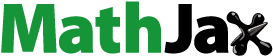
Abstract
Landslides are destructive to property, infrastructure and people in potential landslide zones. Identifying potential landslides is an important step in landslide preparedness and will help develop sustainable landslide risk management. Interferometric synthetic aperture radar (InSAR) and landslide susceptibility assessment (LSA) have poor reliability in individually identifying potential landslide zones. This study proposes a threshold model for identifying potential landslide zones that fuses the InSAR two-dimensional (vertical direction and east-west direction) deformation rates and LSA results. The deformation rate threshold for this threshold model is |DU| or |DE|>10 mm/year (DU and DE are the vertical and the east-west deformation rates, respectively), with threshold levels of LSA set to high and very high susceptibility. The criterion of potential landslide zones is ((|DU| or |DE|>10 mm/year) ∩ (high or very high susceptibility of LSA)), and points with similar deformation and susceptibility are clustered by the K-means algorithm, and the potential landslide zones are obtained by elimination, smooth and speckle removal operations. The results showed that the InSAR two-dimensional deformation rates DU and DE were −32.71 − 12.72 mm/year and −14.88 − 24.81 mm/year, respectively, during 2015–2020 in Lanzhou city. The LSA showed that very low, low, medium, high, and very high susceptibility accounted for 55.36%, 10.54%, 21.37%, 9.63%, 3.1% of the total area, respectively. Using the proposed threshold model, 117 potential landslide zones were identified in Lanzhou. The overlap rate between potential landslide zones and the landslide inventory was 40.17%, indicating that about 40% of the potential landslide zones overlapped with the landslide inventory and that about 60% were new potential landslide zones in Lanzhou. The feasibility of the threshold model in identifying potential landslides was confirmed by field research and time-series InSAR analysis on typical areas (L1, L2, L3, and L4), which had large deformation variables and landslide features. The proposed method can quickly determine the spatial location of potential landslides, providing targeting data for landslide field investigations, technical support for rapid early landslide identification, and data support for landslide management and prevention in Lanzhou.
1. Introduction
A landslide is the phenomenon in which the material forming a slope (such as rock, sediment, artificial fill, or a combination of these) is moved downward and outward by the action of gravity and other influencing factors (Varnes Citation1978). Landslides can not only damage human life, property, and buildings but also cause secondary disasters such as weirs (Xu et al. Citation2008; Huang and Fan Citation2013; Dai et al. Citation2020). Between 1995 and 2014, a total of 3876 landslides killed 163658 people and injured 11689 people worldwide (Haque et al. Citation2019). Landslides are caused by a variety of factors, and there is a great deal of uncertainty about when and where they will occur, thus, it is important to accurately identify where landslides are likely to occur (Huang Citation2004; Novellino et al. Citation2021).
Lanzhou is located at the intersection of the Qinghai Tibet Plateau, Loess Plateau, and Inner Mongolia Plateau, with complex geological conditions, intense human engineering activities, and damage to the ecological environment, resulting in unstable slopes and increasing the likelihood of landslides. According to an official survey, the Linxia-Yongjing-Lanzhou section of the Yellow River was one of the main landslide distribution areas (The People’s Government of Gansu Province, 2017). In recent years, many scholars have conducted research on landslides in Lanzhou city (Lyu et al. Citation2018; Torizin et al. Citation2018; He et al. Citation2020; Shu et al. Citation2021). These studies were mainly based on landslide susceptibility evaluation, but they lacked related research on potential landslide identification. The landslides in Lanzhou moved as heavy rains fell, posing serious threats to the safety of local people’s lives, property, and vital infrastructure. Therefore, it is urgent to identify potential landslide zones in Lanzhou.
Small baseline subset interferometric synthetic aperture radar (SBAS-InSAR) (Berardino et al. Citation2002) overcomes the disadvantages of traditional deformation monitoring methods. The traditional methods are difficult and costly to deploy monitoring points and cannot achieve large-scale landslide monitoring (Berardino et al. Citation2002). SBAS-InSAR has been widely used to monitor ground surface deformation caused by earthquakes (Grandin et al. Citation2017), landslides (Dai et al. Citation2016, Citation2019), ground subsidence (Wang Citation2020; He et al. Citation2021a, Citation2021b), and volcanic movements (Lee and Lee Citation2018). SBAS-InSAR technology is suited for tracking small terrestrial deformations over large areas, which can be used to accurately identify the location and extent of early landslide deformation. Deformation traces are being used for the early identification of potential landslide zones (Tofani et al. Citation2013). Dai et al. (Citation2022) used the SBAS-InSAR to obtain a wide-area potential landslide early identification the whole of Mao County, a mountainous area in western Sichuan (China), with a total of 41 potential landslides successfully detected. Zhu et al. (Citation2021) used SBAS-InSAR to study the pre- and post-failure geomorphic changes and spatiotemporal evolution of loess landslide. Tian et al. (Citation2022) studied the deformation and stability of loess landslides in the Yellow River Basin using SBAS-InSAR. SBAS-InSAR is used to analyse and conduct long-term monitoring of key landslides, which is an effective means of identifying deforming landslides, but it is not easy to directly identify deformed landslides.
Landslide susceptibility assessment (LSA) is a common method of landslide spatial prediction. Here, we used landslide inventory and landslide influencing factors to construct a model for predicting the spatial distribution of potential landslides . There are four main methods of LSA: physics-based models, viewpoint-driven models, statistical models, and machine learning models (Chang et al. Citation2019; Li et al. Citation2019; Pham et al. Citation2019; Thomas et al. Citation2021). Machine learning is a solution for addressing big-data spatial analytics in which the extent of the theoretical knowledge of a problem is incomplete and when statistical pre-assumptions are unreliable or unknown (Dou et al. Citation2020). It is an ideal technique for solving non-linear geo-environmental issues due to its robustness. Machine learning methods can quickly predict landslide susceptibility and have been widely used (He et al. Citation2020; Merghadi et al. Citation2020; Pham et al. Citation2020; Rodrigues et al. Citation2021). Multilayer perceptron (MLP) is widely used in LSA due to its parallel processing, good fault tolerance, and very strong adaptive capability. MLP can be used to the construct accurate landslide susceptibility maps (Zare et al. Citation2013; Yi et al. Citation2020; Tran et al. Citation2021). However, reliable spatial location identification of potential landslide zones is still a challenging task.
The combination of deformation monitoring and LSA can improve the quality of results (Zhao et al. Citation2019), and most studies of this kind tend to update the landslide susceptibility map by integrating InSAR data into the LSA process (Huang et al. 2020). We combined the two methods in an attempt to rapidly identify potential landslide zones. Based on the results of InSAR and MLP-based LSA, a threshold model is proposed to integrate landslide results from the two methods to achieve more accurate potential landslide zone extraction. The main objectives were to: (1)obtain the two-dimensional deformation rates of the two orbitals based on Sentinel1-A descending and ascending tracks images using SBAS-InSAR; (2)obtain the LSA based on landslide influence factors using the MLP model; and (3)construct the threshold model based on the two-dimensional deformation rates and the LSA levels, identifying the potential landslide zones of Lanzhou. Field validation and time-series InSAR vertical results were used to verify the feasibility of the new model. The method proposed in this study can provide technical support for rapid early potential landslide identification, and the research results can provide data support for landslide management and prevention in Lanzhou.
2. Methods
We initially monitored the ground surface deformation rates of Lanzhou based on Sentinel1-A descending and ascending tracks images using SBAS-InSAR. The LSA was then predicted using the MLP model. We combined the ground surface deformation rates with the LSA results to construct a threshold model to identify potential landslide zones in Lanzhou. The accuracy of the threshold model was verified based on the field investigation and time series InSAR results. The flow chart is shown in .
2.1. SBAS-InSAR
The SBAS-InSAR technique (Berardino et al. Citation2002) uses the unique phase information of SAR data, which is combined with accurate SAR satellite imaging geometry and orbital ephemeris parameters to invert ground surface deformation. SBAS-InSAR is a representative of the time-series InSAR technique, which analyses SAR data acquired over a long time series in the same area. SBAS-InSAR obtains discrete targets that remain stable over a long period and that can monitor the ground surface deformation information at the millimetric level, offering significant advantages for monitoring slow surface deformation.
The basic principle of SBAS-InSAR is as follows:
In the period from to
scenes of SAR images of the same region are acquired; one of them is selected as the common master image, and then
scenes of interferograms are generated according to the principle of interferometric combination, which satisfies the following relationship.
(1)
(1)
The view interferogram is generated from the master image
and the slave image
The interference phase produced at point
can be expressed as:
(2)
(2)
where
、
(
>
) are the SAR image acquisition times corresponding to the
interferogram;
is the slope-up deformation corresponding to the moment from
to
is the topographic phase error;
is the atmospheric phase error; and
is the noise phase error.
Assuming that the rate of deformation between different interferograms is the cumulative deformation variables from
to
are expressed as:
(3)
(3)
The interferogram of the N SAR was obtained through phase unwrapping, deformation inversion, geocoding, and time-series deformation. The following diagram shows the flow of SBAS-InSAR ().
The first step in this process is connection map generation, in which the super master image of the descending and ascending tracks is automatically selected as the image of May 28, 2019, with the time baseline set to 200 days. The Sentinel-1A image used in this study has at least one scene per month, and the overall pairing situation is good.
The second step is the interference process. Multi-looking with 4 × 1 in the range and the direction of the azimuth. SRTM DEM with 90 m resolution is used as auxiliary data to remove topographic phase. The interferogram is filtered by Goldstein method. The Minimum Cost Flow (MCF) is used at this step to phase unwrapping. After checking the quality of the interferograms, pairs with poor interference quality were removed.
Re-flattening is performed at the third step, which uses GCPs to estimate and remove the remaining phase constants and ramps from the unwrapped phase stack. We selected GCPs based on their deformation being close to zero, confirmed by the interpretation of the unwrapped phase; GCPs point are not in the phase jump region and are evenly distributed (Ghulam et al. Citation2015). Consequently, 38 GCPs were applied to refine orbital errors.
The fourth step, deformation inversion uses a linear model with good robustness to invert surface deformation. The matrix decomposition singular value decomposition (SVD) method is used to transform the interferogram residuals into the temporal SAR images to estimate the average displacement rate and residual topography. During the first inversion, the displacement rate and residual topography are first estimated. In the second inversion, based on the deformation rate obtained in the first inversion, high-pass filtering is carried out in the temporal dimension and low-pass filtering is carried out in the spatial dimension, so as to estimate and remove the atmospheric phase and obtain the final displacement result on the purer time series.
The SBAS-InSAR inversion results are geocoded in the fifth step, which removes the dummy value, and the deformation results are interpolated. SBAS-InSAR derived deformation is in the line-of-sight (LOS) direction. The LOS deformation directions cannot reflect the true deformation, so two-dimensional deformation (vertical and horizontal) can be solved by using the LOS deformation values of the ascending and descending orbits (Ferretti and Prati Citation2000). As Sentinel1-A is a near-polar satellite, it is not sensitive to monitor the north-south direction, so the vertical and east-west direction deformation can be obtained. The solution formula is as follows:
(4)
(4)
In the formula, θ is the incident angle of the satellite, and α is the azimuth angle of the flight direction of the satellite. Subscript A stands for ascending orbit, and subscript D stands for descending orbit.
2.2. LSA based on the MLP model
2.2.1. Construction of the landslide inventory and landslide influencing factors
In this study, we identified 152 loess landslides using Google Earth images combined with fieldwork, with the size of the landslides ranging from 0.001 km2 to 0.52 km2, reaching a total area of 7.18 km2, and we constructed the landslide inventory (). Based on the principles of systematicity, representativeness, hierarchy, and operability (Chen Citation2016), eight landslide influencing factors were selected (). Variance inflation factors and tolerances (VIF&T) were used to test the covariance of the influencing factors, ensuring that the factors were independent of each other. (Hair et al. Citation1998).
Table 1. Data sources of eight landslide influencing factors.
The VIF&T formula is as follows:
(5)
(5)
where
is the variance between the independent variables, the variance inflation factor (VIF) is the ratio of the variance of the regression coefficients when the regression coefficient equation is not correlated with the independent variables, and tolerance (T) is the inverse of VIF. The larger the standard deviation, the higher the degree of multicollinearity. When VIF > 10 or T < 0.1, it indicates that there is a problem of collinearity in the selected data.
In this study, the covariance of the influencing factors was tested for covariance. VIF&T are obtained through computational analysis, as shown in .
Table 2. Collinearity evaluation table of evaluation factors.
shows that the VIF of the eight influencing factors selected in this study were between 1 and 3, and T is between 0.4 and 0.9. This shows that the VIF and T of the eight landslide influencing factors met the requirements of the critical values, there was no covariance problem and they had good independence (Zhou Citation2019).
2.2.2. MLP model
MLP is a feed-forward supervised learning network consisting mainly of the input layer, hidden layer, and output layer (). The hidden layer consists of artificial neurons that form a full connection between each layer, and this neural network can approximate any continuous function in the real number range. The MLP network is a function of one or more predictors that minimis prediction error.
MLP is expressed as
(6)
(6)
where
denotes the input layer vector,
is the weight,
is the bias coefficient,
is the
function, and
is the
function, The formula is:
(7)
(7)
(8)
(8)
2.3. Threshold model
The threshold model sets different thresholds for two-dimensional deformation rates and LSA levels. The zones that satisfy both the two-dimensional deformation rate threshold and the LSA threshold are potential landslide zones. The potential landslide zones are clustered and smoothed to eliminate errors. The detailed process of the threshold mode construction is shown in .
The study area is divided into 30 × 30 m grids by the fishnet function in ArcGIS10.5. Each grid contains centrally located points, and the ground surface deformation rate data and LSA results are assigned to these points by extracting multi values to points function in ArcGIS10.5.
Surface deformation rates are one of the common methods for identifying landslides, and the deformation characteristics of landslides vary from region to region. According to the findings of other researchers in the same region, the surface deformation rate of −10 to 10 mm/year is stable, with a low probability of landslides (Li et al. Citation2021). We adopt this threshold in our study. The two-dimensional deformation rate threshold for the threshold model in this study is |DU| or |DE|>10mm/year (DU and DE are the vertical and the east-west deformation rates, respectively) (). The LSA is set between 0 and 1, with 0 indicating a very low possibility of landslide, and 1 indicating a very high possibility of landslide. The natural breaks method maximises the similarity within each group and the difference between external groups, this method can separate different landslide possibilities (He et al. Citation2020; De Smith et al. Citation2015). This study used the natural breaks method to classify LSA into very low susceptibility, low susceptibility, medium susceptibility, high susceptibility, and very high susceptibility. The higher the LSA, the greater the probability of landslides (Pourghasemi et al. Citation2018). The landslide susceptibility level of the threshold model was set to very high and high susceptibility. Potential landslide zones in Lanzhou were identified by a threshold model, and points with similar deformation and susceptibility were clustered by a clustering method. There were no isolated points; each point had at least other points that were naturally adjacent to it, and the clustered points were converted to vectors by ArcGIS 10.5. The clustering method is the K-means algorithm (Jain Citation2010), as follows:
Cluster the set of points into K classes,
and the K-means algorithm finds a partition such that the empirical mean of the clusters and the points in the clusters are minimised.
is the mean of the clusters
and the root mean square error of the points
is:
(9)
(9)
The objective of the K-means algorithm is to minimise the sum of squared errors in all K classes.
(10)
(10)
To set a priori value of K-means, we combined field investigation, SBAS-InSAR and LSA results, revealing 200 was a priori value consistent with the actual situation. The points after clustering were split into several polygons, which have the disadvantage of discontinuous polygons below 1,000 m2 and tiny polygons over. In this study, we used the elimination tool to merge the polygons below 1,000 m2 with the neighbouring polygons with the longest shared boundary and to make the polygons continuous. The eliminated polygons were mainly rectangles and triangles, and the smoothing distance was set to 1,000 m for smoothing (Bodansky et al. Citation2001). Polygons smaller than 1,000 m2 that could not be eliminated or smoothed were removed.
3. Study area
Lanzhou is located at the intersection of the Qinghai-Tibet Plateau, Loess Plateau, and Inner Mongolia Plateau. Lanzhou city is long from east to west and narrow from north to south (). The city is flanked by the north and south hills, with the northern bank steeper and a single terrace, and the southern bank gently sloping and a mixed terrace. The geological structure of this area is complex, and the loess layer and gravel layer are widely distributed and mixed with a small part of the fine sand conglomerate, loam, and volcanic rock. The loess layer is mainly Malan loess with a loose structure and easy collapsibility. The city is located in an area with a temperate monsoon climate, with the precipitation concentrated in summer. The characteristics of concentrated precipitation and loess collapsibility easily predispose Lanzhou to landslides (Wang et al. Citation2019; Wang Citation2020).
Due to the constraints of the topography, urban construction had to expand to the slopes and loess plateau areas on both sides of the river valley. A series of strong human activities, such as slope cutting for engineering construction, land creation, irrigation in the surrounding mountains, irrigation of farmland, and mining of mineral resources, increased the frequency and possibility of landslides in Lanzhou (Li et al. Citation2014). A total of 227 landslides were recorded in the inventory during 2017–2018, with an area of approximately 1.4 × 106 m2, accounting for 0.35% of the whole study area (Shu et al. Citation2021). More than 10 landslide disasters have caused a large number of casualties and property losses (Su et al. Citation2019). Loess landslides are the main landslide types in Lanzhou. Loess landslides include bedrock contact landslides, palaeosol contact landslides, mixed landslides, and slides within four loess types. Loess is a typical unsaturated soil (Fredlund and Rahardjo Citation1993). It is very common to see that very steep slopes of loess can be stable for a long time, but they are vulnerable to failure when subjected to earthquake shock, rainfall, human factors, or irrigation (Tu et al. Citation2009). Both laboratory tests and field investigations have shown that loess deposits can accumulate substantial pre-failure strains as a consequence of gradually accumulating micro shears in the loess fabric (Zhang and Wang Citation2017; Shi et al. Citation2019; Zhang et al. Citation2020). Therefore, Lanzhou faces the huge threat of landslide disasters. It is necessary to identify potential landslide zones and contribute to disaster prevention and reduction in Lanzhou. The main urban area of Lanzhou, including Chengguan district, Anning district, Xigu district, and Qilihe district, was selected as the study area ().
Landslides in Lanzhou are distributed in urban areas and in the wild, especially in the south of Lanzhou. SBAS-InSAR can obtain better coherence and more comprehensive deformation data than permanent scatterer interferometric synthetic aperture radar (PS-InSAR). Therefore, SBAS-InSAR technology was selected in this study to monitor the surface deformation of Lanzhou.
4. Results and analysis
4.1. Accuracy verification of SBAS-InSAR results
To verify the reliability of SBAS-InSAR results, the typical areas with large surface deformation is selected for field investigation (). It is found that the areas with large surface deformation are mainly characterized by cracks, subsidence and collapse. Road cracks are common, ranging in width from 5 to 10 cm and in length from several meters. The crack in the wall was several centimeters wide. Through UAV aerial film, it is found that there is a gully obviously collapsed, the facade reaches several meters, the collapse boundary has a toppled power pole, and the surrounding landslide is accompanied by different sizes. It indicates that the SBAS-InSAR results of this study are credible.
4.2. Ground surface deformation rates based on SBAS-InSAR
InSAR can monitor minor ground surface deformation, reflecting the movement characteristics of landslides. SBAS-InSAR technology was used to monitor ground surface deformation rates in the main urban area of Lanzhou based on Sentinel1-A descending and ascending tracks data from 2015 to 2020. SBAS-InSAR derived deformation is in the line-of-sight (LOS) direction. Two-dimensional vertical and east-west ground surface deformation rates can be solved by using the LOS deformation values of the ascending and descending orbits (Ferretti and Prati Citation2000). According to landslide research about Lanzhou (Li et al. Citation2014; Xue et al. Citation2016; Wu et al. Citation2021), the ground surface deformation rate of −10 - 10 mm/year is too small to cause landslides. Thus, regions of −10 – 10 mm/year are defined as stable, and regions of more than −10 – 10 mm/year are defined as unstable. Therefore, the threshold of two-dimensional vertical and east-west ground surface deformation rates in this study is set as ±10 mm. The vertical and east-west surface deformation rates based on descending and ascending tracks are shown in .
Figure 8. Two-dimensional surface deformation rates in Lanzhou city, (a) vertical direction, (b) east-west direction.

The two-dimensional ground surface deformation rates of vertical and east-west directions were −32.71-12.72 mm/year and −14.88-24.81 mm/year, respectively, during 2015–2020. As shown in , the SBAS-InSAR vertical results indicate that the unstable regions spread in Qingbaishi and Kyushu in Chengguan district, Shajingyi in Anning district, and Liuquan town in Xigu district, while the SBAS-InSAR east-west results indicate that the unstable regions are distributed in Qingbaishi in Chengguan district, Yingmenqian village in Qilihe district, and Liuquan town in Xigu district. The two-dimensional SBAS-InSAR results combined descending and ascending tracks compensated for the lack of single-track detection (Liu et al. Citation2018) and detected unstable regions with vertical and east-west directions deformation rates greater than 10 mm/year or deformation rates less than −10 mm/year. Unstable regions are important data sources for the threshold model of landslides.
4.3. LSA based on the MLP model
LSA is a prediction of the possible landslides’ spatial location based on landslide influencing factors. In this study, LSA was generated based on eight landslide influencing factors and landslide inventory using the MLP model in the main urban area of Lanzhou (). The accuracy of the prediction results was verified using the receiver operating characteristic (ROC) curve. ROC is the synthesis of a confusion matrix under different thresholds and is widely used in LSA (Kavzoglu et al. Citation2015). In the landslide inventory true positive (TP) was predicted to be a landslide, and false positive (FP) was predicted to be a non-landslide. ROC with the false positive rate (FPR) as abscissa and the true positive rate (TPR) as ordinate, reflects the continuous changes of data specificity and sensitivity. The area under the curve (AUC) of the ROC can directly reflect the results (). The AUC is greater than 0 and less than 1. An AUC closer to 1 indicates that the model is more likely to correctly classify landslide and non-landslides, and closer to 0 means that the model is less likely to correctly classify landslide and non-landslide (Chen et al. Citation2018). The AUC for this study was 0.839, with a standard deviation of 0.007, indicating good results for LSA using the MLP model.
LSA ranges between 0 and 1, where 0 indicates a very low possibility of landslide, and 1 indicates a very high possibility of landslide. This study used the natural breaks method (He et al. Citation2020; De Smith et al. Citation2015) to classify the LSA into very low susceptibility (0 − 0.34), low susceptibility (0.34 − 0.48), medium susceptibility (0.48 − 0.63), high susceptibility (0.63 − 0.82) and very high susceptibility (0.82 − 1.00), which accounted for 55.36%, 10.54%, 21.37%, 9.63%, 3.1% of the total area, respectively (). High and very high susceptibility areas are located in the north and south hills of the main urban area of Lanzhou. From the perspective of landslide influencing factors, in the high and very high susceptibility areas of landslides, the stratigraphic situation is that of the upper Pleistocene wind deposits, the Xiliugou formation group, and the estuary group, consisting mainly of loess, mudstone, and siltstone. The land use types are grassland and woodland, with slopes ranging from 20° to 40° and close to rivers, roads, and faults. These regions have loose geological conditions, high slopes, and intensive human activities, which are more prone to landslides and other geological hazards. Therefore, the LSA experimental results based on the MLP model are reasonable.
According to the analysis results described above, monitoring the unstable areas based on SBAS-InSAR and the high and very high susceptibility areas of LSA based on the MLP model are consistent in spatial distribution. The unstable areas of SBAS-InSAR are delicate, and the high and very high susceptibility areas of LSA are rough. However, the two results did not identify potential landslide zones. To determine the possible landslide area in Lanzhou, this study constructs a threshold model to further extract the information from the unstable areas of SBAS-InSAR and high, very high susceptible areas of LSA to obtain potential landslide zones in Lanzhou.
4.4. Potential landslide zones based on the threshold model
We used the constructed threshold model to identify potential landslide zones in Lanzhou. The identified potential landslide zones are mainly blocky and triangular, therefore, the potential landslide zones are disposed of eliminating empty, smooth boundaries and removing speckles. A total of 117 potential landslide zones were identified in Lanzhou (). From , potential landslide zones were mainly distributed in Xujiashan National Forest Park in Chengguan district, Jinjiling and Bapanxia stations in Xigu district and Weiling town, Shifogou national forest park, Xiguyuan town, and Jingu town in Qilihe district in Lanzhou. The overlap rate of the potential landslide zones and the landslide inventory were calculated based on pixels, and we found that the overlap rate between the potential landslide zones and the landslide inventory was 40.17%, indicating that about 40% of the potential landslide zones overlapped with the landslide inventory, and about 60% were new potential landslides. These potential landslides were unstable and had high landslide sensitivity, suggesting that they had great landslide risks.
Figure 10. Distribution of the potential landslide zone in Lanzhou city (Landslide verification 1: L1and L2 belong landslide inventory and potential landslide zone; Landslide verification 2: L3 and L4 belong potential landslide zone).

The threshold model proposed in this study performs well in the Lanzhou area. The characteristics and inducing factors of landslides in different areas are regional, and the parameters of the deformation rate and LSA design of the threshold model will also be affected by regional factors. Thus, parameter learning and adjustment of the model will be needed in different regions.
To illuminate the relationship among the potential landslide zones, landslide inventory, and landslide influencing factors, we analysed the distribution among landslide influencing factors, as shown in . The potential landslide zones and the landslide inventory were concentrated at an altitude of 1451–2100 m (), in the slope of 20 - 40° (), on the northern, eastern, and western aspects of the mountain range, especially on the western aspect, accounting for about 1/3 potential landslide zones of the total potential landslide zones, with obvious slope divergence (); Moreover, the zones were mainly distributed at 2000 m from the road (), 4000 m from the railway (), 1000 m from the river () and 1000 m from the a fault (). The upper Pleistocene wind deposits and estuary group were also distributed (). To summarise the above features, the potential landslide zones and the landslide inventory distribution were characterised by areas of low elevation, sunny slopes, moderate slopes, soft lithology, and proximity to man-made facilities and rivers. It is worth noting that the potential landslide zones and the landslide inventory were quite different in the distribution of landslide influencing factors; for example, the landslide inventory had a relatively higher percentage between 1700 and 1900 m compared to potential landslide zones. The same differences can be observed for the east aspect and the distance from the railway and lithology. This may be explained by the fact that potential landslide zones are differentially assessed by the LSA and SBAS-InSAR results. InSAR monitors the movement characteristics of landslides, and LSA is a prediction of the possible landslides’ spatial location based on landslide influencing factors. The landslide inventory reports the historical landslides, which may be stationary and low-risk. Therefore, some differences are expected between the potential landslide zones and landslide inventory in the distribution of the factors influencing landslides.
Figure 11. Distribution of the potential landslide zone and the landslide inventory in landslide influencing factors (a is altitude, b is the slope, c is an aspect, d is the distance from the road, e is the distance from railway, f is the distance from the river, g is the distance from fault, h is lithology).

5. Discussion
The ground surface deformation rate obtained by InSAR technology can only reflect the surface displacement of landslides, and there is an error individually in identifying potential landslides from deformation data (Carlà et al. Citation2016). The results of the LSA can reflect the possibility of landslides from terrain, geological, hydrological, and human engineering factors, but it cannot accurately locate the spatial location of potential landslides (Huang et al. 2020). Accurately identifying landslides has become an important issue in landslide forecasting (Novellino et al. Citation2021). The research idea of this study integrated the advantages of the InSAR deformation rate reflecting landslide displacement and LSA reflecting landslide stability, combining their results to build a threshold model for identifying potential landslide zones.
The threshold model reported in this study identified potential landslide zones with the advantages of fast speed, good portability, and high identification accuracy. As long as InSAR deformation data and LSA results are available, the model can quickly determine the spatial location of potential landslides in the target area and provide target data for landslides field investigation. This study used a threshold model to identify 117 potential landslide zones in Lanzhou. Compared with existing studies about landslides in Lanzhou (Lyu et al. Citation2018; Torizin et al. Citation2018; Shu et al. Citation2021), this study combined InSAR deformation data and landslide influencing factors to realise individualised investigation of potential landslides, and convenient for landslide prevention and control. Shu et al. (Citation2021) used the weighted frequency ratio model to calculate landslide susceptibility for each type of landslides. Lyu et al. (Citation2018) evaluated the risks around Lanzhou by employing the analytic hierarchy process (AHP). Torizin et al. (Citation2018) used the weights of evidence method to calculate statistical LSA in a dynamic environment. Wang et al. (Citation2020) used the deep belief network to calculate landslide sensitivity of Sichuan Province, China, and realised a better prediction precision. Dou et al. (Citation2020) explored the predictive performance power of different sampling techniques in landslide susceptibility mapping in the wake of increased usage of artificial intelligence. These methods are a very important inspiration to improve the accuracy of the landslide prediction model. However, these existing studies assessed only the level of landslide susceptibility and did not identify specific potential landslides. However, the threshold model constructed in this study, based on the InSAR results and LSA results, can rapidly identify potential landslide zones. The accuracy of the threshold model in identifying potential landslide zones was verified through field investigation and InSAR analysis.
To further validate the threshold model, the team selected four identified potential landslides for field validation in December 2020 and for InSAR time series analysis. L1 and L2 were typical areas in both potential landslide zones and the landslide inventory to ensure the accuracy of the landslides. L3 and L4 were typical areas in potential landslide zones but not in the landslide inventory, ensuring the accuracy of potential landslides in potential landslide zones.
5.1. Landslide verification 1 (typical areas L1 and L2)
L1 is located at the Liuquan tunnel in the Xigu district of Lanzhou, with a vertical deformation rate of −26.41 − 7.64 mm/year during 2015–2020, and an accumulated deformation of −39.68 − 8.64 mm. The accumulated deformation fluctuated widely, indicating disorderly movement of the slope surface displacements (). This area has large mountains and deep gullies, with mountain heights of more than 300 m and slopes of more than 20°. Through field research, we found that L1 had obvious landslide traces and small shallow landslides exist on the river edge under the erosion of the river (Tong and Schmidt Citation2016) as shown in , with dense landslides around the tunnel and obvious landslide backwalls (). This proves the correctness of potential landslide identification, and also verifies the rationality of InSAR monitoring results.
Figure 12. L1 field investigation and InSAR analysis (a is InSAR vertical deformation rate, b is InSAR time series deformation, c and d are landslide elements).

L2 is located on the hill behind the China petrol station in Taoshuping, Chengguan district of Lanzhou. On the front edge of the slope are large advertising production factories and a petrol station. L2 vertical deformation rate was −30.56-0.94 mm/year, and the accumulated deformation rate from 2015–2020 reached −150 mm; the deformation rate continued to increase (). The field study showed that landslides had already occurred on L2 (), with significant damage to the surrounding buildings and cracks in the walls (), indicating a history of landslides and the potential for landslides to occur.
5.2. Landslide verification 2 (typical areas L3 and L4)
L3 is located at the back of the Xinhang driving school in Chengguan district. The upper part of the landslide is a residential area, and the front edge of the slope is a floodway. The deformation rate of L3 was −31.12-0.13 mm/year during 2015–2020, and the accumulated deformation rate reached −150 mm (). The unstable slope was an artificial accumulation, mainly composed of slag, rubble, etc., with a length of 80 m and a width of 50 m. After field investigation (), we found the deformation characteristics of the landslide. There were several sinkholes in the upper and lower parts of the landslide and a considerable number of longitudinal cracks in the upper part of the landslide, with a width of 30 cm, which had the possibility of landslide (Highland and Bobrowsky Citation2008).
Figure 14. L3 field investigation and InSAR analysis (a is InSAR vertical deformation rate, b is InSAR time series deformation, c-e are landslide elements).

L4 is located in the first coal mine in Liushuwan, Qilihe district. The deformation data revealed that the deformation rate in this area was large, with a deformation rate of −31.68 − 8.98 mm/year and a cumulative deformation rate of −253.52 mm (). The upper part of the landslide body power poles tilted violently, with longitudinal cracks around the landslide. Many small landslides were found in the village, mostly distributed along roads or gullies (). Affected by slope movement, the wall cracks were serious and tens of centimetres wide, indicating obvious landslide features in L4 (Highland and Bobrowsky Citation2008; Guo et al. Citation2019).
Figure 15. L4 field investigation and InSAR analysis (a is InSAR vertical deformation rate, c is InSAR time series deformation, b, d-f are landslide elements).

These field investigations and InSAR analyses of L1, L2, L3, and L4 typical areas revealed that the potential landslide zones identified by the proposed threshold model had landslide characteristics. The suggests that the potential landslides identified by the threshold model in this study were correctly located and had a high degree of confidence. The proposed threshold model can quickly and accurately identify potential landslide zones and provide target areas for landslide investigation, thus improving the efficiency of landslide identification, reducing errors in manual landslide identification, and providing technical ideas and data support for disaster mitigation and prevention.
However, there are certain limitations to this study. InSAR technology is out of coherence in certain areas with dense vegetation or excessive deformation (Ciampalini et al. Citation2016). This may lead to no deformation values in the area, thus missing these areas. In future research, L-band SAR satellites or ground deformation monitoring equipment, such as GNSS, can be considered. Two situations may pose difficulty in assessing potential landslides. The first is the region with large deformation but low landslide sensitivity, such as the surface deformation caused by artificial activities (Wu et al. Citation2019). The second is the region with high landslide sensitivity but small deformation, which has the possibility of a landslide; however, it is difficult to judge whether the region will have a landslide based on the movement of the landslide. Future research should focus on fine-tuning the proposed model to better identify potential landslides in these two cases.
6. Conclusion
Potential landslide identification is an important step in landslide preparedness, and more accurate potential landslide identification will help to develop sustainable landslide risk management and reduce landslide risks. This study combined the SBAS-InSAR deformation rates of the Sentinel1-A descending and ascending tracks and the LSA based on the MLP model to construct a threshold model that could accurately identify potential landslide zones. The main conclusions are as follows:
The two-dimensional ground surface deformation rates of vertical and east-west directions were -32.71 – 12.72 mm/year and -14.88 – 24.81 mm/year, respectively, during 2015–2020. SBAS-InSAR results indicated that the unstable regions of Lanzhou were distributed in Qingbaishi and Kyushu in Chengguan district, Shajingyi in Anning district, Yingmenqian village in Qilihe district, and Liuquan town in Xigu district.
Very low, low, medium, high, and very high susceptibility regions of the LSA accounted for 55.36%, 10.54%, 21.37%, 9.63%, 3.1% of Lanzhou, respectively. High and very high susceptibility regions were located in the north and south hills of the main urban area of Lanzhou. These regions have loose geological conditions, high slopes, and intensive human activities, which are more prone to landslides and other geological hazards.
The threshold model was constructed based on the InSAR two-dimensional deformation rates and LSA results. Using the proposed threshold model, 117 potential landslide zones were identified in Lanzhou. The overlap rate between the potential landslide zones and the landslide inventory was 40.17%, indicating that about 40% of the potential landslide zones overlapped with the landslide inventory and that about 60% were new potential landslide zones.
The proposed threshold model was verified through field research and InSAR analysis. The feasibility of the threshold model in identifying potential landslides was confirmed by field research on typical areas L1, L2, L3, and L4, which were areas with large deformation variables and landslide features.
The distribution of the potential landslide zones and the landslide inventory among landslide influencing factors was statistically analysed in Lanzhou. The potential landslide zones and the landslide inventory were distributed at low altitudes, on sunny slopes, on moderate slopes, with soft lithology, and in close proximity to man-made facilities and rivers.
The proposed threshold model presented in this paper draws on the advantages of the InSAR deformation rate reflecting landslide displacement and landslide sensitivity evaluation reflecting landslide stability to rapidly determine the spatial location of potential landslides in the study area and to provide targeting data for landslide field investigation. The proposed threshold model is highly transposable and can be flexibly adapted to the individual circumstances of the target area to achieve accurate identification of potential landslides.
Acknowledgments
The authors would like to thank the United States Geological Survey (USGS) for providing access to the SRTM DEM, Tsinghua University for providing access to the land cover types, Geological Survey (CGS) for providing access to Lithology and fault, Department of Resources of Lanzhou for providing access to River and road. At the same time, we would like to thank the reviewers and editors for their valuable suggestions and their hard work.
Disclosure statement
No potential conflict of interest was reported by the authors.
Data availability statement
All data included in this study are available upon request by contact with the corresponding author.
Additional information
Funding
References
- Berardino P, Fornaro G, Lanari R, Sansosti E. 2002. A new algorithm for surface deformation monitoring based on small baseline differential SAR interferograms. IEEE Trans Geosci Remote Sens. 40(11):2375–2383.
- Bodansky E, Gribov A, Pilouk M. 2001. Smoothing and compression of lines obtained by raster-to-vector conversion. In: GREC ’01 Selected Papers from the Fourth International Workshop on Graphics Recognition Algorithms and Applications; p. 256–265.
- Carlà T, Raspini F, Intrieri E, Casagli N. 2016. A simple method to help determine landslide susceptibility from spaceborne InSAR data: the Montescaglioso case study. Environ Earth Sci. 75(24):1–12.
- Chang KT, Merghadi A, Yunus AP, Pham BT, Dou J. 2019. Evaluating scale effects of topographic variables in landslide susceptibility models using GIS-based machine learning techniques. Sci Rep. 9(1):1–21.
- Chen Q. 2016. Analyzing landslide susceptibility in the Upper Mingjiang Basin Fuzhou, China.
- Chen W, Peng JB, Hong HY, Shahabi H, Pradhan B, Liu JZ, Zhu AX, Pei XJ, Duan Z. 2018. Landslide susceptibility modelling using GIS-based machine learning techniques for Chongren County, Jiangxi Province, China. Sci Total Environ. 626:1121–1135.
- Ciampalini A, Raspini F, Lagomarsino D, Catani F, Casagli N. 2016. Landslide susceptibility map refinement using PSInSAR data. Remote Sens Environ. 184:302–331.
- Dai KR, Deng J, Xu Q, Ye F, Li ZH, Shi XL, Hancock C, Wen NL, Zhang LL, Zhou GC. 2022. Interpretation and sensitivity analysis of the InSAR line of sight displacements in landslide measurements. GISci Remote Sens. 59(1):1226–1242.
- Dai KR, Li ZH, Tomásc R, Liu GX, Yu B, Wang XW, Chen HQ, Chen JJ, Stockamp J. 2016. Monitoring activity at the daguangbao mega-landslide (china) using sentinel-1 tops time series interferometry. Remote Sens Environ. 186:501–513.
- Dai KR, Li ZH, Xu Q, Bürgmann R, Milledge D, Tomás R, Fan X, Zhao C, Liu X, Peng J, et al. 2020. Entering the era of Earth-Observation based landslide warning system. IEEE Geosci Remote Sens Mag. 8(1):136–153.
- Dai KR, Zhuo GC, Qj X, Li ZH, Li WL, Guan W. 2019. Tracing the pre-failure t'wo-dimensional surface displacements of Nanyu Landslide, Gansu Province with radar interferometry. Geomatics Inf Sci Wuhan Univ. 44(12):1778–1786.
- De Smith MJ, Goodchild MF, Longley P. 2015. Geospatial analysis: a comprehensive guide to principles, techniques and software tools. Kibworth Beauchamp, Leicester: Troubador Publishing Ltd.
- Dou J, Yunus AP, Merghadi A, Shirzadi A, Nguyen H, Hussain Y, Avtar R, Chen YL, Pham BH, Yamagishi H. 2020. Different sampling strategies for predicting landslide susceptibilities are deemed less consequential with deep learning. Sci Total Environ. 720:137320.
- Ferretti A, Prati C. 2000. Nonlinear subsidence rate estimation using permanent scatterers in differential SAR interferometry. IEEE Trans Geosci Remote Sens. 38(5):2202–2212.
- Fredlund DG, Rahardjo H. 1993. Soil mechanics for unsaturated soils. New York (NY): Wiley.
- Ghulam A, Grzovic M, Maimaitijiang M, Sawut M. 2015. InSAR monitoring of land subsidence for sustainable urban planning. Boca Raton (FL): CRC Press.
- Grandin R, Vallée M, Lacassin R. 2017. Rupture process of the Mw 5.8 Pawnee, Oklahoma, earthquake from Sentinel‐1 InSAR and seismological data. Seismol Res Lett. 88(4):994–1004.
- Guo R, Li SM, Chen YN, Yuan LW. 2019. A method based on SBAS-InSAR for comprehensive identification of potential landslide. Geo-Spatial Inf Sci. 21(7):1109–1120.
- Hair JF, Anderson RE, Tatham RL, Black WC. 1998. Multivariate data analysis. 5th ed. all publications. NJ: Springer Science & Business Media.
- Haque U, da Silva PF, Devoli G, Pilz J, Zhao B, Khaloua A, Wilopo W, Andersen P, Lu P, Lee J, et al. 2019. The human cost of global warming: deadly landslides and their triggers (1995–2014). Sci Total Environ. 682:673–684.
- He Y, Chen Y, Wang WH, Yan HW, Zhang LF, Liu T. 2021a. TS-InSAR analysis for monitoring ground deformation in Lanzhou New district, the loess Plateau of China, from 2017 to 2019. Adv Space Res. 67(4):1267–1283.
- He Y, Wang WH, Yan HW, Zhang LF, Chen YD, Yang SW. 2020. Characteristics of surface deformation in Lanzhou with Sentinel-1A TOPS. Geosciences. 10(3):99b.
- He Y, Zhao ZA, Yang W, Yan HW, Wang WH, Yao S, Zhang LF, Liu T. 2021b. A unified network of information considering superimposed landslide factors sequence and pixel spatial neighbourhood for landslide susceptibility mapping. Int J Appl Earth Obs Geoinf. 104:102508.
- Highland LM, Bobrowsky P. 2008. The landslide handbook -a guide to understanding landslides. Reston, VA: Geological Survey Circular.
- Huang JX, Xie MW, Atkinson PM. 2020. Dynamic susceptibility mapping of slow-moving landslides using PSInSAR. Int J Remote Sens. 41(19):7509–7529.
- Huang R, Fan X. 2013. The landslide story. Nat Geosci. 6(5):325–326.
- Huang RQ. 2004. On predication of landslide. Sci Technol Manage Land Resour. 21(6):15–20.
- Jain AK. 2010. Data Clustering: 50 years beyond K-means. Int Conf Pattern Recognit. 31(8):651–666.
- Kavzoglu T, Sahin EK, Colkesen I. 2015. An assessment of multivariate and bivariate approaches in landslide susceptibility mapping: a case study of Duzkoy district. Nat Hazard. 76(1):471–496.
- Lee S, Lee CW. 2018. Analysis of the relationship between volcanic eruption and surface deformation in volcanoes of the Alaskan Aleutian Islands using SAR interferometry. Geosci J. 22(6):1069–1080.
- Li C, Fu Z, Wang Y, Tang H, Yan J, Gong W, Yao W, Criss RE. 2019. Susceptibility of reservoir-induced landslides and strategies for increasing the slope stability in the Three Gorges Reservoir Area: zigui Basin as an example. Eng Geol. 261:105279.
- Li YX, Zhang Y, Su XJ, Zhao FM, Liang YW, Meng XM, Jia J. 2021. Early identification and characteristics of potential landslides in the Bailong River Basin using InSAR technique. Nat Remote Sensing Bull. 25(2):677–690.
- Li ZH, Zhang YJ, Liang SY. 2014. Urban geological hazards and human engineering activities in Lanzhou City. J Lanzhou Univ (Natural Sciences). 50(05):588–593.
- Liu XJ, Zhao CY, Zhang Q, Peng JB, Zhu W, Lu Z. 2018. Multi-temporal loess landslide inventory mapping with C-, X- and L-band SAR datasets-a case study of Heifangtai Loess Landslides, China. Remote Sensing. 10(11):1756.
- Lyu HM, Shen JS, Arulrajah A. 2018. Assessment of geohazards and preventative countermeasures using AHP incorporated with GIS in Lanzhou, China. Sustainability. 10(2):304.
- Merghadi A, Yunus AP, Dou J, Whiteley J, ThaiPham B, Bui DT, Avtar R, Abderrahmane B. 2020. Machine learning methods for landslide susceptibility studies: a comparative overview of algorithm performance. Earth Sci Rev. 207:103225.
- Novellino A, Cesarano M, Cappelletti P, Martire DD, Napoli MD, Ramondini M, SowtereD A, Calcaterra D. 2021. Slow-moving landslide risk assessment combining Machine Learning and InSAR techniques. Catena. 203:105317.
- Pham BT, Nguyen-Thoi T, Qi C, et al. 2020. Coupling RBF-based neural network with ensemble learning techniques for landslide susceptibility mapping. Catena. 195:104805.
- Pham BT, Prakash I, Dou J, Singh SK, Trinh PT, Tran HT, Le TM, Van Phong T, Khoi DK, Shirzadi A, et al. 2019. A novel hybrid approach of landslide susceptibility modelling using rotation forest ensemble and different base classifiers. Geocarto Int. 35(12):1267–1292.
- Pourghasemi H, Gayen A, Park S, Lee CW, Lee S. 2018. Assessment of landslide-prone areas and their zonation using logistic regression, LogitBoost, and NaïveBayes machine-learning algorithms. Sustainability. 10(10):3697.
- Rodrigues SG, Silva MM, Alencar MH. 2021. A proposal for an approach to mapping susceptibility to landslides using natural language processing and machine learning. Landslides. 18: 2515–2529.
- Shi XG, Xu Q, Zhang L, Zhao KY, Dong J, Jiang H, Liao MS. 2019. Surface displacements of the Heifangtai terrace in Northwest China measured by X and C-band InSAR observations. Eng Geol. 259:105181.
- Shu H, Guo Z, Qi S, Song D, Pourghasemi HR, Ma J. 2021. Integrating landslide typology with weighted frequency ratio model for landslide susceptibility mapping: a case study from Lanzhou City of Northwestern China. Remote Sens. 13(18):3623.
- Su X, Wei WH, Ye WL, Meng XM, Wu WJ. 2019. Predicting landslide sliding distance based on energy dissipation and mass point kinematics. Nat Hazards. 96:1367–1385.
- The Peoples Government of Gansu Province. 2017. The 13th 5 years plan of geological disaster prevention and control in Gansu Province. http://www.gansu.gov.cn/art/2017/1/6/art_4827_296949.html.
- Thomas AV, Saha S, Danumah JH, Raveendran S, Prasad MK, Ajin RS, Kuriakose SL. 2021. Landslide susceptibility zonation of Idukki district using GIS in the aftermath of 2018 Kerala floods and landslides: a comparison of AHP and frequency ratio methods. J Geovis Spat Anal. 5(2):21.
- Tian CC, Tian H, Li CY, Chen FF. 2022. Stability evaluation of massive landslides using ensembled analysis of time-series InSAR and numerical simulation along the Yellow River, Northwestern of China. Geofluids. 2022:1–17.
- Tofani V, Raspini F, Catani F, Casagli N. 2013. Persistent Scatterer Interferometry (PSI) technique for landslide characterization and monitoring. Remote Sens. 5(3):1045–1065.
- Tong X, Schmidt D. 2016. Active movement of the Cascade landslide complex in Washington from a coherence-based InSAR time series method. Remote Sens. Environ. 186:405–415.
- Torizin J, Wang L, Fuchs M, Tong B, Balzer D, Wan L, Kuhn D, Li A, Chen L. 2018. Statistical landslide susceptibility assessment in a dynamic environment: a case study for Lanzhou City, Gansu Province, NW China. J Mount Sci. 15(6):1299–1318.
- Tran TH, Dam ND, Jalal FE, et al. 2021. GIS-based soft computing models for landslide susceptibility mapping: a case study of Pithoragarh District, Uttarakhand State, India. Math Probl Eng. 2021:1–19.
- Tu XB, Kwong AKL, Dai FC, Tham LG, Min H. 2009. Field monitoring of rainfall infiltration in a loess slope and analysis of failure mechanism of rainfall-induced landslides. Eng Geol. 105:134–150.
- Varnes DJ. 1978. Slope movement types and processes. Transp Res Board Spec Rep. 176:11–33.
- Wang FY, Ding Q, Zhang L, Wang MC, Wang Q. 2019. Analysis of land surface deformation in Chagan Lake Region using TCPInSAR. Sustainability. 11(18):5090.
- Wang W, He Z, Han Z, Li Y, Dou J, Huang J. 2020. Mapping the susceptibility to landslides based on the deep belief network: a case study in Sichuan Province, China. Nat Hazard. 103(3):3239–3261.
- Wang WH, He Y, Zhang LF, Chen YD, Qiu LS, Pu HY. 2020. / Analysis of surface deformation and driving forces in Lanzhou. Open Geosci. 12(1):1127–1145.
- Wang ZH. 2020. Research on early warning model of rainfall landslide in LOCSS landform combined with InSAR technology [master’s thesis]. Lanzhou, Gansu: Lanzhou Jiaotong University.
- Wu DL, Ge WP, Wei CM, Zhang B. 2021. Identification and cause analysis of potential landslides in Liujiaxia-Lanzhou Section of the Yellow River Basin with InSAR techniqsue. China Earthquake Eng J. 43(3):607–614.
- Wu Q, Jia CT, Chen SB, Li HQ. 2019. SBAS-InSAR based deformation detection of urban land, created from mega-scale mountain excavating and valley filling in the Loess Plateau: the case study of Yan’an City. Remote Sens. 11(14):1673.
- Xu Q, Tang MG, Xu KX, Huang XB. 2008. Research on space-time evolution laws and early warning-prediction of landslides. Chin J Rock Mech Eng. 27(06):1104–1112.
- Xue Y, Meng X, Wasowsk J, Chen G, Li K, Guo P, Bovenga F, Zeng R. 2016. Spatial analysis of surface deformation distribution detected by persistent scatterer interferometry in Lanzhou Region, China. Environ Earth Sci. 75(1):1–12.
- Yi YN, Zhang ZJ, Zhang WC, Jia HH, Zhang JQ. 2020. Landslide susceptibility mapping using multiscale sampling strategy and convolutional neural network: A case study in Jiuzhaigou region. CATENA. 195:104851.
- Zare M, Pourghasemi HR, Vafakhah M, Pradhan B. 2013. Landslide susceptibility mapping at Vaz Watershed (Iran) using an artificial neural network model: a comparison between multilayer perceptron (MLP) and radial basic function (RBF) algorithms. Arab J Geosci. 6(8):2873–2888.
- Zhang FY, Wang GH. 2017. Effect of irrigation-induced densification on the post-failurebehavior of loess flowslides occurring on the Heifangtai area, Gansu, China. Eng Geol. 236:111–118.
- Zhang Y, Meng XT, Jordan DCJ, Chen G, Zeng RQ, Novellino A. 2020. Forecasting the magnitude of potential landslides based on InSAR techniques. Remote Sens Environ. 241:111738.
- Zhao FM, Meng XM, Zhang Y, Chen G, Su XJ, Yue DX. 2019. Landslide susceptibility mapping of karakorum highway combined with the application of SBAS-InSAR technology. Sensors. 19(12):2685.
- Zhou X. 2019. The mechanism analysis and susceptibility mapping of the landslides along the Maoding River at the Upstream of the Jinsha River [dissertation]. Changchun, Jilin: Jilin University.
- Zhu Y, Qiu H, Yang D, Liu Z, Ma S, Pei Y, He J, Du C, Sun H. 2021. Pre- and post-failure spatiotemporal evolution of loess landslides: a case study of the Jiangou landslide in Ledu, China. Landslides. 18:3475–3484.