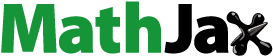
Abstract
Debris flow occurs frequently in mountainous areas due to the special geographical and geological environment, causing significant damage to linear infrastructure. However, a systematic assessment of debris flow risk to the national highway is limited by the lack of regional vulnerability data. Based on a detailed inventory of debris flow and highway structure characteristics, this study presents a comprehensive regional risk assessment framework on the northern line of the Sichuan-Tibet Highway in China. The proposed framework consists of three main procedures: (1) debris flow hazard analyses by the weight-of-evidence method, (2) analyses of physical vulnerability using 12 vulnerability indexes, and (3) risk calculation which considers the natural attributes of debris flow and the social characteristics of a linear highway. The predictive performance was evaluated through receiver operating characteristic (ROC) curves, and the validation results showed that areas under the ROC curves were 83.77%. The risk zoning map indicated that the high-risk areas were particularly distributed in Dege County and Daofu County, with a high disaster frequency and a low highway protection standard. The regional risk assessment framework supports decision-making for disaster prevention and highway rehabilitation in mountainous areas.
HIGHLIGHT
We have constructed a regional risk assessment framework for the national highway in mountainous areas.
Vulnerability indicators provide detailed information on the structural characteristics of the highway.
Analysis of debris flow characteristics and causative factors about the northern line of the Sichuan-Tibet Highway.
1. Introduction
Debris flows are typical natural hazards triggered by intense rainfall or snow-melting in mountainous areas which commonly cause enormous losses (Santi et al. Citation2011). Especially, in the traffic line, even highly localized debris flow events may cause widespread and prolonged disruptions to the regional road due to their linear characteristics. Sichuan-Tibet Highway, as one of the significant channels for the mainland to enter and leave Tibet, is prone to debris flow disasters because of its special geographical and geological environment (Zhang, Citation2016; Wang et al. Citation2020; Huang, Citation2021). As an important approach for hazard mitigation, risk assessment can provide significant information to the administrations and is useful for decision-making, therefore, it is scientifically and practically an important technique (Cui et al. Citation2013; Yin and Zhang, Citation2018; Hu et al. Citation2019; Zheng et al. Citation2021).
The risk assessment of debris flow along traffic lines mainly consists of debris flow hazard assessment and vulnerability assessment (United Nations, Citation1991). With the development of ‘3S’ technology, risk assessment has been continuously improved and gradually realized quantitatively. According to the objectives, the hazard assessment of the traffic lines can be divided into two aspects: the range affected by debris flow, which is mostly realized at a specific catchment scale using numerical simulation approaches (Yin and Zhang Citation2018; Hu et al. Citation2019; Baggio et al. Citation2021; Nie et al. Citation2021), and the distribution and classification of the regional area affected by debris flow, which is generally realized based on GIS technology combined with historical statistical data (Kazakova et al. Citation2017; Jiang et al. Citation2022). Although the former is more accurate in assessment results (Nie et al. Citation2021), the application of the latter is also very necessary for debris flow mitigations along traffic lines, which generally cover long routes and extensive areas. The key point to the debris flow hazard assessment is the selection of a reasonable method, targeted evaluation indexes, and the accurate calculation of index weight. The weights identification methods are roughly divided into two categories: in the first category, the identification is carried out by the information contained in the indicator data, such as the entropy weighting method (Chen et al. Citation2007; Li et al. Citation2020), and in the second category, it is performed based on the volatility of the indicator data or the interrelationship between indicators, such as the grey correlation method (Kuo et al. Citation2008; Wang and Pu, Citation2018; Yuan et al. Citation2022). It is worth noting that the weight-of-evidence method is a combination of entropy weight and grey correlation, combining the advantages of both approaches (Agterberg, Citation2011; Martha et al. Citation2013; Chen et al. Citation2016).
The definition of vulnerability assessment varies according to the objectives (Xu et al. Citation2010; Citation2016; Ciurean et al. Citation2017). Recent studies have concentrated on the direct effects of debris flows on road networks to assess the vulnerability of road infrastructure by establishing vulnerability curves. This approach involves the functionality of road networks (Sullivan et al. Citation2010; Meyer et al. Citation2015; Utasse et al. Citation2016), road structures such as bridge pier foundations (Zhang et al. Citation2018; Yan et al. Citation2020; Ali et al. Citation2021), and passengers and logistics truck drivers (Winter and Wong, Citation2020). However, in mountainous areas, this type of quantitative model like the ‘vulnerability curve’ has not become universal yet due to the complexity and difficulty of data collection (Kazakova et al. Citation2017). In terms of quantitative expression of highway vulnerability and selection of evaluation indexes on a regional scale, a set of vulnerability evaluation systems was proposed by considering the exposure properties of disaster-affected objects (Zou et al. Citation2018; Citation2019). The vulnerability definition is diversified, and index selection and quantitative expression are both still difficult to realize (Zeng et al. Citation2012; Papathoma-Köhle et al. Citation2017). There is no uniform standard for index selection and classification, resulting in great uncertainty. Therefore, it is believed that the core of vulnerability assessment along the traffic lines lies in the quantitative expression of highway damage due to debris flow disasters to realize the transformation of the disaster point to the linear highway.
The objective region in this study was the northern line of the Sichuan-Tibet Highway, which extends from Chengdu in the east to Lhasa in the west, which is the so-called ‘317 National Highway’ in China. It spreads along the Xianshui River Fault Zone with the strongest seismic activities. The frequent geological disasters such as debris flow have influenced traffic safety very significantly, making risk analysis necessary. However, little previous research has been carried out in this area compared to the neighboring regions (Shang et al. Citation2005; Zou et al. Citation2018; Hu et al. Citation2020) due to the lack of multitemporal debris flow inventory and detailed highway structures.
Hence, we propose a regional risk assessment framework for the Sichuan-Tibet Highway. First, we obtained an inventory of past debris flow events, including more than 235 catchments. Second, we built up a set of comprehensive index systems involving five causative factors for hazard and four characteristics indicators for vulnerability from previous works (Zou et al. Citation2018; Dave et al. Citation2021; Dash et al. Citation2022; Huang et al. Citation2022). Finally, we adopted the receiver operating characteristic (ROC) curve and real debris flow events to evaluate the reliability of the framework. An overall schematic illustration of the steps involved is shown in (He et al. Citation2020; Jamali et al. Citation2022). Our main objectives were as follows: (1) to obtain the debris flow inventory and to explore the dominant factors that trigger the occurrence of debris flow, (2) to establish a structural characteristic index system for highways and to assess the vulnerability, (3) to generate the debris flow risk zonation map of the national highway and to provide a reference for emergency countermeasures against the debris flow disasters along the traffic line in mountainous areas.
2. Study area
The selected study area extends from Kangding in Sichuan to Changdu in Tibet and is bounded by the catchment area distributed along both sides of the highway that intersects the outlet (). The area is located on the southeastern edge of the Qinghai-Tibet Plateau, and the overall topography is higher in the northwest and lower in the southeast. Due to the tectonic uplift of the Plateau and river undercutting, several rivers including the Yalong River, Jinsha River, and Xianshui River are distributed in this area and crossed by ‘317 National Highway’. According to the elevation, depth of cut, and type of geomorphology, the study area can be divided into four parts: (A) broad valley areas in the upper reaches of the three rivers, (B) extremely high alpine areas formed by glacial erosion, (C) high alpine valley areas formed by erosion and denudation, and (D) high alpine plateau areas formed by erosion and denudation (). Due to the barrier effect of the Qinghai-Tibet Plateau, the spatial variation of precipitation in the study area is obvious. The precipitation gradually increases from northwest to southeast. For instance, the average annual rainfall is less than 600 mm in Changdu (in the northwest) and 700 mm in the Xianshui River valley in the southeast. In terms of time distribution, rainfall chiefly occurs from June to September, with an annual average of 523–802 mm.
The study area is largely located within the Qiangtang-Sanjiang orogenic system, consisting of three first-order tectonic units (i.e. II: Qiangtang-Sanjiang orogenic system) and eight second-order tectonic units (i.e. II2: Garze-Litang arc-basin system) (). The highway extends along the northwest section of the Xianshui River Fault (F1), the southeast section of the Ganzi-Yushu Fault (F2), and the Jinsha River Fault Zone (F3). These areas are known for dense faulting, strong magmatic activities, and frequent seismic activities (Wen et al. Citation2008; Bai et al. Citation2018; Qiao and Zhou, Citation2021). Since 1963, fifteen earthquakes with magnitudes larger than five have been recorded (Liu and Wang, Citation2020), including the Luhuo Ms 7.6 earthquake on February 6, 1973 and the Daofu Ms 6.9 earthquake on January 24, 1981 (). The lithology types are diversified from Paleozoic to Mesozoic rocks. Triassic tuffs and slates dominate the largest part of the study area. Granite and amphibolite are widely distributed in the central parts, and Jurassic siltstones and mudstones are found in the western area. The river valleys are characterized by Quaternary alluvial deposits and debris flow deposits. The rocks in most parts of the river valleys are strongly weathered, providing sufficiently loose and broken solid materials for debris flows.
Figure 3. Regional geological background and debris flow distribution map: I Yangtze block; I1 Upper Yangtze block; II Qiangtang-Sanjiang orogenic system; II1 Yulongtage-Bayan Har foreland basin; II2 Garze-Litang arc-basin system; II3 Zhongza block; II4 Jinsha River-Ailao Mountain suture zone; II5 Changdu-Lanping block; III Bangong-Shuanghu-Changning suture system; III1 Zuogong block; III2 Bangong-Nujiang suture zone (Shen and Ly. Citation1982; Tang and Huang. Citation1999 ; Pan et al. Citation2012).

3. Data and methods
3.1. Data source and pre-processing
3.1.1. Debris flow inventory and the impacts on road
Through field survey and image interpretation, 235 catchments with historical debris flow activities induced by rainfall on both sides of the highway were investigated. The debris flow inventory map is shown in . We classified the debris flow catchments into three groups according to the occurrence frequency as follows: high (where debris flow has occurred at least once in the last five years), moderate (at least once in the last 5–50 years), and low (at least once in the last 100 years). We identified 66, 67, and 102 catchments with high, moderate, and low frequency in terms of debris flow events, indicating different degrees of danger. Among them, 136 debris flows had a direct impact on the highway.
The infrastructures of highways that were potentially impacted by debris flow included bridges, culverts, roadbeds, and pavements. Statistical field investigations demonstrated that 44% of the 136 debris flows affected the culverts, 39% influenced the bridges, and 17% impacted the roadbeds and buried the pavements. The damage types were summarized as those that scoured and destroyed the roadbeds, blocked the culverts or bridges, and buried the pavements ().
Figure 4. Debris flow damage to the highway: (a) The trunk stream eroded the roadbeds; (b) Debris flow scoured the culverts; (c) Debris flow materials buried the pavements; (d) Debris flow materials blocked the bridges.

The basic data for constructing the assessment index of debris flow included digital elevation model (DEM) data with 30 m resolution, geological maps with a scale of 1:500,000, China’s ground motion parameter zoning map, meteorological data, and global land cover with 10 m resolution. Further, we obtained the road data with a scale of 1: 250,000 from the National Basic Geographic Database ().
Table 1. Data source.
3.1.2. Indexes of hazard assessment
Based on previous research, we selected six indexes representing the environment and causative factors of debris flow, including slope, lithology, distance from the fault, seismic acceleration, annual average rainfall and land cover for hazard assessment (Berenguer et al. Citation2014; Yin and Zhang, Citation2018; Zou et al. Citation2019; Yang et al. Citation2020). The Spatial distribution of six indexes is shown in . Among them, slope and land cover represented the topographic and geographical conditions. Lithology, distance to fault, and seismic acceleration were utilized to represent the geological conditions, and annual average rainfall was used to represent the climate conditions.
Figure 5. Spatial distribution of hazard assessment indexes: (a) slope angle; (b) rainfall; (c) fault distance; (d) Peak ground acceleration (PGA); (e) lithology; (f) land cover.

3.1.2.1. Terrain slope
Terrain slope is one of the essential factors that determine the dynamic distribution inside the slope and controls the stability of the slope, affecting the formation of landslides and other types of loose materials. According to the field investigations, the areas with a slope smaller than 15° were regarded as debris flow accumulation areas; the areas with a slope ranging from 15° to 45° were considered as debris flow formation regions. It is generally difficult to preserve loose materials in areas with a slope greater than 45° (Wu et al. Citation2019). Therefore, the slope was divided into five grades: < 15°, 15°–25°, 25°–35°, 35°–45° and >45° (Wu et al. Citation2019; Zhao et al. Citation2021) using the spatial analysis technology of ArcGIS based on the DEM data. shows the statistical results of different slope classes. Slopes had different contributions to debris flow formation according to the weight analysis (). The slope within the range of 25°–35° was positively correlated with the formation of debris flow.
Table 2. Result of evidence weight of hazard assessment index.
3.1.2.2. Average annual rainfall
Rainfall is not only one of the triggering factors of debris flow but also provides hydrodynamic conditions for debris flow activities and participates in its formation (Berenguer et al. Citation2014; Jomelli et al. Citation2019). The rainfall was divided into five grades within the study area: <550, 550–600, 600–700 and >700 mm. displays the statistical results. By analyzing the evidence weight, we found that there was no clear correlation between the distribution of debris flow and average annual rainfall ().
3.1.2.3. Distance to faults
The study area is significantly affected by the strong regional geological tectonic activity resulting from the Xianshui River Fault and its dense secondary faults (Wen et al. Citation2008; Bai et al. Citation2018; Qiao and Zhou, Citation2021). In the catchments close to the faults, weathered metamorphic rocks such as mylonite and cataclastic rocks are often well-developed, easily fail, and indirectly provide loose materials for debris flow. Therefore, the distance to faults in the study area was constructed by using ArcGIS spatial analysis tools. The area was divided into four levels: < 0.5, 0.5–1, 1–2 and 2–5 km (). Based on the evidence analysis in , all values of C were negative. As far as the interior of the study area was concerned, there was no obvious difference in faulting effects.
3.1.2.4. Peak ground acceleration
The study area is also significantly affected by frequent earthquakes. The strong earthquake disrupted the structure of rock and soil, which reduced strength and resulted in a landslide, and provided loose materials for debris flows (Yu et al. Citation2014; Hu et al. Citation2019). Peak ground acceleration (PGA) is used to represent seismic activity. The study area was classified into four PGA zones of: 0.1, 0.15, 0.2 and 0.3 g according to the Zoning Map of China’s Ground Motion Parameters (GB18306-2015). The statistical results are shown in . PGA had a positive correlation with the formation of debris flow, and the areas with 0.3 g had the largest C ().
3.1.2.5. Lithology
The indexes of lithology in the study area were classified and quantified according to the severity of weathering and the degree of rock fragmentation from the Standard for Engineering Classification of Rock Mass (GB/T50218-2014). The statistical results are shown in and the indexes weights are presented in . Similar to previous research results (Jomelli et al. Citation2019; Zhao et al. Citation2020), the intrusive rocks (1), carbonates (4), ophiolite mélange (6), and quaternary loose deposits (7) were negatively correlated, while the metamorphic rocks (3) and soft clastic rocks (5) were positively correlated with the formation of debris flow. Among them, the shallow metamorphic rock group had the largest C and the highest correlation.
3.1.2.6. Surface land cover
Vegetation can effectively enhance soil strength and prevent soil erosion. Eight types of land cover were classified in the study area (Gong et al. Citation2019) including crop, forest, grass, shrub, wetland, water, impermeable surface, and bareland details the study area was dominated by forest, shrubs and grass. Forest and shrubs were predominantly located on shady slopes with steep topography and low evaporation. Thus, they were prone to landslide-type hazards and produced more loose materials. On the contrary, grassland was located on the plateau with gentle terrain and sufficient light, usually where loose materials were less likely to form.
3.1.3. Indexes of vulnerability assessment
The elements affecting the vulnerability of the disaster-affected objects in the study region included three aspects: the exposure of disaster-affected objects, the attributed characteristics of highway structures, and post-disaster resilience (Zou et al. Citation2018; Dave et al. Citation2021; Zhu et al. Citation2023).
Therefore, taking highway structures and vehicles as objects, the exposure of the highway (I), the structural attributes (II), the disaster recovery capacity (III), and the exposure of the vehicle (IV) were chosen as primary indexes. Category (I) considered the relative position and the angle between the highway and debris flow channel (I1-I2). Category (II) included the dimensional structures of the highway (II1–II7). Category (III) considered the current status of highway damage and recovery cost (III1–III2), and category (IV) referred to the exposure probability of vehicles (IV1). Referring to the classification standard of highway structure parameters and the actual situation in the study area (Xu et al. Citation2010; Cui et al. Citation2013; Xu et al. Citation2016; Zou et al. Citation2018), the quantitative classification results are shown in .
Table 3. Evaluation index classification and vulnerability rating.
Based on the results of the field survey and the technical standards of road engineering (JTG D20-2017), the maximum traffic flow and speed limits for the different grades of highways were attained (). Then the vehicle travel time and distribution density were calculated, respectively, and the exposure probability value of the disaster-affected mobile object was calculated using EquationEq. (13)(13)
(13) . shows the processing results.
Table 4. Design specifications for different grades of road.
Table 5. Vulnerability value table of the disaster-affected mobile object.
3.2. Risk assessment methods
Risk analysis of debris flow consists of two procedures as mentioned already: the hazard assessment of debris flows and the vulnerability assessment of traffic lines. The ultimate result is quantitatively expressed by risk degrees (United Nations, Citation1991). According to the definition, the following equation is obtained:
(1)
(1)
where R is the risk degree (0–1), H is the hazard degree (0–1), and V is the vulnerability degree (0–1).
3.2.1. Hazard
The weight-of-evidence method based on Bayesian conditional probability (Agterberg, Citation2011) was utilized in this study. This method takes the known and discrete debris flow events as training samples and factors related to events (such as evaluation indicators) as evidence factors. The contribution values of each evidence factor to the occurrence of events are calculated as weights, and the possibility of target events in the evaluation unit is expressed by posterior probability (Zheng et al. Citation2021b). Based on the spatial relationship between evidence factors and training samples, the greater the posterior probability, the greater the possibility of events.
The specific process is as follows:
First, the distribution density is calculated as the prior probability according to the ratio between the debris flow area and study area (EquationEqs. (2)(2)
(2) and Equation(3)
(3)
(3) ); Second, the selected evidence factors are reclassified and then coupled with debris flow to calculate the contribution (weight, Wj) of each classification level to debris flow occurrence. The value of C indicates the correlation between the index and debris flow. The larger the C, the greater the relation (EquationEqs. (4)–(10)); Finally, the posterior probability is calculated based on the weight of the influencing factors (EquationEqs. (11)
(11)
(11) and Equation(12)
(12)
(12) ).
The calculation formulas are as follows:
① Calculation of the prior probability:
(2)
(2)
(3)
(3)
② Calculation of the index weight:
(4)
(4)
(5)
(5)
(6)
(6)
(7)
(7)
(8)
(8)
(9)
(9)
(10)
(10)
③ Calculation of the posterior probability:
(11)
(11)
(12)
(12)
where SD denotes the area of debris flow basin along the highway, SL stands for the study area evaluated in this research, SBjD represents the area of intersection of the j index layer and debris flow basin, SBj signifies the area of index layer of j, and Wj+ and Wj− indicate the weight values of the existence or absence of evidence factors, respectively. The higher the value of Wj+, the greater the possibility of a debris flow. If the original data is missing, the weight value is 0, and C symbolizes the correlation degree between each evidence factor (i.e. evaluation index) and evidence layer (i.e. debris flow). Finally, the calculated Wj+ is assigned to the evidence factor, and Wj− is assigned to the remaining other classification levels of this factor. In GIS, the assigned vector layer is transformed into a raster, and the raster calculator in spatial analysis is used to calculate the posterior probability. The Ppost value is between 0 and 1, reflecting the magnitude of the debris flow hazard. The hazard zoning can be performed in the study area according to this value.
3.2.2. Vulnerability
Aiming at the linear highway, our vulnerability evaluation objects included disaster-affected static objects (i.e. the highway structures) and disaster-affected mobile objects (i.e. the vehicles). Therefore, the field investigation is an important step in collecting the primary data for assessing the vulnerability, including the highway’s design parameters and the spatial relationship between debris flow catchment and highway infrastructure.
Because of the randomness and uncertainty of disaster-affected mobile objects, it is difficult to evaluate their vulnerability. However, the disaster-affected mobile object that appears in the affected range of debris flow is independent, and its probability is only related to the traffic intensity (i.e. to the distribution density of vehicles along the highway) and the transit time required to pass the impacted areas. Therefore, we employed the vehicle’s exposure probability under the maximum traffic flow and the maximum speed limit of different highway grades. These threshold values were obtained from design specifications for highway alignment (JTG D20-2017) in the study area to express the vulnerability of disaster-affected mobile objects. The formula for calculating the exposure probability of a disaster-affected moving object is as follows (Zou et al. Citation2018):
(13)
(13)
where λ is the distribution density of vehicles (vehicles/km), P(k ≥ 1) is the exposure probability of vehicles in the debris flow disaster area, k is the number of vehicles, and t is the passing time of vehicles which depends on the passing speed and distance.
Since the route was too long to calculate, we discretized an ‘infinite’ line into a ‘finite’ single-point line based on the idea of ‘discretization’ in the finite element method. It is clear that if more points are discretized, more accurate data will achieve. However, considering the actual distribution of debris flow and its operability, the discretization was carried out in each unit of 500 m. The length of the route in the study area was about 891 km which was discretized into 1702 calculation units by ArcGIS. The vulnerability of each debris flow hazard point can be calculated according to the evaluation indexes, and then it is assigned to the corresponding evaluation unit within its impact range, otherwise, the vulnerability value is zero.
Analytic hierarchy process (AHP) was proposed to systematize a target problem hierarchically. AHP is used to determine the importance and weight coefficient of the index in the evaluation of landslide or debris flow (Sun et al. Citation2021). First, the problem was decomposed into multiple sub-layers. Second, the pairwise comparison matrix was established, and then the weight of each element was calculated. The quality of comparison was described by the consistency ratio (CR). The weighted result was considered acceptable when the CR was smaller than 0.1 (Karanik et al. Citation2016).
The multi-factor comprehensive evaluation method was employed to establish the vulnerability evaluation model of the study area as follows:
(14)
(14)
where V denotes the total vulnerability of the highway, Ui stands for the weight value of the ith vulnerability index of the disaster-affected object, and Xi represents the vulnerability value of the ith vulnerability index of the disaster-affected object.
The judgment matrix was constructed by the aforementioned steps of AHP. The calculated result was CR = 0.0460 < 0.1, which satisfied the consistency test. depicts the weights of vulnerability indexes. Through EquationEq. (14)(14)
(14) and by using the weight of the vulnerability index in , the vulnerability evaluation model was constructed as follows:
(15)
(15)
where V is the vulnerability degree of the highway, and Xi is the vulnerability value of the ith vulnerability index of the highway.
Figure 7. The weights of vulnerability indexes of the northern line of the Sichuan-Tibet Highway: I1 The relative position between debris flow and highway; I2 The angle between the mainstream direction of debris flow channel and highway; II1 Bridge span; II2 Bridge clearance; II3 Pier foundation; II4 Culvert span; II5 Culvert clearance; II6 Longitudinal gradient of culvert bottom; II7 The height difference between roadbed and riverbed; III1 Pavement damage rate; III2 Recovery cost; IV1 Exposure probability of the disaster-affected mobile object.

4. Results
4.1. Hazard
The result of the debris flow hazard degree was calculated by ArcGIS. The values ranged from 0.0037 to 0.8023 and were classified into five grades: (I) very low hazard area, (II) low hazard area, (III) medium hazard area, (IV) high hazard area, and (V) very high hazard area. The hazard zoning map is shown in , and the results are shown in .
Table 6. Statistics and verification results of debris flow hazard zoning in the northern line of Sichuan-Tibet Highway.
The very low hazard (I) areas were mainly distributed from Garze County to Manigange Township (), with a total area of about 636.09 km2 accounting for 5.71% of the study area. The developed debris flow area in this region was 51.13 km2 (two basins), accounting for 1.23% of all debris flows (). The landscape of this region is a plateau with a gentle topography that is mostly smaller than 15°, which is not sufficient to provide dynamic force for the formation of debris flow.
The low hazard (II) areas were primarily located around the very low hazard (I) zones and were more dispersed (). The total area was about 762.98 km2, accounting for 6.85% of the study area. The area of the developed debris flow was 189.12 km2 (three basins), accounting for 4.55% of all debris flow (). The land cover of these areas is dominated by grassland with less human activity.
The medium hazard (III) areas were largely located from Tuoba Township to Qingnidong Township and from Dege County to Manigange Township (), with a total area of 4239.30 km2 accounting for 38.06% of the study area. The debris flow basin area was 1423.36 km2 (62 basins), accounting for 34.28% of the total basin ().
The high hazard (IV) areas were scattered around the Township of Tuoba in Changdu, the southern part of Luhuo County, and Xinduqiao Township (), with a total area of about 4140.19 km2 accounting for 37.17% of the study area. The debris flow basin areas covered 1740.12 km2 (140 basins), accounting for 41.91% of all the debris flows ().
The very high hazard (V) areas were about 1360.94 km2 accounting for 12.22% of the study area. The developed debris flow area in this region was 748.65 km2 (28 catchments), accounting for 18.03% of all debris flows (), which was chiefly distributed in Changdu City, Jiangda County, Dafu County and Luhuo County (). The stratigraphic lithology of this region is dominated by Jurassic mudstones and siltstones which are soft and hard clastic rocks with poor engineering geological properties. Daofu and Luhuo County are tectonically part of the Xianshui Fracture Zone with strong tectonic activity and fragmented rock masses. This leads to unstable landslides and abundant loose solid materials, resulting in large-scale and high-frequency debris flows.
To ensure the reasonableness and accuracy of the assessment result, it is necessary to verify it. Assume Si is the proportion of the area of the corresponding level i (i = I, II, III, IV and V) to the area of the whole study area, and Gi is the proportion of the area of debris flow in the corresponding level i to the total area of debris flow. A reasonable grade zoning Ri (Gi/Si) should meet the following requirements: RI < RII < RIII < RIV < RV. The results () show that the test meets these requirements, indicating that the hazard zoning result is reasonable.
The receiver operating characteristic (ROC) curve is a curve that was originally employed in military radar discrimination during World War II. It has been broadly utilized in clinical medical diagnosis, machine learning, and other fields for model validation and performance evaluation (Fawcett, Citation2006; Qin et al. Citation2019). The curve can directly reflect the advantages and disadvantages of the modeling method. We selected the area under curve (AUC) value to test the accuracy of the assessment result. The AUC value is an area enclosed by the ROC curve, which is acquired by changing the threshold of continuous variables to get sensitivity (true positive rate) as ordinate and specificity (false positive rate) as abscissa (Fawcett, Citation2006). The AUC value ranges from zero to one, and the closer to one, the higher the authenticity of the modeling method. displays the ROC curve result, the AUC value was estimated to be 0.838, suggesting that the hazard assessment result of debris flow was valid.
4.2. Vulnerability
According to the investigations, there were 136 debris flow hazard sites that potentially impacted the highway engineering projects, including 53 bridges and 60 culverts. First, the vulnerability characteristic parameters of highway structures and mobile bodies were assigned to each disaster point; then the vulnerability of each debris flow point was obtained by EquationEq. (15)(15)
(15) , and ultimately, the vulnerability of each disaster point was allocated to the linear unit within its corresponding impact range.
The final vulnerability result was 0–-0.6444. The vulnerability was also classified into five grades: (I) very low vulnerability, (II) low vulnerability, (III) medium vulnerability, (IV) high vulnerability, and (V) very high vulnerability. depicts the results and exhibits the statistics for each grade of the road section.
Table 7. Statistics of road vulnerable sections with different grades.
The length of the very low vulnerability (I) section was 163.63 km, accounting for 19.25% of the total length of the highway. The length of the low vulnerability (II) section was 130 km, accounting for 15.29%. And that of the medium vulnerability (III) section was 217.44 km, accounting for 25.58% of the whole road length, which was geographically close to the low vulnerability section. The length of the high vulnerability (IV) section was 244.07 km, accounting for 28.71% of the whole highway length, which was most widely distributed. The very high vulnerability (V) section covered 94.86 km and occupied 11.16% of the total highway length, with the least distribution compared to other sections.
4.3. Risk
Based on the results of the debris flow hazard and highway vulnerability evaluations, the risk results for the whole route were obtained and ranged from 0-0.5170. The results were also classified into five grades: very low risk (I), low risk (II), medium risk (III), high risk (IV), and very high risk (V). The risk zoning is shown in and .
Table 8. Statistics of road sections with different levels of risk.
Very low-risk section (I) was 171.52 km, accounting for 20.18% of the total length of the highway, mainly distributed in the eastern part of Tuoba Township, Manigange Township to Garze County, and Bami Township. The low-risk section (II) was close to the very low-risk section, with a length of 140.40 km, accounting for 16.52% of the total length of the highway. The medium-risk section (III) was 166.31 km, accounting for 19.57% with a fragmented distribution. The high-risk section (IV) was predominantly distributed in Jiangda County, Dege County, and Luhuo County to Daofu County, with a total length of 253.34 km, accounting for 29.80%. The very high-risk section (V) occupied 13.93% of the highway with a length of 118.43 km, which was centralized in Ritong Township and Zhuwu Township to Luhuo County. With the increase in risk grade, more debris flows were distributed; The number of debris flows was the largest in the high-risk section (88), while the distribution density of debris flows was the greatest in the very-high risk section (0.473).
The risk assessment results were validated by the field survey. We collected recent debris flow events (). Although this collection was incomplete, it did provide validation information.
Table 9. Statistics of typical disaster events in the study area.
All the listed debris flows events () were classified as very high or high hazard areas, and these sections were classified as middle or high vulnerability according to the vulnerability assessment results. Here we took the two following typical debris flow disasters as examples:
‘Moon Bay’ gully debris flows
‘Moon Bay’ gully covers an area of 1 km2 and is located at No. 1 and No. 2 Bridges in Moon Bay (317 National Highway, K480 + 100.2). The debris flow soil sources consisted of loose materials accumulated in slope erosions and unstable slopes along the channel (). Debris flow deposits have silted up the bridge (), posing a threat to the ‘317 National Highway’. According to the field investigations, because the main river became narrow under the squeezes of the alluvial fan, thus, it continuously washed the riverbank, where the surface gravel soil collapsed and developed two landslides (). Therefore, the debris flow-induced landslide on both sides of the Xianshui River constituted a disaster chain, threatening traffic safety. In our risk assessment, the hazard of the upstream area was mainly middle, and that of the downstream area was mostly high and very high. Moreover, the vulnerability of the highway was middle, which coincided with the damaged situation.
‘Juebusi’ gully debris flows
‘Juebusi’ gully covers an area of 111.28 km2 and is located in the city of Jiangda County. The debris flow soil sources were composed of loose materials accumulated in slope erosions and unstable slopes along the channel (). The scouring occurred upstream with a steeper slope, and the siltation occurred downstream with a gentle slope, bringing massive deposits and causing the channel to be blocked. Although there were some control projects in the channel, intense water runoff and scouring destroyed them (), posing a threat to the residential areas of the County and the traffic safety of ‘317 National Highway’. As shown in , the hazard result of the upstream area was predominantly middle (I-the green part), and that of the downstream area was principally high and very high (II-the red part), showing the obvious discontinuity. Based on the geological environment where the ‘Juebusi gully’ is located, the exposed strata in this catchment are both conglomerate and sandstone, without an obvious difference. However, owing to the effect of faults, the peak ground acceleration changed from 0.1 g to 0.15 g (), resulting in a discontinuity of upstream and downstream hazard. According to the survey, on July 8, 2017, after a heavy rainfall that lasted nearly ten days, a debris flow began suddenly, which washed out about 3.2 × 104 m3 of solid materials. This blocked the bridge of ‘317 National Highway’ and the Maiqu River and caused about ten million RMB in economic losses. Therefore, our evaluation result was valid and reliable.
5. Discussion
The hazard assessment indicated the effects of environmental conditions on debris flow formation. The results of the slope and lithology agreed with previous studies (Keefer, Citation2000; Kargel et al. Citation2016; Liu et al. Citation2021). The stimulation of debris flows was the biggest under slopes of 35–45°, and the slope instability and debris flow were more likely to occur in the case of the soft clastic rocks. It is important to note that the W+ values of fault distance in the study area were both smaller than zero, suggesting that the fault played a negative role in the formation of debris flow. Although it did not conform to common cognition that ‘debris flow development is gradually decreased with increasing fault distance’ (Wu et al. Citation2020), it conformed to the actual relationship between debris flow and fault location in the study area. The faults, road, and river were distributed in parallel, and floodplains or river terraces, which acted often as alluvial fans, were very close to the faults. The formation of debris flow is an event in the upper reaches of the basin, thus, the area where the fault distance is smaller than 0.5 km is not the most sensitive area for debris flow formation (Aditian et al. Citation2018; Wu et al. Citation2019). This also demonstrates that the ‘fault distance’ cannot simply be employed to reflect the controlling effect of fault structure on debris flow disaster, and therefore, further investigation is needed in this research field. There was no obvious regularity in the results of average rainfall classification. It showed that rainfall was a triggering factor that possibly stimulated debris flow after reaching a certain intensity. The formation of debris flow was also influenced by the subsurface (Zhang et al. Citation2014; Gao et al. Citation2016). Regarding the land cover, the W+ value of the forest was the largest because the forest covered a large area of shady slopes with steeper terrain. The grass and bareland were distributed on the plateau with gentle terrain, which was not conducive to the formation of debris flow. To summarize, the results of index weights indicated that the weight of the evidence method could reflect the spatial distribution relationship between each index and debris flow, but it did not necessarily reflect the formation mechanism of debris flow.
Artificial mitigation measures are significant to protect highway structures from debris flows. However, some of the design and maintenance aspects of the measures are not well conducted. For instance, the improper design of the measures includes the project’s inappropriate position and insufficient protection ability. In the study area, some of the bridges and/or culverts had an insufficient overflow ability, causing the debris flow deposits to block the aperture of the bridge (). The design standards of some measures were comparatively weak, resulting in insufficient protection ability (). Some debris flows develop beyond the drainage ditches and threaten the highway. Meanwhile, with the continuous accumulation of debris flows deposits, the bridges and/or culverts are blocked, decreasing the overflow capacity. This suggests that the cleaning of the deposits under the bridge and/or culvert is significantly important.
This study was a comprehensive assessment that simultaneously considered the natural attributes of debris flow and the vulnerability attributes of the highway. The ultimate risk was not only related to the danger of the debris flows but also related to the spatial location and structural attributes of the highway. For example, although the assessment showed that the risks of debris flow in the Touba-Qingnidong and Daofu-Bamei sections (III–IV) were both medium to high (), the positions of the road were reasonable, the structures were integrated, and the ditches were well designed. Therefore the risk was low ().
Compared with the southern line of the Sichuan-Tibet Highway, the northern line of the Sichuan-Tibet Highway lacked enough research and data and involved more difficulty in the fieldwork, which led to certain limitations of the assessment results of this study. Despite the limited data, we made a systematic and complete risk assessment based on the current situation of the highway as a typical debris flow disaster-affected object. The assessment results can be used as a theoretical reference for the prevention and mitigation of highway debris flow disasters.
6. Conclusion
In this study, a regional debris flow risk assessment framework was constructed from two perspectives: the natural attributes of the debris flow and the social attributes of linear engineering. During this process, an integrated model was built by considering the disaster causative factors and their corresponding weights to evaluate the hazard levels of debris flows. Combining the investigation with the remote sensing image interpretation and structure characteristics of the highway, their vulnerability was quantitatively analyzed using the spatial analysis method.
Specifically, we applied the proposed framework to the debris flow along the National Highway in Southwest China. Due to the spatial unevenness of disaster frequency and protection standards, the risk zoning map showed spatial inconsistency. The low-risk sections accounted for 20.18%, distributed in the central and southeast areas of the highway, which is a sparsely populated plateau. The high-risk road sections accounted for 13.93%, chiefly concentrated in the west and middle east areas like Changdu County, Luhuo County, and Daofu County, with higher disaster frequency and lower highway protection measures. The results were highly consistent with actual disaster events. Hence, monitoring and prevention of debris flow disasters in these high-level regions should be strengthened in future daily maintenance to reduce the damage.
Despite some limitations and uncertainty in the risk assessment, such as the temporal probability of the hazard scenario, and the range of disaster. This risk zoning map can indicate the spatial difference of highway overall risk and provide regional risk information in mountainous areas for decision-makers.
Disclosure statement
The authors declare that they have no known competing financial interests or personal relationships that could have appeared to influence the work reported in this paper.
Data availability statement
The data that support the findings of this study are available from the corresponding author, upon reasonable request.
Additional information
Funding
References
- Aditian A, Kubota T, Shinohara Y. 2018. Comparison of GIS-based landslide susceptibility models using frequency ratio, logistic regression, and artificial neural network in a tertiary region of Ambon, Indonesia. Geomorphology 318(1):101–111.
- Agterberg F. 2011. A Modified weights-of-evidence method for regional mineral resource estimation. Nat Resour Res. 20(2):95–101.
- Ali S, Haider R, Abbas W, Basharat M, Reicherter K. 2021. Empirical assessment of rockfall and debris flow risk along the Karakoram Highway, Pakistan. Nat Hazards. 106:2437–2460.
- Baggio T, Mergili M, D'Agostino V. 2021. Advances in the simulation of debris flow erosion: the case study of the Rio Gere (Italy) event of the 4th August 2017. Geomorphology 381:107664.
- Bai M, Chevalier ML, Pan J, Replumaz A, Leloup PH, Métois M, Li H. 2018. Southeastward increase of the late quaternary slip-rate of the Xianshuihe fault, eastern Tibet geodynamic and seismic hazard implications. Earth Planet Sci Lett. 485:19–31.
- Berenguer M, Sempere-Torres D, Hürlimann M. 2014. Debris-flow hazard assessment at regional scale by combining susceptibility mapping and radar rainfall. Nat Hazards Earth Syst. 2(10):6295–6338.
- Chen C, Tseng C, Dong J. 2007. New entropy-based method for variables selection and its application to the debris-flow hazard assessment. Eng Geol. 94(1-2):19–26.
- Chen XZ, Chen H, You Y, Chen XQ, Liu JF. 2016. Weights-of-evidence method based on GIS for assessing susceptibility to debris flows in Kangding County, Sichuan Province, China. Environ Earth Sci. 75(1):1–16.
- Ciurean RL, Hussin H, van Westen CJ, Jaboyedoff M, Nicolet P, Chen L, Frigerio S, Glade T. 2017. Multi-scale debris flow vulnerability assessment and direct loss estimation of buildings in the Eastern Italian Alps. Nat Hazards. 85(2):929–957.
- Cui P, Zhou G, Zhu X, Zhang JQ. 2013. Scale amplification of natural debris flows caused by cascading landslide dam failures. Geomorphology 182:173–189.
- Dash RK, Falae PO, Kanungo DP. 2022. Debris flow susceptibility zonation using statistical models in parts of Northwest Indian Himalayas-implementation, validation, and comparative evaluation. Nat Hazards. 111(2):2011–2058.
- Dave R, Subramanian SS, Bhatia U. 2021. Extreme precipitation induced concurrent events trigger prolonged disruptions in regional road networks. Environ Res Lett. 16(10):104050.
- Fawcett T. 2006. An introduction to ROC analysis. Pattern Recog Lett. 27(8):861–874.
- Gao L, Zhang LM, Chen H, Shen P. 2016. Simulating debris flow mobility in urban settings. Eng Geol. 214:67–78.
- Gong P, Liu H, Zhang M, Li C, Wang J, Huang H, Clinton N, Ji L, Li W, Bai Y, et al. 2019. Stable classification with limited sample: transferring a 30-m resolution sample set collected in 2015 to mapping 10-m resolution global land cover in 2017. Sci Bull. 64(6):370–373.
- He S, Wang D, Zhao P, et al. 2020. A review and prospects of debris flow waste-shoal land use in typical debris flow areas, China. Land Use Policy. 99:105064.
- Hu K, Wang X, Liu J, Wang Z, Tang J, Zhong W. 2020. Risk assessment of Sichuan-Tibet Highway susceptible to debris flows at Suotong Basin, southeastern Tibet. IOP Conf Ser: earth Environ Sci. 570(4):42051.
- Hu X, Hu K, Tang J, You Y, Wu C. 2019. Assessment of debris-flow potential dangers in the Jiuzhaigou Valley following the August 8, 2017, Jiuzhaigou earthquake, western China. Eng Geol. 256:57–66.
- Huang H, Wang Y, Li Y, Zhou Y, Zeng Z. 2022. Debris-Flow Susceptibility Assessment in China: a Comparison between Traditional Statistical and Machine Learning Methods. Remote Sens. 14(18):4475.
- Huang Y. 2021. Major engineering geological problems and countermeasures along traffic corridor from Ya’an to Nyingchi. J Eng Geol. 29(2):307–325.
- Jamali AA, Ghorbani Kalkhajeh R, Randhir TO, He ST. 2022. Modeling relationship between land surface temperature anomaly and environmental factors using GEE and Giovanni. J Environ Manage. 302:113970.
- Jiang H, Zou Q, Zhou B, Hu Z, Li C, Yao S, Yao H. 2022. Susceptibility Assessment of Debris Flows Coupled with Ecohydrological Activation in the Eastern Qinghai-Tibet Plateau. Remote Sens. 14(6):1444.
- Jomelli V, Pavlova I, Giacona F, Zgheib T, Eckert N. 2019. Respective influence of geomorphologic and climate conditions on debris-flow occurrence in the Northern French Alps. Landslides 16(10):1871–1883.
- Karanik M, Wanderer L, Gomez-Ruiz JA, Pelaez JI. 2016. Reconstruction methods for AHP pairwise matrices: how reliable are they? Appl Math Comput. 279:103–124.
- Kargel JS, Leonard GJ, Shugar DH, Haritashya UK, Bevington A, Fielding E, Fujita K, Geertsema M, Miles E, Steiner J. 2016. Geomorphic and geologic controls of geohazards induced by Nepal’s 2015 Gorkha earthquake. Science 351:6269.
- Kazakova E, Lobkina V, Gensiorovskiy Y, Zhiruev S. 2017. Large-scale assessment of avalanche and debris flow hazards in the Sakhalin region., Russian Federation. Nat Hazards. 88:237–251.
- Keefer DK. 2000. Statistical analysis of an earthquake-induced landslide distribution-the 1989 Loma Prieta, California Event. Eng Geol. 58(3-4):231–249.
- Kuo Y, Yang T, Huang GW. 2008. The use of grey relational analysis in solving multiple attribute decision-making problems. Comput Ind Eng. 55(1):80–93.
- Li Y, Chen J, Zhang Y, Song S, Han X, Ammar M. 2020. Debris flow susceptibility assessment and runout prediction: a case study in Shiyang Gully, Beijing, China. Int J Environ Res. 14(3):365–383.
- Liu GN, Wang Z. 2020. Correlation of the deep structural characteristics, tidal stress variation and earthquake initiation along the Xianshuihe-Anninghe fault zone. Chin J Geophys. 63(03):928–943.
- Liu L, Gao W, Xu C, Chen J. 2021. Susceptibility assessment of debris flow from Baoxing River basin in Lushan earthquake zone, China. IOP Conf Ser: earth Environ Sci. 861(5):52010.
- Martha TR, van Westen CJ, Kerle N, Jetten V, Vinod Kumar K. 2013. Landslide hazard and risk assessment using semi-automatically created landslide inventories. Geomorphology 184:139–150.
- Meyer NK, Schwanghart W, Korup O, Nadim F. 2015. Roads at risk: traffic detours from debris flows in southern Norway. Nat Hazards Earth Syst Sci. 15(5):985–995.
- Nie YP, Li XZ, Zhou W, Xu RC. 2021. Dynamic hazard assessment of group-occurring debris flows based on a coupled model. Nat Hazards. 106(3):2635–2661.
- Pan G, Wang L, Li R, Yuan S, Ji W, Yin F, Zhang W, Wang B. 2012. Tectonic evolution of the Qinghai-Tibet Plateau. J Asian Earth Sci. 53:3–14.
- Papathoma-Köhle M, Gems B, Sturm M, Fuchs S. 2017. Matrices, curves and indicators: a review of approaches to assess physical vulnerability to debris flows. Earth Sci Rev. 171:272–288.
- Qiao X, Zhou Y. 2021. Geodetic imaging of shallow creep along the Xianshuihe fault and its frictional properties. Earth Planet Sci Lett. 567:117001.
- Qin SW, Lv JF, Cao C, Ma ZJ, Hu XY, Liu F, Qiao SS, Dou Q. 2019. Mapping debris flow susceptibility based on watershed unit and grid cell unit: a comparison study. Geomat Nat Hazards Risk. 10(1):1648–1666.
- Santi PM, Hewitt K, Vandine DF, Cruz EB. 2011. Debris-flow impact, vulnerability, and response. Nat Hazards. 56(1):371–402.
- Shang Y, Park H-D, Yang Z. 2005. Engineering geological zonation using interaction matrix of geological factors: an example from one section of Sichuan-Tibet Highway. Geosci J. 9(4):375–387.
- Shen J, Ly YY. 1982. Earthquake Situation, Economic Loss and Disaster Relief Measures of Daofu Ms 6.9 Earthquake in Sichuan, China. Recent Developments in World Seismology. 09:22–23 + 30.
- Sullivan JL, Novak DC, Aultman-Hall L, Scott DM. 2010. Identifying critical road segments and measuring system-wide robustness in transportation networks with isolating links: a link-based capacity-reduction approach. Transport Res Part A: Policy Pract. 44(5):323–336.
- Sun X, Zhang G, Wang J, Li C, Wu S, Li Y. 2021. Spatiotemporal variation of flash floods in the Hengduan Mountains region affected by rainfall properties and land use. Nat Hazards. 111(1):465–488.
- Tang RC, Huang ZZ. 1999. Seismological Investigation Notes in Luhuo Earthquake, Celebration of the 20th anniversary of the seismological society of China. Beijing China. 534–538.
- United Nations. 1991. Mitigating natural disasters: phenomena, effects and options: a manual for policy makers and planners. New York UN.
- Utasse M, Jomelli V, Grancher D, Leone F, Brunstein D, Virmoux C. 2016. Territorial accessibility and decision-making structure related to debris flow impacts on roads in the French Alps. Int J Dis Risk Sci. 7(2):186–197.
- Wang N, Pu K. 2018. Grey relational analysis of geological hazards and urbanization construction in China. IOP Conf Ser: mater Sci Eng. 381(1):12012.
- Wang WD, Li JY, Han Z. 2020. Comprehensive assessment of geological hazard safety along railway engineering using a novel method: a case study of the Sichuan-Tibet railway, China. Geomat Nat Hazards Risk. 11(1):1–21.
- Wen XZ, Ma SL, Xu XW, He YN. 2008. Historical pattern and behavior of earthquake ruptures along the eastern boundary of the Sichuan-Yunnan faulted-block, southwestern China. Phys Earth Planet Inter. 168(1-2):16–36.
- Winter MG, Wong JCF. 2020. The assessment of quantitative risk to road users from debris flow. Geoenviron Dis. 7(1):1–19.
- Wu S, Chen J, Xu C, Zhou W, Yao LH, Yue W, Cui ZJ. 2020. Susceptibility assessments and validations of debris-flow events in meizoseismal areas: case study in China’s Longxi River watershed. Nat Hazard Rev. 21(1):5019005.
- Wu S, Chen J, Zhou W, Iqbal J, Yao L. 2019. A modified Logit model for assessment and validation of debris-flow susceptibility. Bull Eng Geol Environ. 78(6):4421–4438.
- Xu LR, Wang L, Su ZM. 2010. Assessment of engineering vulnerability of tunnel suffering from debris flow. Rock and Soil Mechanics. 31(7):2153–2158.
- Xu SB, Qian DL, Yao LF, Fang CJ, Liu J. 2016. Evaluation of vulnerability of suffering from debris flow disasters. Bull Soil Water Conserv. 36(05):235–241. +247.
- Yan S, He S, Deng Y, Liu W, Wang D, Shen F. 2020. A reliability-based approach for the impact vulnerability assessment of bridge piers subjected to debris flows. Eng Geol. 7(1):1–19.
- Yang HZ, Zhao Y, Cheng QF. 2020. Geohazards regionalization along highways in Shandong province, China. Geomatics Nat Hazards Risk. 11(1):1760–1781.
- Yin C, Zhang JL. 2018. Hazard regionalization of debris-flow disasters along highways in China. Nat Hazards. 91(1):129–147.
- Yu B, Wu Y, Chu S. 2014. Preliminary study of the effect of earthquakes on the rainfall threshold of debris flows. Eng Geol. 182:130–135.
- Yuan Y, Ning ZJ, Zuo ZH, Zhou AH, Liu C. 2022. Problems related to single gully debris flow assessment models. Nat Hazards. 110:1527–1544.
- Zeng C, He N, Song GH. 2012. Analysis and assessment of methods to assess vulnerability of building in debris flow hazard. Adv Earth Sci. 27(11):1211–1220.
- Zhang G. 2016. Research on the mountain disaster and geological alignment fundamental of Sichuan-Tibet railway running through N-S mountain area. J Railway Eng Soci. 33(2):21–24. +33.
- Zhang S, Yang H, Wei F, Jiang Y, Liu D. 2014. A model of debris flow forecast based on the water-soil coupling mechanism. Earth Sci J. 25(4):757–763.
- Zhang S, Zhang L, Li X, Xu Q. 2018. Physical vulnerability models for assessing building damage by debris flows. Eng Geol. 247:145–158.
- Zhao Y, Meng X, Qi T, Chen G, Li Y, Yue D, Qing F. 2021. Modeling the spatial distribution of debris flows and analysis of the controlling factors: a machine learning approach. Remote Sens. 13(23):4813.
- Zhao Y, Meng X, Qi T, Qing F, Xiong M, Li Y, Guo P, Chen G. 2020. AI-based identification of low-frequency debris flow catchments in the Bailong River basin, China. Geomorphology 359:107125.
- Zheng Q, Lyu HM, Zhou AN, Shen SL. 2021. Risk assessment of geohazards along Cheng-Kun railway using fuzzy AHP incorporated into GIS. Geomat Nat Hazards Risk. 12(1):1508–1531.
- Zheng Y, Xie YZ, Long XJ. 2021. A comprehensive review of Bayesian statistics in natural hazards engineering. Nat Hazards. 108(1):63–91.
- Zhu W, Liu K, Wang M, Nirandjan S, Koks EE. 2023. Improved assessment of rainfall-induced railway infrastructure risk in China using empirical data. Nat Hazards. 115(2):1525–1548.
- Zou Q, Cui P, He J, Lei Y, Li S. 2019. Regional risk assessment of debris flows in China-An HRU-based approach. Geomorphology 340:84–102.
- Zou Q, Cui P, Zhou GG, Li S, Tang J, Li S. 2018. A new approach to assessing vulnerability of mountain highways subject to debris flows in China. Prog Phys Geogr earth Environ. 42(3):305–329.
- Zou Q, Zhou GG, Li S, Ouyang C, Tang J. 2018. Dynamic process analysis and hazard prediction of debris flow in eastern Qinghai-Tibet plateau area-a case study at Ridi Gully. Arctic Antarctic Alpine Res. 49(3):373–390.