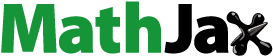
Abstract
This study proposed a rapid approach to determine the fuzzy number to evaluate roof fall risk in the coal mine. In risk assessments by fuzzy theory, the determination of fuzzy numbers using triangular fuzzy numbers (TFN) incorporated into the analytic hierarchy process is a difficult and time-consuming task. A novel approach to determine fuzzy number in TFN was proposed. To reduce occurrence of roof fall accidents, it is necessary to identify the main risk factors in roof fall risk assessments. A nine-score table-type questionnaire was adopted to collected expert judgement, based on which language scales were determined, ranging from 0.1 to 0.9, with increments of 0.1. Each language scale corresponded to the selected value of the experts. Subsequently, a triangular fuzzy complementary judgement matrix and a consistent possibility judgement matrix were constructed. The effectiveness of this proposed method was verified via analysing a case of roof collapse at Chaidar coal mine, Qinghai Province, China. The final ranking results of the risk factors were consistent with those observed in the field.
1. Introduction
Coal is one of the most prominent energy and mineral resources and an indispensable form of energy for production and daily life. Notably, a sufficient supply of coal is crucial for the stability and development of industries and even entire societies (Thorbjornsson et al. Citation2015; Guo et al. Citation2016). However, the coal mining processes need to take coal block from the seam, which must make the coal seam fractured at first (Du et al. Citation2020a; Ding et al. Citation2022a). Thus, both mechanical and hydraulic properties should be measured using various testing technologies, e.g. acoustic emission (Du et al. Citation2020b), hydraulic test (Ding et al. Citation2022b), mechanical testing (Ding et al. Citation2022c), and resistivity testing (Bai et al. Citation2022). Therefore, during coal mining, significant issues include roof falls, gas explosions, and rock bursts, which are related with the mechanical and hydraulic properties (Du et al. Citation2020a, Citation2020b; Zhang et al. Citation2020; Ding et al. Citation2022a, Citation2022b, Citation2022c). In China, roof fall accidents are the most common cause of severe harm in coal mining. Such accidents threaten the safety of personnel and equipment and cause significant economic losses (Li et al. Citation2020a). shows the statistics of the number of roof fall accidents and the death toll in China from 2011 to 2020. As can be seen, more than 130 roof falls and 370 deaths have over the past decade (http://www.mkaq.org/mklw/scaq/). To prevent the occurrence of such roof fall accidents, the risk assessment of mining roof fall accidents is considerably important.
The occurrence of roof fall accidents in coal mining is often influenced by many complicated, fuzzy, and random factors. Geological conditions, mine environments, and hydrogeological conditions have important impacts on the roofs of coal mines and can increase the risk of roof falls (Düzgün Citation2005; Wang et al. Citation2018). Notably, supporting structures play an important role in maintaining roof stability and reducing the risk of roof falls (Palei and Das Citation2008; Prusek et al. Citation2016; Chi et al. Citation2019). Many studies have predicted the caving rate of coal mine roofs through the coal mine roof rating (CMRR) and primary roof support (SRSUP) (Mark and Molinda Citation2005; Ghasemi and Ataei Citation2013). There have been study to predict the risk of coal mines by using time series modelling (Mohseni and Ataei Citation2016). These methods are effective but neglect the management problems pertaining to human and equipment factors, resulting in incomplete factor identification. Important factors associated with the safety management of coal mining are often neglected, resulting in serious consequences. Therefore, effectively identifying these risk factors is considerably important for risk assessments. Risk assessments are helpful for risk management and decision making (Tahernejad et al. Citation2012; Hosseini et al. Citation2017; Nikkhah et al. Citation2019; Norouzi Masir et al. Citation2021). Previous studies have proposed a variety of risk assessment methods such as analytic hierarchy process (AHP) (Lyu et al. Citation2020), fault tree (Jahanbani et al. Citation2018), and fuzzy mathematics (Liu et al. Citation2014, Citation2015). Certain scholars have proposed probability and mathematical statistical methods to evaluate the risk of coal mining roofs (Düzgün Citation2005; Prusek et al. Citation2016). This approach mainly uses the probability and consequence of the risk occurrence to evaluate roof risk levels, which plays a certain role; however, it is difficult to determine the important risk factors. Some scholars have proposed a semi-quantitative technology for risk assessment. The method is applied to evaluate various possible scenarios and corresponding risks of retreat mining (Ghasemi et al. Citation2012). Furthermore, researchers have also used artificial neural networks to predict the risk of coal roof fall accidents (Li et al. Citation2020b; Małkowski and Juszyński Citation2021). This method is efficient but lacks expert judgements.
To search literatures, VOSviewer was used to analyze the titles and abstracts of more than 9000 literatures in web of science (WOS), in which keywords of coal mine roof fall, AHP, risk assessment, triangular fuzzy numbers were used, and then, screened 300 terms. shows VOSviewer term map visualization for the related literatures. It can be seen that the fuzzy analytic hierarchy process (FAHP) is widely used in risk assessments in mining (Mikaeil et al. Citation2009; Zare Naghadehi et al. Citation2009; Jafari et al. Citation2011; Ameri Siahuei et al. Citation2021). It addresses the fuzziness and inaccuracies in the decision-making process by combining fuzzy numbers with the AHP (Hamidi et al. Citation2010; Lyu et al. Citation2020). For example, in the risk assessment of underground engineering, Nezarat et al. (Citation2015) considered this probability and influencing factor and used the FAHP to determine risk levels. In many methods, the determination of fuzzy numbers and the satisfaction of consistency requirements are always problematic. Li et al. (Citation2013) used the improved AHP to improve the consistency of the CM and effectively identify risk factors. Lyu et al. (Citation2020) proposed a simple questionnaire with expert opinions and determined fuzzy numbers under the requirement of consistency; this is a highly effective method for multifactor evaluations.
This article aims to (i) proposes a triangular fuzzy complementary AHP (TFC-AHP) to identify evaluate the most risk factors of coal mining roof fall accidents and (ii) apply the improved approach to evaluate a case of roof collapse at Chaidar coal mine, Qinghai Province, China. Based on a simple questionnaire combined with expert experience, the results of the proposed TFC-AHP method and the existing FAHP method (TFN-AHP) are analysed. In section 2, this part introduces the method of determining triangular fuzzy numbers based on a table-type questionnaire and calculating the weight of each factor combined with AHP. In section 3, the proposed TFC-AHP method is applied to the risk analysis of the roof fall accident in Chaidar coal mine of Qinghai Province. In section 4, the weight results calculated by the proposed TFC-AHP method and an existing FAHP method (TFN-AHP) are compared and analyzed with the actual situation of the site; it shows that TFC-AHP method is more suitable for the actual situation. Finally, in section 5, this section concludes the article.
2. Methodology
2.1. Framework of risk assessment
shows the framework of the proposed risk assessment model. It consists of six steps: (i) data collection, (ii) establishment of assessment index system and generation of datasets, (iii) conduction experts’ questionnaire, (iv) determination of TFC number, (v) conduct TFC-AHP method, (vi) results and discussion. The steps (i) and (ii) will be introduced in case study subsections 3.1 and 3.2, respectively; steps (iii) to (v) will be introduced in subsections 2.2 to 2.4, some numerical results of TFC number will be presented in subsection 3.3, and the detailed weight factor numbers will be presented in subsection 3.4; step (vi) will be presented in section 4.
2.2. Nine-score questionnaire
According to the original triangular fuzzy number AHP (TFN-AHP), a questionnaire was developed to collect the opinions of different experts. The traditional questionnaire requires a significant amount of time for the evaluation process, and it is difficult to understand even for experienced experts; hence, it is difficult to meet the consistency requirements of the matrix. Therefore, a table-type nine-score questionnaire (Lyu et al. Citation2020) was adopted, in which nine points indicate the impact of a factor on the risk; a value of 1 denotes equally important, whereas a value of 9 indicates extremely important. Essentially, the higher the score, the higher is the priority of the factor. It is difficult to determine the fuzzy number using the ratio between the two factors when there are limited comparative factors and there exists a difference between the scores. For example, the score of Factor A is 5–9, the score of Factor B is 6–9, and the score of Factor C is 6–9. The ratios of any two factors were all less than 2. Thus, the calculated weight of each factor was the same, leading to inaccurate evaluation results. Therefore, this work suggests that the difference between two factors be used to determine the fuzzy number; this difference is represented by Δ, which will be described in details in the following section.
2.3. Determination of TFN
TFN are common in the field of risk assessments and can be expressed as N = (g, h, m) (g ≤ h ≤ m), where g, h, and m represent the values of the lowest risk possibility, medial risk possibility, and highest risk possibility, respectively. The membership function of fuzzy numbers can be expressed as in EquationEq. (1)(1)
(1) ; for 0 ≤ μ (x|N) ≤ 1, which represents the weight of the risk factors (Zadeh Citation1965).
(1)
(1)
For any two TFN, the common simple operation expressions are shown in EquationEqs. (2)–(5). The following operations were used in this study to solve the evaluation weights of the indicators using the triangular fuzzy number judgement matrix (Zadeh Citation1965).
N1⨁ N2 = (g1+g2, h1+h2, m1+m2) (2)
N1⨂ N2 = (g1×g2, h1×h2, m1×m2) (3)
λ ⨂ N= (λ×g2, λ×h2, λ×m2) (4)
(5)
(5)
where λ is an arbitrary constant, N1 = (g1, h1, m1), and N2 = (g2, h2, m2).
In a previous study, to determine the TFN of each factor, Xu (Citation1999, Citation2004) proposed a complementary fuzzy scale with values from 0.1 to 0.9, at increments of 0.1. In the complementary fuzzy scale, a value of 0.1 indicates that Factor A is more important than Factor B, whereas a value of 0.9 denotes that Factor B is more important than Factor A. Thus, we established a relationship between the score difference Δ and the complementary fuzzy scale value. shows the corresponding evaluation language between complementary fuzzy scale value and score difference Δ. A value of 0.5 indicates equal important between two factors; this corresponds to Δ = 0. The corresponding results between the complementary fuzzy scale value and the score difference Δ are tabulated in .
Table 1. Corresponding evaluation language between complementary fuzzy scale.
The steps to determine the fuzzy number are as follows:
The scoring results of each expert were counted, and the average score of each factor and the difference between the two factors were calculated based on each expert’s opinion.
According to the range of values and the corresponding range of the complementary fuzzy scale, the trend of the fuzzy number was determined via a comparison with the average scoring value.
When multiple experts evaluated the same two factors, if the difference interval was –2 to 2, the two factors were deemed equally important. lists the TFN for the relative importance.
Table 2. TFN for relative importance.
2.4. TFC-AHP
According to the AHP (Saaty Citation1977), the factors related to decision making are decomposed into the target, source, and element layers. Indexes at the same level are compared in pairs, and the degree of comparison is quantified to establish a judgement matrix. When the consistency requirement is satisfied, the matrix is solved, and the weight vector of each index can be obtained. TFC-AHP uses triangular fuzzy numbers to express the relative importance between indexes. The fuzzy and quantitative methods for qualitative indexes were used to calculate the single ranking and total ranking as a systematic method for the optimisation decision of the target scheme. The establishment of a reasonable AHP is essential for the rationality of the final decision result, which corresponds to the process of identifying each factor and decomposing the target factor into the source and element layer factors.
The construction of the TFC judgement matrix Fn×n and the calculation of EquationEq. (6)(6)
(6) can be expressed as follows:
(6)
(6)
where (gij, hij, mij) = (1-gji, 1-hji, 1-mji), gij+ gji =1, hij+ hji =1, mij+ mji =1, and gij ≥hij ≥mij >0, i, j∈N.
To satisfy the consistency requirement of the TFC judgement matrix using the consistency probability matrix (Qin Citation2007; Meng et al. Citation2021), the initial fuzzy degrees Pi = (gi, hi, mi) and Pj = (gj, hj, mj) were compared. Thereafter, the possible degree Sij of the initial fuzzy degree Pi ≥ Pj can be calculated using EquationEqs. (7)(7)
(7) and Equation(8)
(8)
(8) . Experts prefer neutrality; thus, the coefficient was considered as 0.5.
(7)
(7)
where i, j = 1,2,···n.
(8)
(8)
Based on the aforementioned results, the fuzzy consistency possibility matrix R can be obtained using EquationEqs. (9)–(11):
(9)
(9)
(10)
(10)
(11)
(11)
Thus, the weights of each evaluation indicator can be expressed as in EquationEqs. (12)(12)
(12) and Equation(13)
(13)
(13) .
(12)
(12)
(13)
(13)
3. Case study
3.1. Project background
The Qinghai Chaidar coal mine is located in Gangcha County, Haibei Prefecture, Qinghai Province, with geographical coordinates of 37°36′ N and 100°28′ E. shows a map of the coal mine on the southern side of the Qilian Mountains. Its elevation is 3700–3900 m, with an average height of 3880 m (see ) (data obtained from Geospatial Data Cloud, http://www.gscloud.cn/search). The average height of the coal mine site is 3720 m (Wang et al. Citation2018). This field is dominated by alpine landforms and a discontinuous distribution of frozen island soil. The coal seam of the Chaidar coal mine is steeply inclined, and the coal-bearing stratum rises to the north and falls to the south. The roof has low strength, features cracks, and can collapses easily. Therefore, it is necessary to evaluate the risk levels of roof fall accidents during coal mining and formulate emergency plans according to the assessment results to reduce this risk.
Figure 4. Map of Qinghai Province: (a) satellite map of Qinghai Province and (b) elevation map of eight counties in Haibei Prefecture.

On 14 August 2021 the Chaidar coal mine of Xihai coal company in Gangcha County, Haibei Prefecture, Qinghai Province, suffered a roof fall accident. The accident killed 20 people and resulted in a direct economic loss of 53.9102 million yuan (US$8.0272 million) (Guo Citation2022). Before the incident, the mine was an open pit with a depth of 160 m. At the time of the incident, it was backfilled to 80 m, and continuous water seepage occurred around the pit during the rainy season. There was also no rock stratum in the backfilling part, which was mainly composed of sandy soil. Furthermore, the geological conditions of the mine were complex and extremely unstable (Wang et al. Citation2022). The accident was caused by top coal pumping, and a large amount of the top coal, slag, and water mixture quickly collapsed into the working face and transportation channel in the form of debris flow. To avoid future disasters, a fast on-site risk analysis should be conducted.
3.2. Establishment of assessment index system
According to many research results, there are three main risk sources affecting the occurrence of roof fall accidents: (i) the natural environment, (ii) mine excavation operations, and (iii) safety management. These sources are considered as three factors in the source layer for the risk assessment of roof fall accidents in coal mines. Each risk source has a corresponding risk index, based on which the element layer is determined. shows the established hierarchy analysis structure, including the target, source, and element layers.
3.2.1. Natural environment (B)
The quality of a coal mine roof is directly related to the natural environment and mainly includes four influencing factors: (1) stratum lithology (B1), (2) geological structure (B2), (3) climate and precipitation (B3), and (4) groundwater distribution (B4).
Stratum lithology (B1): Formation lithology includes the composition of rock forming strata, as well as the physical and mechanical properties, which directly affect the quality of the roof, stability of the roof, and ore pressure borne by the roof in mining engineering. The rock is a geological body formed after complicated geological changes. The stress field of a rock mass is redistributed following excavation disturbances. The quality of the rock mass in the excavation face directly affects the engineering design, roadway layout, and stability control.
Geological structure (B2): When a coal mining face encounters folds, joints, and faults, it is usually accompanied by breakage of the roof of the working face, and the geological structure tends to feature different degrees of influence on safety during coal mining.
Climate and precipitation (B3): Climate change affects the thawing settlement of seasonally frozen soil, while precipitation alters the soil water content and increases the volume of mine water, thereby affecting the stability of the roof.
Groundwater distribution (B4). The distribution of groundwater affects the goaf water content. Simultaneously, excavation processes may destroy the aquifer, which, in turn, affects the safe production in coal mines, including the stability of the roof. There is no water drainage system in the Chaidar well field; furthermore, only a few branches of the vertical watershed feature short flows, which tend to freeze in the winter, without a perennial water flow.
3.2.2. Excavation operation (C)
During mine excavation, many factors directly affect the stability of the roof, and the risk sources include three main factors: (1) support structure (C1), (2) excavation progress (C2), and (3) drainage facilities (C3).
Support structure (C1): In coal mine production activities, the support structure is an essential problem, as its construction quality directly affects the production safety of the coal mine. The support structure used to support the roof can increase the stability of the roof and transfer loads to cope with complex geological conditions; thus, it is an important component of the coal mining process.
Excavation progress (C2): This refers to the annual number of coal mines. Excessively high speeds can cause instabilities in the surrounding rock and delay support replacement, which can easily lead to roof collapse accidents.
Drainage facilities (C3): Appropriate drainage facilities affect the soil water content, which, in turn, influences the mine pressure load and roof stability. Water entering the mine is collectively referred to as mineral water; this water mainly originates from surface water, underground water, and water gushing from mining projects.
3.2.3. Safety management (D)
The operation processes in coal mines are also significantly difficult. To avoid the occurrence of roof accidents, attention should be paid to coal mine safety management, which mainly includes three risk factors: (1) equipment safety maintenance (D1), (2) personnel safety awareness (D2), and (3) monitoring and management (D3).
Equipment safety maintenance (D1). The equipment used for mining coal should be maintained regularly to ensure the normal operation of equipment and safe production in coal mines.
Personnel safety awareness (D2): Construction personnel should possess a comprehensive understanding of mining situations, have corresponding safety consciousness, and actively prevent roof-falling accidents.
Monitoring and management (D3): Monitoring technology is an effective means for roof collapse accident prevention. By monitoring of the support system, the status of the support system can be understood accurately, enabling timely adjustments.
3.3. Establishment of fuzzy judgement matrix
We developed a nine-score questionnaire for this case and invited experts to score each influencing factor. Since the invited experts are all from China, the questionnaire was written in Chinese.We invited seven experts to evaluate the roof fall risk of the Chaidar coal mine in Qinghai Province. shows the research areas and work experience of the expert group. lists the expert scoring results. According to the original triangular fuzzy AHP, the matrix and weight of the source layer can be obtained (). Similarly, the matrix and weight of the element layer under Source B can also be obtained (see ).
Table 3. Research areas and work experience of the expert group.
Table 4. Statistics of expert scoring results.
Table 5. Matrix and weight of source layer.
Table 6. Matrix and weight of element layer under Source B.
As shown in , the expert scores ranged from 5 to 9. According to this rule, when the evaluation factor number of each layer is less than 5, it is inappropriate to adopt a scoring ratio between two factors to determine the fuzzy number. Thus, some of the calculated weights became equal (see and ). Therefore, there is no method to identify the important factors. To solve this problem, we propose another method to determine the fuzzy number using the difference between two factors (see in subsection 2.1). The detailed procedure for establishing the fuzzy judgement matrix is as follows.
Considering the source layer as an example, for Δ(B – C) = −5–2, the corresponding number of complementary fuzzy scale is 0.2 to 0.6, with the mid-value of 0.4. However, the average difference values were negative, and all the five differences were negative. Therefore, the relative importance of Factor C is greater than that of Factor B, and the mid-value of the triangular fuzzy number is identified as 0.3, such that the triangular fuzzy number becomes (0.2, 0.3, 0.4). For Δ(B – D) = −3–1, the corresponding scale of languages is 0.3 to 0.6, and the mid-value is 0.5. The average difference was negative, and the four difference values were negative. Therefore, the relative importance of Factor D is greater than that of Factor B, and the mid-value of the triangular fuzzy number is 0.4. Thus, the TFN are (0.3, 0.4, 0.5). Similarly, the trigonometric fuzzy numbers of the other elements can be obtained. lists the TFC judgement matrices of the source layer.
Table 7. Triangular fuzzy complementary judgement matrix of source layer.
3.4. Weight vector from triangular fuzzy AHP
After the triangle fuzzy judgement matrix is determined, the initial fuzzy degree Pi of the triangle fuzzy matrix in the source layer can be obtained using EquationEq. (7)(7)
(7) , and the calculation results are as follows:
P1 = (0.196, 0.267, 0.359);
P2 = (0.314, 0.4, 0.513);
P3 = (0.255, 0.333, 0.436).
According to the calculated initial fuzzy degree Pi, the fuzzy consistency possibility matrix R can be calculated according to EquationEqs. (8)–(11).
After the fuzzy consistency possibility matrix is obtained, the weights of evaluation factors B–D can be obtained according to EquationEqs. (12)(12)
(12) and Equation(13)
(13)
(13) , as shown below:
In accordance with the same method, the weight of each factor at the element layer was calculated using the TFC-AHP method, and the comprehensive weight of each indicator was finally obtained.
4. Results and discussion
4.1. Results
shows the single and comprehensive weights of each indicator using the TFC-AHP method. The weight results show that mine excavation operation (C) and safety management (D) are more important than the natural environment (B). Supporting structures (C1), personnel safety training (D2), and drainage facilities (C3) are the three most important factors, followed by excavation progress (C2), equipment safety maintenance (D1), and monitoring management (D3). The stratum lithology (B1), geological structure (B2), climate and precipitation (B3), and groundwater distribution (B4) have relatively small weights.
Table 8. Single weight and comprehensive weight of each indicator.
Ministry of Emergency Management of the People’s Republic of China (https://www.mem.gov.cn/) published the final investigation report of this coal mining roof fall accident. shows the causes of the Chaidar coal mine roof fall accident. The experts summarised that the main causes of the accident included eight points of five aspects: (1) supporting structure, (2) drainage facilities, (3) management, (4) personnel safety awareness at construction, and (5) unreasonable operation. Nevertheless, the disaster factors were not identified effectively. The natural environment also has an impact; for example, in the rainy season, continuous water seepage occurs around the pit. This area lies in the permafrost layer, and thawing and sedimentation occur during the summer. Coal seams are powdery and can easily form slime. Thus, protective measures, especially for the supporting structures and drainage facilities, are extremely important. The rescue difficulty was the same. It is necessary to implement a large number of drainage facilities at the rescue site, drill holes in the ground, and inject cement and waterglass simultaneously (see ). The formation of a stable cement layer on top of the support of the working face (Tian Citation2021) results in desilting, thereby reducing the risk to safety and preventing the occurrence of secondary disasters.
Figure 6. Drainage facilities at the rescue site: (a) the disaster site and (b) drainage pipe (recreated based on Qi Citation2021).

Table 9. Cause and key factors of Chaidar coal mine roof fall accident.
As shown in , the identified results from the proposed method indicate that the sorting results are in accordance with the field situation, which, in turn, suggests that the method can be used for the risk assessment of roof collapse accidents in coal mining. Therefore, for the Chaidar coal mine, more attention should be paid to whether the replacement of the support structure is timely and whether the drainage facilities are appropriate.
Moreover, the safety training of construction personnel is critical for ensuring safety in coal mining. In addition, the company in charge of the coal mine failed to perform safety management duties and continued with illegal production, even though the relevant certificates and licences were suspended. Local governments should, therefore, strengthen their supervision.
4.2. Discussion
The nine-score questionnaire proposed by Lyu et al. (Citation2020) is characterised by a faster and more convenient collection of expert opinions. Because the expert opinion results are concentrated between values of 5–9, it is difficult to use the score ratio between two factors to determine the fuzzy number in the TFN-AHP method. The TFC-AHP method uses the difference to determine a fuzzy number. To compare the two methods, based on the expert opinion results of the same questionnaire, the weight of each factor for the TFN-AHP and TFC-AHP methods are shown in . In TFN-AHP method, the calculated weights of excavation operation (C) and management (D) in the source layer are the same, so it is difficult to distinguish which is more important to the target layer. In addition, in the element layer, the calculated weights of the stratum lithology (B1), geological structure (B2), climate and precipitation (B3), and groundwater distribution (B4) are the same, so it is impossible to distinguish their respective importance to B, and the same is true for maintenance (D1), personnel safety training (D2), and monitoring management (D3), so it is difficult to evaluate the risk factors, which shows that the TFN-AHP method has defects. In TFC-AHP method, it can be seen that the calculated weights of each element in different layers are different, so it can be used for the next step of evaluation shows the comprehensive weight of factors for the TFN-AHP and TFC-AHP methods. The supporting structure and drainage facilities are the two most important factors for both methods, which is consistent with the field situation. However, in the TFN-AHP method, the relative importance of each factor under the same risk source is not highlighted. For example, the weights of each indicator under risk sources B and D are equal, indicating that the method lacks sensitivity in terms of identifying factors. The method proposed herein solves this problem by using the difference to represent the relative importance of each factor and construct a TFC matrix. The calculated results are not only consistent with the field situation but also reflect the different importance levels of each factor. In addition, the weight of the highest risk factor identified by the TFC-AHP method is 0.19, which is larger than the value of 0.15 calculated via the TFN-AHP method, indicating that this method has higher sensitivity in terms of identifying high-risk factors.
Figure 7. Weight of each factor for the TFN-AHP and TFC-AHP methods: (a) TFN-AHP weight and (b) TFC-AHP weight.

Figure 8. Comprehensive weight of factors for the TFN-AHP and TFC-AHP methods: (a) TFN-AHP result and (b) TFC-AHP result.

The results of both methods reveal that protection measures and management systems are critical, consistent with the findings of most previous studies. It is also worth mentioning that drainage facilities are often neglected during roof fall disasters. The area considered in this study is complicated and comprises frozen soil. With changes in temperature, the strength and moisture content of the soil also vary, which affects the quality of the roof. Therefore, drainage facilities are crucial. In this study, the sensitivity of this factor was high, which should be emphasised in future research. However, the TFC-AHP method relies on the experts’ experience to determine fuzzy numbers, making it highly subjective. Thus, there was some uncertainty in the results. Although this method has limitations, it can provide a reference for formulating measures to reduce risks and provide guidance for further research using relevant parameters.
5. Conclusions
This article proposed a method to determine the fuzzy numbers in risk assessments using the fuzzy AHP to eliminate the complexity and fuzziness of the influencing factors for coal mine roof fall risk. Based on the results, the following conclusions can be drawn:
This study identified the main influencing factors for the coal mine roof fall risk. A nine-score questionnaire was used to collect expert opinions. To avoid a deficit in the expert opinion scores, ranging from 5 to 9, the expert score results were converted into a TFC matrix using the difference between two factors based on the same questionnaire results. This weight calculation was consistent with the field situation.
The TFC-AHP was used to construct the source layer, including three main risk sources, with a total of ten risk factors. We invited relevant experts to assign evaluation index scores, and the triangular fuzzy theory was used to calculate the weight of each factor. The comprehensive weights of the evaluation factors determine the degree of influence of each risk factor on the roof fall accidents during coal mining. The entire process alleviates the uncertainty associated with expert scoring.
The proposed method was applied for the risk assessment of roof falls during coal mining. The most important influencing factors were identified: (i) support structure, (ii) personnel safety training, and (iii) drainage facilities. The assessed results were compared with the field situation, revealing that the proposed method has high efficiency and reliable.
Simultaneously, the results also show that, in frozen soil mining areas, although the soil changes with the temperature and rainfall, controllable factors should be emphasised over uncontrollable factors. For example, the sensitivity of the supporting structure is five times that of groundwater distribution and four times that of climate and precipitation; furthermore, the sensitivity of drainage facilities and personnel safety training is more than three times that of climate, precipitation, and groundwater distribution. Furthermore, drainage facilities in frozen soil are often neglected during roof fall disasters.
Data availability statement
Supplementary material associated with this article can be found which the online link of the questionnaire is shown below: https://www.wjx.cn/vm/rvVbjpf.aspx.
Disclosure statement
No potential conflict of interest was reported by the authors.
Additional information
Funding
References
- Ameri Siahuei MR, Ataei M, Rafiee R, Sereshki F. 2021. Assessment and management of safety risks through hierarchical analysis in fuzzy sets type 1 and type 2: a case study (faryab chromite underground mines), rudarsko-geološko-naftni zbornik. Mining-Geol-Petroleum Eng Bull. 36(3):1–17.
- Bai GG, Sun Q, Geng JS, Wang SF, Jing XD. 2022. Resistivity of granite and sandstone varies with frequency and water saturation. Geomech Geophys Geoenerg Georesour. 8:198.
- Chi X, Yang K, Fu Q. 2019. Analysis of regenerated roof and instability support control countermeasures in a steeply dipping working face. Energy Explor Exploit. 38:1082–1098.
- Ding ZW, Jia JD, Huang X, Tang QB, Li XF. 2022a. Mechanical properties and energy damage evolution characteristics of coal under cyclic loading and unloading. Rock Mech Rock Eng. 55:4765–4781.
- Ding ZW, Li XF, Huang X, Wang MB, Tang QB, Jia JD. 2022b. Feature extraction, recognition, and classification of acoustic emission waveform signal of coal rock sample under uniaxial compression. Int J Rock Mech Min Sci. 160:105262.
- Ding ZW, Tang QB, Feng RM, Jia JD, Li XF, Huang X, Zhang CF. 2022c. A novel technique for determining transverse permeability of sorptive reservoirs. Geomech Geophys Geo-Energ Geo-Resour. 8(2022):193.
- Du K, Li XF, Tao M, Wang SF. 2020a. Experimental study on acoustic emission (AE) characteristics and crack classification during rock fracture in several basic lab tests. Int J Rock Mech Min Sci. 2020. 133:104411.
- Du K, Yang CZ, Su R, Tao M, Wang SF. 2020b. Failure properties of cubic granite, marble, and sandstone specimens under true triaxial stress. Int J Rock Mech Min Sci. 130:104309.
- Düzgün HSB. 2005. Analysis of roof fall hazards and risk assessment for Zonguldak coal basin underground mines. Int J Coal Geol. 64:104–115.
- Ghasemi E, Ataei M. 2013. Application of fuzzy logic for predicting roof fall rate in coal mines. Neural Comput Appl. 22:S311–S321.
- Ghasemi E, Ataei M, Shahriar K, Sereshki F, Jalali SE, Ramazanzadeh A. 2012. Assessment of roof fall risk during retreat mining in room and pillar coal mines. Int J Rock Mech Min Sci. 54:80–89.
- Guo CK. 2022. Qinghai Chaidar coal mine causes 20 deaths: illegal dredging and roof pumping lead to sand and mud bursting. Chinanews; [accessed 2022 Jun 16]. https://m.chinanews.com/wap/detail/chs/zw/9657227.shtml. Chinese.
- Guo PB, Wang T, Li D, Zhou XJ. 2016. How energy technology innovation affects transition of coal resource-based economy in China. Energy Policy. 92:1–6.
- Hamidi JK, Shahriar K, Rezai B, Rostami J, Bejari H. 2010. Risk assessment based selection of rock TBM for adverse geological conditions using Fuzzy-AHP. Bull Eng Geol Environ. 69(4):523–532.
- Hosseini A, Najafi M, Shojaatlhosseini SA, Rafiee R. 2017. Determination of a suitable extraction equipment in mechanized longwall mining in steeply inclined coal seams using fuzzy analytical hierarchy method (Case study: Hamkar coal mine, Iran). J Min Environ. 8(3):487–499.
- Jafari AR, Ataei M, Jalali SME, Ramazanzadeh A. 2011. Preference of effective factors in suitable selection of microtunnel boring machines (MTBM) by using the fuzzy analytic hierarchy process (FAHP) approach, Revista de Matem’atica. Teorıa y Aplicaciones. 18(2):249–263.
- Jahanbani Z, Ataei M, Sereshki F, Jamshasb F. 2018. Risk assessment of spontaneous combustion coal by using fuzzy fault tree analysis in eastern alborz coal mines. International conference on new horizons in the engineering science, Yildix technical university, March 19; Istanbul, Turkey. https://civilica.com/doc/781178/
- Li FW, Phoon KK, Du XL, Zhang MJ. 2013. improved ahp method and its application in risk identification. J Constr Eng Manag. 139(3):312–320.
- Li W, Ye Y, Wang Q, Wang X, Hu N. 2020a. Fuzzy risk prediction of roof fall and rib spalling: based on FFTA-DFCE and risk matrix methods. Environ Sci Pollut Res Int. 27(8):8535–8547.
- Li XL, Cao ZY, Xu YL. 2020b. Characteristics and trends of coal mine safety development. Energy Sources A-Recovery Util Environ Eff. https://doi.org/10.1080/15567036.2020.1852339.
- Liu DF, Wang D, Wu JC, Wang YK, Wang LC, Zou XQ, Chen YF, Chen X. 2014. A risk assessment method based on RBF artificial neural network - cloud model for urban water hazard. J Intell Fuzzy Syst. 27:2409–2416.
- Liu P, Yang LX, Gao ZY, Li SK, Gao Y. 2015. Fault tree analysis combined with quantitative analysis for high-speed railway accidents. Saf Sci. 79:344–357.
- Lyu HM, Shen SL, Zhou A, Yang J. 2020. Risk assessment of mega-city infrastructures related to land subsidence using improved trapezoidal FAHP. Sci Total Environ. 717(2020):135310.
- Małkowski P, Juszyński D. 2021. Roof fall hazard assessment with the use of artificial neural network. Int J Rock Mech Min Sci. 143:104701.
- Mark C, Molinda GM. 2005. The coal mine roof rating (CMRR) - a decade of experience. Int J Coal Geol. 64(1–2):85–103.
- Meng GW, Ye YC, Wu B, Luo GJ, Zhang X, Zhou ZQ, Sun WT. 2021. Risk assessment of shield tunnel construction in karst strata based on fuzzy analytic hierarchy process and cloud model. Shock Vib. 2021:7237136.
- Mikaeil R, Zare Naghadehi M, Ataei M, Khalokakaie R. 2009. A decision support system using fuzzy analytical hierarchy process (FAHP) and TOPSIS approaches for selection of the optimum underground mining method. Arch Min Sci. 54(2):349–368.
- Mohseni M, Ataei M. 2016. Risk prediction based on time series, Case Study: Tazreh Coal Mine. J Min Environ. 7(1):127–134.
- Nezarat H, Sereshki F, Ataei M. 2015. Ranking of geological risks in mechanized tunneling by using fuzzy analytical hierarchy process (FAHP). Tunn Undergr Space Technol. 50:358–364.
- Nikkhah M, Ghasvareh MA, Farzaneh Bahalgardi N. 2019. Risk management in urban tunnels using methods of game theory and multi-criteria decision-making. J Min Environ. 10(3):597–611.
- Norouzi Masir R, Ataei M, Mottahedi A. 2021. Risk assessment of flyrock in surface mines using a FFTA-MCDM combination. J Min Environ. 12(1):191–203.
- Palei SK, Das SK. 2008. Sensitivity analysis of support safety factor for predicting the effects of contributing parameters on roof falls in underground coal mines. Int J Coal Geol. 75:241–247.
- Prusek S, Rajwa S, Wrana A, Krzemień A. 2016. Assessment of roof fall risk in longwall coal mines. Int J Min Reclam Environ. 31:558–574.
- Qi ZB. 2021. Qinghai Chaidar coal mine accident rescue: the drilling equipment has confirmed the drilling position. Chinanews. [accessed 2022 Jun 16]. https://www.chinanews.com.cn/tp/hd2011/2021/08-17/996824.shtml. Chinese.
- Qin JY. 2007. Analyzing for the quantity of the consistency judgment matrixes with four fuzzy scales. Guangxi Sci. 14(4):367–370.
- Saaty TL. 1977. A scaling method for priorities in hierarchical structures. J Math Psychol. 15(3):234–281.
- Tahernejad MM, Ataei M, Khalokakaei R. 2012. Selection of the best strategy for Iran’s quarries: SWOT-FAHP method. J Min Environ. 3(1):1–13.
- Thorbjornsson A, Wachtmeister H, Wang JL, Hook M. 2015. Carbon capture and coal consumption: implications of energy penalties and large-scale deployment. Energy Strategy Rev. 7:18–28.
- Tian BQ. 2021. 22 drilling rigs will be arranged at the accident site of Chaidar coal mine in Qinghai Province for rescue. Chinanews. [accessed 2022 Jun 16.] https://www.chinanews.com.cn/sh/2021/08-17/9545592.shtml. Chinese.
- Wang HW, Xue S, Jiang YD, Deng DX, Shi SZ, Zhang DQ. 2018. Field investigation of a roof fall accident and large roadway deformation under geologically complex conditions in an underground coal mine. Rock Mech Rock Eng. 51:1863–1883.
- Wang YJ, Guan QC, Duan SR. 2018. Investigation and analysis on the geological, environment problems of coal mine, Chaidaer. Miner Explor. 9(5):1031–1036.
- Wang YJ, Zhao LS, Xu YS. 2022. Analysis of characteristics of roof fall collapse of coal mine in Qinghai Province, China. Appl Sci. 12(3):1184.
- Xu ZS. 1999. Study on the relation between two classes of scales in AHP. Syst Eng—Theory & Practice. 19(7):97–101.
- Xu ZS. 2004. Goal programming models for obtaining the priority vector of incomplete fuzzy preference relation. Int J Approximate Reason. 36(3):261–270.
- Zadeh LA. 1965. Fuzzy sets, Inf Control. 8(3):338–353.
- Zare Naghadehi M, Mikaeil R, Ataei M. 2009. The application of fuzzy analytic hierarchy process (FAHP) approach to selection of optimum underground mining method for Jajarm Bauxite Mine, Iran. Expert Syst Appl. 36(4):8218–8226.
- Zhang JS, Fu J, Hao HY, Fu G, Nie FC, Zhang WY. 2020. Root causes of coal mine accidents: characteristics of safety culture deficiencies based on accident statistics. Process Saf Environ Prot. 136:78–91.