomenon that causes the gradual or sudden subsidence of the earth’s surface under the action of natural and man-made affiliation (Zheng et al. Citation2002; Liu et al. Citation2015). It is a slow and progressive process, once formed, it is difficult to recover (Yin et al. Citation2005). Surface subsidence is one of the most common geological hazards in many countries. For example, more than 40 states in the United States and nearly 20 provinces in China have experienced land subsidence to varying degrees (Dong et al. Citation2014). It was severely destructive, bringing serious environmental problems and large economic losses, such as damage to urban infrastructure, including bridges (Selvakumaran et al. Citation2018), buildings (Yang et al. Citation2016), railways (Karanam et al. Citation2021), highways (Zhu et al. Citation2021), and underground facilities (water supply pipelines, gas, power facilities, etc.) (Jiang et al. Citation2018; Hu et al. Citation2014; Garg et al. Citation2022).
Although the traditional monitoring methods have relatively reliable monitoring results at the monitoring points, their spatial resolution is low, it is difficult to obtain the deformation at non-monitoring points, and the monitoring cost is high, so it is difficult to carry out fine monitoring of wide-area surface deformation, which limits our comprehensive understanding of the deformation field and the spatio-temporal evolution characteristics of deformation in the entire study area (Luo et al. Citation2014). Synthetic Aperture Radar Interferometry (InSAR) is a new type of earth observation technology that has been developed rapidly in the past 30 years. It is widely used in various kinds of deformation monitoring owing to its high spatial resolution, all-day, all-weather and other characteristics (Zhu et al. Citation2017; Yuan et al. Citation2020; Zhang et al. Citation2022). Compared with conventional geodetic techniques such as global positioning system (GPS) and levelling, InSAR technology can obtain wide-area surface deformation time series with significantly improved spatial resolution (Bai et al. Citation2016).
In recent years, many advanced InSAR methods and some improved methods have emerged (Cao et al. Citation2016). The PS-InSAR technique is the first systematic time-series processing solution, whose main idea is to select one scene image from the image collection as the reference primary image, other images as secondary images and the reference primary image for differential interference processing (Ferretti et al. Citation2002a, Citation2002b). Select the point target with high signal noise ratio (SNR) and coherence, and achieve the extraction of deformation information by the optimal estimation is used to extract the deformation information and effectively overcome the decoherence and atmospheric effects of the images (Ferretti et al. Citation2011). With the widespread application of PS-InSAR technology, the drawback that its coherent targets are not effectively mined has become increasingly obvious, especially in non-urban areas where the PS point density is usually less than 10/km2, making it difficult to obtain continuous surface deformation information (Samiei-Esfahany et al. Citation2016). Nevertheless, its technical ideas have been influencing the subsequent development of time-series processing techniques.
The SBAS-InSAR method, which was proposed almost simultaneously with PS-InSAR, fully considers the mining of distributed target information. In order to overcome the effect of decoherence, this technique usually selects only the interferometric pairs with relatively short baselines and performs singular value decomposition of the deconvolution results of a subset of interferometric pairs according to the redundant observations formed by the combination of image interferometric pairs to improve the accuracy of deformation monitoring (Berardino et al. Citation2002; Yastika et al. 2019). Although this technique has been improved, it has not been able to escape from the dilemma of two-dimensional deconvolution, and the accuracy of deformation monitoring has not been improved fundamentally (Lauknes et al. Citation2011).
To overcome the shortcomings of the above InSAR methods. Ferretti et al. (Citation2011) proposed the SqueeSAR technique, which determines whether a pixel is homogeneous by the similarity of each pixel intensity statistical distribution and performs spatial adaptive filtering based on the set of homogeneous pixels, and then uses the Phase-Linking technique to achieve the parity calculation of the winding phase (Sun et al. Citation2018). Since then, the DS-InSAR technique has been widely used, for example. Pawluszek-Filipiak et al. (Citation2020) combined SBAS-InSAR and D-InSAR to study mining subsidence. Seyfallah Bouraoui (Citation2013) merged PS and small baseline (SB) methods for oilfield, landslide, and seismic subsidence monitoring. Hu et al. (Citation2014) analyzed the temporal and spatial characteristics of land subsidence in the Beijing area using SBAS-InSAR technology, revealing that the monitoring results of InSAR technology are consistent with the levelling results. Luo et al. (Citation2014) adopted Multi-temporal InSAR (MT-InSAR) technology to monitor and analyze land subsidence in the Tianjin area, and verified it with GPS data. The results suggested that the monitoring results of the two monitoring methods were in good agreement. Lubna et al. (Citation2018) employed ISBAS-InSAR technology to monitor the long-term surface movement of peatlands in northeastern Scotland, and the monitoring results were consistent with the trend of surface movement. Zhang et al. (Citation2019) monitored and investigated the surface subsidence in Wuhan with SBAS-InSAR technology, and validated its results through levelling data. With the landslide in Danba County, Sichuan as an example, Zhang et al. (Citation2018) and Dong et al. (Citation2018) used the time-series InSAR technology to identify the hidden hazards of landslide disasters early and demonstrated the reliability of the results through GPS data. Zhao et al. (Citation2019) conducted multi-temporal cataloging and long-term monitoring of potential loess landslides in Heifangtai, Gansu using InSAR technology, combined topographic maps and optical remote sensing images to further explore the instability modes of landslides, and obtained the accuracy of the landslide instability model through field surveys to verify the performance. Given the time-consuming issue of time-series analysis in acquiring images and data preprocessing in the study area, a new method of time-series analysis, LiCSBAS, was adopted to monitor surface deformation (Gao et al. Citation2020). The results unveiled that this method can considerably improve the efficiency of the solution. Yao et al. (Citation2022) used a cross-comparison approach to study landslides along the Jinsha River in Gongjue County using the SBAS-InSAR and PS-InSAR techniques. Bui et al. (Citation2021) monitoring recent land deformation detected in Hanoi, vietnam using InSAR technology and analyzing its relationship with groundwater level changes. Orhan (Citation2021) investigate the causes of the land subsidence problem in Konya and its surroundings with the help of the SBAS-InSAR technique and auxiliary data, namely optic, Coordination of Information on the Environment (CORINE), and groundwater monitoring station data.
In summary, the DS-InSAR method fused PS point has been successfully applied to various surface deformation monitoring, but at present, only the DS-InSAR method fused PS points is used for surface deformation monitoring and analysis, lacking effective comprehensive evaluation and analysis. Therefore, this paper takes Hongta District of Yuxi City as the experimental object and uses the above the DS-InSAR method fused PS points to monitor its surface deformation, and The results are verified and analyzed with PS-InSAR and SBAS-InSAR results to highlight the advantages of DS-InSAR method fused PS point in surface deformation monitoring. The results of the study provide a scientific basis for the selection of the time-series InSAR technique.
2. Distributed scatterer InSAR method fused with PS
Distributed scatterers (DS) are implemented in the presence of random resolution scatterers with similar amplitudes, and none of them exhibit dominant behaviour. The DS candidate points (DSC) are obtained by comparing the similar features of the interference phase in the time dimension, and then homogenous filtering is performed on them. The phase is optimized in the complex domain to obtain the final DS points. This processing can effectively suppress the influence of noise and improve the signal-to-noise ratio (SNR), contributing to boosting the quality of the interferogram phase and obtaining deformation information with higher precision and enhanced spatial resolution. Compared with other time-series processing methods, this method can effectively improve the spatial density of coherent scatterer points. The key technologies of DS-InSAR involve (1) improving the estimation accuracy of the time series InSAR covariance matrix by performing spatial adaptive filtering on the pixel set of the homogeneous scatterer; (2) recovering the time series SAR image from the covariance matrix through the phase optimization algorithm to achieve the time-dimension phase adjustment.
Anderson-Darling (A-D) hypothesis test is an adaptive multi-view non-parametric test method improved based on the Kolmogorov-Smirnov (K-S) test [13]. Similar to the K-S test, the A-D test statistics are also dependent on the empirical cumulative distribution function (ECDF) of the amplitude between two pixels to judge their similarity. During the processing, a proper estimation window is set with each pixel of the image as the centre, and the consistency of the pixels in the window is judged by the statistical test method. It is assumed that N SAR images are given, one is selected as the primary image, and the remaining (N-1) images are used as auxiliary images. The amplitude empirical cumulative distribution function (ECDF) of any pixel p can be calculated by:
(1)
(1)
where
indicates a function. When
it is equal to 1, otherwise it is 0. For any two pixels
in the statistical window, the AD test statistics can be expressed as:
(2)
(2)
where
denotes the empirical cumulative distribution function of two pixels p and q combined into one pixel. The A-D test statistic is more complicated than the K-S test statistic, and there is no fixed solution. Pettitt (Citation1976) detailed the approximation of the A-D test and the selection of the threshold according to the significance level. Compared with the K-S method, the A-D test pays more attention to the tail of the distribution. Parizzi and Brcic (Citation2011) demonstrated that the A-D test has better performance than other non-parametric tests for the homogeneous point selection in the synthetic aperture radar stack.
After the homogenous points are obtained by the A-D test, the eigendecomposition method is adopted to estimate the parameters of the covariance matrix of the homogenous points. The reflection signal received from the DS resolution unit is equal to the sum of the coherent information of the reflections from multiple elementary scatterers in the unit. Following the central limit theorem, its pixel values obey the complex circular Gaussian distribution. It is assumed that a set consists of N single-view complex SAR images. This set can be regarded as a complex random variable and its probability density function (PDF) can be expressed as:
(3)
(3)
where
indicates the
complex covariance matrix, and H represents the Conjugate multiplication.
The complex coherence coefficient between the two images
and
can be expressed by Chen and Meng (2020):
(4)
(4)
where
denotes the complex coherent phase value between the two scene images, and
signifies the absolute value of the coherence coefficient, which is a measure of the correlation between the two scene images. The noise component between the two scene images has relatively high similarity when the coherence is high. Hence, most of the noise components will cancel out during the interference phase. The complex correlation matrix between images is called the coherence matrix, defined as:
(5)
(5)
The coherence matrix Equation(5)(5)
(5) is a positive definite Hermitian matrix, which can be written as:
(6)
(6)
where
denotes a diagonal matrix composed of non-negative eigenvalues with diagonal elements, and
indicates its corresponding feature vector. The eigenvalues are sorted in descending order to obtain
T can be interpreted as the sum of
scatterer coherence matrices, where each coherence matrix represents an independent scatterer.
(7)
(7)
When the following eigenvalues are small, the coherence matrix can be written approximately as:
(8)
(8)
Generally, the characteristic eigenvector corresponding to the maximum eigenvalue is its optimal phase value. Due to the uncertainty of the obtained eigenvectors (Zhu et al. Citation2017), there are multiple eigenvectors for the same eigenvalue. In other words, if the eigenvector corresponding to the eigenvalue of matrix
is
then:
(9)
(9)
For any non-zero complex constant there is:
(10)
(10)
Since is also the eigenvector of
the eigenvector multiplied by a non-zero complex constant is an eigenvector. This will result in a constant offset between the actual phase and the estimated phase. Thus, the original interference phase value and estimated phase value of the primary image are set to zero in our study to calculate and eliminate the above offset.
After the final DS point is obtained, the 2D linear regression analysis of the unfolded interference phase is performed with PS to correct the error introduced by the external DEM. Subsequently, the residual atmospheric phase and nonlinear deformation are removed by the difference in time and space characteristics.
3. Research area and experimental data
3.1. Study area
The study area is located in the hinterland of central Yunnan, in the interval of 24°08′30″–24°32′18″ north latitude and 102°17′32″–102°41′37″ east longitude. It is connected to Jiangchuan District in the east and Tonghai County in the southeast. The southwest borders with Eshan Yi Autonomous County, and the north borders with the Jinning District of Kunming City. The transportation in the area is convenient. The 213 National Road and Kun-Yu Railway run through the north and south, forming a north-south transportation hub in Yunnan Province. The average annual total amount of water resources is 430 million cubic metres, of which groundwater accounts for 29%. The specific location of the study area is exhibited in .
Figure 1. Study area location and DEM. The left picture shows the location of the study area and the right picture shows the DEM of the study area.

The study area belongs to the middle-north section of Yuxi north-south tectonic basin, which is a tectonic denudation low-mountain (hilly) topography, and is in the long-term bending area on the platform base, so the topography and geomorphology vary greatly in east-west direction, and the topography does not change much in north-south direction, but the stratigraphic lithology varies greatly, and the overall area is the intersection of Quaternary lake-phase alluvium, siltation and mountain margin, and there is bedrock outcropping only in local sections. Only the localized rock is revealed. According to relevant geological data, the formation of geodorrocysts in the study area mainly adopts artificial filling, sludge clay, powder clay, gravel soil, fine sandstone, muddy, and gray rock (Figure2), with poor stratosphere stability. The pleats in the study area are relatively developed, exhibit relatively fragile environmental geological conditions, and could induce corresponding geological disasters related to human activities.
3.2. Experimental data and processing
In this study, 29 scenic C-band descending sentienl-1A images in the study area are selected as experimental data, and their imaging time span is from January 2019 to December 2019. The polarization method is VV polarization, the wavelength is 5.63 cm, the revisit cycle is 12 days. The resolution is 5 m × 20 m, and each image consists of three sub-bands and multiple bursts. In order to remove the influence of terrain phase, the elevation data of the Shuttle Radar Topography Mission (SRTM-1) is selected as a reference, and the resolution is 30 m. The specific experimental data parameters are listed in .
Table 1. Experimental data parameters.
The distributed scatterer InSAR method data processing flow of the PS point is illustrated in . Since the azimuth registration accuracy of sentinel-1A satellite needs to reach 0.001 pixel during interference processing. On this basis, the precision track geometry enhanced spectrum set registration is performed (Wu et al. Citation2019). Based on the comprehensive consideration of the influence of temporal baseline, space baseline and doppler centroid frequency, the image data of April 22, 2019 is selected as the primary image (see the blue triangle in ), and other secondary images are registered to the primary image, and then the interferograms is generated by multiple primary images. When selecting PS points, the amplitude deviation threshold method is used to initially candidate PS points, and the amplitude deviation threshold is set to 0.4. The temporal coherence threshold is set to 0.35.
Figure 3. Distributed scatterer InSAR data processing flow of fusion PS. The red box is PS point selection process, the green box is DS point selection process.

Figure 4. Time-space baseline map. The blue triangle represents the primary image, the red rectangle represents the secondary image.

When the homogenous filter is processed, the filter window is set to 49 pixels, the orientation is 13 pixels, and the homogeneous pixel number threshold is minimized to 50 pixels. The coherent matrix and covariance matrix estimations are performed by interference graphics. The Feature decomposition (EVD) method is employed to decompose the coherent matrix, and phase optimization estimation is implemented. The original differential interferograms and the differential interference chart after phase optimization are provided in . show the original differential interferograms for the shortest temporal baseline (12d) and the longest temporal baseline (252d), respectively. shows the differential interferograms after phase optimization for the shortest temporal baseline (12d) and the longest temporal baseline (252d), respectively. suggests that the phase optimization is significantly better compared to the original interferograms, especially when the temporal baseline is long. Besides, the non-urban area is more significant, as demonstrated outside the black rectangular frame in , inside the urban area.
Figure 5. Differential interferogram before and after phase optimization. (a) and (c) are interferograms before phase optimization, and (b) and (d) are interferograms after phase optimization.

We choose bust6, burst7 and burst8 for data processing, and when using the DS-InSAR method of integrating PS points for data processing, it uses the joint PS and DS points for unified networking. Additionally, the corresponding parameter estimation algorithm is adopted to solve its phase observation value Wrap and error separation. Finally, the deformation rate and cumulative deformation in the study area are obtained.
4. Results analysis
4.1. Deformation rate analysis
shows the deformation rate obtained by PS-InSAR, SBAS-InSAR and DS-InSAR method integrating PS points. A positive value indicates that the monitoring scatterer is close to the line of sight (LOS) movement; a negative value reflects that the line of sight (LOS) is moved away. The red rectangular frame in the figure is where the Red Tower main city is located. The measurement points obtained by the PS-InSAR method are mainly distributed in urban areas, while in bare land and vegetation covered areas, the PS points are sparse, and he deformation position can only be roughly detected from the deformation results, which is difficult to reflect the specific deformation range and boundary (). Compared with the PS-InSAR method, the number of measurement points identified by SBAS-InSAR method has increased, but it can be seen from the figure that the range of deformation area is relatively vague, and the boundary of deformation area cannot be clearly determined (). The number of measurement points obtained by DS-InSAR method integrating PS points has increased significantly, especially in bare land and vegetation covered areas, and the deformation location and range boundary can be clearly determined according to deformation information. The DS-InSAR monitoring results suggest 4 deformation areas in the Hongta District of Yuxi City, which are distributed in the main city, located near Beicheng Street, Black Village – Yellow Mud Field (2 #), Daxia Street – Near Gaotang Street (3 #), Relien Street (4 #). Among them, the most severe deformation is 1 # region (), the Shenzhang District is the centre, north to Meiyuan Village, west to Lianchi Village, south to Liu Zhangying, and East to Honglong Road. The range is large, the deformation is more severe, and the maximum deformation rate is −71.58 mm/a. 2 #, 3 #, and 4 # deformation areas will not be significant, and the deformation rate is within -30mm/a.
Figure 6. Deformation rate map of InSAR monitoring results in various time series. (a) PS-InSAR results, (b) SBAS-InSAR results, (c) DS-InSAR results, and (d) 1# magnified rendering.

In addition, it is worth noting that there is an obvious deformation area in the north of (shown by the black rectangle), but the deformation area is not obvious in . After analysis, it is considered that the deformation area is affected by mining, and the decoherence is serious in a long time interval. However, both PS-InSAR and DS-InSAR methods with PS points have interference pairs with long time intervals, so these two methods lose their monitoring performance. SBAS-InSAR adopts the combination strategy of short-time baseline, which can effectively obtain the deformation information of this area.
4.2. Key deformation regional analysis
As observed in , the surface deformation rate of the study area is mainly distributed between −71.58 ∼ 31.4 mm/a. Near North City Street (1 #) is the largest in the monitoring area, and the deformation is the most remarkable. Therefore, the analysis is focussed on the subsidence area 1#, as shown inside the black dashed rectangle in , and the local amplified subsidence rate maps of the 1# subsidence area from the monitoring results of PS-InSAR, SBAS-InSAR, and DS-InSAR technique with fused PS points are shown in , respectively. Monitoring results of 1 # deformation area partially enlarged the deformation rate map. Besides, the DS-InSAR monitoring results can reflect the surface deformation details, which are more significant. The white dashed lines and white solid lines are presented in . The figure suggests that the white dashed lines are more severe, and the deformation rate is less than −30 mm/a. Meanwhile, the deformation between white dashed lines and solid lines is relatively small, and the deformation rate is −30 mm/a ∼ −10 mm/a. There is almost no significant deformation of the white solid line outside.
Figure 7. Deformation rate map of 1# deformation area obtained by InSAR technology in each time series. (a) results of DS-InSAR, (b) results of PS-InSAR in Area 1#, (c) results of SBAS-InSAR in Area 1#, and (d) results of DS-InSAR in Area 1#.

Through statistical analysis of the number and spatial density of typical area measurement points (MPs) in , as shown in . A total of 33,720 MPs were extracted by PS-InSAR technology, and their spatial density was 450/km2. A total of 93,329 MPs were extracted by SBAS-InSAR, and their spatial density was 1244/km2. The DS-InSAR technology with PS points extracted a total of 372,188 MPs with a spatial density of 4693/km2. The statistical results show that the MPs density extracted by DS-InSAR method with PS points is about 11 times that of PS-InSAR method and 4 times that of SBAS-InSAR method.
Table 2. Number of MPs and their spatial density in the results of the three methods.
is a segmentation rate cross-sectional view drawn along the A-A 'and B-B' sections shown in for further illustrating the settling characteristics of the settling region. is a deformation rate along the cross-sectional view of A-A', and is a deformation rate along B-B'. It can be seen from that the variation trend of deformation rate obtained by the three methods is highly consistent. As the DS-InSAR method integrating PS points increases the spatial density of MPs, it has great advantages in drawing the details of deformation rate changes. There is an obvious jumping phenomenon at the boundary positions of deformed and non-deformed areas, as shown by the red vertical dotted line in . Therefore, the deformation area boundary can be accurately drawn according to the details of deformation rate changes. The other two methods are difficult to reflect the details of surface deformation due to the small number of MPs obtained.
4.3. Analysis of changes in time and space characteristics
In order to better demonstrate the spatio-temporal characteristics of the temporal deformation of the key subsidence area during January 2019–December 2019, the temporal cumulative deformation of the 1# subsidence area was obtained by taking the primary image (2019-04-22) as a reference and setting its cumulative deformation to 0. The cumulative deformation of the area is exhibited in . The figure demonstrates that the cumulative variable and deformation range gradually increases with the increase in the temporal baseline, the cumulative deformation did not show a strictly linear variation with the temporal baseline; the maximum cumulative deformation reaches 57 mm. From 2019-04-22 to 2019-12-30, its cumulative deformation and temporal baseline did not present strict linear changes from 2019-04 to 2019-07. It is faster from 2019-04 to 2019-07, during which the maximum deformation amount is −59 mm. The study area exhibited a significant uplift phenomenon from October 19, 2019 to October 31, 2019 and a significant subsidence phenomenon until December 30, 2019, reaching the maximum cumulative subsidence value of −71 mm. During the monitoring period, the first is in the northern part of the deformation area, and the deformation centre gradually moves in the southwest direction.
Thus, the length of the study area surface deformation and temporal baseline is not a strictly linear relationship, but exhibits seasonal changes. renders the timing of 3 points in the deformation area. The deformation in the study area is the most serious during April 2019 to July 2019 (see red rectangle in ). The maximum cumulative deformation amount is about −59 mm during this period. A rising phenomenon appeared in August and October 2019 (the black ellipse in ).
4.4. Distributed Scatterer InSAR reliability analysis of fusion PS points
The ground deformation rate of multiple temporal InSAR technologies is correlated to illustrate the reliability of the distributed scatterer InSAR surface deformation monitoring results. The PS-InSAR is used as a reference value, and the nearest neighbour method is adopted. The DS-InSAR monitoring results of SBAS-InSAR and the fusion PS points are searched for in a certain spatial range (50 m). The Measurement PS Point is the measurement point acquired by multiple temporal InSAR technologies and correlates to the deformation rate of the same name (). Among them, exhibits a PS-InSAR method to monitor the deformation rate coherence analysis results, with a total of 2375 coincident points and a correlation coefficient of 0.9302. shows the results of correlation analysis between the PS-InSAR method and the DS-InSAR method monitoring results of fused PS points, and a total of 5429 coincident points were identified with the correlation coefficient of 0.9473. shows the results of correlation analysis between the SBAS-InSAR method and the DS-InSAR method monitoring results of fused PS points, and a total of 2094 coincident points were identified with the correlation coefficient of 0.8583. The three InSAR monitoring methods are highly correlated, especially in areas with low deformation rate, while in areas with high deformation rate, the correlation is relatively low, as shown in the red ellipse in . The monitoring results of PS-InSAR and SBAS-InSAR show that the deformation rate is obviously low, because the MPs density identified by PS-InSAR and SBAS-InSAR is low, and the distance between two adjacent points is too large, which leads to errors. Additionally, the coherent coefficient between PS-InSAR and the DS-InSAR of the fusion PS points is 0.9473, because the PS point is fused when the DS-InSAR processing of the PS point is fused. The method has a high coherence. The settling results obtained by the three methods have high consistency. However, the DS-InSAR monitoring method of fusion PS points is superior to the PS-InSAR and SBAS-InSAR methods in a large region.
Figure 11. The correlation map of deformation rate of the InSAR monitoring results in each time. (a) the deformation rate correlation between PS-InSAR and SBAS-InSAR, (b) the deformation rate correlation between PS-InSAR and DS-InSAR, and (c) the deformation rate correlation between SBAS-InSAR and DS-InSAR.

statistically illustrates the deformation rate difference histogram of the monitoring results obtained by three timing InSAR methods. As can be seen from the histogram of the difference in deformation rate, the difference in deformation rate between the coincident points of the monitoring results of the three time-series InSAR techniques is small, and the vast majority of them are distributed between -15mm/a and 15 mm/a. The number of coincident points with the absolute value of the difference in deformation rate between PS-InSAR and SBAS-InSAR, DS-InSAR of PS-InSAR and fused PS points, and SBAS-InSAR and fused PS The numbers of coincident points with absolute values of the difference in deformation rate between the DS-InSAR monitoring results of the points within 5 mm/a were 1088, 2710, and 780, accounting for 45.8%, 49.9%, and 37.2% of the number of coincident points screened, respectively. The sequential rate differences in each timing InSAR technology monitoring result suggest that the distributed scatterer InSAR monitoring results have high reliability.
Figure 12. The histogram of difference in the deformation rate of the points with the same mame among the InSAR monitoring results in each time series. (a) the histogram of deformation rate difference between PS-InSAR and SBAS-InSAR, (b) the histogram of deformation rate difference between PS-InSAR and DS-InSAR, and (c) the histogram of deformation rate difference between SBAS-InSAR and DS-InSAR.

From the perspective of data processing, the stability of the Delaunay triangulated irregular network can be significantly increased by combining PS and DS to construct Delaunay triangulated irregular network. Meanwhile, the reliability of phase unwrapping can be improved. Additionally, the quality of the interferogram is improved through the optimization of its phase. This is beneficial to obtain relatively reliable monitoring results of land subsidence.
4.5. Relationship between surface deformation and rainfall in research area
Normally, rainfall is one of the crucial factors leading to surface deformation. The five InSAR measuring points shown in in the study area are selected for the image of 2019-01-04 to analyze the formation of the research area and its relationship with the rainfall. The time series accumulated deformation amount is calculated. The accumulated deformation curves in and the same period of rainfall are plotted. The rainfall data are collected from GodDard Earth Sciences Data and Information Services Center (https://disc.gsfc.nasa.gov/). unveils that the amount of accumulated deformation at each point exhibits a certain seasonal change, and its change has a certain relationship with rainfall. Besides, the displacement deformation has a significantly accelerated sign before the rainy season of June 2019 and October 2019. The cumulative deformation during the rainy season of June to October reaches its peak, and its deformation speed slows down. After the rainy season, a short-term lifting phenomenon occurs after October 2019, and the subsequent movement significantly changes. The accelerated phenomenon in the rainy season and the time of the rainy season could be investigated based on the above analysis. The displacement speed is slower during the rainy season. This phenomenon could be explained as follows. Heavy rain causes a large amount of rain into the ground, and the soil water content increases as the rain is constantly accumulated. As a result, the soil porosity and the volume increase, and the surface variable speed slows down and even lifts. With the decrease in rainfall, the groundwater gradually loses, and the surface level movement will have a significant acceleration.
5. Discussion
In this paper, we apply PS-InSAR, SBAS-InSAR and DS-InSAR method combined with PS points to the same research area, and find that DS-InSAR method combined with PS points can effectively increase the number of measurement points and significantly improve the density of MPs. It also allows us to define and update the boundaries of the deformed and non-deformed regions more accurately.
Compared with PS-InSAR and SBAS-InSAR methods, the DS-InSAR method combining PS points can obtain more measurement points and better monitoring results. However, the pre-processing of this method is quite time-consuming and the computation time cost is about 4-5 times of other time-series InSAR methods, which is the main bottleneck of its application. For surface deformation monitoring over large areas, the time cost of DS-InSAR analysis may increase to a very high level. Therefore, how to utilize modern massively parallel computing techniques, such as graphics processing units (GPUs), to improve computational efficiency becomes a critical issue to be addressed. Another key issue worth investigating is how to optimize the DS-InSAR algorithm, especially the DS preprocessing process, in order to significantly reduce the storage space consumption.
6. Conclusion
In this paper, the sentinel-1A data is selected, and the distributed scatterer InSAR method of fusion PS is used to monitor and analyze the surface deformation of Yuxi City Hongta District in January 2019, and the reliability of the method in this paper was verified by the conventional PS-InSAR and SBAS-InSAR methods. The conclusions can be drawn as follows.
There are 4 main deformation areas in the Hongta District of Yuxi City, which is located in the deformation area near North City Street. The maximum deformation rate reaches −71.58 mm/a, as indicated in the cross-sectional analysis. The significant jump phenomenon reveals that the segmentation of the region may be induced by surface collapse or tomography. The deformation rates of the remaining three settling regions are relatively small, all within −30 mm/a.
At the time of time-sequential variable spatial feature analysis, the research area surface deformation showed seasonal changes, and the deformation in April and July 2019 was the most severe. The maximum deformation reached 59 mm. The uplift occurred twice in August 2019 and October 2019, and the slow subsidence occurred in other periods. Over time, the location of the subsidence centre moves from northeast to southwest.
The distributed scatterer InSAR method of fusion PS can be employed to obtain higher measurement point density than conventional PS-InSAR and SBAS-InSAR methods, especially compared to the PS-InSAR method. Moreover, its measurement point density is boosted. Thereby, the boundary between the deformation area and the non-deformation area can be delineated more precisely.
The monitoring results of the fused distributed scatterer InSAR method and conventional PS-InSAR and SBAS-InSAR methods are highly consistent on spatial position distribution, implying that their monitoring results are high. The correlation analysis demonstrates that in a region with a small deformation rate, the correlation is high, and the region-dependent correlation rate in the deformation rate is relatively low. Moreover, the deformation rate of the two methods of PS-InSAR and SBAS-InSAR are significantly smaller. This further verifies that the method can be utilized to more accurately acquire the deformation rate
Although the study area formation information has been successfully obtained, there are some shortcomings in this study. First, the conditions are limited, and the reliability and accuracy of this method are verified only by the monitoring results of the conventional timing InSAR method, instead of the measured data. Second, the results in this paper have been monitored and analyzed only in the study area, and its deformation mechanism is neglected. In order to better analyze the deformation mechanism of the study area, the corresponding deformation mechanism model will be established subsequently by combining the knowledge of other disciplines such as geohydrology and environment. To provide more accurate prediction and service to prevent potential geological hazards such as cave-in in the study area.
Acknowledgments
The sentinel-1A datasets were freely provided by the European Space Agency (ESA) and freely downloaded from the website https://vertex.daac.asf.alaska.edu. The NASA for providing the SRTM-1 DEM data and freely downloaded from the website http://rmw.recordist.com/index.html. The Precipitation Orbit Data (POD) was freely downloaded from the website https://qc.sentinel1.eo.esa.int/. The Rainfall data were freely downloaded from the website https://disc.gsfc.nasa.gov/.
Disclosure statement
No potential conflict of interest was reported by the authors.
Data availability statement
The data that support the findings of this study are available from the first author, Yongfa Li, upon reasonable request.
Additional information
Funding
References
- Bai L, Jiang L, Wang H, Sun Q. 2016. Spatiotemporal characterization of land subsidence and uplift (2009–2010) over Wuhan in central China revealed by TerraSAR-X InSAR analysis. Remote Sens. 8(4):350–350.
- Berardino P, Fornaro G, Lanari R, Sansosti E., 2002. A new algorithm for surface deformation monitoring based on small baseline differential SAR interferograms. IEEE Trans Geosci Remote Sens. 40(11):2375–2383.
- Bouraoui S. 2013. Time series analysis of SAR images using persistent scatterer (PS), small baseline (SB) and merged approaches in regions with small surface deformation. Université de Strasbourg (Doctoral dissertation). English. NNT: 2013STRAH023. tel-01019429.
- Bui LK, Le PVV, Dao PD, Long NQ, Pham HV, Tran HH, Xie L. 2021. Recent land deformation detected by sentinel-1a InSAR data (2016–2020) over Hanoi, Vietnam, and the relationship with groundwater level change. GISci Remote Sens. 58(2):161–179.
- Cao N, Lee H, Jung HC. 2016. A phase-decomposition-based PSInSAR processing method. IEEE Trans Geosci Remote Sens. 54(2):1074–1090.
- Chen X, Meng C. 2019. Analysis of ground fissures formation and disaster characteristics in Northern Henan Plain. Groundwater. 41(01):139–141.
- Dong SC, Samsonov S, Yin HW, Ye SJ, Cao YR. 2014. Time-series analysis of subsidence associated with rapid urbanization in Shanghai, China measured with SBAS-InSAR method. Environ Earth Sci. 72(3):677–691.
- Dong J, Zhang L, Tang M, Liao M, Xu Q, Gong J, Ao M. 2018. Mapping landslide surface displacements with time series SAR interferometry by combining persistent and distributed scatterers: a case study of Jiaju Landslide in Danba, China. Remote Sens Environ. 205:180–198.
- Ferretti A, Fumagalli A, Novali F, Prati C, Rocca F, Rucci A. 2011. A new algorithm for processing interferometric data-stacks: squeesar. IEEE Trans Geosci Remote Sens. 49(9):3460–3470.
- Ferretti A, Prati C, Rocca F. 2002a. Permanent scatterers in SAR interferometry. IEEE Trans Geosci Remote Sens. 39(1):8–20.
- Ferretti A, Prati C, Rocca F. 2002b. Nonlinear subsidence rate estimation using permanent scatterers in differential SAR interferometry. IEEE Trans Geosci Remote Sens. 38(5):2202–2212.
- Gao Z, He XF, Xiao RY, Yu JJ. 2020. Application analysis of LiCSBAS method for joint estimation of deformation and atmospheric errorin surface monitoring. Geomat Inf Sci Wuhan Univ.:1–13. (in Chinese).
- Garg S, Motagh M, Indu J, Karanam V. 2022. Tracking hidden crisis in India’s capital from space: implications of unsustainable groundwater use. Sci Rep. 12(1):651.
- Hu B, Wang H-S, Sun Y-L, Hou J-G, Liang J., 2014. Long-term land subsidence monitoring of Beijing (China) using the small baseline subset (SBAS) technique. Remote Sens. 6(5):3648–3661.
- Jiang L, Bai L, Zhao Y, Cao G, Wang H, Sun Q., 2018. Combining InSAR and hydraulic head measurements to estimate aquifer parameters and storage variations of confined aquifer system in Cangzhou, North China Plain. Water Resour Res. 54(10):8234–8252.
- Karanam V, Motagh M, Garg S, Jain K. 2021. Multi-sensor remote sensing analysis of coal fire induced land subsidence in Jharia Coalfields, Jharkhand, India. Int J Appl Earth Obs Geoinf. 102:102439.
- Lauknes TR, Zebker HA, Larsen Y.,. 2011. InSAR deformation time series using an -norm small-baseline approach. IEEE Trans Geosci Remote Sens. 49(1):536–546.
- Lee JS, Pottier E. 2012. Polarimetric radar imaging: From basics to applications. Boca Raton, FL: CRC Press.
- Liu Y, Huang H, Dong JH. 2015. Large-area land subsidence monitoring and mechanism research using the small baseline subset interferometric synthetic aperture radar technique over the Yellow River Delta, China. J Appl Remote Sens. 9(1):096019.
- Lubna A, David L, Doreen B, et al. 2018. Long-term peatland condition assessment via surface motion monitoring using the ISBAS DInSAR technique over the flow country, Scotland. Remote Sens. 10(7):1103.
- Luo QL, Perissin D, Lin H, Zhang YZ, Wang W. 2014. Subsidence monitoring of Tianjin suburbs by Terrasar-x persistent scatterers interferometry. IEEE J Sel Top Appl Earth Observ Remote Sens. 7(5):1642–1650.
- Luo Q, Perissin D, Zhang Y, Jia Y., 2014. L- and X-band multi-temporal InSAR analysis of Tianjin subsidence. Remote Sens. 6(9):7933–7951.
- Orhan O. 2021. Monitoring of land subsidence due to excessive groundwater extraction using small baseline subset technique in Konya, Turkey. Environ Monit Assess. 193(4):174.
- Parizzi A, Brcic R. 2011. Adaptive InSAR stack multilooking exploiting amplitude statistics: a comparison between different techniques and practical results. IEEE Geosci Remote Sens Lett. 8(3):441–445.
- Pawluszek-Filipiak K, Borkowski A. 2020. Integration of dInSAR and SBAS techniques to determine mining-related deformations using sentinel-1 data: the case study of Rydutowy mine in Poland. Remote Sens. 12(2):242.
- Pettitt A. 1976. A two-sample Anderson–Darling rank statistic. Biometrika. 63(1):161–168.
- Samiei-Esfahany S, Martins JE, Leijen FV, Hanssen RF. 2016. Phase estimation for distributed scatterers in InSAR stacks using integer least squares estimation. IEEE Trans Geosci Remote Sens. 54(10):5671–5687.
- Selvakumaran S, Plank S, Geiß C, Rossi C, Middleton C. 2018. Remote monitoring to predict bridge scour failure using Interferometric Synthetic Aperture Radar (InSAR) stacking techniques. Int J Appl Earth Obs Geoinf. 73:463–470.
- Sun Q, Jiang L, Jiang M, Lin H, Ma P, Wang H. 2018. Monitoring coastal reclamation subsidence in Hong Kong with distributed scatterer interferometry. Remote Sens. 10(11):1738.
- Wu WH, Li T, Long SC, Zhou ZW. 2019. Coregistration of sentinel‐1 TOPS data for interferometric processing using real-time orbit. Geomat Inf Sci Wuhan Univ. 44(05):745–750.
- Yang K, Yan L, Huang G, Chen C, Wu Z. 2016. Monitoring building deformation with InSAR: experiments and validation. Sensors. 16(12):2182.
- Yao J, Yao X, Liu X. 2022. Landslide detection and mapping based on SBAS-InSAR and PS-InSAR: A case study in Gongjue County, Tibet, China. Remote Sens. 14(19):4728.
- Yastika PE, Shimizu N, Abidin HZ. 2019. Monitoring of long-term land subsidence from 2003 to 2017 in coastal area of Semarang, Indonesia by SBAS DInSAR analyses using Envisat-ASAR, ALOS-PALSAR, and Sentinel-1A SAR data. Adv Space Res. 63(5):1719–1736.
- Yin YP, Zhang ZC, Zhang KJ. 2005. The present situation of land subsidence in China and its prevention and control measures. Chin J Geol Hazard Control. 16(02):1–8.
- Yuan S, He P, Wen YM, etc. 2020. Comprehensive InSAR and strain tensor estimation of coseismic three-dimensional deformation field of the 2016 MW7.0 Kumamoto earthquake. Chin J Geophys. 63(04):1340–1356.
- Zhang L, Liao MS, Dong J, et al. 2018. Early detection of landslides hazards in mountainous areas of West China using time series SAR interferometry-A case study of Danba, Sichuan. Geomat Inf Sci Wuhan Univ. 043(012):2039–2049.
- Zhang Y, Liu Y, Jin M, et al. 2019. Monitoring land subsidence in Wuhan City (China) using the SBAS-InSAR method with Radarsat-2 imagery data. Sensors. 19(3):743.
- Zhang L, Lu Z. 2022. Advances in InSAR imaging and data processing. Remote Sens. 14(17):4307.
- Zhao CY, Liu XJ, Zhang Q, et al. 2019. Research on loess landslide identification, monitoring and failure mode with InSAR technique in Heifangtai, Gansu. Geomat Inf Sci Wuhan Univ. 44(7):996–1007.
- Zheng XX, Qg W, Hou YS, et al. 2002. Some frontier issues on urban land subsidence research. Acta Geol Sin. 3:279–282.
- Zhu JJ, Li ZW, Hu J. 2017. InSAR deformation monitoring methods and research progress. Acta Geod Cartogr Sin. 10:1717–1733.
- Zhu L, Xing X, Zhu Y, Peng W, Yuan Z, Xia Q. 2021. An advanced time-series InSAR approach based on poisson curve for soft clay highway deformation monitoring. IEEE J Sel Top Appl Earth Observations Remote Sens. 14:7682–7698.