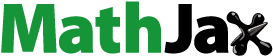
Abstract
Slope length gradient (LS) is one of the crucial factors in the Universal Soil Loss Equations (USLE, RUSLE). This study aimed at estimating the slope-length and slope-steepness (LS) factor for the entire watersheds of Afghanistan by using three different methods, namely; (1) LS-TOOLMFD (Method 1); (2) The Method of Equations (Method 2); and (3) The approach of Moore and Burch (Method 3). The first method uses the digital elevation model (DEM) in the ASCII format, and the other two methods use the DEM in the spatial domain. The results show that the LS-factor of the study area ranges from 0.01 to 44.31, with a mean of 5.24 and standard deviation of 6.95, according to Method 1; 0.03 to 163.49, with a mean of 9.6 and standard deviation of 13.58, according to Method 2; and 0 to 3985, with a mean of 7.16 and standard deviation of 29.7, according to Method 3. The study reveals that Methods 1 and 2 are more appropriate than Method 3 because Method 3 yields high LS-factor values close to or at streamlines located near mountainous regions. The highest LS values are found to be in the northeast, north, and central regions of Afghanistan, which is consistent with the high mountains and deep valley geomorphology, indicating that these regions are particularly vulnerable to soil erosion by rainfall-runoff processes. The sediment delivery ratio (SDR) for the Upper-Helmand River Basin (Upper-HRB) is also estimated by the RUSLE, employing the LS factors produced by the three methods. The results revealed that the average annual soil loss is found to be, respectively, 9.3, 18.2, and 11.1 (ton/ha/year) by using the three methods, corresponding to SDR of 23.5%, 12.1%, and 19.9%.
Abbreviations: ABD: Asian Development Bank; ASCII: American Standard Code for Information Interchange; Bsh: cold semiarid steppes; Bsk: cold semiarid steppes; Bwh: warm and cold deserts; Bwk: warm and cold deserts; CMS: Convention on Migratory Species; Csa: humid subtropical; Csb: Mediterranean; D: humid continental; DEM: Digital Elevation Model; ET: extreme tundra; GCS: Geographical Coordinate System; GIS: Geographic Information System; GUI: Graphically User Interface; Ha: Hectare; HMRB: Harirod-Murghab River Basin; HRB: Helmand River Basin; KRB: Kabul River Basin; LS: Slope–Length and Slope–Steepness; Masl: meters above sea level; MFD: Multiple-Flow Direction; NRB: Northern River Basin; PARB: Panj-Amu Darya River Basin; RUSLE: Revised Universal Soil Loss Equations; SDR: Sediment Delivery Ratio; SFD: Single–Flow Direction; SRTM: Shuttle Radar Topography Mission; SY: Sediment Yield; UCA: Unit Contributing Area; USGS: United States Geological-Survey; USLE: Universal Soil Loss Equations
1. Introduction
Soil erosion process and sediment transport by rainfall-runoff are frequently cited as the most severe kinds of land degradation with significant environmental and socio-economic consequences (Fu et al. Citation2006; Rahman et al. Citation2009; Aiello et al. Citation2015; Phinzi and Ngetar Citation2019; Xiao et al. Citation2021). Agricultural and livestock production provides about 95% of the world’s food, making the soil a critical resource for feeding a growing population (FAO Citation2015). The most recent United Nations report on the situation of the world’s soil resources emphasizes that ‘the majority of the world’s soil resources are in just fair, poor, or very poor situation’ and highlights that the ‘soil loss, erosion and sediment transportation continue to pose a significant agricultural and environmental threat all over the world’ (FAO and ITPS Citation2015). Developing countries, particularly Malaysia, Indonesia, Iran, Turkey, and India are currently dealing with a significant soil erosion problem primarily caused by rainfall intensity, raindrop energy, and topographic and anthropogenic characteristics (Morgan Citation1974; Demirci and Karaburun Citation2012; Markose and Jayappa Citation2016; Noori et al. Citation2016; Kashiwar et al. Citation2022). Several studies have also cited human activity as the key source of current land use and cover changes (Oldeman et al. Citation1990; Bridges and Oldeman Citation1999; J. Thomas et al. Citation2018; Kashiwar et al. Citation2022; Villarreal et al. Citation2022).
Slope-length (L) and steepness (S) are two topographic parameters, used in the USLE and RUSLE, contributing to soil erosion. The topographical effects of soil erosion are depicted by the combined effect of slope-length and slope-steepness (LS). The volume and rate of cumulative runoff increase as the length of the land slope becomes steep. Additionally, when the slope increases, the runoff velocity also increases, contributing to soil erosion (Ghosal and Das Bhattacharya Citation2020; Xiao et al. Citation2021). The topographic factor LS and soil erosion have a direct relationship; erosion by runoff would be high when slope length and slope steepness are high and vice-versa (Gupta and Kumar Citation2017; Kashiwar et al. Citation2022; Kulimushi, Maniragaba, et al. Citation2021). It indicates the influence of slope-length and steepness on sheet, rill, and inter-rill erosion by water. Ganasri and Ramesh (Citation2016) and Kashiwar et al. (Citation2022) state that the LS-factor represents its impact on soil loss over a watershed area. The LS-factor in the RUSLE model accounts for the influence of flow accumulated in cells upstream to those downstream (Wischmeier and Smith Citation1978; Rubianca et al. Citation2018; Elnashar et al. Citation2021), representing soil loss ratio under given conditions to that area with a slope-length of (L = 22.12 m) and slope-steepness of 9% (Renard et al. Citation1997). The factor is the ratio of anticipated soil erosion from a field’s slope gradient to the actual USLE unit plot length (Wischmeier and Smith Citation1978).
The topographic factors (L and S) were in one dimension when the USLE and the RUSLE were initially developed for gentle-sloping fields. However, to estimate the average annual rill and sheet soil erosion per unit area at country-level river basins, the topography becomes 2D, and the calculation of the LS factor becomes more complex than other variables in the USLE and RUSLE (Ligonja and Shrestha Citation2015; Zhang et al. Citation2017). The researchers can account for more topographically complicated terrain by using the digital elevation models (DEMs) to compute flow accumulation, cell size, upslope draining regions, and corresponding LS-factor (Moore and Burch Citation1986; Desmet and Govers Citation1996; Rubianca et al. Citation2018; Kulimushi, Choudhari, et al. Citation2021).
The USLE and the RUSLE are commonly used to predict soil erosion at regional levels; nevertheless, a fundamental limitation is the difficulty of obtaining the LS factor (Zhang et al. Citation2017). Several adjustments have been made to the original LS factor model proposed by Wischmeier and Smith (Citation1978) (). According to McCool et al. (Citation1989), soil loss increases on slopes that are steeper than 9% (Panagos et al. Citation2015; Kruk et al. Citation2020). Using the point approach of Griffin et al. (Citation1988) for the L factor and Moore and Wilson (Citation1992) for the S factor, Moore and Wilson (Citation1992) proposed the unit contributing area (UCA) method in a simple equation to estimate LS for three-dimensional topography. The technique developed by Desmet and Govers (Citation1996), based on raster resolution and unit area, are widely used by researchers all around the globe. Panagos et al. (Citation2015) suggested a novel methodology based on the UCA method to determine topographic parameters in regions comparable in size to European countries and examined the effect of DEM resolution on the distribution of the LS factor (Kruk et al. Citation2020). L can be estimated using the UCA or the slope length (λ) as input parameters for large watersheds. The UCA approach is inadequately accurate due to the lack of a slope length estimation. The spatial accuracy of slope length and the LS factor must be improved to predict soil erosion accurately (Zhang and Wang Citation2017). To overcome these limitations, Zhang et al. (Citation2017) proposed an improved approach to calculated the slope length and LS factor. To determine the slope length (λ) and the LS factor, they integrated the multiple-flow direction algorithm (MFD), used in the UCA technique with the LS-TOOL (LS-TOOLSFD) algorithms, accounting for calculation errors and distance cutoff conditions. The (LS-TOOLMFD) approach was implemented and validated in a watershed with complexly changing slopes. The slope length and LS estimated using (LS-TOOLMFD) agreed with field data better than the (LS-TOOLSFD) and UCA techniques.
Table 1. Formulas and methods for calculating the topographic factor (LS).
The methodology for determining the LS factor by the USLE or the RUSLE employs slope- length, angle, and a parameter that is proportional to the slope’s-steepness in percent (Wischmeier and Smith Citation1978). Several approaches have been developed over the last two decades that enable the application of the ArcGIS platform to develop verified algorithms for calculating the LS-factor that are both USLE- and RUSLE-based (Khosrokhani and Pradhan Citation2014; Zhang et al. Citation2017; Lanorte et al. Citation2019; Pal and Chakrabortty Citation2019b; Chakrabortty et al. Citation2022; Das et al. Citation2022; Kaffas et al. Citation2021). According to Zhang et al. (Citation2017), using the LS-TOOLMFD is more realistic and easy to determine LS-factor values in large watersheds, while the approach of (Moore and Burch Citation1986) (Method 3) also has advantages over the original LS-factor equations proposed by Wischmeier and Smith (Citation1978), in which they had applied a particular contribution area as a slope length estimate, which is more suitable to 3D landscapes. Das et al. (Citation2022) used the two methods combined with the GIS procedure to estimate the LS-factor. Such approaches employing several methods are key improvements in estimating the LS-factor for large regions such as Afghanistan.
The topographic factors (L and S) were in one dimension when the USLE and the RUSLE were initially developed for gentle-sloping fields. However, to estimate the average annual rill and sheet soil erosion per unit area at country-level river basins, the topography becomes 2D, and the calculation of the LS factor becomes more complex than other variables in the USLE or RUSLE equation (Ligonja and Shrestha Citation2015; Zhang et al. Citation2017). The researchers can account for more topographically complicated terrain by using the DEMs to compute flow accumulation, cell size, upslope draining regions, and corresponding LS-factor (Moore and Burch Citation1986; Desmet and Govers Citation1996; Rubianca et al. Citation2018; Kulimushi, Choudhari, et al. Citation2021).
It is impossible to obtain an exact estimate of how much of Afghanistan’s land surface is affected by the soil erosion problem, due to the absence of sufficient soil information. Soil mapping in Afghanistan has only been done on a minor scale, and detailed study is limited to alluvial valleys-little is known about the country’s upland soils. However, due to the geography of the region, the dry environment, and the desert character of the study area, approximately 80% of it might be exposed to soil loss by water. The topography in Afghanistan’s mountainous regions has been severely folded by tectonic activity, resulting in steeper slopes and deep valleys (Tapponnier et al. Citation1981). Additionally, to study on a country level, specifically in developing and under-developing countries, in particular, the lack of field measurement and data due to complex topography, the danger of land mines, and the region’s instability (special case in Afghanistan), and social, political, and economic constraints results in less data and necessitates the use of cost-effective spatial techniques prior to field assessment (Sujatha and Sridhar Citation2018). The loess soil is characterized by will-formed horizontal fractures and susceptible soil erosion. Additionally, several factors contribute to soil loss in Afghanistan: poor land use practices, such as cultivating crops on open land or reclaiming steep slopes, as well as an over-reliance on shrubs for fuelwood, all contribute to severe soil loss in Afghanistan (Saba Citation2001).
A careful review of the literature reveals that no substantial research has been undertaken on the importance of quantifying the LS-factor in the study area and its impact on sustainability in Afghanistan, containing steep slopes in the central, north, and northeast regions. The objectives of this study are mainly three folds: (1) to estimate the LS-factor for the entire watersheds in Afghanistan by three widely used LS methods, namely; the LS-TOOLMFD (Method 1), the Method of Equations (Method 2), and the approach of Moore and Burch (Citation1986) (Method 3); (2) to compare the output of three different methods with studies carried out in similar topography with similar approaches and objectives; and (3) to assess the characteristics of the topographic factors and determine the most appropriate model for the LS-factor estimation, which would help researchers to estimate soil loss and prepare risk assessment maps and management plans for watersheds of Afghanistan.
2. Description of the study area
Afghanistan has a varied geography and is located in the Central Asian region. It is bounded by Turkmenistan, Uzbekistan, and Tajikistan in the north; China in the northeast; Pakistan to the east and south; and Iran to the west. It lies between 29° 35′ and 38° 40′ north latitude, and 60° 31′ and 74° 55′ east longitude (Shrestha Citation2007). The mountains cover the majority of the country’s land area, where the elevation ranges from nearly 248 m at the lowest altitude in the northern valley of the Amu Darya River to the highest, at 7435 m above sea level (masl) in the mountainous regions. The study area covers all five major river basins and 41 sub-basins in Afghanistan, surrounding a total area of about 647,500 km2 that corresponds to Helmand River Basin (HRB), Harirod-Murghab River Basin (HMRB), Northern River Basin (NRB), Panj-Amu Darya River Basin (PARB), and Kabul River Basin (KRB) (see ).
Figure 1. Locations of Afghanistan’s five major River Basins map, meteorological station, Kajaki Reservoir, Lakes, and inland water.

shows the climate map for Afghanistan. According to Köppen-Geiger’s climate type classification, Afghanistan is classified as cold and warm semi-arid steppes (Bsh, Bsk, see ), and cold and warm flat deserts (Bwh, Bwk, see ). The warmer deserts are located in southwest Sistan Catchment, while the warmer steppes are situated in the Jelal Abaad Catchment and the east side of Rigestan. The cooler steppes and flat lands are seen in the north regions of Afghanistan. The Mediterranean (Csb, see ) and humid sub-tropical (Csa, see ) climates occur at lower altitudes in the north and south. These climates are characterized by mild winters and dry summers, while the humid continental climates (D, see ) vary from warmer to cooler and colder, which depends on altitudes from lower to higher elevations. The Cold climates, such as the extreme tundra (ET, see ) and ice caps, can be found at the highest mountain peaks (Koppen Citation1936; De Bie et al. Citation2007; Shroder Citation2014).
Figure 2. Detailed climate map of Afghanistan (Bsh, Bsk: cold semiarid steppes; Bwh, Bwk: warm and cold deserts; Csa: humid subtropical; Csb: Mediterranean; D: humid continental; ET: extreme tundra) (Source: De Bie et al. Citation2007; Shroder Citation2014).

Soil erosion by water is a natural process whereby soil particles are detached by the splash when the raindrop hits the land surface. The detached particles are transported to the rills by overland flow, and the process is called inter-rill erosion (Thorman Citation2007; Yang Citation2014). The five dominant process of soil erosion in Afghanistan is classified as (Hillslope, gully, stream bank, landslide, and Deflation). The most common type of water erosion in Afghanistan’s topography is hillslope erosion, which includes sheet and rill erosion. This type of water erosion lowers land productivity because it removes fertile topsoil, which contains the majority of the soil’s plant nutrients and microorganisms that help keep the soil healthy (Saba Citation2001; Shroder Citation2014; John and Sher Jan Citation2016). Sheet and rill erosion is also a significant contributor to sedimentation and deterioration of water quality in rivers, ponds, and reservoirs, and it could contribute to the loss of ∼50% of dams’ storage capacity, specifically the Kajaki and Dahla dams, which lost about ∼42% and ∼40% of their water storage due to sedimentation, respectively (Orville et al. Citation1976; US Army Corps of Engineers Citation2012; US Army Corps Citation2014; ADB et al. Citation2019).
3. Materials and methods
In this study, for the LS calculations, three methods were employed. The aim of using three methods for LS calculation was to obtain the appropriate method for Afghanistan. In all these methods, the DEM was first employed to generate the LS factor maps.
An SRTM-DEM raster image was used to calculate the slope-length and slope-steepness factor (LS). Approximately one hundred and two (102) SRTM 1 Arc-Second (30 m spatial resolution) raster tiles were downloaded for the entire Afghanistan region from the United States Geological-Survey (USGS) earth-explorer tool (https.://earthexplorer.usgs.gov./). The DEM raster tiles were imported from Add Data command to the ArcGIS environment as an input. For further data processing and mosaicking of the DEM for Afghanistan from raster tiles, the ArcGIS Toolbox > Data Management-Tools > Raster-Tool > Raster-dataset > Mosaic to New Raster processing steps were used in ArcGIS version 10.7.1 software (Izmir Institute of Technology, Turkey.). For extracting the DEM file for Afghanistan from mosaicked tiles, the Arc Toolbox > Spatial Analyst-Tools > Extraction-Tool > Extract by mask processes were followed to clip the desired region in ArcGIS 10.7.1 platform. For the projection of DEM file of entire Afghanistan, the Arc Toolbox > Data Management-Tools > Projection and Transformation > Raster-Tool > Project raster steps were performed to change the coordinate system from Geographical Coordinate System (GCS) 1984 to Lambert Conformal Conic projection system in ArcGIS 10.7.1. The final step was to convert the DEM raster format to ASCII format; the Arc Toolbox > Conversion tools > From Raster > Raster to ASCII procedure was followed to convert the DEM raster format to ASCII format. The study area’s surface elevation ranges from nearly 238 to 7461 m above sea level (masl) (see ). The slope map of Afghanistan was derived in percentage and degree and classified based on the recommendation of Lee (Citation2014).
presents the generated slope map for the whole Afghanistan. The colours of green and dark green represent relatively flat areas while red colour shows a relatively steep slope (S > 15%). Slopes are classified into five slope classes as: very gentle slope grade zone (less than 5%), gentle slope grade zone (5–15%), moderate slope grade zone (15–30%), steep slope grade zone (30–50%), and very steep slope grade zone (more than 50%) as per the recommendations in the literature (Berihun et al. Citation2020; Das et al. Citation2022; Negese et al. Citation2021). As seen in , the high degree of slopes is observed at high elevations.
The details of the three methods for calculating the LS factor are given in the following section.
3.1. LS-TOOLMFD (Method 1)
Zhang et al. (Citation2017) presented a simple LS-TOOLMFD to estimate LS. The LS-TOOLMFD algorithm saves processing time while improving the accuracy of large-scale erosion modelling (Zhang et al. (Citation2017). They found a strong correlation between the LS-factor map estimated by LS-TOOLMFD and results collected from field data. Therefore, in this study, the slope length and slope steepness factors (L and S, respectively) were estimated using the LS-TOOLMFD method proposed by Zhang et al. (Citation2017). For further processing and sequences of LS calculation; the processed ASCII file was used as input data in Load DEM > Output file path > Model (select; RUSLE) > Cutoff (select; Yes) > Sink Fill (select; Yes) > Nodata Fill (select; Average) > Channel (select; YES) > Threshold Unit (8000) > Cumulated way (select; Total) > Flow Direction (select; MFD) > Select MFD (select; MS) > Save Database (select; LS factor) along with LS-TOOL calculator. depicts the graphically user interface (GUI) for the LS-TOOLMFD.
3.2. LS factor calculation using method of equations (Method 2)
In this method, the Shuttle Radar Topography Mission (SRTM) DEM obtained from the United States Geological Survey (https://earthexplorer.usgs.gov/) and resampled to 250 m spatial resolution was used to derive the L and S factors. For the LS factor calculation and mapping, EquationEquations (1)–(3) (Desmet and Govers Citation1996) and EquationEquations (4)(4)
(4) and Equation(5)
(5)
(5) (McCool et al. Citation1989; Renard et al. Citation1997) were employed.
(1)
(1)
(2)
(2)
(3)
(3)
(4)
(4)
(5)
(5)
where L represents the equivalent slope length factor for the cell, S is the slope steepness factor, A is the contribution area at the inlet of the grid cell (m2); D is the size of the grid cell (m); m represents the standard slope length exponent, B is the ratio of rill to inter-rill erosion under the situations where the soil is fairly sensitive to the both, sl is the slope in degree and to convert the angle (sl) from degree to radian, 0.01745 should be multiplied by sl.
L and S factor can be computed in the ArcGIS raster calculator using the map algebra expression, expressed by the EquationEquations (6)–(8), suggested by Chadli (Citation2016) and Elnashar et al. (Citation2021) as follows:
(6)
(6)
(7)
(7)
(8)
(8)
The value of slope in EquationEquations (7)(7)
(7) and Equation(8)
(8)
(8) was obtained directly from a 250 m resolution DEM. Accordingly, the flow accumulation was generated from the DEM after the filling and flow direction procedures in ArcGIS 10.7.1 are incorporated with the Arc-Hydro extension tool.
3.3. LS calculation using approach of moor and Burch (1986) (Method 3)
Moore and Burch (Citation1986) developed the LS estimation method using the DEM for topographically complicated terrain, based on the unit stream theory. The method requires the components such as flow accumulation and slope for computing L and S factors. In this article, the slope-length and accumulation of flow were calculated using the SRTM DEM in the Arc-hydro extension of ArcGIS software. The slope gradient map was derived using the spatial analyst tool in the ArcGIS platform (Gupta and Kumar Citation2017; Pal and Shit Citation2017). For extracting the study area from the DEM, these sequences were followed in the GIS platform: ArcGIS 10.7.1 > Spatial Analyst Tools > Extract by mask. In the input raster command box, the DEM file was selected, and in the feature mask data, the Shapefile of the study area was inserted using the input raster or feature mask command (Jain and Das Citation2010; Gwapedza et al. Citation2018; Pal and Chakrabortty Citation2019b). illustrates the methodological flowchart, employed in this study, for the computation of the LS factor using the method of Moore and Burch (Citation1986).
The accumulation of flow indicates an upstream region flowing into a particular cell by accumulating the draining region of all upslope cells (Elnashar et al. Citation2021). For estimation of the LS-factor in Method 3, maps, such as flow accumulation and slope (in degree) are required. The SRTM-DEM raster file was used as an input image in the Arc Toolbox and the processing sequences of Spatial Analyst tools > Hydrology > Fill > Flow Direction > Flow Accumulation were followed in the ArcGIS 10.7.1 environment to compute the flow accumulation map, shown in . The areas with very low flow accumulation are represented in green colour, and the regions corresponding to high flow accumulation are depicted in yellow and red colours in . The accumulation of flow was found to be highest (reaching to a value of 1.299e + 06) along the streamlines near the channels and lowest in the rest of the watershed of the research region, as seen in .
The slope (degree) map of Afghanistan was generated from the DEM raster file utilizing the spatial analyst tool in ArcGIS 10.7.1 platform, as seen in . Finally, the maps of flow accumulation and slope were combined to generate the LS map using the methodology in the raster calculator of the ArcGIS environment > Spatial Analyst tool.
Using the flow accumulation, slope steepness, and size of cell generated from the DEM raster file, as explained in the flowchart in , EquationEquation (9)(9)
(9) , as proposed by Moore and Burch (Citation1986), was used to calculate the LS factor on the ArcGIS platform, as follows:
(9)
(9)
where LS is the slope length gradient factor. The flow-accumulation represents the accumulated up-slope contribution catchment region for a specific cell, the cell-size represents the size of the grid cell (for this research, 250 m spatial resolution), and sin-slope denotes the angle of slope in degrees.
EquationEquation (9)(9)
(9) is also employed in the literature, such as by Prasannakumar et al. (Citation2011), Mahalingam et al. (Citation2015), Baby and Nair (Citation2016), and Markose and Jayappa (Citation2016) for the Siruvani river watersheds in the Attapady valley, Kerala; Pandavapura, Mandya, Karnataka; Kuttiyadi river basin, Northern Kerala; and Kali river basin, Karnataka, respectively. Pal and Shit (Citation2017) studied soil erosion in Jaipanda watershed in West Bengal while Pal and Chakrabortty (Citation2019a) investigated soil estimation in sub-tropical Arkosa watershed in eastern India. All researchers above have assumed m = 0.4 and n = 1.3, according to Moore and Burch (Citation1986).
3.4. Application of RUSLE for estimation of soil erosion in the Upper-Helmand River Basin
The RUSLE components (R, K, LS, C, and P) have been incorporated into the GIS platform to estimate the average annual soil erosion. The linear equation (R × K × LS × C×P) has been considered for generating the soil erosion raster map while considering the pixel information of each component (Renard et al. Citation1997; Chakrabortty et al. Citation2022; Pal et al. Citation2021).
4. LS factor results
The findings from assessing the three LS-factor models for the entire Afghanistan watersheds are presented herein.
4.1. Topographic factor (LS) results by Method 1
The calculated LS factors for Afghanistan, presented in , range from 0.01 to 44.31, with a mean of 5.24 and a standard deviation of 6.957. According to , the regions with low LS values are primarily located in the southwest, west, and northern parts of Afghanistan, near the borders of Turkmenistan and Uzbekistan while large LS values are found to be located in the northeast, north, and central parts of Afghanistan, which is consistent with its high mountains and deep valley geomorphology. These results imply that northeast, north, and central parts of Afghanistan are particularly prone to soil erosion by runoff water.
4.2. Topographic factor (LS) results by Method 2
presents the LS factor map generated by employing Method 2. The LS-factors are ranging between 0.03 and 163.49, with a mean of 9.6 and a standard deviation of 13.58. As seen in , high LS-factors are found to be in regions with steep slopes near rivers and streamlines. This indicates that the combination of L and S factors significantly influences soil loss in the northeast, north, and central regions of Afghanistan. Method 2 also produced low and high LS-factor values at almost the same regions, as in Method 1.
4.3. Topographic factor (LS) results by Method 3
presents the LS factor map generated by Method 3. The LS-factors for the entire Afghanistan range between 0 and 3985, with an average value of 7.16 (). Maximum value of the LS-factor is found to be significantly high at irregular topography where more flow accumulates (see ) near or along the streamlines, contributing to increasing the LS factor. Low LS factor values are found in the southwest, west, and northern parts (near the borders of Turkmenistan and Uzbekistan) of Afghanistan. High values of the LS-factor are seen in the northeast, north, and central parts of Afghanistan, which is consistent with its high mountains and deep valley geomorphology (see ). These locations (northeast, north, and central parts of Afghanistan) are especially prone to erosion by water due to the steep slope gradient (see ).
4.4. Annual soil loss estimation for Upper-Helmand River Basin
The primary goal of this analysis is to assess the LS factors generated by three different methods. In addition, it is desired to assess how the estimated LS factors by each method can produce sediment delivery ratio (SDR). This is presented by applying the RUSLE to Upper Helmand River Basin.
4.4.1. Helmand River Basin
The HRB covers about 50% of the Afghanistan landmass including 14 provinces (AIMS-FAO Citation2004; Goes et al. Citation2013). The HRB is the vital source of flowing water into the Kajaki dam, which is a significant resource for domestic water supply in an indirect way by recharging the shallow aquifers along the river and also used extensively for irrigation network (AIMS-FAO Citation2004; Goes et al. Citation2013). The basin has long been recognized as Afghanistan’s breadbasket and has the potential for agricultural-based industries, particularly high-value horticulture (Goes et al. Citation2013). The Upper-HRB is a sub-watershed of the HRB (see ), covering an area of 46,881.97 km2 (about 7% of Afghanistan), and the catchment is a vital water supply resource for irrigation of agricultural lands and hydropower production. The Upper HRB is considered in Section 4.4 because of the existence of Kajaki Dam, which is subjected to the extensive sedimentation problem. The dam is vital for Afghanistan for both irrigation and power purposes.
The revised universal soil loss equation (RUSLE) (EquationEquation (10)(10)
(10) ) was used to estimate the soil erosion (Renard et al. (Citation1997):
(10)
(10)
where average annual soil erosion potential A (ton ha−1 year−1) is computed by multiplying the developed raster data from each RUSLE analysis. R is the rainfall–runoff erosivity factor (MJ mm ha−1 h−1 year−1); K is the soil erodibility factor (ton ha h ha−1 MJ−1 mm−1), L and S are topographic factors that vary with slope length and gradient (dimensionless), C is the crop and crop management factor (dimensionless), and P is the soil conservation practice factor (dimensionless, and ranges from zero to one).
The SDR represents the ratio of sediment yield to gross soil erosion (Maner Citation1958; De Vente et al. Citation2007). The SDR was calculated by dividing the average annual sediment yield by the gross soil loss, predicted by the RUSLE, as shown in EquationEquation (11)(11)
(11) .
(11)
(11)
where SY stands for the annual average sediment yield in t year−1; SDR stands for sediment delivery ratio, dimensionless scalar; and ARUSLE is the average annual soil loss determined by the RUSLE erosion model in ton year−1. The five major RUSLE factors (R, K, LS, C, and P) were combined using the raster calculator in the ArcGIS spatial analyst tool to quantify the annual average soil loss (ARUSLE) from the Upper-Helmand River Catchment.
Average annual soil loss was estimated based on five potential data layers of RUSLE (R, K, (LS-Method 1, LS-Method 2, and LS-Method 3), C, and P)) using the raster calculator of the Spatial Analyst tool extension in the ArcGIS 10.7.1 environment. The findings demonstrate that the annual soil loss of the Upper-Helmand River Basin () ranged from 0 to 131.2 by using the LS-factor of Method 1 (see ) and 0 to 242.3 by using the LS-factor of Method 2 (see ) and 0 to 2877.1 by using the LS-factor of Method 3 (see ) in the RULSE equation, with mean annual soil loss of 9.3, 18.2, and 11.1 ton/ha/year.
Figure 13. (a) Upper-Helmand River Basin Elevation, (b) Soil erosion map by Method 1, (c) Soil erosion map by Method 2, and (d) Soil erosion map by Method 3.

The average annual sediment yield rate of the Kajaki reservoir, located on Helmand River was obtained from the US Army Corps of Engineers (Citation2012) survey report. According to their survey report, the average annual (trapped/settled) sediment yield rate in the active pool of the Kajaki reservoir is 8.53 M-m3/year. Using the mean annual sediment yield of the Kajaki reservoir and mean annual soil loss rates, the SDR of the Upper-Helmand River Basin was determined by EquationEquation (11)(11)
(11) and the results are summarized in .
Table 2. Average LS-factor values, average annual soil loss, and SDR.
The average annual soil loss in Upper-Helmand River basin is 9.3, 18.2, and 11.1 (ton/ha/year) by applying the three different LS-factor values in RUSLE equation, respectively, and resulting SDR values are 23.48%, 12.02%, and 19.85%, that are compatible with the findings of Arekhi et al. (Citation2012), Bagherzadeh et al. (Citation2013), and Nikkami and Shadfar (Citation2021) who estimated the average SDR of 23%, 24.8%, and 25.22% for the watersheds which has similar land characteristics and topography.
The study revealed that the LS-factor has a close agreement with soil loss rate and SDR. Increases in the LS-factor lead to an increase in runoff water-driving power, which in turn leads to an increase in soil loss. From , it can be seen that the soil loss increases with increasing the LS-factor, while SDR decreases.
4.5. Discussion of results
Three approaches were employed in this study to calculate the LS-factor for the entire Afghanistan. The LS results of the three methods ranged from 0.01 to 44.31 according to Method 1, from 0.03 to 163.49 according to Method 2, and from 0 to 3985 according to Method 3, with respective means of 5.24, 9.6, and 7.16 and standard deviations of 6.95, and 13.58, and 29.70.
The validation of the results was difficult due to a lack of local data, a common problem in developing countries, especially in a war-torn Afghanistan. As an alternative, the consistency of the results of this study was compared against those of previous studies having used similar techniques and objectives in regions with similar land characteristics and topography (Sujatha and Sridhar Citation2018). Iran, Turkey, some parts of China, and India have almost the same topography and follow the same seasonal patterns; therefore, in this study, the findings were compared against those related to these countries. The estimated mean LS-factor results (5.24, 9.6, and 7.16) of the study agreed with the findings of Mohammadi et al. (Citation2021), who calculated the mean topographic factor value of ∼4 for all watersheds in Iran. Panagos et al. (Citation2015) also found the mean LS factor of 5.2 for the whole Austria, which is consistent with the result of this study. Jobin Thomas et al. (Citation2018) estimated mean LS value of ∼8 in the southern Western Ghats, India.
In addition, according to the entire India LS factor map prepared by Pal et al. (Citation2021), the LS factor in the mountainous regions located in Srinagar, Gilgit-Baltistan, and Ladakh of India is in the same increasing trend as our results obtained in the mountainous areas of Afghanistan.
The location of Afghanistan is in the greater Alpine-Himalayan Orogenic Belt in Asia (Shroder Citation2014). In north Italy, the Alpine basin with steep mountainous topography and high elevation Kaffas et al. (Citation2021) calculated mean LS factor of ∼8.8 using the equations of (Desmet and Govers Citation1996) and (McCool et al. Citation1989). The spatial pattern of the LS factor is consistent with that estimated by Yang (Citation2014), Yang et al. (Citation2018), Sujatha and Sridhar (Citation2018); Chakrabortty et al. (Citation2020), who have demonstrated LS values increase in mountainous regions and lower in flat plains. Compared to these studies, the calculated LS value and the spatial distribution are generally reasonable.
The comparable mean values (5.24, 9.6, and 7.16), estimated by the three methods imply that these methods can be employed for estimating LS factors. All the three methods, although the magnitude has a wide variation, produced maps having low LS factor values in the southwest, west, and northern parts and high LS values in the northeast, north, and central parts of Afghanistan. These results imply that the northeast, north, and central parts of Afghanistan might be particularly prone to soil erosion. Mohammadi et al. (Citation2021) have spotted high LS factor values in the mountainous regions of Iran and revealed the effect of slope steepness on soil erosion that agree with the findings in this study. They found the highest soil loss values in the steep slopes of the Alborz and Zagros mountains, located in the north, west, and southwest parts of Iran.
The results showed that increasing LS-factor values increases soil loss that corresponds to with the findings of Renard et al. (Citation1997). Increasing of the LS parameters increases velocity of water on the ground surface and finally, giving rise to higher soil loss values (Wischmeier and Smith Citation1978; Gupta and Kumar Citation2017; Ghosal and Das Bhattacharya Citation2020; Pal et al. Citation2021). Soil erosion is a significant concern, particularly in the research area, where several causes contribute to the fast erosion and sedimentation of soil. Factors, such as the steepness of the slope of the study area, higher overland runoff velocity on steeper slopes, and environmental variables all enhance the soil erosion and sedimentation rate. Kaffas et al. (Citation2021) and Chakrabortty et al. (Citation2022) have demonstrated that the steepness of the slope and greater overland runoff velocity on steeper slopes lead to a higher gravity and produce more increment in soil erosion. This result is compatible with that of Negese et al. (Citation2021), who reported severe soil erosion in the steep and very steep slopes of Chereti Watershed in Northeastern Ethiopia. Das et al. (Citation2022) and Negese et al. (Citation2021) have concluded that the soil erosion and LS-factor increase as the accumulation of flow and slope increases across the catchment area. Hui et al. (Citation2010) conducted a study in Liao Watershed in Jiangxi Province of China; they also found that the LS-factor values are high in the mountainous regions with no vegetation cover and steep topography. All these results may conform to the reliability of the findings in this study.
The result shows that the equation of Moore and Burch (Citation1986), used in Method 3, significantly overestimated the maximum value for the LS factor and gave a considerably higher LS value, compared to the maximum values of Methods 1 and 2. The first reason for such a large discrepancy might be that Method 3 considers flow accumulation as a significant input and thus large LS factor values are only computed close to or at the streamlines located near to mountainous regions.
The graphical comparison of the three methods was performed for the LS-factor, using a 250 m resolution DEM covering the entire Afghanistan river basins. Based on the hillshade map shown in , which is a segment of study area, it was observed from the graphical comparison among the three methods that Method 1, as seen in , is more apparent and shows clearer distribution LS-factor values over the catchment segment compared to the other methods and it gives an unambiguous indication of the LS of the research area. The reason might be that the multiple flow algorithm (MFD) used in LS-TOOL can adapt to convergent and divergent overland flow and work more effectively than single-flow direction (SFD) algorithms for watersheds. That is how the LS-TOOLMFD can produce a better LS-factor map. Method 2, as shown in , also presents a clear distribution of LS-factor over the region because, while calculating, the slope-length was fixed to 22.13 m in order to prevent exceedingly long hill-slope length; that can have an impact on and explain the geographical distribution of soil erosion throughout the watershed. Method 3, as shown in , on the other hand, showed a poor picture of the LS-factor map compared to the LS-factor maps generated by Methods 1 and 2. This fact is also seen in the LS-factor map calculated using the Moore and Burch (Citation1986) equation for sub-catchment regions of Juari River in Tripuara by Das et al. (Citation2022). However, it should be noted that comparison is based on the graphical assessment, while the numerical values (mean and standard deviation) of the LS-factor calculated using the mentioned three methods showed the best results for the entire Afghanistan.
4.6. Sedimentation
About 0.5–1% of the sedimentation worldwide disrupts the annual loss of reservoir storage capacity. In addition, it is estimated that by the year 2050, most of the world’s dams would retain just half of their current capacity, which is quite alarming (Dams Citation2000; Chuenchum et al. Citation2019). According to Walling (Citation2011), sediments presently cover 40% of Asia’s reservoir storage, suggesting a significant loss of storage capacity. These factors have an impact on the long-term sustainability of water resources (Chuenchum et al. Citation2019).
The watersheds in Afghanistan do not have sediment gauging network for measurement of sediment transported by runoff water. Therefore, this study’s findings are comparable to those of similar studies in other parts of the world, particularly in neighbouring countries where similar geo-climatological and topographic constraints are prevalent (Flügel et al. Citation2003; Lu et al. Citation2004), which validate the scalability of the suggested models and methods.
Despite the fact that erosion is a serious concern in Afghanistan, there is no erosion and sedimentation mapping for all basins. Most of the data on sedimentation currently accessible comes from studies of sediment yield survey conducted from 1953 to 1968 and from 1968 to 2010 after the construction of the Kajaki reservoir on the Helmand River in southern Afghanistan.
Helmand River Basin (Upper, Middle, and Lower), which covers about 50% of the Afghanistan landmass, is selected as a representative watershed for sedimentation and the SDR study. According to Perkins and Culbertson (Citation1970) and US Army Corps (Citation2014) survey conducted in Kajaki reservoir, the sedimentation occupies 42% of total storage capacity of Kajaki reservoir, which is consistent with findings of Walling (Citation2011) for Asian reservoirs. Based on their results, average annual fill rate of Kajaki reservoir is 8.53 M-m3/year from 1953 to 2010. The estimated SDR of this study was found to be relatively consistent with the mean SDR of Arekhi et al. (Citation2012), Bagherzadeh et al. (Citation2013), and Nikkami and Shadfar (Citation2021) who estimated the average SDR for the watersheds which has similar land characteristics and topography. In comparison to these studies, the computed SDR value is reasonable.
Considering the importance of soil erosion, sedimentation, and the delivery ratio of the watershed as an environmental issue in Afghanistan and the world (Shrestha Citation2007; Shroder Citation2014; Sujatha and Sridhar Citation2019), it is essential to model and identify the effects of potential erosion zones and land use on land degradation, as it helps sustainable planning and management of the watershed.
5. Conclusions
This study estimated, for the first time in the literature, the LS factors and produced the related maps for the entire Afghanistan using three different methods. The average LS-factor values estimated in the studied area ranged 5.24, 9.6, and 7.16 with a range of 0.01–44.31, 0.03–163.49, and 0–3985, respectively, for Methods 1–3. In this study, the performance of the methods was evaluated by comparing the results with the studies conducted in the regions that have the similar land characteristics or with the neighbouring countries that have approximately similar topography.
All the three methods, although the magnitude has a wide variation, produced maps having low LS factor values in the southwest, west, and northern parts and high LS values in the northeast, north, and central parts of Afghanistan. These results imply that the northeast, north, and central parts of Afghanistan might be particularly prone to soil erosion.
The result shows that the equation of Moore and Burch (Citation1986), used in Method 3, significantly overestimated the maximum value for the LS factor and gave a considerably higher LS value, compared to the maximum values of Methods 1 and 2. The first reason for such a large discrepancy might be that Method 3 considers flow accumulation as a significant input and thus large LS factor values are only computed close to or at the streamlines located near to mountainous regions.
The SDR results also reveal that the RUSLE employing the LS factors produced by the Method 1 have more compatibility with the findings in the literature for the watersheds which have similar land characteristics and topography.
Concerning the erosion management strategies to minimize soil loss rate, the intervention may concentrate on the LS-factor; hence, terrace management may reduce the length of the slope and, as a result, control the soil loss. The combined use of LS with ArcGIS software and remote sensing technologies may be noticed to be crucial, effective, and less expensive for the estimation and mapping of topographic factors, as well as the identification of vulnerable catchment areas for conservation planning and implementation objectives.
In general, one of the important limitations in studies for aiming at mapping topographic factors is the lack of validation data. This is particularly true for Afghanistan. Although the produced LS factor values are reliable, they have not been yet validated against the field measurements. Such a validation would update and enhance the LS factor digital map for Afghanistan. The availability of high-resolution DEMs and the continuous development of new algorithms and tools would provide possibilities for overcoming the drawbacks of the current approaches and enhancing the accuracy and resolution of the LS factor mapping.
The DEM and the GIS are used together in this study for the first time to estimate the topographic factors for the entire watersheds of Afghanistan, which rectifies the uniqueness of the work. The resultant LS map can be used as one of the multiplying parameters in the RUSLE model for estimating the soil loss for various watersheds in Afghanistan, as presented in this study, for the Upper Helmand river Basin. In addition, this study would be important for national decision-makers to prioritize investment in treating land degradation in Afghanistan.
This study is not able to carry out the actual field-data comparison due to the current situation in Afghanistan, that makes it practically impossible to accomplish this mission. However, this study is aware of the importance of this matter, and therefore it intends to complete this mission as soon as it is possible.
Acknowledgments
The authors need to thank the SRTM for their data support. We would also like to thank Hongming Zhang, Jicheng Wei, Qinke Yang, Jantiene E.M. Baartman, Lingtong Gai, Xiaomei Yang, ShuQin Li, Jiantao Yu, Coen J. Ritsem, and Violette Geissen for their help with the LS-TOOL for estimating the LS-factor.
Disclosure statement
The authors declare that they have no conflict of interest.
Data availability statement
The data that support the findings of this study are available from the corresponding author upon a requirement and request.
References
- ADB-CMS. 2019. Afghanistan: Arghandab Integrated Water Resources Development Project-Asian Development Bank (ADB).
- Aiello A, Adamo M, Canora F. 2015. Remote sensing and GIS to assess soil erosion with RUSLE3D and USPED at river basin scale in southern Italy. Catena. 131:174–185.
- AIMS-FAO. 2004. Watershed atlas of Afghanistan. Rome, Italy: AIMS-FAO.
- Arekhi S, Darvishi A, Shabani A, Fathizad H, Ahmadai Abchin S. 2012. Mapping soil erosion and sediment yield susceptibility using RUSLE, remote sensing and GIS (Case study: Cham Gardalan Watershed, Iran). J Adv Environ Biol. 6(1):109–124.
- Baby A, Nair A. 2016. Soil erosion estimation of Kuttiyadi River basin using RUSLE. Int Adv Res J Sci Eng Technolo. 3(3):275–279.
- Bagherzadeh A, Mansouri D, Mohammad R. 2013. Evaluation of sediment yield and soil loss by the MPSIAC model using GIS at Golestan watershed, northeast of Iran. Arab J Geosci. 6(9):3349–3362.
- Berihun ML, Tsunekawa A, Haregeweyn N, Dile YT, Tsubo M, Fenta AA, Meshesha DT, Ebabu K, Sultan D, Srinivasan R. 2020. Evaluating runoff and sediment responses to soil and water conservation practices by employing alternative modeling approaches. Sci Total Environ. 747:141118.
- Bridges E, Oldeman L. 1999. Global assessment of human-induced soil degradation. Arid Soil Res Rehabil. 13(4):319–325.
- Chadli K. 2016. Estimation of soil loss using RUSLE model for Sebou watershed (Morocco). Model Earth Syst Environ. 2(2):51.
- Chakrabortty R, Pal SC, Arabameri A, Ngo PTT, Chowdhuri I, Roy P, Malik S, Das B. 2022. Water-induced erosion potentiality and vulnerability assessment in Kangsabati river basin, eastern India. Environ Dev Sustain. 24(3):3518–3557.
- Chakrabortty R, Pal SC, Sahana M, Mondal A, Dou J, Pham BT, Yunus AP. 2020. Soil erosion potential hotspot zone identification using machine learning and statistical approaches in eastern India. Nat Hazards. 104(2):1259–1294.
- Chuenchum P, Xu M, Tang W. 2019. Estimation of soil erosion and sediment yield in the Lancang–Mekong River using the modified revised universal soil loss equation and GIS techniques. Water. 12(1):135.
- Dams WCo. 2000. Dams and development: a new framework for decision-making: the report of the world commission on dams. Oxford: Earthscan.
- Das S, Bora PK, Das R. 2022. Estimation of slope length gradient (LS) factor for the sub-watershed areas of Juri River in Tripura. Model Earth Syst Environ. 8(1):1171–1177.
- De Bie K, Skidmore AK, Toxopeus B, Venus V. 2007. An updated Köppen–Geiger climate classification of the world using very high resolution interpolated climate surfaces of monthly P and T data from 1950 to 2000. ITC News. 4:16–17.
- De Vente J, Poesen J, Arabkhedri M, Verstraeten G. 2007. The sediment delivery problem revisited. Prog Phys Geogr. 31(2):155–178.
- Demirci A, Karaburun A. 2012. Estimation of soil erosion using RUSLE in a GIS framework: a case study in the Buyukcekmece Lake watershed, northwest Turkey. Environ Earth Sci. 66(3):903–913.
- Desmet P, Govers G. 1996. A GIS procedure for automatically calculating the USLE LS factor on topographically complex landscape units. J Soil Water Conserv. 51(5):427–433.
- Elnashar A, Zeng H, Wu B, Fenta AA, Nabil M, Duerler R. 2021. Soil erosion assessment in the Blue Nile Basin driven by a novel RUSLE-GEE framework. Sci Total Environ. 793:148466.
- FAO. 2015. FAO, healthy soils are the basis for healthy food production. Rome, Italy: (FAO); p. 1–4. http://www.fao.org/documents/card/en/c/645883cd-ba28-4b16-a7b8-34babbb3c505/.
- FAO & ITPS. 2015. Status of the world’s soil resources (SWSR)–main report. (ISBN 978-92-5-109004-6). (Food and agriculture organization of the United Nations and intergovernmental technical panel on soils. Rome, Italy: FAO & ITPS.
- Flügel WA, Märker M, Moretti S, Rodolfi G, Sidrochuk A. 2003. Integrating geographical information systems, remote sensing, ground truthing and modelling approaches for regional erosion classification of semi‐arid catchments in South Africa. Hydrol Process. 17(5):929–942.
- Fu G, Chen S, McCool DK. 2006. Modeling the impacts of no-till practice on soil erosion and sediment yield with RUSLE, SEDD, and ArcView GIS. Soil Tillage Res. 85(1–2):38–49.
- Ganasri BP, Ramesh H. 2016. Assessment of soil erosion by RUSLE model using remote sensing and GIS - A case study of Nethravathi Basin. Geosci Front. 7(6):953–961.
- Ghosal K, Das Bhattacharya S. 2020. A review of RUSLE model. J Indian Soc Remote Sens. 48(4):689–707.
- Goes BJM, Howarth SE, Wardlaw RB, Hancock IR, Parajuli U. 2013. Helmand river basin master plan-technical report 5. Afghanistan: Ministry of Energy and Water.
- Griffin M, Beasley D, Fletcher J, Foster G. 1988. Estimating soil loss on topographically non-uniform field and farm units. J Soil Water Conserv. 43(4):326–331.
- Gupta S, Kumar S. 2017. Simulating climate change impact on soil erosion using RUSLE model − A case study in a watershed of mid-Himalayan landscape. J Earth Syst Sci. 126(3):43.
- Gwapedza D, Slaughter A, Hughes D, Mantel S. 2018. Regionalising MUSLE factors for application to a data-scarce catchment. Proc IAHS. 377:19–24.
- Hui L, Xiaoling C, Lim KJ, Xiaobin C, Sagong M. 2010. Assessment of soil erosion and sediment yield in Liao watershed, Jiangxi Province, China, Using USLE, GIS, and RS. J Earth Sci. 21(6):941–953.
- Jain MK, Das D. 2010. Estimation of sediment yield and areas of soil erosion and deposition for watershed prioritization using GIS and remote sensing. Water Resour Manage. 24(10):2091–2112.
- John FS, Sher Jan A. 2016. Transboundary water resources in Afghanistan. Climate change and land-use implications. Amsterdam, Netherlands: Elsevier.
- Kaffas K, Pisinaras V, Al Sayah MJ, Santopietro S, Righetti M. 2021. A USLE-based model with modified LS-factor combined with sediment delivery module for Alpine basins. Catena. 207:105655.
- Kashiwar SR, Kundu MC, Dongarwar UR. 2022. Soil erosion estimation of Bhandara region of Maharashtra, India, by integrated use of RUSLE, remote sensing, and GIS. Nat Hazards. 110(2):937–959.
- Khosrokhani M, Pradhan B. 2014. Spatio-temporal assessment of soil erosion at Kuala Lumpur metropolitan city using remote sensing data and GIS. Geomat Nat Hazard Risk. 5(3):252–270.
- Koppen W. 1936. Das geographische system der klimat. Handbuch Der Klimatologie. Vol. 46. Berlin, Germany: Gebrüder Borntraeger.
- Kruk E, Klapa P, Ryczek M, Ostrowski K. 2020. Influence of DEM elaboration methods on the USLE model topographical factor parameter on steep slopes. Remote Sens. 12(21):3540.
- Kulimushi LC, Choudhari P, Mubalama LK, Banswe GT. 2021. GIS and remote sensing-based assessment of soil erosion risk using RUSLE model in South-Kivu province, eastern, Democratic Republic of Congo. Geomatics Nat Hazards Risk. 12(1):961–987.
- Kulimushi LC, Maniragaba A, Choudhari P, Elbeltagi A, Uwemeye J, Rushema E, Singh SK. 2021. Evaluation of soil erosion and sediment yield spatio-temporal pattern during 1990–2019. Geomatics Nat Hazards Risk. 12(1):2676–2707.
- Lanorte A, Cillis G, Calamita G, Nole G, Pilogallo A, Tucci B, De Santis F. 2019. Integrated approach of RUSLE, GIS and ESA Sentinel-2 satellite data for post-fire soil erosion assessment in Basilicata region (Southern Italy). Geomat Nat Hazard Risk. 10(1):1563–1595.
- Lee S. 2014. Geological application of geographic information system. Korea Inst Geosci Min Resour. 9:109–118.
- Ligonja PJ, Shrestha RP. 2015. Soil erosion assessment in Kondoa eroded area in Tanzania using universal soil loss equation, geographic information systems and socioeconomic approach. Land Degrad Dev. 26(4):367–379.
- Lu D, Li G, Valladares GS, Batistella M. 2004. Mapping soil erosion risk in Rondonia, Brazilian Amazonia: using RUSLE, remote sensing and GIS. Land Degrad Dev. 15(5):499–512.
- Mahalingam B, MALIK M, Vinay M. 2015. Assessment of soil erosion using USLE technique: A case study of Mysore district, Karnataka, India. J Remote Sens GIS. 6(3):1–7.
- Maner SB. 1958. Factors affecting sediment delivery rates in the Red Hills physiographic area. Trans AGU. 39(4):669–675.
- Markose VJ, Jayappa K. 2016. Soil loss estimation and prioritization of sub-watersheds of Kali River basin, Karnataka, India, using RUSLE and GIS. Environ Monit Assess. 188(4):1–16.
- McCool DK, Foster GR, Mutchler C, Meyer L. 1989. Revised slope length factor for the Universal Soil Loss Equation. Trans ASAE. 32(5):1571–1576.
- Mohammadi S, Balouei F, Haji K, Khaledi Darvishan A, Karydas CG. 2021. Country-scale spatio-temporal monitoring of soil erosion in Iran using the G2 model. Int J Digital Earth. 14(8):1019–1039.
- Moore ID, Burch GJ. 1986. Physical basis of the length‐slope factor in the universal soil loss equation. Soil Sci Soc Am J. 50(5):1294–1298.
- Moore ID, Wilson JP. 1992. Length-slope factors for the Revised Universal Soil Loss Equation: simplified method of estimation. J Soil Water Conserv. 47(5):423–428.
- Morgan R. 1974. Estimating regional variations in soil erosion hazard in Peninsular Malaysia. Malay Nat J. 28:94–106.
- Negese A, Fekadu E, Getnet H. 2021. Potential soil loss estimation and erosion-prone area prioritization using RUSLE, GIS, and remote sensing in Chereti Watershed, Northeastern Ethiopia. Air Soil Water Res. 14:117862212098581.
- Nikkami D, Shadfar S. 2021. Soil erosion mapping in sediment gauged watersheds of Iran. Watershed Eng Manage. 13(2):479–496.
- Noori H, Siadatmousavi SM, Mojaradi B. 2016. Assessment of sediment yield using RS and GIS at two sub-basins of Dez Watershed, Iran. Int Soil Water Conserv Res. 4(3):199–206.
- Oldeman LR, Hakkeling R, Sombroek WG. 1990. World map of the status of human-induced soil degradation: an explanatory note. Netherlands: International Soil Reference and Information Centre.
- Orville F, William F, Richard H, Glen L, Charles E. 1976. Helmand Valley, soil and water survey of the Helmand River Basin. The International Association of Hydrological Sciences, IAHS-AISH publication.
- Pal SC, Chakrabortty R. 2019a. Modeling of water induced surface soil erosion and the potential risk zone prediction in a sub-tropical watershed of Eastern India. Model Earth Syst Environ. 5(2):369–393.
- Pal SC, Chakrabortty R. 2019b. Simulating the impact of climate change on soil erosion in sub-tropical monsoon dominated watershed based on RUSLE, SCS runoff and MIROC5 climatic model. Adv Space Res. 64(2):352–377.
- Pal SC, Chakrabortty R, Roy P, Chowdhuri I, Das B, Saha A, Shit M. 2021. Changing climate and land use of 21st century influences soil erosion in India. Gondwana Res. 94:164–185.
- Pal SC, Shit M. 2017. Application of RUSLE model for soil loss estimation of Jaipanda watershed, West Bengal. Spat Inf Res. 25(3):399–409.
- Panagos P, Borrelli P, Meusburger K. 2015. A New European slope length and steepness factor (LS-factor) for modeling soil erosion by water. Geosciences. 5(2):117–126.
- Perkins DC, Culbertson JK. 1970. Hydrographic and sedimentation survey of Kajakai Reservoir, Afghanistan. Washington (DC): US Government Printing Office.
- Phinzi K, Ngetar NS. 2019. The assessment of water-borne erosion at catchment level using GIS-based RUSLE and remote sensing: a review. Int Soil Water Conserv Res. 7(1):27–46.
- Prasannakumar V, Shiny R, Geetha N, Vijith H. 2011. Spatial prediction of soil erosion risk by remote sensing, GIS and RUSLE approach: a case study of Siruvani river watershed in Attapady valley, Kerala, India. Environ Earth Sci. 64(4):965–972.
- Rahman MR, Shi ZH, Chongfa C. 2009. Soil erosion hazard evaluation—an integrated use of remote sensing, GIS and statistical approaches with biophysical parameters towards management strategies. Ecol Modell. 220(13–14):1724–1734.
- Renard KG, Foster GR, Weesies GA, McCool DK, Yoder DC. 1997. Predicting soil erosion by water: a guide to conservation planning with the Revised Universal Soil Loss Equation (RUSLE). Washington (DC): United States Government Printing.
- Rubianca B, Bethanna J, Deborah M, Kevin N. 2018. A review of the (Revised) Universal Soil Loss Equation ((R)USLE): with a view to increasing its global applicability and improving soil loss estimates. Hydrol Earth Syst Sci. 22:6059–6086.
- Saba DS. 2001. Afghanistan: environmental degradation in a fragile ecological setting. Int J Sustain Dev World Ecol. 8(4):279–289.
- Shrestha R. 2007. Land degradation in Afghanistan.
- Shroder JF. 2014. John F Shroder - Natural resources in Afghanistan _ geographic and geologic perspectives on centuries of conflict-Elsevier (2014).pdf.
- Sujatha E, Sridhar V. 2018. Spatial prediction of erosion risk of a small mountainous watershed using RUSLE: a case-study of the Palar Sub-Watershed in Kodaikanal, South India. Water. 10(11):1608.
- Sujatha ER, Sridhar V. 2019. Mapping of erosion susceptibility using a weighted linear combination model: a case study of a hill sub-watershed in Kodaikkanal, Western Ghats, South India. Remote Sens Appl Soc Environ. 14:34–45.
- Tapponnier P, Mattauer M, Proust F, Cassaigneau C. 1981. Mesozoic ophiolites, sutures, and arge-scale tectonic movements in Afghanistan. Earth Planet Sci Lett. 52(2):355–371.
- Thomas J, Joseph S, Thrivikramji K. 2018. Assessment of soil erosion in a tropical mountain river basin of the southern Western Ghats, India using RUSLE and GIS. Geosci Front. 9(3):893–906.
- Thorman R. 2007. Water erosion hazard: indicator protocols for soil condition. Canberra, Australia: National Land and Water Resources Audit.
- US Army Corps, U. S. A. C. E. 2014. Water control manual Kajaki Dam and reservoir Helmand River Basin. Washington (DC): US Army Corps, U. S. A. C. E.
- US Army Corps of Engineers, U. A. C. E. 2012. Storage capacity estimate for Kajaki reservoir, Afghanistan. Concord (MA): US Army Corps of Engineers, U. A. C. E.
- Villarreal ML, Norman LM, Yao EH, Conrad CR. 2022. Wildfire probability models calibrated using past human and lightning ignition patterns can inform mitigation of post-fire hydrologic hazards. Geomatics Nat Hazards Risk. 13(1):568–590.
- Walling DE. 2011. Human impact on the sediment loads of Asian rivers. The International Association of Hydrological Sciences, IAHS-AISH publication.
- Wischmeier WH, Smith DD. 1978. Predicting rainfall erosion losses: a guide to conservation planning. Hyattsville (MD): Department of Agriculture, Science and Education Administration.
- Xiao Yang Guo B, Lu Y, Zhang R, Zhang D, Zhen X, Chen S, Wu H, Wei C, Yang L, Zhang Y, et al. 2021. Spatial–temporal evolution patterns of soil erosion in the Yellow River Basin from 1990 to 2015: impacts of natural factors and land use change. Geomat Nat Hazard Risk. 12(1):103–122.
- Yang X. 2014. Deriving RUSLE cover factor from time-series fractional vegetation cover for hillslope erosion modelling in New South Wales. Soil Res. 52(3):253.
- Yang Y, Zhao R, Shi Z, Viscarra Rossel RA, Wan D, Liang Z. 2018. Integrating multi-source data to improve water erosion mapping in Tibet, China. Catena. 169:31–45.
- Zhang H, Wei J, Yang Q, Baartman JEM, Gai L, Yang X, Li S, Yu J, Ritsema CJ, Geissen V. 2017. An improved method for calculating slope length (λ) and the LS parameters of the Revised Universal Soil Loss Equation for large watersheds. Geoderma. 308:36–45.
- Zhang XC, Wang ZL. 2017. Interrill soil erosion processes on steep slopes. J Hydrol. 548:652–664.