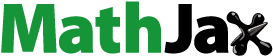
Abstract
Drought monitoring is a complicated issue as it requires multiple meteorological variables to monitor and anticipate drought accurately. Therefore, developing a method that enables researchers, data scientists, and planners to comprehend drought mitigation policies more accurately is essential. In this research, based on the concepts behind the calculation of the Standardized Precipitation Evapotranspiration Index (SPEI), a new drought index is proposed for regional drought monitoring: the Modified Standardized Precipitation Evapotranspiration Index (MSPEI). The potential of the proposed index is based on the estimation of Reference Evapotranspiration (ETo). Therefore, the Modified Hargreaves-Samani (MHS) equation based on fuzzy logic calibration is used to estimate ETo. The proposed index is validated on ten meteorological stations in Pakistan at a one-month time scale. Afterward, based on the Pearson correlation, the performance of the proposed index is compared with the commonly used drought index (SPEI). Results showed a significant correlation (r > 0.7) between the quantitative values of MSPEI and SPEI for all ten stations. Moreover, a modified Tjostheims coefficient is used to estimate and test the spatial correlation between SPEI and MSPEI for different drought classes. According to our findings, the association between the SW, ND, ED, EW, MW, and SD patterns of MSPEI and SPI is 0.74, 0.834, 0.673, 0.592, 0.393, and 0.434, respectively. Meanwhile, considering the significance of future drought trend detection, this research is further extended to detect the future trend of MSPEI by using the Hurst index. In accordance with the results, Bahawalnagar, Sialkot, Lahore, Kotli, and Gilgit all have HI values greater than 0.5 (0.63, 0.58, 0.56, 0.55, and 0.53, respectively). In contrast, Muzaffarabad, Skardu, and Jhelum have HI values 0.47, 0.45 and 0.38, respectively; however, HI values of 0.5 are observed at Dera Ismail Khan (DIK) and Islamabad. Therefore, this research provides a basis for developing and enhancing drought hazard characterization, encouraging researchers and policymakers to monitor and forecast regional droughts using a more accurate drought index.
1. Introduction
Drought is one of the most expensive but least understood natural calamities and causes a substantial impact on natural systems, water supplies, socio-economics, and ecosystems (Jiao et al. (Citation2019); Bayissa et al. (Citation2015)). Although there is no universal definition of drought, in its simplest form, drought has been defined as a scarcity of water, which may occur over a prolonged period in several climatic locations. Unlike floods with a clear start and a sudden end, drought can be characterized by slow progress and long-lasting influences (West et al. (Citation2019)).
Generally, drought is divided into four categories: meteorological, hydrological, agricultural, and socio-economic drought. Meteorological drought is caused by a shortage of rainfall, whereas a lack of stream flow, groundwater, or overall water storage causes hydrological drought. The deficit of moisture in the soil is a critical source of agricultural drought. On the other hand, the demand and supply of water and other societal responses can lead to socio-economic droughts. In addition to the types, drought can also be categorized by severity, intensity, duration, and area extent (Shah and Mishra (Citation2020)).
Since the turn of the century, several droughts have occurred in many regions across the globe with profound effects on agriculture, socio-economic development, and the ecosystem, e.g. Australia (2000-2009), USA (2000-2016), China (2007-2012) and Europe (2007-2010). Moreover, its consequences in famines and crop failures cause each year 6-8 billion dollars (on average) of worldwide economic losses (Chao et al. (Citation2016); Cook et al. (Citation2016)).
Over the past few eras, drought has become more dangerous and fatal in many regions of the world, especially in developing countries like Pakistan, such as; substantial water and food insecurities, which leads to economic losses and financial risks. Furthermore, the drought poses enormous drinking and irrigation water challenges and affects Pakistan’s economy, where agriculture provides a living for more than 60% of the population (Jiao et al. (Citation2019)). Therefore, finding an effective drought monitoring system that integrates many aspects (types and characteristics) of drought at the regional and global scales is essential. Various studies (Wang et al. (Citation2016); Wang et al. (Citation2012); Huang et al. (Citation2016)) have been conducted to monitor and forecast the drought effectively. However, the algorithms of each drought indicator vary by zone.
Moreover, drought indicators based on a single meteorological variable are not sufficient to monitor drought precisely because many factors (rainfall, humidity, wind speed, and temperature) are involved in drought (Shah and Mishra (Citation2020)). Initially, Palmer (Citation1965) introduced a drought index, namely, Palmer Drought Severity Index (PDSI), based on rainfall, temperature, latitude, and available water to characterize and quantify the drought. However, later studies showed that PDSI is unable to compare areas due to the lack of a multi-scaling quality. In contrast to PDSI, the Standardized Precipitation Index (SPI), was proposed by McKee et al. (Citation1993). The SPI is amongst the most extensively used drought indicators and can represent the drought classification states over various time intervals. However, an issue was observed in SPI, i-e. SPI considered only precipitation as an input variable, while many other relevant variables such as temperature, wind speed and Reference Evapotranspiration (ETo), etc., are not considered in manipulation for drought monitoring (Montazeri et al. (Citation2015)); (Arsenault and Brissette (Citation2014)). Parallel to SPI, Tsakiris et al. (Citation2007) proposed Reconnaissance Drought Index (RDI), incorporating ETo to characterize drought. Similarly, Vicente-Serrano et al. (Citation2010) proposed the Standardized Precipitation Evapotranspiration Index (SPEI), and the multivariate characteristics of SPEI make it more adequate and superior to SPI, which considers only one climatic variable (i.e. only rain). Thus, SPEI has been extensively used in various research fields, such as investigations of drought variability, drought reconstruction, global warming, and the drought’s impact on hydrology, agriculture, and the environment (Beguería et al. Citation2014). But, in the original proposal of SPEI, Vicente-Serrano et al. (Citation2010) used Thornthwaite (TH) equation to estimate ETo (i.e. Penman-Monteith equation (Task Committee on Revision of Manual 70 70 (Citation2016)). However, one of the major flaws of the TH equation is that it produces undefined ETo values in low-temperature areas (Van Wijk and De Vries Citation1954; Papadopoulou et al. (Citation2003)). Furthermore, the TH equation underestimates ETo in dry and semi-arid locations but overestimates ETo in semi-humid and wet areas (Van der Schrier et al. (Citation2011)).
Therefore, to overcome the abovementioned issues, this research intends to develop and evaluate a new index: the modified Standardized Precipitation Evapotranspiration Index (MSPEI). In MSPEI, the Modified Hargreaves-Samani (MHS) equation, proposed by Habeeb et al. (Citation2021), is used to estimate ETo. First, the parameters of the calibrated Hargreaves-Samani equation (ah, bh, ch, dh) are empirically estimated using the fuzzy logic interference system. However, it is important to note that, in this manuscript, we used averages instead of the difference between two-time steps (i.e. 0000 UTC and 1200 UTC) to calibrate the parameters. Because the differences might be helpful to estimate ETo for each of the instantaneous hours, but due to the extreme variations in metrological parameters, these instant hours do not accurately represent daily ETo.Therefore, daily ETo is recommended in most studies instead of particular hours, and similarly for other variables on which ETo depends. Thus, considering the importance of daily ETo, we used the two-time steps; average wind speed, humidity, and temperature in this research.
Thus, the proposed index’s capability and effectiveness are evaluated using Pearson correlation. Further, the modified Tjostheims coefficient is employed to estimate the spatial correlation between different patterns of SPEI and MSPEI. This research used the Lambert projection method in the Tjostheims coefficient to convert geographic coordinates into rectangular coordinates.
Although MSPEI is an excellent measure of meteorological drought monitoring, it cannot predict future drought patterns. In this context, using the Hurst exponent to forecast drought trends could be a useful technique for better analyzing and interpreting MSPEI time series (Tong et al. Citation2018; Vega et al. Citation2019; Zhang et al. Citation2021). Therefore, the Hurst index is used to predict the future spatiotemporal pattern of MSPEI.
Thus, from the above discussion, it can be concluded that several types of drought indicators have been suggested to monitor distinct types of drought (i.e. agriculture, meteorology, and hydrology) for diverse climatic zones. However, due to the instinctive selection of the probability distribution, geological attributes, and index parameters, there is always uncertainty regarding the precise classification of drought under different techniques (Stagge et al. (Citation2016)). Therefore, it is essential to find a technique that assists researchers, data scientists, and planners in understanding drought mitigation policies more precisely, especially at the provincial level. Thus, considering the importance of accurately estimating drought indices, this study focused on developing and evaluating MHS-based drought indices for drought monitoring under different climate conditions. Meanwhile, considering the importance of the spatial correlation between SPEI and MSPEI, the Modified Tjostheims coefficient is used to estimate and test the spatial correlation between SPEI and MSPEI. In addition, Hurst index based on Rescaled Range (R/S) analysis is used to predict the future trend of MSPEI.
2. Materials and methods
2.1. Standardized precipitation evapotranspiration index (SPEI)
Vicente-Serrano et al. (Citation2010) proposed a new multi-scale drought index SPEI, by considering more than one climatic variable. Both the SPEI and the SPI are calculated using the same mathematical expression. SPEI, on the other hand, has one major advantage over SPI: it considers ETo in the domain. The SPEI is also known as a water balance model. The mathematical expression of SPEI is:
(1)
(1)
Where Di, which represents the moisture deficit for month i, indicates the difference between the Pi and EToi. Pi represents total monthly precipitation, whereas EToi is the estimated amount of monthly evapotranspiration.
2.2. Modified standardized precipitation evapotranspiration index (MSPEI)
2.2.1. Phase 1: Estimation of reference evapotranspiration
2.2.1.1. Modified Hargreaves-Samani equation
The HS equation is simplified by not considering the effects of humidity and wind speed on the ETo rate. As a result, under extreme weather conditions, the original HS equation cannot generate accurate location estimates (Patel et al. (Citation2015)). Therefore, the Fuzzy Inference System (FIS) is used in this study to compute the updated values of HS parameters (ah, bh, ch) based on the type of location, such as dry, semi-arid, humid, semi-humid, cold, and so on. The input variables are: WS and Hum represent the average of wind speed and relative humidity observed at 1200 UTC and 0000 UTC, respectively, whereas Temp represents the mean of maximum and minimum temperature and sunshine hours. illustrates how FIS is conceptually organized. For example, the MHS equation is shown as:
(2)
(2)
Where, Tmax and Tmin are maximum and minimum temperatures, Teff = dh × ((3 * Tmax) – Tmin)/2 (dh is a parameter whose value is also determined by FIS.), Rs denotes extra-terrestrial solar radiation, estimated according to Allen et al. (Citation1998). depicts the block diagram for the fuzzy logic-based calibration of the MHS parameters. For a complete description of FIS, see Habeeb et al. (Citation2021).
2.2.2. Phase 2: Standardization of index
This phase is concerned with the standardization of the index. The step-by-step procedure is described as follows: The modified water balance model is computed for each selected station in the first step, depending on the difference between precipitation and ETo(MHS).
(3)
(3)
In the second step, various probability distributions (more specifically, 32 of the most commonly used) are fitted on MDi, e.g. Gamma, Logistic, Normal, Laplace, and Gumbel distributions. The propagate R package (Spiess et al. (Citation2018)) is used to apply the Kolmogorov-Smirnov test (Hassani and Silva (Citation2015)) and Anderson Darling tests (Ghosh et al. (Citation2016)) for the goodness of measure. The distributions with the lowest values of Akaike Information Criteria (AIC) and Bayesian Information Criteria (BIC) are then chosen in the third stage. Next, the parameters of each well-fitted distribution are estimated using the appropriate parameter estimation method with the lmom R package. The fourth step of the process involves determining the Empirical Cumulative Distribution Function (ECDF) using the estimated parameters of each well-fitted distribution.
(4)
(4)
In order to eliminate the impact of zero values in the MDi, a little modification in the CDF is done in Equationequation (4)(4)
(4) , e.g. in the case of a Gamma distribution. p is the probability of zero value in the time series data MDi for each station. If h is the total number of zeros in the MDi sequence, then p can be calculated using h/T. Where, T denoted the total number of observations in the MDi time series.
Finally, the ECDF of the probability distribution is converted into a standardized normal distribution with parameters 0 and 1. The approximate transformation method which is proposed by Abramowitz and Stegun (Abramowitz et al.(2012)) is used in this research. Here Zit is expressed as:
(5)
(5)
Here,
When,
Similarly,
(6)
(6)
And for,
When,
Where, = 2.515517,
= 0.802853,
= 0.010328,
= 1.432788,
= 1.432788,
= 0.985269,
= 0.001308.
2.3. Modified Tjostheims coefficient
Tjostheims coefficient is a non-parametric coefficient, initially introduced by Tjøstheim (Citation1978) and then generalized by Hubert et al. (Citation2010). In a spatial statistic term, Tjostheims coefficient is an index of correlation between two spatial sequences. A standard form of the observed value of the Tjostheims index is given by:
(7)
(7)
Where; denotes some appropriate measure of spatial distance,
designates the degree to which homogeneous rank values on F and G occupy spatially close geographical locations. In addition, the untied ranks from
to
serve as the definitions of
and
the position of rank
on
and
on
are indicated by
and
respectively,
In Equationequation 7
(7)
(7) , the location
is represented by two coordinates
) (latitude and longitude of the location
), and
by (
) (latitude and longitude of the location
If
is the distance between two points and is defined as the square of the Euclidean distance, then;
(8)
(8)
(9)
(9)
Thus,
(10)
(10)
For convenience, Tjostheims confines his discussion to the distance measure that is the active component of the squared Euclidean distance measure, thus;
(11)
(11)
This index establishes a relationship between two variables using measures that resemble the appearance of However, the earth is spherical, and the geographical coordinates are also spherical. Therefore, it is essential to transform the spherical coordinates into rectangular ones pertaining to spherical distance.
In this research, the modified Tjostheims coefficient is employed to estimate the spatial correlation between different patterns of SPEI and MSPEI. In the modified Tjostheims coefficient, the Lambert projection method converts geographic coordinates into rectangular coordinates. One is referred to see (Hussain et al. Citation2011) for a comprehensive description and computational procedure involved in Lambert projection. SpatialPack of R package (Osorio et al. (Citation2014)) is used to check the spatial correlation between two indices.
2.4. Trend analysis of MSPEI
The Mann-Kendall (M-K) test is commonly used to analyze climatological and hydrological time series trends. It has two benefits: first, as it is a non-parametric test, the data need not to be normally distributed. The other is that it is less sensitive to sudden interruptions due to non-uniform time series. In this test, the null hypothesis assumes that there is no trend and is compared to the alternative, which infers that there is a trend (Rahmat et al. (2012); Zhang et al. (Citation2021); (Rahmat et al. (2012); Zhang et al. (Citation2021)). Thus, the M-K method was selected to test the drought trend in this study. The M-K statistic is denoted as calculated in the following manner:
(12)
(12)
Where, sign () is a sign function. S_MK has a mean zero, and the variance can be calculated as follows:
(13)
(13)
The test statistic Z _MK is therefore written as:
(14)
(14)
A positive Z_MK value represents an upward trend, while a negative one indicates a downward trend.
2.5. Future trend of MSPE based on a Hurst index
Hurst Index (HI) is primarily used to assess a long-term memory of a time series. In other words, it determines how much the series deviates from the random walk. In this research, MSPEI's future trend is examined using HI. If HI = 0.5, the time series is a random walk, which means that if a location is presently experiencing drought, it is impossible to predict whether the drought will persist in the future or not. When 0 ≤ HI < 0.5, the time series is inconsistent, meaning that if a region is dry, it will progressively ease and become wet in the future. When 0.5 < HI ≤ 1, it implies that the time series is continuing in a positive direction, meaning that the drought conditions will continue in the same direction as before (Tong et al. Citation2018); Vega et al. (Citation2019); Zhang et al. (Citation2021).
Algorithm
The calculation of includes the following steps:
Step 1: Consider the MSPEI time series and calculate the mean sequence of the MSPEI (), where
= 1, 2, 3, … n.
(15)
(15)
Step 2: Calculate the cumulative dispersion using the following formula.
(16)
(16)
Step 3: The sequence of the Range is computed as follows:
(17)
(17)
Step 4: The following is the standard deviation sequence.
(18)
(18)
Step 5: Finally, the Hurst index is calculated as follows.
(19)
(19)
In the formula, H is the Hurst exponent (0 < H < 1), and is a constant representing the rescaled range at a unified time scale.
(20)
(20)
The logarithm of both sides of the Equationequation (20)(20)
(20) is used to generate the empirical Hurst formula. The Modified Hurst Index is a straight-line slope that exposes the fractal properties of a time series, calculated using time series and Hurst’s practical technique to create a cluster of H values for least-squares fitting.
3. Application
Drought is one of the most perplexing and little-understood natural disasters, affecting more people than any other disaster. Like the rest of the globe, Pakistan experiences several water scarcity and contamination issues. In addition, almost every region of Pakistan has experienced severe droughts, causing serious damage to the industrial, agricultural, and economic sectors Hussain and Mumtaz (Citation2014). Furthermore, several human fatalities have been recorded over the last three decades in Tharpakar (Sindh Province, Pakistan). Therefore, time is needed to fortify drought-tracking modules and alleviation strategies by developing robust drought-monitoring appliances and frameworks. Thus, the proposed index is initially applied to ten meteorological stations in Pakistan to assess the proposed framework’s efficiency. For that purpose, monthly precipitation, wind speed (min and max), temperature (min and max), humidity (min and max), and sunlight hours were all needed. Therefore, secondary data on these variables is collected by the Karachi Data Processing Center (KDPC) through the Pakistan Meteorological Department (PMD) from January 1980 to December 2019. This dataset complies with World Meteorological Organization (WMO) criteria, whereas KDPC does error deduction and quality control.
4. Results and discussion
4.1. Assessment of temporal behavior of precipitation
Droughts are caused by a lack of precipitation and an unpredictable precipitation pattern. The monthly precipitation occurrence in various months of the three meteorological stations of Pakistan, Skardu, Muzaffarabad, and Lahore, is presented in , respectively. The fluctuations in the precipitation patterns of Skardu, Muzaffarabad, and Lahore are caused by the different altitudes of these meteorological stations. To avoid the multiple figures, we presented the precipitation behavior at three stations; however, monthly precipitation occurrence at other stations can be observed similarly.
Figure 3. The pattern of monthly precipitation at Skardu Statio. Different colours in the figures depict different monthly precipitation levels that occurred in the chosen years. The selected years are indicated on the horizontal axis, while the varying months are shown on the vertical axis.

4.2. Estimation and comparative analysis of modified reference evapotranspiration
4.2.1. Empirical estimation of modified Hargreaves-Samani equation parameters
The fuzzy logic inference system is used to empirically estimate the parameters of MHS equation at ten meteorological stations in Pakistan. The fuzzy inference engine generates the fuzzy output variable for the parameters of MHS. Meanwhile, a suitable defuzzification center of gravity technique converts fuzzy variables into distinct outputs. According to , the mean value of ah varies between 0.0012 and 0.0022, which is lower than the initial value 0.0023), revealing that the ETo for this site is likely to be overestimated by the initial equation. Furthermore, Bahawalnagar and Sialkot have higher ah values, which is lower in Gilgit.
Table 1. The calibrated Hargreaves-Samani equation’s empirical parameters.
In the same way, the value of bh fluctuates between 0.45 and 0.6 and is higher in five stations (Gilgit, Skardu, DIK, and Islamabad), indicating that the initial HS equation tends to overestimate the ETo for these stations while being lower in the remaining stations than the initial value 0.5, indicating that the initial HS equation will underestimate the ETo for the leftover stations. Meanwhile, ch is observed to be varied from 12.7 to 22.4, with higher values in Skardu, Muzaffarabad and Islamabad, and lower values in the remaining stations in contrast to the actual value 17.8, and can be interpreted in the same way as the preceding. The dh is a calibration coefficient of effective temperature whose initial value varies from research to research. Camargo et al. (Citation1999) used dh = 0.72, Pereira and Pruitt (Citation2004) recommended dh = 0.69, while Ahmadi and Fooladmand (Citation2008) showed that the dh value will be changed for different locations. Similarly, in this research, the value of dh is changed according to the station, dh is observed to be varied from 0.22-0.61. Bahawalnagar has the greatest dh value, while Muzaffarabad has the lowest one.
4.2.2. Comparative analysis of modified reference evapotranspiration
This subsection presents a comparative evaluation of the non-calibrated and calibrated HS equation for ten meteorological stations in Pakistan. The original HS and MHS equations were evaluated on the basis of statistical indicators Root Mean Square Error (RMSE), Mean Absolute Error (MAE), and Performance Index (PI) classification. For a complete description of these statistical indicators, see Habeeb et al. (Citation2021).
demonstrates the comparison of HS and MHS with PM equation for estimating ETo. In Jhelum, with a PI value of 0.17, the non-calibrated HS equation has the highest RMSE and MAE values. Therefore, according to the classification criteria stated in Habeeb et al. (Citation2021), the performance of the non-calibrated HS equation is categorized as not good. By using the single parameter (dh) calibrated HS equation, the results were significantly improved, MAE decreased from 257.49 to 106.58, RMSE decreased from 270.4 to 118.12, and PI increased from 0.17 to 0.56. Therefore, the performance of a single parameter calibrated HS equation is improved. Meanwhile, we obtained better results after calibrating two, three, and four parameters of HS equation, demonstrating the effectiveness of fuzzy logic calibration for this study site.
Table 2. Comparison of HS and calibirated HS equations with PM equation for estimating ETo.
The outcomes associated with other stations can also interpret similarly. However, to summarize the results, the non-calibrated HS equation showed good performance in Skardu, DIK, Islamabad, medium in Gilgit, Lahore, and Sialkot, and not good in Bahawalnagar, Jhelum, and Muzaffarabad. The calibration of only dh ranked good for Jhelum, Sialkot, Muzaffarabad, Gilgit, Skardu, and Bahawalnagar and as medium for the leftover stations, making it possible to obtain improved results after the calibration. Similarly, the calibration of four parameters is ranked as good for Gilgit, while other stations are ranked very good. Meanwhile, the results of the study can also be verified by analyzing . showed that, in comparison to the non-calibrated HS equation, the calibrated HS equations are closer to PM equation, indicating the certainty that all calibrations, especially four parameters calibrated HS equation promoted better ETo estimate, and the success of fuzzy logic calibration is the reason behind this.
4.3. Estimation and comparative analysis of standardized indices
4.3.1. Fitting probability distribution
The appropriate probability distribution for each station is considered when calculating the drought index. Furthermore, the formulation incorporates 32 of the most commonly used probability distributions, and the most appropriate distributions are identified using the R package "propagate", which provides a list of these distributions. Minimum AIC and BIC values are used to determine the suitable probability distribution for each station. In and , six meteorological stations of Pakistan, namely Bahawalnagar, Kotli, Gilgit, DIK, Islamabad, and Jhelum, are chosen randomly to illustrate the probability distributions that are most suitable for the SPEI and MSPEI.
4.3.2. Comparative analysis of SPEI and MSPEI
The effectiveness and capability of the proposed index (MSPEI) are measured by including the results of the existing index (SPEI). Therefore, this subsection provides the comparative analysis of SPEI and MSPEI for four randomly selected stations of Pakistan. The temporal deviation between the estimated value of SPEI and MSPEI is illustrated in . To avert the multiple figures, the behavior of the four stations is presented here. Thus, a minimal variation has been found between the quantitative values of both methods in all selected stations. Moreover, the comparative analysis of SPEI and MSPEI revealed that although both indices’ patterns are identical, the variation has been slightly reduced in MSPEI. Meanwhile, in , the significant correlation between the quantitative values of SPEI and MSPEI for the selected stations can be observed.
Table 3. Pearson’s product-moment correlation between quantitative values of SPEI and MSPEI.
4.3.3. Spatial comparative analysis
The results of the comparative spatial analysis are based on Tjostheims coefficient index. Tjostheims coefficient measures the correlation between two stochastic processes observed over space. In this research, the Modified Tjostheims coefficient is employed to estimate the spatial correlation between different patterns of SPEI and MSPEI. For that purpose, the quantitative values of MSPEI are classified as Extremely Wet (EW), Sever Wet (SW), Moderate Wet (MW), Normal Drought (ND), Moderate Drought (MD), Sever Drought (SD) and Extreme Drought (ED) by using Oliver’s classification criteria, given in . Hence, the total counts for SPEI and MSPEI are given in and , respectively. The overall results for this index demonstrated that the frequencies of MD in both indices are high as compared to other patterns for all stations. Thus, outcomes associated with this research show little deviation in the counts of annual variability patterns determined by MSPEI. In addition, this section also describes the results of the comparative spatial analysis of EW, SW, MW, ND, MD, SD, and ED classes under both indices (MSPEI and SPEI). , demonstrated that there is a perfect spatial correlation between MSPEI and SPEI for the ND (r = 0.834), for SW and the MD spatial correlation is also high (r = 0.740, r = 0.718), for EW and ED spatial correlation is moderate, r = 0.673 and r = 0.592 respectively, whereas, for SD and MW, spatial correlation is slightly low (r = 0.434, r = 0.393).
Table 4. Drought classification criteria of SPEI and MSPEI.
Table 5. Total counts for SPEI of EW, SW, MW., ND, MD, SD and ED patterns.
Table 6. Total counts for MSPEI of EW, SW, MW, ND, MD, SD and ED patterns.
Table 7. Summary statistics of modified tjostheims coefficient.
4.4. Trend analysis
This subsection presents the current and future trend analysis of meteorological drought. The M-K test is first applied to the MSPEI time series on a one-month time scale. The Z_MK statistic obtained from M-K test for selected stations in Pakistan is depicted in . A Positive value of Z_MK indicates an upward trend and whereas a downward trend is indicated by a negative value of Z_ MK. In addition, the null hypothesis is tested at 5% level of significance. From , it can be demonstrated that only station Muzaffarabad showed a significantly increasing (wet) trend with the Z_MK value 1.69, Islamabad showed a trend that only slightly increased with Z_MK value 0.60, while the remaining stations showed a significantly decreasing (drying) trend. In Muzaffarabad, the minimum value of MSPEI is −1.413 in October 2010, which was a moderate drought, and the maximum value was 2.336 in July 1980, which was extremely wet. We can also interpret the results for other stations in the same way.
Table 8. Summary of the Mann–Kendall (M.K.) test of MSPEI.
In addition, considering the significance of future drought trend detection, the R/S analysis method is used to calculate the HI values of MSPEI for selected stations of Pakistan from 1980-2019 and to predict the trend of MSPEI. The result of the HI is demonstrated in . According to the statistics, the HI value of MSPEI in Bahawalnagar Sialkot, Lahore, Kotli, and Gilgit is 0.63, 0.58, 0.56, 0.55, and 0.53, respectively. In particular, HI> 0.5 indicates that present and future MSPEI tend to persist, at least in the near future. In other words, the MSPEI will continue in the same direction as before. On the other hand, Muzaffarabad, Skardu, and Jhelum had HI values of 0.47, 0.45, and 0.38, respectively, indicating moderate anti-persistence with the trend from 1980-2019. Meanwhile, two stations DIK and Islamabad, both have HI = 0.5, indicating that the MSPEI of DIK and Islamabad are a random walk. Therefore, in these two stations, it is not certain whether the trend of MSPEI in the future is consistent or anti-consistent with the current state.
Table 9. R/S analysis table for MSPEI 1980–2019.
4.5. Discussion
This research provides an assessment of meteorological drought by using the modified drought index, MSPEI. The results of this study were compared with the previous studies conducted on meteorological drought monitoring, such as; Vicente-Serrano et al. (Citation2010) proposed SPEI, and the multivariate characteristics of SPEI make it more adequate and superior to SPI. But, in SPEI Vicente-Serrano et al. (Citation2010) used TH equation to estimate ETo. However, the TH equation underestimates ETo in dry and semi-arid locations but overestimates ETo in semi-humid and wet areas (Van der Schrier et al. (Citation2011)). Therefore, this study focused on developing and evaluating a novel drought index for drought monitoring under different climate conditions to overcome the aforementioned issue. In MSPEI, we used a fuzzy logic-based calibrated HS equation to estimate ETo, see, Habeeb et al. (Citation2021). Thus, the efficiency and capability of the proposed index (MSPEI) are measured by including the results of the existing index (SPEI). Our analysis shows a substantial positive correlation between SPEI and MSPEI at each station. Moreover, the comparative analysis of SPEI and MSPEI () revealed that although both indices’ patterns are identical, the variation has been slightly reduced in MSPEI. Therefore, it can be concluded that the SPEI's inadequacies can be addressed by employing the MSPEI.
Subsequently, we used M-K test to investigate spatial and temporal variations in Pakistan’s drought characteristics. Out of ten stations, only Muzaffarabad showed a significantly increasing trend or wet trend, and Islamabad showed a semi-drying trend. In contrast, the remaining stations indicated a drying trend due to the significant decrease in precipitation and a considerable increase in temperature occurring in the southern part of Pakistan, leading to severe drought. Moreover, according to Slop Difference (SD) classification (Nabeel and Athar Citation2018), 66% of Pakistan’s land is classified as arid, whereas 30% (4%) as semi-arid (humid). Meanwhile, Hurst exponent predicted the future drought trend of Pakistan. Statistics showed that the present and future MSPEI of 5 out of 10 stations tend to sustain, at least shortly; on the other hand, three stations indicated mild anti-persistence with the trend in 1980-2019, whereas HI values of DIK and Islamabad indicated that their MSPEI's are a random walk. However, this research is unable to determine how long the anticipated drought trend will continue in the future, nor can it forecast future drought patterns in various categories.
In summary, our experimental results indicate that the performance of MSPEI was very well in monitoring the meteorological drought of the study area. Moreover, the outcomes of this research may accurately and timely inform decision-makers to anticipate sound strategies and plans to mitigate the adverse effects of drought. But, despite all these advantages, some limitations could affect the performance of MSPEI. First of all, there is no absolute "true" drought measure. We established a benchmark for comparisons by utilizing SPEI as the reference but cannot provide a true validation of MSPEI. Secondly, MSPEI was used to monitor drought only on a monthly scale. But the severity and spatial distribution of drought is continuously changing. This requires a smaller time scale, such as 8 days or 16 days. Thirdly, although there is a strong positive correlation between each quantitative value of SPI and SPEI, it does not guarantee that each indicator produces the same drought class in a particular month. Fourthly, the trend statistics presented in this research can be different if the trend analysis was conducted over a longer or shorter period. In order to develop a more credible region-specific drought trend, future studies should investigate the turning or change point in the drought trend.
5. Conclusion
In order to avert economic losses and natural disasters, it is essential to monitor the drought effectively. Various studies (Palmer (1965); McKee et al. (Citation1993); Tsakiris et al. (Citation2007); Vicente-Serrano et al. (Citation2010)) have suggested different indices to effectively monitor and forecast the drought. However, each drought index’s methodology differed by zone. Therefore, in this research, we introduced a new drought index based on MHS for regional drought monitoring, MSPEI, and then, spatio-temporal analysis of drought is performed. From the analysis of ten meteorological stations of Pakistan, we came to the following conclusions:
The effectiveness and capability of the proposed index (MSPEI) are measured by including the existing index (SPEI) results, and significant correlation between the quantitative values of MSPEI and SPEI has been found.
Therefore, we have concluded that the inadequacies of SPEI can be addressed by using this modified index MSPEI. Consequently, MSPEI can be used to develop effective drought mitigation policies.
Modified Tjostheims coefficient showed a strong positive spatial correlation between SPEI and MSPEI in SW, ND, and MD for ED and EW spatial correlation is also positive but slightly low, whereas the spatial correlation for SD and MW is low.
Hurst index for Bahawalnagar, Sialkot, Lahore, Kotli and Gilgit showed a strongly persistence long term trend, whereas Muzaffarabad, Skardu and Jhelum indicated moderate anti-persistence with the trend of MSPEI in 1980-2019. HI values of DIK and Islamabad indicated that their MSPEI's are random walk.
However, the results of HI are unable to foretell how long the predicted drought trend would last in the future or how future drought patterns will behave in different categories.
Concerning future work, the inferences and mathematical calculations can be generalized for other time scales. Moreover, for a more precise estimate of the ETo, it is recommended to utilize the actual Rs data instead of relying on the estimates.
Acknowledgments
Their authors extend Their appreciation to the Deanship of Scientific Research at King Khalid University for funding this work through Larg Groups. (Project under grant number (RGP.2/23/44). And this study is supported via funding from Prince Sattam bin Abdulaziz University project number (PSAU/2023/R/1444).
Disclosure statement
No potential conflict of interest was reported by the authors.
Data availability statement
Data will be provided upon request.
References
- Ahmadi SH, Fooladmand HR. 2008. Spatially distributedmonthlyreference evapotranspiration derived from the calibration of Thornthwaite equation: a case study, south of Iran. Irrig Sci. 26(4):303–312.
- Allen RG, Pereira LS, Raes D, Smith M. 1998. Crop evapotranspiration-Guidelines for computing crop water requirements-FAO Irrigation and drainage paper 56. Fao, Rome. 300(9):D05109. http://www.avwatermaster.org/filingdocs/195/70653/172618e_5xAGWAx8.pdf.
- Arsenault R, Brissette F. 2014. Determining the optimal spatial distribution of weather station networks for hydrological modeling purposes using RCM datasets: an experimental approach. J Hydrometeorol. 15(1):517–526.
- Bayissa YA, Moges SA, Xuan Y, Van Andel SJ, Maskey S, Solomatine DP, … Tadesse T. 2015. Spatio-temporal assessment of meteorological drought under the influence of varying record length: the case of Upper Blue Nile Basin, Ethiopia. Hydrol Sci J. 60(11):1927–1942.
- Beguería S, Vicente‐Serrano SM, Reig F, Latorre B. 2014. Standardized precipitation evapotranspiration index (SPEI) revisited: parameter fitting, evapotranspiration models, tools, datasets and drought monitoring. Int J Climatol. 34(10):3001–3023.
- Camargo AP, Marin FR, Sentelhas PC, Picini AG. 1999. Adjust of the Thornthwaite’s method to estimate the potential evapotranspiration for arid and superhumid climates, based on daily temperature amplitude. Rev Bras Agrometeorol. 7(2):251–257.
- Chao N, Wang Z, Jiang W, Chao D. 2016. A quantitative approach for hydrological drought characterization in southwestern China using GRACE. Hydrogeol J. 24(4):893–903.
- Cook BI, Anchukaitis KJ, Touchan R, Meko DM, Cook ER. 2016. Spatiotemporal drought variability in the Mediterranean over the last 900 years. J Geophys Res Atmos. 121(5):2060–2074.
- Ghosh S, Roy MK, Biswas SC. 2016. Determination of the best fit probability distribution for monthly rainfall data in Bangladesh. Am J Mathe Stat. 6(4):170–174.
- Habeeb R, Zhang X, Hussain I, Hashmi MZ, Elashkar EE, Khader JA, Soudagar SS, Shoukry AM, Ali Z, Al-Deek FF. 2021. Statistical analysis of modified Hargreaves equation for precise estimation of reference evapotranspiration. Tellus A: Dynamic Meteorol Oceanograph. 73(1):1966869.
- Hassani H, Silva ES. 2015. A Kolmogorov-Smirnov based test for comparing the predictive accuracy of two sets of forecasts. Econometrics. 3(3):590–609.
- Huang S, Huang Q, Leng G, Chang J. 2016. A hybrid index for characterizing drought based on a non-parametric kernel estimator. J Appl Meteorol Climatol. 55(6):1377–1389.
- Hubert LJ, Golledge RG, Costanzo CM, Gale N. 2010. Measuring association between spatially defined variables: an alternative procedure. Geograph Anal. 17(1):36–46.
- Hussain I, Pilz J, Spoeck G. 2011. Homogeneous climate regions in Pakistan. Int J Glob Warm. 3(1–2):55–66.
- Hussain M, Mumtaz S. 2014. Climate change and managing water crisis: Pakistan’s perspective. Rev Environ Health. 29(1–2):71–77.
- Jabloun MD, Sahli A. 2008. Evaluation of FAO-56 methodology for estimating reference evapotranspiration using limited climatic data: application to Tunisia. Agric Water Manage. 95(6):707–715.
- Jiao W, Tian C, Chang Q, Novick KA, Wang L. 2019. A new multi-sensor integrated index for drought monitoring. Agric for Meteorol. 268:74–85.
- McKee TB, Doesken NJ, Kleist J. 1993. The relationship of drought frequency and duration to time scales. Proceedings of the 8th Conference on Applied Climatology. 17(22):179–183. https://climate.colostate.edu/pdfs/relationshipofdroughtfrequency.pdf.
- Montazeri H, Blocken B, Derome D, Carmeliet J, Hensen JL. 2015. CFD analysis of forced convective heat transfer coefficients at windward building facades: influence of building geometry. J Wind Eng Ind Aerodyn. 146:102–116.
- Nabeel A, Athar H. 2018. Classification of precipitation regimes in Pakistan using wet and dry spells. Int J Climatol. 38(5):2462–2477.
- Osorio F, Vallejos R, Cuevas F. 2014. SpatialPack: package for analysis of spatial data. R package version 0.2-3, URL: CRAN. R-project. org/package = SpatialPack.
- Palmer WC 1965. Meteorological drought (Vol. 30). US Department of Commerce, Weather Bureau.
- Papadopoulou E, Varanou E, Baltas E, Dassaklis A, Mimikou M. 2003. Estimating potential evapotranspiration and its spatial distribution in Greece using empirical methods. In Proc,. 8th Int. Conf. on Environmental Science and Technology (pp. 650–658). https://conferences.gnest.org/cest8/8cest_papers/abstracts_pdf_names/posters_abs/p198_Papadopoulou.pdf.
- Patel J, Patel H, Bhatt C. 2015. Modified Hargreaves equation for accurate estimation of evapotranspiration of diverse climate locations in India. Proc Natl Acad Sci, India, Sect B Biol Sci. 85(1):161–166.
- Pereira AR, Pruitt WO. 2004. Adaptation of the Thornthwaite scheme for estimating daily reference evapotranspiration. Agric Water Manage. 66(3):251–257.
- Rahmat SN, Jayasuriya N, Bhuiyan M. 2012. Trend analysis of drought using standardised precipitation index (SPI) in Victoria, Australia. In 34th Hydrology and Water Resources Symposium November, Sydney, Australia (Vol. 19, p. 22). https://d1wqtxts1xzle7.cloudfront.net/46594280/Trend_analysis_of_drought_using_Standard20160618-25483-1shcix1-with-cover-page-v2.pdf?Expires=1665385227&Signature=E7ef1XDmhsytyeMEawou6G7r5Wq83Be3aHcLSVBpkjp1xxDr7fP0OfPQG8Tf9b-xBrpX-6z5UTI0-qt662KT9apJEZayP3j7fyVnB-k-WXSQ5QS3dJIOsPBK5CMAnxKkPMaYsTbTn∼8AKoUuAOkCzhOVxWWOVu6Vry-PvuR16hdyl4IU08rHicpxRcCUhXNOQtZA∼zU-h7Cvsi3pJsu∼8XmbCNwbBs-5vCaHMKOUXLCTe9Ka35hEMwi∼wRvhZbdIc6Wc5k2p7NjuBdrywC0YTQDfXAJZ7hn1xy5LOVVFGu4bIBqPg8zbNLhagfiT9OVqtXlHgUR-edvy-nhj41nu4A__&Key-Pair-Id=APKAJLOHF5GGSLRBV4ZA.
- Shah D, Mishra V. 2020. Integrated Drought Index (IDI) for drought monitoring and assessment in India. Water Resour Res. 56(2):e2019WR026284.
- Spiess AN, Spiess MAN, Rcpp L. 2018. Package 'propagate’. https://mirror.las.iastate.edu/CRAN/web/packages/propagate/propagate.pdf.
- Stagge J, Kingston D, Tallaksen L, Hannah D. 2016. Diverging trends between meteorological drought indices (SPI and SPEI) in Europe. In EGU General Assembly Conference Abstracts (pp. EPSC2016-10703). https://ui.adsabs.harvard.edu/abs/2016EGUGA.1810703S.
- Task Committee on Revision of Manual 70. 2016. Evaporation, evapotranspiration, and irrigation water requirements. American Society of Civil Engineers (ASCE).
- Tjøstheim D. 1978. A measure of association for spatial variables. Biometrika. 65(1):109–114.
- Tong S, Lai Q, Zhang J, Bao Y, Lusi A, Ma Q, Li X, Zhang F. 2018. Spatiotemporal drought variability on the Mongolian Plateau from 1980–2014 based on the SPEI-PM, intensity analysis and Hurst exponent. Sci Total Environ. 615:1557–1565.
- Tsakiris G, Pangalou D, Vangelis H. 2007. Regional drought assessment based on the Reconnaissance Drought Index (RDI). Water Resour Manage. 21(5):821–833.
- Van der Schrier G, Jones PD, Briffa KR. 2011. The sensitivity of the PDSI to the Thornthwaite and Penman‐Monteith parameterizations for potential evapotranspiration. J Geophys Res: Atmospheres 116(D3):1–16.
- Van Wijk WR, De Vries DA. 1954. Evapotranspiration. NJAS. 2(2):105–119.
- Vega HM, Lima JR, Cerniak SN. 2019. SPEI and Hurst analysis of precipitation in the Amazonian Area of Brazil. Rev Bras Meteorol. 34(2):325–334.
- Vicente-Serrano SM, Beguería S, López-Moreno JI. 2010. A multiscalar drought index sensitive to global warming: the standardized precipitation evapotranspiration index. J Climate. 23(7):1696–1718.
- Wang GQ, Zhang JY, Jin JL, Pagano TC, Calow R, Bao ZX, Liu CS, Liu YL, Yan XL. 2012. Assessing water resources in China using PRECIS projections and a VIC model. Hydrol Earth Syst Sci. 16(1):231–240.
- Wang L, Yuan X, Xie Z, Wu P, Li Y. 2016. Increasing flash droughts over China during the recent global warming hiatus. Sci Rep. 6(1):1–8.
- West H, Quinn N, Horswell M. 2019. Remote sensing for drought monitoring & impact assessment: progress, past challenges and future opportunities. Remote Sens Environ. 232:111291.
- Zhang H, Song J, Wang G, Wu X, Li J. 2021. Spatiotemporal characteristic and forecast of drought in northern Xinjiang, China. Ecol Indic. 127:107712.