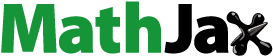
Abstract
Profiling eco-environmentally vulnerable (EV) areas can contribute to the development of mechanisms for environmental protection and sustainable management of ecological resources. Land cover change, population density, annual precipitation, mean temperature, remotely sensed indices, actual evapotranspiration, land surface temperature (LST), and runoff for the catchment for 1990, 2000, 2011, and 2020 were used to assess spatiotemporal ecological vulnerability. Spatial principal component analysis (SPCA) was used to assess thematic vulnerability for each year. The analytical hierarchical process (AHP) was then used to determine the weighting of the thematic maps to produce the final ecological vulnerability maps. Our results showed that most of the sub-basin had low and moderate vulnerability in 1990, 2000, 2011, and 2020, with the combined proportion of these areas being 80.6%, 55.45%, 83.92%, and 85.1%, respectively. The areas classified as high vulnerability decreased steadily over the investigated years, except in 2000, where an increase was observed. Most areas in the southern parts of the sub-basin were classified as moderately vulnerable, while high vulnerability values were recorded in the northern areas. The southern parts of the Upper Mzingwane basin are drier and less populated than the northern parts. The spatial architecture of vulnerability presented will help inform decision makers in mitigation planning and overall disaster risk management initiatives in the basin.
1. Introduction
Global change forces are shaping societal vulnerability in an unprecedented manner. For example, ecosystems around the world are significantly affected by human activities, climate change, and climate variability (He et al. Citation2018; Xia et al. Citation2021). The rapid growth and spread of the population have caused ecological problems such as soil erosion, land degradation, biodiversity loss and reduction of vegetation cover, especially in fragile ecosystems (Zhou et al. Citation2020). The extent of the impact of human activities and climate change on an ecosystem depends on its vulnerability (Roy et al. Citation2023). In Africa, vulnerability assessments are often sectoral; for example, they primarily deal with drought or food security (Williams et al. Citation2018). In addition, most environmental assessment studies have focused on specific variables, such as pollution and the effects of forest fires on ecosystem services and land cover changes (Jiang et al. Citation2021; Wang et al. Citation2012). Consequently, such approaches fall short of providing a holistic view of the evolution of vulnerability, which may undermine attempts to mitigate risk within the broader context of sustainable development by failure to identify root causes of risk. Eco-environmental evaluation provides a promising approach for holistically determining vulnerability since it integrates biophysical and socio-economic drivers to define vulnerability at a place and time (Wang et al. Citation2012; Jiang et al. Citation2018). Ecological vulnerability is defined as the extent to which a system, subsystem or system component can be affected by a hazard, either a disturbance or a stressor (Raj and Sharma Citation2023). It is crucial to evaluate the capacity of ecosystems to adapt to human and earth system disturbances. Furthermore, ecological vulnerability assessments help reduce potential tensions between social growth and ecological security and promote the coordinated development of populations, resources, ecosystems and the social economy (Chen and Yao Citation2022) to achieve the elusive goal of sustainability. There is paucity of literature on eco-environmental evaluations in Africa, but growing literature, particularly in Asia, for example (Kang et al. Citation2018; Wang et al. Citation2022; Zhang et al. Citation2022).
There are several methods for assessing ecological vulnerability. Comprehensive assessment (Soulé et al. Citation2021; Moreno-Miranda and Dries Citation2022), fuzzy assessment (Li et al. Citation2009; Singh et al. Citation2021; Zhang et al. Citation2021b), analytical hierarchy process (AHP) (Nguyen et al. Citation2019), principal component analysis (Wei et al. Citation2020; Xia et al. Citation2021) and landscape assessment (Pavlickova and Vyskupova Citation2015) are some of the ecological vulnerability assessment methods that have been prominently used elsewhere. Most of these methods are applied individually in literature. To adequately account for variation or heterogeneity in the ecological environment, vulnerability assessment methods need to have a high degree of applicability (Zhang et al. Citation2022). As a result, different research methods and assessment indicators are required for different ecological assessments. Combining different methods during a single assessment has the potential to deliver desired benefits as the field is still developing, and there is no widely accepted methodological and theoretical system for eco-environmental quality assessment (Wang et al. Citation2012). It is against this backdrop that, the present study combined the principal components approach and analytical hierarchical weighting approaches to improve the characterisation of vulnerability. A number of studies have focused on sensitive sites to improve the applicability of vulnerability assessment at different scales, such as, agro-ecosystems (Meng et al. Citation2010; Yang et al. Citation2019), mountainous regions (Thakur et al. Citation2021), wetlands (Wang Citation2021; Duan et al. Citation2022) and geomorphological areas (such as karst) (Chakrabortty et al. Citation2022; Saengsupavanich Citation2022) to improve the applicability of vulnerability assessment at different scales. However, this work aims to provide a comprehensive vulnerability assessment to help inform future development interventions.
Advances in remote sensing and geographic information systems (GIS) have made it possible to observe long-term spatial changes and provide more baseline data for studying ecological vulnerability. This study investigates the spatio-temporal aspects of the environment and threats to water and ecological resources in the Upper Mzingwane sub-basin. We applied remote sensing data owing to the paucity of observed data. Several studies have already successfully used remote sensing datasets for environmental assessments to identify hotspots of natural and anthropogenic risks (Frey et al. Citation2010; Chang et al. Citation2019; Cao et al. Citation2022; Effat et al. Citation2022; Gong et al. Citation2022; Thirumurthy et al. Citation2022) and produced useful results.
The semi-arid area of the upper Mzingwane catchment in Zimbabwe is a sensitive area with numerous ecological stressors. According to a study by Maviza and Ahmed (Citation2020), mining, human settlement development, water supply pollution, land degradation, and soil degradation are causing water quality degradation and water supply decline in the sub-catchment. In addition, climate change and variability is expected to increase the frequency and intensity of extreme weather events, such as droughts, which affect the ecological balance in Upper Mzingwane sub-catchment areas (Chisadza et al. Citation2023). Consequently, the ecological reserve and water supply from the sub-basin and external needs for neighbouring communities such as the city of Bulawayo may not be guaranteed. With the increasing scale and intensity of human impacts on land use, the spatial patterns of land use and landforms have also changed significantly (Zheng and Li Citation2022). As a result, ecological and environmental problems have emerged in the sub-catchment. Therefore, there is an urgent need to assess the ecological vulnerability of the upper Mzingwane catchment.
We hypothesised that environmental problems in the Upper Mzingwane sub-catchment could threaten downstream basins’ water and ecological security. Therefore, an assessment of the ecological vulnerability of a sub-basin is critical for several reasons, including environmental protection, pollution management, ecological restoration, and water resource use. The results from this study will be critical in informing mitigation planning and general disaster risk reduction initiatives in the sub-catchment. The study was conducted with the following objectives: (a) assess the spatio-temporal ecological dynamics in the sub-basin and (b) map ecologically vulnerable hotspots and (c) assess the impact of ecological vulnerability on water resources and environmental management. The novelty of this work is that it applied a combination of methods and a holistic approach to assessing vulnerability in the semi-arid upper Mzingwane sub-catchment. To the best of our knowledge, this is the first study in Zimbabwe to holistically evaluate ecological environmental vulnerability.
2. Materials and methods
2.1. Study site
This study was conducted in the Upper Mzingwane sub catchment, located in Zimbabwe’s () natural agro-ecological region IV (Manatsa et al. Citation2020), and it covers approximately 2138 km2. The sub-basin has an estimated potential evapotranspiration gradient of 1,800–2,000 mm, an annual rainfall of 450–650 mm, and mean low and high temperatures of 5 and 30 °C, respectively, as described in a study by Chisadza et al. (Citation2022). The sub-catchment accounts for 9.3% of the total flow into the Limpopo River (Maviza and Ahmed Citation2020) and is an important area with five major dams supplying water to the city of Bulawayo and parts of the sub-catchment. Therefore, thousands of people upstream and downstream of the Upper Mzingwane sub-catchment depend on the surface water from the main dams for crop irrigation, domestic and industrial use.
Figure 1. (a) Upper Mzingwane sub-catchment study area map, (b) location of the sub-catchment in Zimbabwe and (c) in Africa (Source: Author).

The soils in the sub-catchment consist of moderately coarse-grained kaolinitic sands formed from granites, and gneisses, very shallow to moderately shallow clays and loams derived from greenstone, and very shallow sands derived from basalt (Maviza and Engelbrecht Citation2021). The geology of the sub-basin is characterised by mafic rocks such as greenstone, gneiss and granite (Sibanda et al. Citation2014).
The Upper Mzingwane sub-catchment has diverse land uses, including agriculture, mining and urbanisation (Chisadza et al. Citation2023). However, subsistence agriculture is the primary source of livelihood of rural communities (Maviza and Ahmed Citation2020). Although most communal farmers in the sub-catchment practice rain-fed agriculture, some practise irrigated agriculture. The average population density in the sub-catchment is 18 people/km2 (ZIMSTAT Citation2022).
2.2. Study design and concept
A conceptual framework integrating external ecological variables (socio-economic and land resources) and internal ecological variables (hydro climate and topography) was developed for the assessment of EV over the Upper Mzingwane sub-catchment from 1990 to 2020 (). The weighting of the indicators was based on objective PCA eigenvalue weighing processes. The approach is based on the inherent characteristics of the ecological indicators (Wei et al. Citation2020). EVs were categorised into specific groups, such as hydro climate, socio-economic, land resources, and topography vulnerabilities, to obtain a comprehensive picture of the extent of EVs in the entire Upper Mzingwane sub-catchment. Finally, the group vulnerabilities were overlayed using AHP-derived weights to produce the overall EV maps for the study years.
The conceptual framework () can be summarised as follows:
S1: Selection of eco-vulnerability indicator classes and indicators.
S1 and S2: Data standardisation and subsequent processing. Determination of weights using principal component analysis (PCA) and producing thematic vulnerability maps.
S4 and S5: Determination of the overall thematic map using the analytical hierarchy process (AHP) and overall modelling to produce the EV.
S6: Assessment of the implications of the sub-basin’s spatiotemporal EV
2.3. Data
The study used several complementary datasets to examine changes in ecological vulnerability ().
Table 1. Indicators used in the study.
2.3.1. Landsat data
Landsat satellite imagery Thematic Mapper (TM), Enhanced Thematic Mapper (ETM), and Operation Land imager (OLI) was obtained from the United States Geological Survey website (http://earthexplorer.usgs.gov/) for 1990, 2000, 2011, and 2020. It was used to calculate several indices, derive land surface temperature and perform land cover classification.
2.3.1.1. Spectral indices
Landsat Top of Atmospheric reflectance imagery data was used to derive spectral indices such as the Bare Soil Index (BSI), Normalised Difference Moisture Index (NDMI), Normalised Difference Water Index (NDWI), Normalised Difference Vegetation Index (NDVI) and Normalised Difference Built Up Area Index (NDBI) (Venkatesh et al. Citation2020). All the formulae for the spectral indices and the sensors are given in .
Table 2. Formula for calculating the remote sensing indices used in the study.
2.3.1.2. Land surface temperature
The LST over the Upper Mzingwane sub-catchment was retrieved from the thermal bands of the multi-year Landsat datasets. Thermal bands 6 (10.40–12.50 μm), 6H (10.4–12.5 μm) and 10 (10.60–11.19 μm) from TM, ETM + and OLI–TIRS, respectively, with spatial resolutions of 120 m, 60 m and 100 m, were used in the study to determine the natural surface temperature over the sub-catchment (Ncube-Phiri et al. Citation2015).
2.3.1.3. Land cover classification
The supervised land cover classification technique was used for image classification using the SCP in QGIS version 3.4 under the maximum likelihood algorithm. The images were classified based on their digital numbers (DNs) using spectral signatures obtained from training samples (Matlhodi et al. Citation2019). The classified output image was used to create thematic maps. The SRTM DEM was downloaded from (https://earthexplorer.usgs.gov/) as a GeoTIFF file with a resolution of 30 m and further processed by filling in holes and then classified. The slope and aspect were derived from the SRTM DEM in ArcMap 10.8.
2.3.2. Population data
Population data for 1990, 2000, 2011 and 2020 were obtained from the Zimbabwe National Statistics Agency (ZIMSTAT) records (https://www.zimstat.co.zw/). The population data were processed and converted into population density by dividing the population for each ward in the sub-catchment by the area of the respective ward in Microsoft excel. The file was subsequently saved in CSV file format and converted into a shape file, which was further converted into GeoTIFF format.
2.3.3. Rainfall data
Annual precipitation data were obtained from the Climate Hazards Group InfraRed Precipitation with Station data (CHIRPS) dataset (https://www.chc.ucsb.edu/data/chirps) with a spatial resolution of 0.05° (Gunda et al. Citation2017). The data were resampled to 30 m spatial resolution and reprojected to UTM 35S.
2.3.4. Actual evapotranspiration
Actual evapotranspiration (ETa) and runoff data were obtained from Terra climate datasets (https://www.climatologylab.org/terraclimate.html) at 4000 m resolution (Abatzoglou et al. Citation2018). Despite the low spatial resolution of Terra Climate, these were the only data sets available that covered the entire research period (1990–2020). Terra climate actual evapotranspiration data were validated by Liu et al. (Citation2023), and the results showed comparable results with other ETa products from other remote sensing sources.
2.4. Vulnerability indicator selection
Assessing ecological vulnerability is challenging since there is no global standard or guide for the quantity and list of factors to measure ecological vulnerability (He et al. Citation2018), as the ecological environment is a dynamic system (Gu et al. Citation2015). For a scientific and credible ecological vulnerability assessment index system, the selected indicators should reflect the ecological environment of the catchment and show ecological vulnerability, developments and dynamic changes (Zhang et al. Citation2022). In this study, a detailed literature review was conducted to identify indicators for assessing the vulnerability of a semi-arid catchment. The selected indicators represent the main factors influencing societal vulnerability related to climate change and variability, hydrology, and other biophysical, social and anthropogenic environments in a semi-arid catchment. Furthermore, the availability of indicators for the study period 1990–2020 was a decisive factor in the choice of the selected indicators. The study selected 13 ecological indicators that reflected natural and human aspects and were divided into four groups/categories: socio-economic, hydro-climatic, land resources, and topographic indicators, which may influence or interact with each other in determining ecological vulnerability. The interactions between the factors can lead to information redundancy. This would lead to overlaps and redundancies in the analysis process and erroneous, unpleasant results. Thus, the PCA technique was employed to address the redundancies, if any. PCA can reduce overlaps in indicator data while retaining important principal components. The following section explains the selected indicators.
2.4.1. Socio-economic indicators
Socio-economic indicators were selected to quantify anthropogenic pressures across the study area. The selected indicators include the population and normalised difference built-up area index (NDBI). A high population and NDBI directly reflect human pressure on ecological resources and the intensity of economic activities. Population density plays a vital role in assessing ecological vulnerability, as it helps to determine human pressure on the environment. In general, higher population density leads to more pressure on the environment and, thus, higher vulnerability. The NDBI was calculated for each year using the respective Landsat data (). The NDBI can map settlements and areas of development in the surrounding area (Nguyen et al. Citation2019). Human development and settlements increase the vulnerability of an ecosystem. The derived population density and NDBI for 1990, 2000, 2011 and 2020 were used to represent socio-economic indicators in the sub-catchment. The socio-economic variables were limited to only these two indicators because adequate, consistent datasets for other indicators, such as ward-level gross domestic product (GDP), were unavailable for the study period (1990–2020).
2.4.2. Hydro climate indicators
Hydroclimatic indicators show climatic and hydrological changes. Due to the significant impact of climate variability and change on the environment (Zhang et al. Citation2021a,Citationb), meteorological indices, especially temperature and precipitation, are crucial for the assessment of EV. Precipitation is a critical component in determining the sensitivity of the ecological environment to anthropogenic and natural risks, as it represents the prevailing weather patterns in a given region (Ding et al. Citation2018). Annual precipitation is a useful indicator of weather trends that can provide early warning of drought and floods. Precipitation is crucial for soil health and plant growth. The land surface temperature can also help determine weather trends, especially in conjunction with other meteorological variables, such as precipitation, humidity and solar radiation. Temperature rise can destabilise climate conditions and vegetation. Actual evapotranspiration (ET) is also important for determining EV (Nguyen et al. Citation2019). High evapotranspiration rates cause significant soil and surface water loss, making the ecological system more vulnerable to damage. Runoff is crucial because it ensures that rivers and lakes are adequately supplied with water and changes the terrain through erosion. However, it is closely related to precipitation, slope, soil type, and plant cover parameters.
In this study, the NDMI index was used to characterise the amount of moisture in the environment, usually in the form of plants, soils and rocks (Venkatesh et al. Citation2020). The NDMI was calculated for each year using the respective Landsat data and applying the formula in . After calculating the NDMI for each year, the resulting NDMI maps were reclassified with reference to the vulnerability scale. NDWI is used for the delineation of surface water. Water is vital to the ecological balance of an ecosystem. For instance, droughts make the environment extremely vulnerable. In this study, the NDWI was used to characterise the amount of water in the surface water bodies.
2.4.3. Land resources
Land resources represent the possibility of external vulnerability. There are several other variables that can be considered under the same category, such as gross primary productivity (GPP) and net primary productivity (NPP) (Xia et al. Citation2009). For this study, only NDVI, LULC and BSI were used in this category, as consistent, reliable and adequate datasets are unavailable for the other potential variables. The NDVI () measures the amount of green cover or vegetation density. Vegetation is important in maintaining a healthy ecological environment (Nguyen et al. Citation2019). Less or no vegetation increases the vulnerability of an ecosystem. LULC determines ecosystem vulnerability by identifying human-induced changes (Shao et al. Citation2014; Nguyen et al. Citation2019). For example, ecosystems are vulnerable to changes such as land degradation, water loss and vegetation cover loss, all related to human land use. Less densely vegetated areas are more vulnerable. Impermeable surfaces retain more heat during the day and release it more slowly at night than soils or plants. BSI is most commonly used in bare soil analysis. Human activities on land, such as mining, veld fires, overgrazing and deforestation, are primarily responsible for forming barren soils. BSI is used not only to identify areas with the least vegetation but also to determine the composition and condition of the soil. The formula applied to obtain the BSI is shown in .
2.4.4. Topography
Higher altitudes are more susceptible to temperature extremes, while steep topography can lead to soil erosion and landslides. North-facing slopes receive less sunlight than south-facing slopes, which affects plant development. Thus,topography is crucial for the analysis of regional EVs. The vegetation structure, soil moisture, different plant classes, surface temperature, potential energy, and evapotranspiration are regulated by aspect. The slope should be taken into account when assessing ecological susceptibility. The terrain influences erosion. Steep slopes have shallow soils and carry a significant risk of soil degradation.
2.5. Data standardisation
The EV was evaluated using a spatial and a temporal resolution of 30 m and 1 year, respectively. Using the nearest neighbor method, indicators were resampled to a 30-m resolution. After processing the data, each indicator’s positive or negative impact was determined (). The directions of the effect indicate whether each indicator has a positive or negative relationship with ecological vulnerability. There is a positive or negative correlation between ecological vulnerability and the assessment variables (Zhao et al. Citation2018). The positive correlation indicates that ecological vulnerability decreases as the indicator score decreases (Kang et al. Citation2018). In contrast, the negative correlation suggests that ecological vulnerability increases as the indicator score decreases.
Comparing or integrating indicators is difficult because of the different time scales and units. For this reason, all indicators have been normalised to the same standard. The standardisation algorithm described by Jiang et al. (Citation2018) was used in this study [EquationEqs. (1)(1)
(1) and Equation(2)
(2)
(2) ].
Positive ecological indicator:
(1)
(1)
Negative ecological indicator:
(2)
(2)
where χij is the standardised value of the ith evaluation object for the jth indicator,
is the original value, and max(χj) and min(χj) are the maximum and minimum, respectively.
2.6. PCA to determine thematic maps
The selection of a particular assessment method for determining ecological vulnerability is an important consideration. The nature of the selected ecological variables and the resulting index’s characteristics primarily influence the choice of the assessment method. For this study, we combined remote sensing (RS) and GIS technologies and used PCA, and AHP approaches to build a reliable scientific assessment index system for dynamic ecological vulnerability assessment. PCA and AHP are widely known for providing various benefits exclusive to accumulating indicator data.
PCA is a data pre-processing method that reduces the number of original data types by applying the dimensionality reduction approach to reduce the number of indicator data to a few principal components. This reduces the total number of original variables to a smaller one (Wei et al. Citation2020). To achieve simpler handling of the indicator data and to easily show the relationships between the different indicators, the PCA approach retains as much information as possible that was originally included in the analysed data. The PCA approach identifies a small number of principal components that are more comprehensive than the original indicators. Since a smaller number of principal components represent most of the information in the primary data, the primary forces influencing ecological vulnerability could be identified. PCA was conducted separately for the indicators in each subcategory following a similar procedure by Dossou et al. (Citation2021) and Cao et al. (Citation2022). For example, six indicators were subjected to PCA for the hydro climate category. Four principal components were then selected to calculate the hydroclimate vulnerability, with the cumulative contribution of the principal components exceeding more than 90% as the default standard for this study as indicated by (Cao et al. Citation2022). The same approach was used for the socio-economic, land resource, and topographic indicators. In the latter cases, all indicators in each category were selected in the PCA. shows the eigenvalues and cumulative contributions of the principal components for hydro climate indicators for 1990–2020.
Table 3. Eigenvalues and contributions of the four selected hydro climate principal components.
2.7. AHP to determine final ecological vulnerability maps
Datasets with low correlation are unsuitable for principal component analysis (Xia et al. Citation2021). Therefore, to avoid the low correlation challenge for the final thematic maps, the AHP was applied to determine the weight and ranking of the thematic maps (Gong et al. Citation2022) of the EV for the study years. The thematic map layers were weighted using Saaty’s scale of 1–9 (Effat et al. Citation2022). The ecological vulnerability maps were developed using overlay analysis and EquationEquations (3)(3)
(3) and Equation(4)
(4)
(4) (Thirumurthy et al. Citation2022).
EquationEquations (3)(3)
(3) and Equation(4)
(4)
(4) show that the ecological vulnerability index equals the sum of the weighted principal components.
(3)
(3)
(4)
(4)
where Ci and Wi are the main components and the ith weight while λi is the eigenvalue of the ith principal component (C).
The weights of each theme are shown in . shows the weights of each thematic map. The weights reflect the significance of the thematic maps in the EVI analysis. Based on the weights of the four indicator categories, the hydro climate vulnerability map plays the most crucial role, with the highest weight of 0.312. The topographic category contributes the least weight (0.127) to the final EV analysis.
Table 4. Normalised criteria matrix with AHP.
Table 5. Weighting of the category ecological indicators using the AHP technique.
The consistency of the AHP matrix was checked using the consistency ratio (CR). For reliable comparison matrix results, the CR should be below 0.1 (He et al. Citation2018); otherwise, the result is not satisfactory.
2.8. Ecological grading scale
The ecological vulnerability was obtained by the weighted summation of the category indicators. The results were classified into five levels based on the natural breaks classification method: very low, low, moderate, high, and extreme (Zhao et al. Citation2018). Without artificial variables, this strategy minimised within-class variance and maximised between-class variation. To keep the ecological vulnerability in the four study years at the same grading standard, the mean values of the grading standards for the 4 years were taken as the final grading standard, namely, very low (<0.104), low (0.104 − 0.208), moderate (0.208 − 0.302), high (0.302 − 0.404), and extreme (0.404 − 0.503).
3. Results
3.1. Spatiotemporal dynamics of ecological vulnerability indicators
3.1.1. Dynamics in socio-economic vulnerability
shows the spatial distribution of the socio-economic vulnerability in the sub-catchment for the respective study years. Areas of high socio-economic vulnerability were mainly located in the northern parts of the catchment, close to built-up areas and densely populated settlements. (). According to the population density maps, the Insiza and Esigodini service centres had the highest population densities of 1160 km−2 and 593 km−2 in 2020, respectively. In 1990, the population densities for Insiza and Esigodini were 1092 km−2 and 522 km−2, respectively. The average population density in the sub-basin was 15 km−2, 16.56 km−2, 17.45 km−2 and 18.1 km−2 for the years 1990,2000, 2011 and 2020 respectively. Most sub-catchment areas fall under the low to moderate socio-economic vulnerability class (). A very low socio-economic vulnerability was dominant in 1990, accounting for 44.5% (). The proportion of the area classified as highly vulnerable increased from 1.4% in 1990 to 2.6% in 2020.
3.1.2. Dynamics in hydro-climate vulnerability
The spatial distribution of the hydro-climate indicators is shown in . The northern parts of the sub-catchment are more vulnerable than the southern parts, as they are mostly categorised as high to extreme vulnerability. Areas of very low, low, moderate and high vulnerability classes were equally distributed across the study years (). Low hydro climate vulnerability areas were dominant in the southern parts of the sub-catchment, accounting for 22.8%, 38.1%, 33.9%, and 38.1% of the sub-catchment area for 1990, 2000, 2011 and 2020, respectively. On the other hand, high hydroclimate vulnerability was observed in the northern parts, accounting for 31.9%, 20.9%, 27.4%, and 20.9% of the area for 1990, 2000, 2011 and 2020, respectively.
3.1.3. Dynamics in land resource vulnerability
The spatial distribution of the land resource indicators is shown in . The sub-basin’s central and northern portions had mostly high to extreme land resource vulnerability. Most sub-basin areas have been classified under low to moderate vulnerability over the years (). The LULC/NDVI vegetation class covering the sub-catchment decreased from 32 percent to 11 percent, indicating a downward trend in vegetation coverage. The settlements and cropland increased between 1990 and 2020 from 154,028.3 ha to 66,621.06 ha.
3.1.4. Dynamics in topographic vulnerability
The spatial distribution of topographic vulnerability is shown in . The sub-catchment is predominantly low (32%) to moderate (44%) in terms of topographic vulnerability (). High vulnerability is observed in the sub-catchment’s northern and central regions.
3.2. Spatiotemporal dynamics of the total ecological vulnerability of the sub-catchment
3.2.1. Distribution of ecological vulnerability
The proportion of the vulnerability class of the northern parts has been mostly moderate to high over the study years, whereas the southern regions are mostly classified under low vulnerability (). We found that the majority of the sub-catchment was classified under low and moderate hydro ecological vulnerability in 1990, 2000, 2011 and 2020, and the combined proportion areas of low and moderate vulnerability were 80.6%, 55.45%, 83.92%, and 85.1%, respectively. The area classified as high vulnerability has been steadily decreasing over the years (19.03% in 1990 to 14.72% in 2020), except for the year 2000 (43.83%) (). The mean ecological vulnerabilities are shown in . Overall, the year 2000 was the most vulnerable compared to all the study years. The mean ecological value for 2000 was 0.30, representing a high vulnerability on the grading scale. For 1990, it was 0.24, and for 2011 and 2020, it was 0.25, representing moderate vulnerability. Areas of very low vulnerability accounted for a total of 0.67% during all the study years. On the other hand, areas of extreme vulnerability accounted for a total of 0.78% over the study years.
Figure 7. Spatial distribution of ecological vulnerability of the Upper Mzingwane sub-catchment (1990–2020).

4. Discussion
4.1 Spatiotemporal dynamics of ecological vulnerability
Ecological vulnerability in the sub-basin showed a relatively similar spatio-temporal pattern of low to moderate vulnerability from 1990 to 2020, with the exception of the year 2000. One possible reason for this pattern could be that the sub-basin is mainly rural, and significant human-induced changes are relatively low and slow. It should be noted that artisanal mining is the main activity in the catchment that has potential to transform ecosystems and increase vulnerability rapidly. However, artisanal mining is isolated and sparsely distributed in the catchment, being limited to areas where the ore bodies are available and close to the surface. Hence, the overall impact on the catchment is low, resulting in low to moderate vulnerability. Furthermore, the annual values of the most important climate variables, such as temperature, precipitation and evapotranspiration, are relatively similar over time, as there have only been insignificant trends in the sub-basin over the last 30 years (Sibanda et al. Citation2020; Maviza and Engelbrecht Citation2021). Hydroclimate, socio-economic and land resources had relatively high eigen values that contributed to some spatial and temporal variations in ecological vulnerability in some areas of the sub-basin. This is in agreement with Wang et al. (Citation2022), who also observed spatiotemporal heterogeneity in the Dongliao River Basin’s ecological vulnerability in China.
The northern parts of the sub-catchment were more vulnerable than the southern parts. This could be due to increased land conversion to built-up land or settlements and a decrease in vegetation cover in the northern parts of the sub-basin. Land use directly influences ecological vulnerability, which is a natural reflection of human activity (Ding et al. Citation2018). According to studies, high intensity of anthropogenic activities leads to low EV and vice versa, reducing the ecological land base and increasing the variability of land cover patterns (Boori et al. Citation2022; Ran et al. Citation2022; Thirumurthy et al. Citation2022). Similarly, the northern parts of the catchment had a higher population density than the southern parts, and this predisposes it to high ecological vulnerability owing to the intense use of ecological resources. This is consistent with Cao et al. (Citation2022), who observed that population agglomeration is correlated with ecological vulnerability. The increase in built-up areas in the towns of Esigodini and Filabusi, located in the northern part of the sub-basin, leading to deforestation, higher water demand and deterioration of water quality, may explain the high vulnerability in the northern areas. High ecological vulnerability is associated with intense human activity, especially in towns, and environmental restoration after human disturbance is complex (Zou and Yoshino Citation2017). The concentration of mining areas in the northern parts of the catchment could be another reason for high vulnerability. Similar observations were made by Ncube-Phiri et al. (Citation2015) in the Mzingwane district. The findings by Sibanda et al. (Citation2014) also demonstrated that small-scale mining significantly reduces the number of individual trees and the species diversity of the forest and poses ecological risk.
Overall, the hydro climate variable had the highest weighting contribution of 0.312 compared to the other category indicators. This is in line with a study by Jiang et al. (Citation2018), which indicates that the ecological environment in an arid and semi-arid region is largely determined by natural forces, especially precipitation and evapotranspiration. The evapotranspiration indicator had a higher PCA contribution rate than the other hydro-climate variables. The northern parts of the sub-catchment had consistently high evapotranspiration values owing to a relatively higher terrestrial water storage as there are three large dams (Chisadza et al. Citation2022) and mean annual precipitation of 650 mm is relatively higher than the 450 mm average in the southern part of the sub catchment.
Hence, the northern parts of the catchment were classified under high ecological vulnerability. High evapotranspiration reduces the ecosystem’s ability to provide water, which could eventually affect the social system’s ability to cope with water scarcity caused by climatic drought (Yang et al. Citation2015).
Most areas that experienced significant ecological vulnerability increases over the years are those in the central and northern portions of the sub-basin. As a result, these areas were classified under high ecological vulnerability. The dense settlement patterns located in the northern and central portions of the catchment and along the surface water sources are the reason for high to extreme vulnerability in these areas. This is consistent with Thakur et al. (Citation2020), who reported high land resource vulnerability coinciding with intensified agricultural land and an increase in degraded areas. Furthermore, the same northern parts of the sub-basin host several small-scale mining activities, particularly in the Insiza and Umzingwane districts (Moyo et al. Citation2018), which, although isolated, could be increasing vulnerability due to injudicious mining methods. Therefore, they are prone to land degradation and pollution.
4.2. Implications of EV for water resources and environmental management
The main finding from this study is that the sub-catchment is generally classified under low to moderate ecological vulnerability from 1990 to 2020. However, the spatial distribution of this EV shows that the northern and central parts of the sub-basin are highly vulnerable. As such, this has negative implications for water resources and the environment. This is consistent with Davis et al. (Citation2014), who reported that existing dams built for water supply and irrigation may become less reliable because of high evapotranspiration and erratic rainfall patterns.
The northern parts of the catchment receive more rainfall than the southern regions. Moreover, the northern parts of the sub-catchment host the major surface water bodies and contribute significantly (9%) to the Limpopo River basin through runoff (Maviza and Engelbrecht Citation2021). Therefore, the high ecological vulnerability in the northern areas could lead to reduced inflows into the Limpopo River basin, reduced agricultural production and less water available to the sub-catchment and Bulawayo city. In addition, water conservation mechanisms are needed, particularly in the northern areas of the catchment, to address the high evapotranspiration-driven vulnerability. A similar suggestion was made by Yang et al. (Citation2015), who suggested that in cases of high evapotranspiration, adaptive methods to lessen climate change should include the development of water-saving agriculture practices.
The spatial distribution of EVs can provide a platform for informed water and environmental management. In the case of this study, the results indicate that the northern and central regions are classified under high EV, thus implying that there is a need to prioritise environmental management in the northern areas, which are highly vulnerable. The causes of this high vulnerability in the northern parts of the catchment include an increase in population agglomeration and an increase in agriculture, mining and degraded land, as observed by Chisadza et al. (Citation2022). Therefore, the management of land use development patterns needs to be considered together with environmental management planning. Our results suggest that the ecological vulnerability of the upper Mzingwane sub-basin is spatially heterogeneous due to regional differences in the areas’ exposure, sensitivity and adaptive capacity. The results of our ecological vulnerability assessment can help inform future decisions and facilitate the safeguarding of ecological quality. Ecological vulnerability mapping can be valuable for policymakers, non-governmental organisations and other development partners, especially to quickly identify hotspots of high vulnerability.
The study was limited by the lack of long-term data for indicators, especially social indicators. In addition, some of the indicators, like precipitation and runoff, had a low spatial resolution. Nevertheless, this vulnerability assessment still provides useful information to inform decision-makers in targeting mitigation measures to specific areas more relevant recommendations and guidance for developing environmental management strategies (Peng et al. Citation2023). To be effective in this regard, there is a clear need for policies and technical solutions to mitigate pressures such as ecosystem degradation and climate change that make the environment and water resources more vulnerable. A long-term strategic development plan should be prepared, focusing on implementing effective management practises in line with the concept of integrated water resources management and sustainable use of natural resources. Additional efforts are required to improve irrigation water use efficiency, water conservation technologies, rainwater harvesting, etc.
5. Conclusion
The spatial distribution of the ecological vulnerability of the sub-basin was low to moderate and showed a relatively similar pattern from 1990 to 2020, except for the year 2000. The combined low and moderate ecological vulnerability areas were 80.6%, 55.45%, 83.92%, and 85.1% for 1990, 2000, 2011 and 2020, respectively. The northern parts of the sub-basin appear to be more vulnerable than the southern parts and fall into the high ecological vulnerability category owing to high evapotranspiration, increased population density, mining, and increases in built-up areas and settlements. The temporal changes were relatively small in the EV, indicating that the system was relatively stable during the study period. The vulnerability of the northern and central areas of the sub-basin is the result of anthropogenic and natural climatic factors. The high vulnerability areas contain the main dams of the sub-basin, which could undermine the availability of surface water resources and impair ecosystem services. This may also lead to reduced inflows into the transboundary Limpopo River basin, reduced agricultural production and less water available to the sub-catchment and the city of Bulawayo. This work presented the architecture of vulnerability, which could be used in prioritising areas and inform types of development interventions to be unrolled to address underlying causes of vulnerability and achieve sustainable development. The maps produced could be used by government in environmental and development planning. The study recommends that highly vulnerable areas be subjected to high-priority ecological management and that ecological restoration of areas with high population densities, mining sites and degraded areas be carried out. Some recommended management practices include adopting water conservation for domestic, mining and agriculture, restoration of post-mining land, and reforestation in and around urban development.
Compliance with ethical standards
Research involving human participants and/or animals
The research did not involve human participants and/or animals.
Data availability statement
Data and materials that support the results presented in this paper can be freely made available from the first author, Bright Chisadza, upon reasonable request.
Disclosure statement
No potential conflict of interest was reported by the authors.
Additional information
Funding
References
- Abatzoglou JT, Dobrowski SZ, Parks SA, Hegewisch KC. 2018. TerraClimate, a high-resolution global dataset of monthly climate and climatic water balance from 1958–2015. Sci Data. 55(1):1–12.
- Boori MS, Choudhary K, Paringer R, Kupriyanov A. 2022. Using RS/GIS for spatiotemporal ecological vulnerability analysis based on DPSIR framework in the Republic of Tatarstan, Russia. Ecol Inform. 67:101490.
- Cao Js, Yang Yq, Deng Zy, Hu Yd. 2022. Spatial and temporal evolution of ecological vulnerability based on vulnerability scoring diagram model in Shennongjia, China. Sci Rep. 12(1):1–16.
- Chakrabortty R, Pal SC, Santosh M, Roy P, Chowdhuri I. 2022. Gully erosion and climate induced chemical weathering for vulnerability assessment in sub-tropical environment. Geomorphology. 398:108027.
- Chang Y, Hou K, Wu Y, Li X, Zhang J. 2019. A conceptual framework for establishing the index system of ecological environment evaluation: a case study of the upper Hanjiang River, China. Ecol Indic. 107:105568.
- Chen S, Yao S. 2022. Evaluation and dynamic prediction of ecological security from the perspective of sustainable development: a case study of Shaanxi Province, China. Environ Sci Pollut Res Int. 29(28):42331–42346.
- Chisadza B, Gwate O, Ncube F, Moyo N, Chiwara P. 2022. Detecting land surface water changes in the Upper Mzingwane sub-catchment using remotely sensed data. J Water Supply Res Technol. 71(10):1180–1196.
- Chisadza B, Gwate O, Ncube F, Mpofu N. 2023. Assessment and characterisation of hydrometeorological droughts in the Upper Mzingwane sub-catchment of Zimbabwe. Nat Hazards. https://doi.org/10.1007/s11069-022-05807-9
- Davis R, Hirji R, Murwira A, et al. 2014. Climate change and water resources planning, development, and management in Zimbabwe.
- Ding Q, Shi X, Zhuang D, Wang Y. 2018. Temporal and spatial distributions of ecological vulnerability under the influence of natural and anthropogenic factors in an eco-province under construction in China. Sustainability. 10(9):3087.
- Dossou JF, Li XX, Sadek M, Sidi Almouctar MA, Mostafa E. 2021. Hybrid model for ecological vulnerability assessment in Benin. Sci Rep. 11(1):1–15.
- Duan H, Yu X, Zhang L, Xia S, Liu Y, Mao D, Zhang G. 2022. An evaluating system for wetland ecological risk: case study in coastal mainland China. Sci Tot Environ. 828:154535.
- Effat HA, Ramadan MS, Ramadan RH. 2022. A spatial model for assessment of urban vulnerability in the light of the UN New Urban Agenda guidelines: case study of Assiut City, Egypt. Model Earth Syst Environ. 8(3):3687–3706.
- Frey H, Huggel C, Paul F, Haeberli W. 2010. Automated detection of glacier lakes based on remote sensing in view of assessing associated hazard potentials. Autom Detect Glacier Lakes Based Remote Sens View Assess Assoc Hazard Potentials Grazer Schriften Der Geogr Und Raumforschung. 45:261–272.
- Gong J, Jin T, Cao E, Wang S, Yan L. 2022. Is ecological vulnerability assessment based on the VSD model and AHP-entropy method useful for Loessial forest landscape protection and adaptative management? A case study of Ziwuling Mountain Region, China. Ecol Indic. 143:109379.
- Gu Q, Li J, Deng J, Lin Y, Ma L, Wu C, Wang K, Hong Y. 2015. Eco-environmental vulnerability assessment for large drinking water resource: a case study of Qiandao Lake Area, China. Front Earth Sci. 9(3):578–589.
- Gunda R, Chimbari MJ, Shamu S, Sartorius B, Mukaratirwa S. 2017. Malaria incidence trends and their association with climatic variables in rural Gwanda, Zimbabwe, 2005–2015. Malar J. 16(1):1–13.
- He L, Shen J, Zhang Y. 2018. Ecological vulnerability assessment for ecological conservation and environmental management. J Environ Manage. 206:1115–1125.
- Jiang L, Huang X, Wang F, Liu Y, An P. 2018. Method for evaluating ecological vulnerability under climate change based on remote sensing: a case study. Ecol Indic. 85:479–486.
- Jiang Y, Shi B, Su G, Lu Y, Li Q, Meng J, Ding Y, Song S, Dai L. 2021. Spatiotemporal analysis of ecological vulnerability in the Tibet Autonomous Region based on a pressure-state-response-management framework. Ecol Indic. 130:108054.
- Kang H, Tao W, Chang Y, Zhang Y, Xuxiang L, Chen P. 2018. A feasible method for the division of ecological vulnerability and its driving forces in Southern Shaanxi. J Clean Prod. 205:619–628.
- Li L, Shi Z-H, Yin W, Zhu D, Ng SL, Cai C-F, Lei A-L. 2009. A fuzzy analytic hierarchy process (FAHP) approach to eco-environmental vulnerability assessment for the danjiangkou reservoir area, China. Ecol Modell. 220(23):3439–3447.
- Liou Y-A, Nguyen AK, Li M-H. 2017. Assessing spatiotemporal eco-environmental vulnerability by Landsat data. Ecol Indic. 80:203–223.
- Liu G, Wang J, Li S, et al. 2019. Dynamic evaluation of ecological vulnerability in a lake watershed based on RS and GIS Technology. Polish J Environ Stud. 28:1785–1798.
- Liu H, Xin X, Su Z, Zeng Y, Lian T, Li L, Yu S, Zhang H. 2023. Intercomparison and evaluation of ten global ET products at site and basin scales. J Hydrol. 617:128887.
- Manatsa D, Mushore TD, Gwitira I, et al. 2020. Revision of Zimbabwe’s agro-ecological zones. Produced by the Government of Zimbabwe under Zimbabwe National Geospatial and Space Agency (ZINGSA) for the Ministry of Higher and Tertiary Education, Innovation. Sci Technol Dev Zimbabwe Gov Printers.
- Matlhodi B, Kenabatho PK, Parida BP, Maphanyane JG. 2019. Evaluating land use and land cover change in the Gaborone dam catchment, Botswana, from 1984–2015 using GIS and remote sensing. Sustainability. 11(19):5174.
- Maviza A, Ahmed F. 2020. Analysis of past and future multi-temporal land use and land cover changes in the semi-arid Upper-Mzingwane sub-catchment in the Matabeleland south province of Zimbabwe. Int J Remote Sens. 41(14):5206–5227.
- Maviza A, Engelbrecht F. 2021. Twentieth century precipitation trends in the Upper Mzingwane sub-catchment of the Northern Limpopo basin, Zimbabwe. Theory Appl Climatol. 149:309–325.
- Meng J, Zhang Y, Zhou P. 2010. Ecological vulnerability assessment of the farming-pastoral transitional zone in Northern China: a case study of Ordos city. J Desert Res. 30(4):850–856.
- Moreno-Miranda C, Dries L. 2022. Integrating coordination mechanisms in the sustainability assessment of agri-food chains: from a structured literature review to a comprehensive framework. Ecol Econ. 192:107265.
- Moyo F, Ndlovu T, Francis B, et al. 2018. The effects of artisanal mining on irrigation farming: the case of Umzinyathini Irrigation Scheme in Umzingwane District, Southern Matabeleland, Zimbabwe. Afr J Public Aff. 10:139–162.
- Ncube-Phiri S, Ncube A, Mucherera B, Ncube M. 2015. Artisanal small-scale mining: potential ecological disaster in Mzingwane District, Zimbabwe. Jamba. 7(1):158.
- Nguyen CT, Chidthaisong A, Diem P K, Huo LZ. 2021. A modified bare soil index to identify bare land features during agricultural fallow-period in southeast asia using landsat 8. Land. 10:1–18.
- Nguyen KA, Liou YA, Terry JP. 2019. Vulnerability of Vietnam to typhoons: a spatial assessment based on hazards, exposure and adaptive capacity. Sci Tot Environ. 682:31–46.
- Pavlickova K, Vyskupova M. 2015. A method proposal for cumulative environmental impact assessment based on the landscape vulnerability evaluation. Environ Impact Assess Rev. 50:74–84.
- Peng Y, Welden N, Renaud FG. 2023. A framework for integrating ecosystem services indicators into vulnerability and risk assessments of deltaic social-ecological systems. J Environ Manage. 326(Pt A):116682.
- Raj A, Sharma LK. 2023. Spatial E-PSR modelling for ecological sensitivity assessment for arid rangeland resilience and management. Ecol Modell. 478:110283.
- Ran P, Hu S, Frazier AE, et al. 2022. Exploring changes in landscape ecological risk in the Yangtze river economic belt from a spatiotemporal perspective. Ecol Indic. 137:108744.
- Roy P, Pal SC, Chakrabortty R, Chowdhuri I, Saha A, Shit M., 2023. Effects of climate change and sea-level rise on coastal habitat: vulnerability assessment, adaptation strategies and policy recommendations. J Environ Manage. 330:117187.
- Saengsupavanich C. 2022. Flaws in coastal erosion vulnerability assessment: physical and geomorphological parameters. Arab J Geosci. 15:1–17.
- Shao H, Liu M, Shao Q, et al. 2014. Research on eco-environmental vulnerability evaluation of the Anning River Basin in the upper reaches of the Yangtze River. Environ Earth Sci. 72:1555–1568.
- Sibanda S, Grab SW, Ahmed F. 2020. Long-term rainfall characteristics in the Mzingwane catchment of south-western Zimbabwe. Theor Appl Climatol. 139:935–948.
- Sibanda S, Masaka M, Chirima A. 2014. Effect of small scale gold mining on vegetation species diversity in Umzingwane. Environ Sci Indian J. 9:71–84.
- Singh P, Hasnat M, Rao MN, Singh P. 2021. Fuzzy analytical hierarchy process based GIS modelling for groundwater prospective zones in Prayagraj, India. Groundw Sustain Dev. 12:100530.
- Soulé E, Michonneau P, Michel N, Bockstaller C. 2021. Environmental sustainability assessment in agricultural systems: a conceptual and methodological review. J. Clean Prod. 325:129291.
- Thakur S, Negi VS, Dhyani R, et al. 2021. Vulnerability assessments of mountain forest ecosystems: a global synthesis. Trees for People. 6:100156.
- Thakur S, Negi VS, Pathak R, et al. 2020. Indicator based integrated vulnerability assessment of community forests in Indian West Himalaya. For Ecol Manage. 457:117674.
- Thirumurthy S, Jayanthi M, Samynathan M, et al. 2022. Multi-criteria coastal environmental vulnerability assessment using analytic hierarchy process based uncertainty analysis integrated into GIS. J Environ Manage. 313:114941.
- Venkatesh R, Abdul Rahaman S, Jegankumar R, Masilamani P. 2020. Eco-environmental vulnerability zonation in essence of environmental monitoring and management. Int Arch Photogramm Remote Sens Spat Inf Sci ISPRS Arch. 43:149–155.
- Wang A, Liao X, Tong Z, et al. 2022. Spatiotemporal variation of ecological carrying capacity in Dongliao River Basin, China. Ecol Indic. 135:108548.
- Wang H. 2021. Regional assessment of ecological risk caused by human activities on wetlands in the Muleng–Xingkai plain of China using a pressure–capital–vulnerability–response model. Wetl Ecol Manag. 30:111–126.
- Wang X, Cao Y, Zhong X, Gao P. 2012. A new method of regional eco-environmental quality assessment and its application. J Environ Qual. 41:1393–1401.
- Wei W, Shi S, Zhang X, et al. 2020. Regional-scale assessment of environmental vulnerability in an arid inland basin. Ecol Indic. 109:105792.
- Williams PA, Crespo O, Abu M, Simpson NP. 2018. A systematic review of how vulnerability of smallholder agricultural systems to changing climate is assessed in Africa. Environ Res Lett. 13:103004.
- Xia M, Jia K, Zhao W, et al. 2021. Spatio-temporal changes of ecological vulnerability across the Qinghai–Tibetan plateau. Ecol Indic. 123:107274.
- Xia X, Yang Z, Wu Y. 2009. Incorporating eco-environmental water requirements in integrated evaluation of water quality and quantity: a study for the Yellow River. Water Resour Manag. 23:1067–1079.
- Yang H, Luo P, Wang J, et al. 2015. Ecosystem evapotranspiration as a response to climate and vegetation coverage changes in Northwest Yunnan, China. PLoS One. 10:e0134795.
- Yang Y, Wang K, Liu D, et al. 2019. Spatiotemporal variation characteristics of ecosystem service losses in the agro-pastoral ecotone of Northern China. Int J Environ Res Public Heal. 16:1199.
- Zhang H, Sun Y, Zhang W, et al. 2021a. Comprehensive evaluation of the eco-environmental vulnerability in the Yellow River Delta wetland. Ecol Indic. 125:107514.
- Zhang X, Liu K, Wang S, et al. 2022. Spatiotemporal evolution of ecological vulnerability in the Yellow River Basin under ecological restoration initiatives. Ecol Indic. 135:108586.
- Zhang Y, Chang Y, Yu K, et al. 2021b. Difference analysis of ecological vulnerability and Zoning changes of National energy and chemical bases using FAHP method. Int J Environ Res Public Heal. 18:6785.
- Zhao J, JG, Tian Y, et al. 2018. Environmental vulnerability assessment for mainland China based on entropy method. Ecol Indic. 91:410–422.
- Zheng H, Li H. 2022. Spatial–temporal evolution characteristics of land use and habitat quality in Shandong Province, China. Sci Rep. 12:15422.
- Zhou Y, Li Y, Liu Y. 2020. The nexus between regional eco-environmental degradation and rural impoverishment in China. Habitat Int. 96:102086.
- ZIMSTAT 2022. July 2022. Harare.
- Zou T, Yoshino K. 2017. Environmental vulnerability evaluation using a spatial principal components approach in the Daxing’anling region, China. Ecol Indic. 78:405–415.