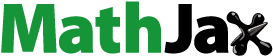
Abstract
The selection and planning of the location of emergency shelters have a crucial impact on the safety of residents and cities. In this paper, based on multivariate open geographic data, the Gaussian two-step floating catchment area method, K-means clustering, and particle swarm optimization algorithm methods are utilized to carry out a spatial accessibility analysis and location optimization of emergency shelters in Deyang City, Sichuan Province, China. The study shows that: (1) Deyang City’s emergency shelters are higher than the government’s relevant standard requirements in terms of major indicators such as single building area, total area and per capita shelter area. (2) The spatial distribution of emergency shelters in the study area is uneven and unreasonable, with accessibility from the urban center outwards, exhibiting a “high-low” distribution pattern. (3) The study suggests that 10 new emergency shelters can reduce the number of accessible blind areas by 43.31%. The study recommends that the assessment and construction of emergency shelter facilities in rural areas in China and globally should be emphasized. Reliable recommendations for improvement of emergency shelter planning in Deyang city are provided in the study results.
1. Introduction
China is one of the countries in the world with the most diverse types of natural disasters, the highest number of occurrences, and the most severe disaster losses (Liu et al. Citation2012). Since ancient times, China’s urban development has been affected by earthquakes, floods, typhoons, landslides, mudslides, snowstorms, dust storms, and marine disasters. From 2000 to 2019, 571 natural disasters were counted in China, the highest number of disasters suffered in the world (Centre for Research on the Epidemiology of Disaster, United Nations Office for Disaster Risk Reduction Citation2020). In 2008, China experienced two mega disasters, the Snowstorm, and the Wenchuan Earthquake, which caused unprecedented damage to economic development and the safety of people’s lives and property. The Wenchuan earthquake was also ranked by United Nations Office for Disaster Risk Reduction (UNDRR) as one of the deadliest disasters in the two decades from 1995 to 2015. Conducting research on early warning, monitoring, response, analysis and planning for the whole process of risk, process, impact and reconstruction of natural disasters, which is an important element to protect people’s lives and properties and promote sustainable development of regions and communities (Giupponi and Gain Citation2017). It is also an important guarantee for achieving the goal of disaster risk reduction and improving post-disaster reconstruction capacity as proposed in the UN Sendai Framework for Disaster Risk Reduction 2015-2030 (United Nations Office for Disaster Risk Reduction Citation2015). In recent years, the international community has come to recognize that coping with and reducing disasters and mitigating the damage they cause while enabling countries to develop sustainably is one of the key challenges facing the international community.
Emergency shelters play a pivotal role in disaster response and post-disaster recovery, and in recent years, the construction of emergency shelters has been incorporated by cities into their respective planning. UN report shows that disaster risk assessments for housing, schools, health facilities and workplaces are an important part of land use policy development (Centre for Research on the Epidemiology of Disaster, United Nations Office for Disaster Risk Reduction Citation2016). Therefore, the evaluation and optimization of the number and spatial location of emergency shelters is not only helpful for the development of policy, but also an important element of natural disaster emergency response and urban infrastructure layout planning. Spatial accessibility is a commonly used method to assess the reasonableness of the layout of announcement facilities. There are various interpretations of spatial accessibility (Liu Citation2007). However, it essentially refers to “the ease of getting from one place to another,” which reflects the ease of communication between the starting point and the end point overcoming various obstacles in space, and is the basic law of human activity. Spatial accessibility is measured by three main aspects: supply, demand, and the way they are connected (Hansen Citation1959; Shen Citation1998; Luo and Wang Citation2003). As an important indicator to assess the rationality of infrastructure layout, spatial accessibility is often used in studies on the rationality and equity of the distribution of public facilities such as parks, medical facilities, schools, and shelters (Guagliardo Citation2004; Tang et al. Citation2017; Lee et al. Citation2020).
In the past few decades, scholars have developed various metrics appropriate to the problem of spatial accessibility assessment for different types of facilities. The classical methods are the proportional method (Guagliardo Citation2004), the nearest distance method, the method based on spatial interactions (Spencer and Angeles Citation2007; Salze et al. Citation2011; Wang Jiao-e Citation2012; Luo Citation2014), and the method based on chance accumulation (Radke and Mu Citation2000; Luo and Qi Citation2009; Song et al. Citation2010). Each of these methods has its own advantages and disadvantages. The proportional method tends to compare between units, but it cannot reveal the variation of accessibility within larger study units, the nearest distance method cannot consider the situation of multiple surrounding facilities, the method based on spatial interactions is more complex and has a certain abstraction, and some of the parameters needed in the calculation are difficult to obtain. The 2FSCA method, which is based on chance accumulation, is easy to understand and relatively simple to operate, but lacks consideration of distance decay. To address the potential problems of 2SFCA, researchers have developed D2SFCA, M2FSCA, CB2SFCA, E2SFCA, 3FSCA and G2SFCA by introducing different assumptions (Delamater Citation2013; McGrail and Humphreys Citation2014), considering different application scenarios (Fransen et al. Citation2015), and using different attenuation functions (Luo and Qi Citation2009; Zhuolin and Yang Citation2016), and competition between facilities (Wan et al. Citation2012; Jörg and Haldimann Citation2023). G2SFCA introduces a Gaussian function as the distance decay function, which considers the scale and spatial obstruction of the supply and demand sides, and is easy to understand and more accurately reflects the relationship between supply and demand sides, while having a stronger ability to simulate the actual situation, and is more suitable for the study of the accessibility of emergency evacuation sites, and therefore has been widely used.
While improving the accessibility evaluation model, researchers have continued to refine and deepen the study scale, gradually improving the spatial scale and analysis granularity of the study. The basic unit of accessibility research has further developed from administrative units such as counties, districts, and towns to more detailed units such as communities and settlements, and the study population has developed from a wide range of people to different age, gender, and cultural subgroups, and the time scale has been refined to day and night and different time periods. For example, Tang et al. (Citation2017) analyzed the accessibility of emergency shelters at the community scale in Yuexiu District, Guangzhou. Liu et al. (Citation2022) analyzed the differences in accessibility to disaster-sheltered green spaces in Nanjing at four time periods using G2FSCA. Kocatepe et al. (Citation2016) developed a GIS-based transportation accessibility analysis of the aging population in Florida. Nakai et al. (Citation2021) considered safe evacuation routes for children with neurodevelopmental disorders in Japan and conducted a safe place accessibility analysis for this population. These fine-scale, population-specific accessibility studies provide an example of how to conduct accessibility studies of emergency shelters at the settlement scale. These fine-scale, population-specific accessibility studies provide an example of how to conduct accessibility studies of emergency shelters at the settlement scale.
For areas with poor spatial accessibility, new supply facilities are needed to improve the situation. The objectives of disaster shelter location allocation models are usually to minimize evacuation time or distance, the cost or number of shelters to be built, and the risk during a disaster (Ma et al. Citation2019). Currently, commonly used methods for siting facilities include mathematical planning and fuzzy decision models, multi-criteria optimization models based on GIS technology, and Swarm intelligence based meta-heuristic algorithms such as genetic algorithm (GA) and particle swarm optimization (PSO). Mathematical programming and fuzzy decision model solve the optimal location problem by solving extreme value problems under linear constraints (Anjos and Vieira Citation2017), which focuses more on optimization calculations and is more suitable for small-scale linear planning and cannot solve the optimal siting of emergency shelters. Using GIS spatial multi-criteria decision-making technology as the theoretical basis, the site selection factors are analyzed for suitability using various methods such as superposition analysis, buffer zone analysis, and network analysis, and the optimal site location is selected to meet the conditions (Voets et al. Citation2013; Fang et al. Citation2014; Wei and Murray Citation2014; Xu et al. Citation2019). Researchers usually develop different optimization functions according to different refuge requirements and use other algorithms to solve them. Commonly used algorithm includes Swarm intelligence based meta-heuristic algorithms. GA has a long history of being used for location optimization (Reese Citation2006). Hosage and Goodchild (Citation1986) applied the GA to location allocation in 1986. Li and Yeh (Citation2005) proposed the use of GA to solve the problem of optimal siting of facilities. Zhang et al. (Citation2020) provided a research tool for siting emergency shelters based on a multi-objective constrained siting model, which was solved using the GA. Doerner et al. (Citation2009) proposed a model for siting tsunami disaster shelters based on a non-dominated ranking GA with the weighted average of the minimized minimum and maximum distances as the objective function. Zhao et al. (Citation2015, Citation2017) introduced an earthquake shelter allocation model, with the objectives of minimizing the total weighted evacuation time and the total area of shelters, and solved it using a modified PSO algorithm combined with the SA algorithm. Gu et al. (Citation2010) proposed the use of 2SFCA and a heuristic population intelligence algorithm to optimize the spatial layout of medical facilities. Zhao et al. (Citation2018) used the PSO algorithm to carry out the siting of emergency shelters under earthquake conditions in Yunnan, China. Tao et al. (Citation2014] used the minimization of the difference in accessibility from the elderly population to the elderly facilities in Beijing in 2020 as the optimization objective function and used the PSO algorithm to solve the objective function. Compared with the GA algorithm, the PSO algorithm omits the cumbersome crossover and mutation, and the whole running process is the process of particles searching for the optimal solution, which has the advantages of fast convergence speed and fewer input parameters. Therefore, this study uses the PSO algorithm to solve the location of emergency shelters.
The eastern edge of the Tibetan Plateau in China has a special geological structure with many large fracture zones and seismic zones, and there is a possibility of large-scale earthquakes. Part of Deyang City is located on the Longmen Mountain seismic zone on the eastern edge of the Qinghai-Tibet Plateau topographic terrain edge zone, which is categorized as a medium-low seismic risk zone with certain seismic risks (Zhang et al. Citation1999, Citation2009). A study on the accessibility of emergency evacuation facilities for cities in the region can reveal the current distribution of the service capacity of urban evacuation sites and improve the capacity of cities for earthquake relief and post-disaster reconstruction. There have been several studies on the accessibility of the area, hospital accessibility, emergency services accessibility and other indicators (Ding et al. Citation2018; Zhong et al. Citation2022). However, in the current research, the demographic data in the street, community or residential unit is mostly used to estimate the population size, and the statistical unit is used as the basic unit for calculating the accessibility, assuming that the population in the basic unit is evenly distributed. This approach will greatly reduce the reliability of the reachability calculation results (Zhang and Gao Citation2012; Tao et al. Citation2014; Liu et al. Citation2022). Most of the existing research uses methods such as qualitative analysis and minimum impedance model to optimize site selection. There are few cases of site selection optimization using Heuristic-based population intelligence optimization models, and the existing research results focus on economically developed cities. For example, in economically developed plain cities such as Beijing and Shenzhen, the research on high-earthquake-risk cities such as Deyang City in Sichuan Province is even more lackluster.
To address the above issues, this paper uses data from the Deyang City Emergency Shelter List published on the Sichuan Data Open website, combined with other open geographical and environmental backgrounds, population spatial distribution, and other data, to analyze the number of emergency shelters supplied and the extent to which they meet actual needs on a small community scale in Deyang City. The G2FSCA method is used to calculate the spatial accessibility of emergency shelters and based on the results of the spatial accessibility analysis, combined with the PSO algorithm, suggestions are made for the future optimization of the location optimization of emergency shelters. This paper attempts to answer the following 3 questions:
Is the spatial distribution of shelters in Deyang city reasonable and meet the specification requirements?
What is the spatial accessibility of emergency shelters in Deyang City?
How should the emergency shelters in Deyang City be planned and laid out in the future?
2. Study area, data, and methods
2.1. Study area
Deyang City () is in the northeastern part of the Chengdu Plain in Sichuan Province, between Mianyang City and Chengdu City. The latitude and longitude range is between 30.527589°N and 31.700507°N and between 103.793173°E and 105.171898°E. Deyang City has a total area of about 5911 km2, with 6 districts and counties, namely Jingyang District, Luojiang District, Guanghan City, Mianzhu City, Shifang City and Zhongjiang County, including 187 townships and street offices (People’s Government of Deyang Citation2022). According to the data of the 7th census in 2020, Deyang city has a registered population of 3.815 million and a resident population of about 3.456 million, including 828,200 in Jingyang District, 209,000 in Luojiang District, 626,000 in Guanghan City, 440,000 in Mianzhu City, 406,800 in Shifang City and 946,000 in Zhongjiang County (People’s Government of Deyang Citation2021).
Figure 1. Basic information of Deyang. MZ: Mianzhu; SF: Shifang; LJ: Luojiang; JY:J ingyang; GH: Guanghan; ZJ: Zhongjiang.

Deyang City is in the transition zone from Longmen Mountains to Sichuan Basin, and is situated in the combination of Himalayan, Pacific Ocean, and ancient Asian tectonic domains, with an elevation of 308 and 4984 m and a relative height difference of 4776 m. The topography of the city includes mountains, hills, and plains, with mountains in the northeast, plains, and hills in the central and south. The plain area of the city accounts for 30.8% of the total area, the mountainous area accounts for 19.7% of the total area and the hilly area accounts for 49.5% of the total area. The cities of Mianzhu and Shifang under the jurisdiction of Deyang City are located on the Longmenshan Fault Zone, and the whole territory is susceptible to earthquakes and the activities of the Fault Zone (Li et al. Citation2019).
2.2. Basic data and pre-processing
The underlying data used in this study are shown in . The administrative zoning data were obtained through the Geographic Open Data website, which is 2021 data, but none of the administrative divisions in Deyang City has been adjusted in recent years, so they can be used in this study. The emergency shelters data are obtained from the Deyang Data Open Network, with a total of 196 emergency shelters. The types of emergency shelters include sports centers and various educational sites (such as universities, secondary schools, vocational schools, elementary school), squares, parks, etc. Emergency shelters were divided according to the size of the level, including an area of more than 50,000 m (Centre for Research on the Epidemiology of Disaster, United Nations Office for Disaster Risk Reduction Citation2020) for Class I, an area between 10,000 m2 − 50,000 m (Centre for Research on the Epidemiology of Disaster, United Nations Office for Disaster Risk Reduction Citation2020) for Class II, and an area of less than 10,000 m (Centre for Research on the Epidemiology of Disaster, United Nations Office for Disaster Risk Reduction Citation2020) for Class III (Ministry of Housing and Urban-Rural Development of the People’s Republic of China Citation2015). There are 34 Class I emergency shelters, 45 Class II shelters, and 117 Class III shelters and emergency shelters in the study area, and their distribution is shown in .
Table 1. Main data and sources.
The road data used for the study was obtained from OSM (Open Street Map), and dates from 2022. The dataset is in shp format and contains vectorized data on various types of features. The OSM data has good accuracy in urban areas and can be used in the study (Zhang and Haitao Citation2019; Zhou and Yijiang Citation2020). The authors combined the road types in the data and classified them into highways, urban primary, secondary and tertiary arterial roads, and residential roads based on the actual situation. Before use, road connectivity was checked, topological errors in it were corrected, road centerlines were extracted, and the Deyang city road network dataset was created (). In the process of establishing the network dataset in Arcgis, a turning model was added to the dataset, no elevation data was used, impedance was set to distance, and no road travel direction was set because the consideration was the accessibility of refuge places in the case of residents on foot.
Figure 2. General situation of data. (a) Road net of Deyang city. (b) Distribution of settlements and population density (person/100 m2). The label is the number of people in each district.

The residential point data were obtained through AMap, which includes the coordinates, name, and type of residential neighborhoods and other fields in the format of point data, with a total of 1888 residential neighborhoods in the study area. Their distribution is shown in .
WorldPop population spatial distribution data for China in 2020 were used in the study (Tatem Citation2017). Data resolution of 3 radians, approximately 100 m (). The data was generated from census data combined with remote sensing images by applying random forest.
2.3. Methods
2.3.1. Technical route
The overall technical route is shown in . First, the authors analyze the overall level and spatial characteristics of the construction of emergency shelters in the study area in terms of the quantity of supply and spatial distribution patterns. Then, based on the spatial data of emergency shelters, residential neighborhoods, and spatial distribution of population, the authors applied the Gaussian two-step floating catchment area method based on the network analysis function of ArcGIS to evaluate the accessibility of emergency shelters and reveal the areas with poor spatial accessibility (accessibility blind areas). Finally, for the above accessibility blind areas, the authors use the K-means method to perform clustering and plot the relationship between the number of clusters and the path length, thereby determining the number of new emergency shelters. And then the particle swarm algorithm is used to simulate and optimize the location of the new emergency shelter and finally propose the site selection.
2.3.2. G2SFCA method
The G2SFCA method is based on the two-step shift search method, which is generated by introducing a Gaussian decay function. The steps are mainly the following two parts (Park and Goldberg Citation2021):
First, the supply-demand ratio is calculated for the supply point. Take the supply point
as the center, take the maximum service radius
as the search range, get the demand point
of the service radius, and get the potential demand in the search area. Subsequently, the ratio
of supply capacity
at supply point to potential demand
at demand point is calculated:
(1)
(1)
In this study, is the residential site,
is the emergency shelter.
is the capacity, which is the area of emergency shelter in the study.
is the demand size, which is the population size in the study.
is the number of cells in the search range.
is the service area of different levels of shelters. In this study, different service areas are divided for different levels of shelters, where the service area of Class I shelters is 5000 m, Class II shelters is 1000 m, and Class III shelters is 500 m.
is the distance between demand point
and supply point
The final obtained supply/demand ratio
is in units of area per capita (m2/person).
Subsequently, a search for a refuge within the reachable range
is performed with the demand point
as the center. The Gaussian distance decay function is used to sum up the supply-demand ratio
of the emergency shelters within the reachable range after decaying to obtain the reachability
of demand point
(2)
(2)
where
is the accessibility of the emergency shelter at the cell site, expressed as area per capita (m2/person) in this study, and its larger value indicates the higher accessibility level of the residential site to the shelter.
is the number of emergency shelters searching radius
with demand point
as the core. The service radius of a type of shelter in the national standard should be greater than 2.5 km (Ministry of Housing and Urban-Rural Development of the People’s Republic of China Citation2015), and considering that the emergency shelter should be reached within 15 min in case of a disaster, and the average walking speed of an ordinary person is 180 m/min, the search radius
is set to 2700 m in this study.
is the ratio of supply to demand obtained from Equationequation (1)
(1)
(1) .
is the Gaussian distance decay function:
(3)
(3)
In the study, the authors used ArcGIS 10.2 network analysis function to obtain the accessibility of residential points to emergency shelters using service area analysis and OD matrix, centered on supply and demand points, respectively, to implement the Gaussian two-step movement search method for subsequent analysis.
2.3.3. Accessibility blind clustering based on K-means
K-means clustering is a classical unsupervised machine learning method. It calculates the distance between class cluster centroids and sample points by minimizing, or no longer varying (MacQueen Citation1967). The steps are: the data is divided into k groups in advance, then k objects are randomly selected as the initial cluster centers, then the distance between each object and each seed cluster center is calculated, and each object is assigned to the cluster center closest to it.
(4)
(4)
where
denotes the i-th object in the data,
denotes the j-th cluster center, denotes the t-th attribute of the ith object, and
denotes the tth attribute of the jth cluster center. When all sample points are assigned, the centroid of the k class clusters is recalculated, which is the optimal solution with the most reasonable distance of all sample points in each direction within the class clusters, and is calculated as follows:
(5)
(5)
where
denotes the i-th cluster center,
denotes the number of objects in the l-th cluster, and
denotes the i-th object in the l-th cluster.
In the study, the authors completed a K-means clustering analysis with the support of MATLAB software. The specific steps are: firstly, based on the national standard (Zhao et al. Citation2022), the points with poor accessibility are extracted and called accessibility blind areas. Through ArcGIS software, we obtained the latitude and longitude coordinates of the accessibility blind areas, and then performed the cluster analysis based on the coordinates of the accessibility blind areas: by setting different numbers of cluster centers, we obtained the relationship curves between the coordinates of cell points and the maximum distance of cluster centers, and carried out the analysis based on the curve shape to provide guidance for the subsequent optimization of the particle swarm algorithm.
It is worth noticing that the results of the K-Means spatial clustering method only consider the distance between the sample points and the cluster centers, and the optimization cannot be carried out by applying other attributes of the sample points, so the obtained center coordinates are not reasonable. In this paper, we further use the particle swarm algorithm to optimize and plan the coordinates of new emergency shelters more finely based on the optimal number of clustering centers provided.
2.3.4. Site selection optimization method based on PSO algorithm
The particle swarm algorithm (PSO) (Kennedy and Eberhart Citation1994) is based on the motion of the particles within the population and continuously optimizes the position coordinates of all particles in the population by sharing the optimal solution in a single search, and finally the active population obtains the optimal solution within the range.
In the PSO algorithm, the individual particle searches for the individual optimal solution by moving at the velocity
and the current position
Meanwhile, the population optimal solution
is obtained by sharing information within the population, and the velocity and position for the next search step are adjusted based on the difference between them until they are equal or the deviation is less than a set threshold. The expressions are as follows.
(6)
(6)
(7)
(7)
where
becomes the inertia factor,
is the velocity of particle
in the k-th iteration;
is the position of particle i in the k-th iteration;
is the position of the individual pole point of particle i,
is the position of the global pole point of the whole population,
are random numbers between [0,1],
are non-negative constants called acceleration factors.
In the study, the authors based on MATLAB POS toolkit to carry out the optimization of additional refuge site selection. The specific parameters in the study are set as follows: the particle search range is the boundary range under the WGS84 coordinates of the study area, and the particle motion speed is 20% of the boundary coordinate change range. The objective optimization function is the sum of Euclidean distances considering the accessibility of current residential sites (emergency shelter sites to accessibility blind points), i.e. the sum of the products of distances and accessibility normalized values, and its expression is:
(8)
(8)
where
is the accessibility of the cell,
is the maximum value of accessibility.
are the latitude and longitude coordinates of the residential point
are the current individual optimal solution coordinates of the particle. This optimization function can prioritize the residential areas with low current accessibility, with a weight of 1 when the accessibility is 0. The higher the accessibility of the current residential point, the lower the weight given to that point. Bringing new emergency shelters closer to neighborhoods with low current accessibility.The optimization process is set up for 3000 iterations of optimization, and when the optimal solution of the population does not change for 200 consecutive iterations, or the difference between the individual optimal solution and the global optimal solution is less than 1e-25, the iteration is stopped and the coordinates of the site selection point are output. Finally, the specific address information of the new emergency shelter is obtained by the coordinate backchecking tool.
3. Analysis of results
3.1. Number and spatial distribution of emergency shelters
By 2020, Deyang City will have built a total of 196 emergency shelters at all levels, including 110 Class I emergency shelters, 59 Class II shelters and 27 Class III shelters, including sports centers, universities, high schools, vocational schools, elementary school, squares, parks, green spaces, parking lots, and warehouses. The total area of evacuation sites is 57.91 × 105 m2, the planned number of evacuees is 10.887 × 105 people, and the planned area of evacuation sites per capita is 5.32 m2/person. The construction of emergency shelters in each district is shown in .
Table 2. Statistics for the construction of emergency shelters in Deyang.
The overall level of construction of emergency shelters in the study area is high, and the total area of single places, per capita area and other indicators are higher than national standards (Ministry of Housing and Urban-Rural Development of the People’s Republic of China Citation2015). The area of emergency shelter per capita in the region is 1.63 m2/person, 81% higher than the national standard of 0.9 m2/person. Within the study area, the number of emergency shelters, total capacity, and per capita area for disaster avoidance in different administrative regions also varied significantly. Among them, Luojiang District has the smallest per capita area of emergency shelters (1.55 m2/person) and Shifang District has the largest per capita area (7.67 m2/person); Jingyang District has the largest number of shelters, with 107 in total, and Luojiang District has the least number of emergency shelters, with only 2. The utilization rate of emergency shelters in urban areas is high and the population served is large, while the utilization rate of shelters set up in rural areas is low and the population in the service area is small.
Spatially, the emergency shelters in Deyang City are mainly distributed in the main city of the study area, Jingyang District, accounting for 54.5% of the total number of shelters, and the distribution of shelters in Jingyang District is more dispersed, which can satisfy most of the areas in the district. The distribution of emergency shelters in other counties in the study area is less and more concentrated, mostly in the townships around the main urban areas (). High overlap between the distribution trends of shelters and population density distribution. With the support of ArcGIS, the standard deviation ellipse map, which characterizes the spatial distribution trend of emergency shelters, can be drawn. In this figure, the long half-axis indicates the direction of the spatial distribution of data points, and the short axis indicates the extent and density of the spatial distribution of data points. As can be seen from , the distribution of emergency shelters in most counties and districts has some directionality, with emergency shelters in Jingyang District and Shifang City showing the pattern of NNE-SSW directional distribution, emergency shelters in Mianzhu City showing E-W directional distribution, and shelters in Zhongjiang County, Luojiang District and Guanghan City being more concentrated with no obvious directionality in distribution.
3.2. Accessibility of emergency shelters
To show the distribution of accessibility in Deyang city region, the authors use ordinary kriging interpolation within Arcgis to interpolate the accessibility of each resident to obtain the spatial distribution of accessibility in Deyang. In the calculation process, the interpolated area is set to be the administrative boundary of Deyang city, and the variation function is Spherical, cell_size size is 30 m, and other parameters are set by default. Finally, the interpolation results are rendered to obtain the bottom map in . Overall, the accessibility of neighborhoods located in the center of each district and county in the study area is better due to the dense road network in the city center, the high number of shelters, and the ease of access within walking time. The accessibility in rural areas of counties and districts, such as the plain areas in the southeast of Zhongjiang County and the mountainous areas in the north of Mianzhu City, is low because of the small number of emergency shelters in these areas, the sparse road network, and the complex terrain, which makes it difficult for residents to reach shelters in a short time. The accessibility distribution of each county starts to decrease from the center outward, showing a “high-low” distribution pattern.
Figure 5. Accessibility of settlements. (a) Accessibility and distribution of accessibility calculated by kriging method for all settlements in Deyang City. The closer to the city center, the higher the accessibility. (b) and (c) Local accessibility distribution. The accessibility of settlements is mainly affected by roads and emergency shelters.

Statistics at the settlement level show that the accessibility varies significantly between the different settlements. In the worst settlement, the accessibility value is 0, and in the best settlement, the accessibility is 119.06 m2/person. The number of people who can reach the nearest emergency shelter within 15 min is 1,415,600, accounting for 40.96% of the city’s population in 2020.
On the basis of the above, the accessibility scores of each county and region can be further calculated (). Statistics show that the average accessibility of each county and district varies greatly, with the lowest accessibility of 1.56 m2/person in Mianzhu City and the highest accessibility of 7.67 m2/person in Shifang City. The accessibility of all districts is higher than the specification (0.9 m2/person).
Figure 6. Districts accessibility. (a) The accessibility of each area is marked on the map and is obtained by averaging the accessibility of all cells in the area. (b) Distribution of low accessibility.

There are a certain number of weakly accessible cells in each county and district. In this study, 187 cells with accessibility indexes lower than the national specification requirement (0.9 m2/person) are extracted separately as accessible blind areas (). It can be found that the distribution of weakly accessible neighborhoods is scattered, mostly in remote areas with limited access. In terms of the number of accessible blind areas, there are 6 in Jingyang District, accounting for 0.73% of the total number of cells in the region, 48 in Guanghan City, accounting for 14.1% of the total number of cells in the city, 13 in Luojiang District, accounting for 13.1% of the total number of cells in the region, 47 in Mianzhu City, accounting for 21.2% of the total number of cells in the city, 27 in Shifang City, accounting for 12.9% of the total number of cells in the city, and 46 in Zhongjiang County, accounting for 22.1% of the total number of cells in the county. 22.1%.
3.3. Site selection for new emergency shelters
In order to improve the emergency evacuation capacity of communities with very weak accessibility, it is necessary for the local government to build new emergency shelters according to the problems in the spatial layout of existing emergency shelters and the regional development and spatial distribution of the regional population, so as to reduce the travel distance and time for the regional population to reach the emergency shelters and improve the spatial accessibility level of the whole region.
To this end, in this paper, the above 187 accessibility blind areas are clustered based on the K-means algorithm, and the relationship between the number of clusters and the maximum distance traveled by weakly accessible cell sites is analyzed (). The results show that at least 26 new emergency shelters are needed to make the average distance of all weakly accessible cell points to the cluster center less than 2700 m.
Figure 7. The relationship between the number of new points and average max distance and its derivative curve.

Considering the realistic possibility of urban construction in the next 1-2 years, this study determines the number of new emergency shelters as 10 Class III emergency shelters. The authors applied particle swarm optimization algorithm to determine the best location for the 10 new emergency shelters (). The results show that:
Figure 8. New emergency shelters in Deyang. GH1: Near the sixth kindergarten of Guanghan City; GH2: Near Qingbaijiang Bridge; JY1: Near Baochantian Leisure Village; LJ1: Near the intersection of Qionghai Road and Xingfu Road; MZ1: Near Hanwang Wetland Park; SF1: Near the Tang compound; SF2: Near the intersection of Tudang Road and Chuanxi Ring Road; ZJ1: Near the intersection of the second section of the Second Ring Road East and Lion Hill Avenue; ZJ2: Near Renshouzhai Village; ZJ3: Near Sanjiang Village.


Guanghan City needs to add 2 emergency shelters, GH1 is located near the sixth kindergarten of Guanghan City and GH2 is located near Qingbaijiang Bridge. Jingyang District should add 1 emergency shelter, which is located in the leisure village of Baobangtian (JY1). Luojiang District should add 1 emergency shelter, located near the intersection of Qionghai Road and Happiness Road (LJ1). Mianzhu City should add 1 emergency shelter located near Hanwang Wetland Park (MZ1), which can be directly converted using the park. Shifang City should add 2 emergency shelters, SF1 located near Tangjia Dayuanzi and SF2 located near the intersection of Tudang Road and Chuanxi Ring Road. Zhongjiang County should add 3 new emergency shelters, ZJ1 is located near the intersection of the second ring road east section 2 and Lishan Avenue, ZJ2 is located near Renshuzhai Village in Zhongjiang County, and ZJ3 is located near Sanjiang Village in Zhongjiang County.
Simulations of the city after the new shelters were built show that after the 10 new Class III emergency shelters were built according to the above scheme, there are 81 weakly accessible neighborhoods within 15 min of the shelter, accounting for 43.31% of all accessible blind areas. The number of people who can reach the nearest emergency shelter reaches 1556049, accounting for 45.02% of the city’s population; this is 140449 higher than the population that can be served by the existing spatial layout, and the population share is increased by 4%.
4. Discussion
4.1. Distribution and policy recommendations
This study found that the building area and per capita building area of emergency shelters in Deyang City exceed the requirements of relevant Chinese national standards and regulations. In Jingyang District of Deyang City, there are many emergency shelters, and the spatial distribution is relatively reasonable, which can cover most of the residential areas in the urban area. In other counties, there is a serious imbalance in the spatial accessibility of emergency shelters. Studies have shown that the differences in accessibility at the county level are mainly due to differences in local economic development, urbanization levels, and administrative regions (Tang et al. Citation2020). Especially in the mountainous and rural areas that are far away from urban areas, the accessibility of shelters in the entire city presents a “high-low” distribution pattern from the city center to the outside. This research result is also consistent with the problem raised by related studies that underdeveloped areas face a mismatch between refuge needs and shelters (Wei et al. Citation2020). This problem also exists widely in other cities in China (Zhang and Gao Citation2012; Liu et al. Citation2022; Wang et al. Citation2022) and in other cities around the world (Kocatepe et al. Citation2016; Unal and Uslu Citation2016; Cao et al. Citation2017; Coles et al. Citation2017). This shows that whether it is in Deyang City, or in the construction of emergency shelters in other cities in China, as well as in other developed and developing cities around the world, there is still a long way to go before completing the requirements of the Sendai Framework for Disaster Risk Reduction 2015-2030. Large distances (United Nations Office for Disaster Risk Reduction Citation2015). Especially in China, there are 509.79 million people living in rural areas, accounting for 36.11% of the total population (Office of the Leading Group of the State Council for the Seventh National Population Census Citation2021), which makes it even more urgent to improve disaster avoidance capabilities in rural areas.
This study recommends that 1) there is a need to examine the service capacity of emergency shelters for settlements in rural and peri-urban areas in areas with associated natural risk hazards worldwide. 2) The equity of shelters should be looked at by local governments. Making emergency shelters accessible to most residents the issue of facility equity has been an important issue in accessibility studies, and in most similar studies there is inequity in the accessibility of facilities (Delamater Citation2013; Yang et al. Citation2016; Xiao et al. Citation2017). For developing cities, urban sprawl should be controlled and reduced (Unal and Uslu Citation2016), and the duplication of emergency shelter construction should be reduced (Fan et al. Citation2017). 3) To strengthen the emergency shelter capacity in villages in the short term, the widely available open spaces and public facilities in rural areas, such as village council squares, farmers’ markets, grain drying yards, and large open spaces, can be used to carry out the necessary foundation and building reinforcement and store certain disaster-resistant emergency This is similar to the findings of related studies (Liu et al. Citation2011) It is worth mentioning that in the polish town of Shifang City, small emergency shelters have been set up in the village committees of each village, which can meet the needs of each village for shelter, greatly increasing the coverage of the shelters and improving the ability of people in rural areas to escape in a disaster. This approach may be a typical solution for building emergency shelters in villages.
4.2. Uncertainty analysis
The accuracy of the results obtained in this study may have some bias in some specific regions. Firstly, the data used in the study are the OSM map dataset and WorldPop population spatial distribution data, which are subject to certain limitations in terms of accuracy in Chinese regions due to policy and law and research methods, etc. Some scholars have pointed out that the accuracy of OSM data in some regions is constrained by the number of quality uploaders and may not be of high quality in rural areas (Zhang and Haitao Citation2019; Zhou and Yijiang Citation2020). Secondly, the 2SFCA method in the study still has some room for improvement, such as improving the decay function (Tao et al. Citation2014; Kocatepe et al. Citation2017) and considering the supply and demand relationship (Wan et al. Citation2012; Luo Citation2014).
In terms of optimization algorithm parameter setting, the study uses the average of the crowd movement speed to move 15 min, both 2700 m. In real life, the crowd walking speed is also influenced by many factors, such as age, road conditions, etc., so there is a certain range of flexibility in the choice of the reachable range of cell points. the optimization function of the PSO algorithm uses the current reachability of the cell as a weight, making the new emergency shelter points more accessible. The PSO algorithm’s optimization function uses the current accessibility of the plot as a weight, so that new emergency shelter points are located closer to poorly accessible plots, which would give different results if other data on the plot points were used as weights, such as population and area. The application of different optimization functions and more accurate data, using dynamic data to analyze accessibility at different moments in time (Wu et al. Citation2018), maybe the next step in the research.
5. Conclusions
Based on various public geographic data resources such as government public data, road network data, POI data, and population spatial distribution data, this paper uses the Gaussian two-step movement search method to analyze the accessibility of shelters in six counties and districts of Deyang City, clarify the accessibility blind areas, propose the number and location of new emergency shelters and uncertainties. This study is a typical example of a study that combines spatial accessibility assessment and intelligent algorithms for emergency shelter site optimization and policy recommendations to serve early warning and risk assessment of mountain hazards in areas with high seismicity.
This study points out that the overall level of emergency shelters within Deyang city is high, but there is an uneven distribution of resources; urban areas are better than rural areas, and central areas are better than peripheral areas, and in the future, 10 Class III emergency shelters can be built to improve Deyang city’s overall emergency shelter capacity. At the same time, the local government should focus on the construction of emergency shelters in rural areas in future urban planning, and take targeted measures to make up for the lack of disaster resilience in rural areas.
This study also points out that it is feasible to carry out emergency shelter accessibility analysis and site optimization for cities in highly disaster-prone areas based on multivariate open-source geographic data, combined with 2SFCA and PSO algorithms. The technical route can offer the possibility of learning from other cities and regions. However, the method also suffers from insufficient data accuracy and a lack of dynamic information. In the future, we should improve the algorithm for urban evacuation capacity evaluation and evacuation facility siting based on improving the accuracy of the basic data.
Author contribution
Conceptualization: Yunfeng Hu; methodology: Zuopei Zhang, Yunfeng Hu; formal analysis and investigation: Zuopei Zhang; writing – original draft preparation: Zuopei Zhang; writing – review and editing: Zuopei Zhang, Yunfeng Hu, Wei Lu, Wei Cao; visualization: Zuopei Zhang; project administration: Yunfeng Hu; funding acquisition: Yufeng Hu; supervision: Yunfeng Hu. All authors have read and agreed to the published version of the manuscript.
Data deposition
The data used in the study are publicly available, and the way in which they were obtained is shown in .
Acknowledgments
We thank all authors for their contributions to this study. We thank the Network Security and Information Program of the Chinese Academy of Sciences (CAS-WX2021SF-0106),the National Natural Science Foundation of China (41977421) and the Key Project of Innovation LREIS(KPI011) for support.
Disclosure statement
The authors declare no conflict of interests.
Data availability statement
The data used in the study and the access to them are available in . The code used during the current study is available from the corresponding author on reasonable request.
Additional information
Funding
References
- Anjos MF, Vieira MVC. 2017. Mathematical optimization approaches for facility layout problems: the state-of-the-art and future research directions. Eur J Oper Res. 261(1):1–16.
- Cao L-S, Zhong-Feng Z, Xia, Tian T, Tai-Ho K. 2017. A Study on accessibility of disaster-prevention Green Space for earthquake avoidance – focused on Jung-gu and Nam-gu Office, Ulsan Metropolitan City. J Korean Instit Land Archit. 45:90–97.
- Centre for Research on the Epidemiology of Disaster, United Nations Office for Disaster Risk Reduction. 2016. Poverty & death: disaster mortality 1996-2015. Switzerland: United Nations Office for Disaster Risk Reduction. p. 20.
- Centre for Research on the Epidemiology of Disaster, United Nations Office for Disaster Risk Reduction. 2020. The human cost of disasters: an overview of the last 20 years (2000-2019). Center for Research on the Epidemiology of Disasters (CRED). p. 30.
- Coles D, Yu D, Wilby RL, Green D, Herring Z. 2017. Beyond ‘flood hotspots’: modelling emergency service accessibility during flooding in York, UK. J Hydrol. 546:419–436.
- Delamater PL. 2013. Spatial accessibility in suboptimally configured health care systems: a modified two-step floating catchment area (M2SFCA) metric. Health Place. 24:30–43. doi:10.1016/j.healthplace.2013.07.012
- Ding S-x, Zhong M-l, Pu-Yun Y, Wei Z, Xiao MA. 2018. Capability and spatial accessibility of the emergency service at township hospitals in Fushun County, Sichuan. Chin J Health Policy. 11(2):69–73.
- Doerner KF, Gutjahr WJ, Nolz PC. 2009. Multi-criteria location planning for public facilities in tsunami-prone coastal areas. OR Spectr. 31(3):651–678.
- Fan C, Zhai G, Zhou S, Zhang H, Qiao P. 2017. Integrated framework for emergency shelter planning based on multihazard risk evaluation and its application: case study in China. Nat Hazards Rev. 18(4).
- Fang F, Xu L, Can L, Qian X. 2014. Review of spatial multi-crtteria decision making. Sci Survey Map. 39:9–12.
- Fransen K, Neutens T, De Maeyer P, Deruyter G. 2015. A commuter-based two-step floating catchment area method for measuring spatial accessibility of daycare centers. Health Place. 32:65–73. doi:10.1016/j.healthplace.2015.01.002
- Giupponi C, Gain AK. 2017. Integrated spatial assessment of the water, energy and food dimensions of the sustainable development goals. Reg Environ Change. 17(7):1881–1893.
- Gu W, Wang X, McGregor SE. 2010. Optimization of preventive health care facility locations. Int J Health Geogr. 9:17. doi:10.1186/1476-072X-9-17
- Guagliardo MF. 2004. Spatial accessibility of primary care: concepts, methods and challenges. Int J Health Geogr. 3(1):3.
- Hansen WG. 1959. How accessibility shapes land use. J Am Instit Plann. 25(2):73–76.
- Hosage CM, Goodchild MF. 1986. Discrete space location-allocation solutions from genetic algorithms. Ann Oper Res. 6(2):35–46.
- Jörg R, Haldimann L. 2023. MHV3SFCA: a new measure to capture the spatial accessibility of health care systems. Health Place. 79:102974. doi:10.1016/j.healthplace.2023.102974
- Kennedy J, Eberhart R. Proceedings of ICNN’95 International Conference on Neural Networks. 1994. p. 1942–1948.
- Kocatepe A, Ozguven EE, Ozel H, Horner MW, Moses R. 2016. Human aspects of IT for the aged population: healthy and active aging. In ITAP 2016, PT II. p. 407–416.
- Kocatepe A, Ulak MB, Ozguven EE, Horner MW, Arghandeh R. 2017. Socioeconomic characteristics and crash injury exposure: A case study in Florida using two-step floating catchment area method. Appl Geogr. 87:207–221.
- Lee YH, Lee JS, Seung Chan B, Hong WH. 2020. Spatial equity with census population data vs. floating population data: the distribution of earthquake evacuation shelters in Daegu, South Korea. Sustainability. 12(19):8046–8063.
- Li Y, Sun M, Yuan G, Zhou Q, Liu J. 2019. Study on development sustainability of atmospheric environment in northeast China by rough set and entropy weight method. Sustainability. 11.
- Liu X. 2007. General description of spatial accessibility. Urban Trans China. 5:36–43.
- Liu Q, Ruan X, Shi P. 2011. Selection of emergency shelter sites for seismic disasters in mountainous regions: lessons from the 2008 Wenchuan Ms 8.0 Earthquake, China. J Asian Earth Sci. 40(4):926–934.
- Liu W, Xu H, Wu J, Li W, Hu H. 2022. Measuring spatial accessibility to refuge green space after earthquakes: a case study of Nanjing, China. PLoS One. 17(6):e0270035–e0270035. doi:10.1371/journal.pone.0270035
- Liu Y, Yang Y, Li L. 2012. Major natural disasters and their spatio-temporal variation in the history of China. J Geogr Sci. 22(6):963–976.
- Li X, Yeh AG. 2005. Integration of genetic algorithms and GIS for optimal location search. Int J Geogr Inform Sci. 19(5):581–601.
- Luo J. 2014. Integrating the Huff model and floating catchment area methods to analyze spatial access to healthcare services. Trans GIS. 18(3):436–448.
- Luo W, Qi Y. 2009. An enhanced two-step floating catchment area (E2SFCA) method for measuring spatial accessibility to primary care physicians. Health Place. 15(4):1100–1107. doi:10.1016/j.healthplace.2009.06.002
- Luo W, Wang FH. 2003. Measures of spatial accessibility to health care in a GIS environment: synthesis and a case study in the Chicago region. Environ Plann B Plann Des. 30(6):865–884. doi:10.1068/b29120
- Ma Y, Xu W, Qin L, Zhao X. 2019. Site selection models in natural disaster shelters: a review. Sustainability. 11(2):399. https://mdpi-res.com/d_attachment/sustainability/sustainability-11-00399/article_deploy/sustainability-11-00399-v3.pdf?version=1548143424.
- MacQueen J. 1967. Some methods for classification and analysis of multivariate observations. In: Proceedings of the 5th Berkeley Symposium on Mathematical Statistics and Probability 1:281–297.
- McGrail MR, Humphreys JS. 2014. Measuring spatial accessibility to primary health care services: utilising dynamic catchment sizes. Appl Geogr. 54:182–188.
- Ministry of Housing and Urban-Rural Development of the People’s Republic of China. 2022. National Standard "Technical Standards for Disaster Preparedness and ShelterGB 51143-2015 Partially revised provisions. Standardization of Engineering Construction. 283(06):35–38. doi:10.13924/j.cnki.cecs.2022.06.008.
- Nakai H, Itatani T, Kaganoi S, Okamura A, Horiike R, Yamasaki M. 2021. Needs of children with neurodevelopmental disorders and geographic location of emergency shelters suitable for vulnerable people during a tsunami. IJERPH. 18(4):1845.
- Office of the Leading Group of the State Council for the Seventh National Population Census. 2021. Major figures on 2020 population census of China. National Bureau of Statistics.
- Park J, Goldberg DW. 2021. A review of recent spatial accessibility studies that benefitted from advanced geospatial information: multimodal transportation and spatiotemporal disaggregation. IJGI. 10(8):532.
- People’s Government of Deyang. 2021. The seventh census of Deyang.
- People’s Government of Deyang. 2022. Basic information of Deyang. http://data.deyang.gov.cn/dexchange/open/?state=&app_id=5418910&/#/Home.
- Radke J, Mu L. 2000. Spatial decompositions, modeling and mapping service regions to predict access to social programs. Geogr Inform Sci. 6(2):105–112.
- Reese J. 2006. Solution methods for the p-median problem: an annotated bibliography. Networks. 48(3):125–142.
- Salze P, Banos A, Oppert J-M, Charreire H, Casey R, Simon C, Chaix B, Badariotti D, Weber C. 2011. Estimating spatial accessibility to facilities on the regional scale: an extended commuting-based interaction potential model. Int J Health Geogr. 10:2. doi:10.1186/1476-072X-10-2
- Shen Q. 1998. Location characteristics of inner-city neighborhoods and employment accessibility of low-wage workers. Environ Plann B. 25(3):345–365.
- Song ZN, Chen W, Zhang GX, Zhang L. 2010. Spatial accessibility to public service facilities and its measurement approaches. Prog Geogr. 29:1217–1224.
- Spencer J, Angeles G. 2007. Kernel density estimation as a technique for assessing availability of health services in Nicaragua. Health Serv Outcomes Res Method. 7(3-4):145–157.
- Tang B, Danni W, Yun S, Jinan Q, Yongtao Y, Zhihuan Z. 2017. Research on the emergency shelter accessibility in urban communities. J Risk Anal Response. 7(4):230–237.
- Tang X, Deng Y, Yang H, Tian F, Li Y, Pan J. 2020. Spatial accessibility to emergency care in Sichuan province in China. Geospat Health. 15(2):274–284.
- Tao Z, Cheng Y, Dai T, Rosenberg MW. 2014. Spatial optimization of residential care facility locations in Beijing, China: maximum equity in accessibility. Int J Health Geogr. 13:33. doi:10.1186/1476-072X-13-33
- Tatem AJ. 2017. Comment: WorldPop, open data for spatial demography. Sci Data. 4(1):170004. https://doi.org/10.1038/sdata.2017.4.
- Unal M, Uslu C. 2016. GIS-based accessibility analysis of urban emergency shelters: the case of Adana city. Int Archit Photogramm Remote Sens Spatial Inf Sci. XLII-2/W1:95–101.
- United Nations Office for Disaster Risk Reduction. 2015. Sendai framework for disaster risk reduction 2015-2030. p. 32.
- Voets T, Neven A, Thewys T, Kuppens T. 2013. GIS-BASED location optimization of a biomass conversion plant on contaminated willow in the Campine region (Belgium). Biomass Bioenergy. 55:339–349.
- Wan N, Zou B, Sternberg T. 2012. A three-step floating catchment area method for analyzing spatial access to health services. Int J Geogr Inform Sci. 26(6):1073–1089.
- Wang Jiao-e HH. 2012. Accessibility of medium- and small-sized cultural tourist cities based on spatial distance and time cost. J Nat Resour. 27(11):1951–1961.
- Wang X, Guan M, Dong C, Wang J, Fan Y, Xin F, Lian G. 2022. A multi-indicator evaluation method for spatial distribution of urban emergency shelters. Remote Sensing. 14(18):4649.
- Wei Y, Jin L, Xu M, Pan S, Xu Y, Zhang Y. 2020. Instructions for planning emergency shelters and open spaces in China: lessons from global experiences and expertise. Int J Disaster Risk Reduct. 51:101813. doi:10.1016/j.ijdrr.2020.101813
- Wei R, Murray AT. 2014. Evaluating polygon overlay to support spatial optimization coverage modeling. Geogr Anal. 46(3):209–229.
- Wu H, Liu L, Yang Y, Peng Z. 2018. Evaluation and planning of urban green space distribution based on mobile phone data and two-step floating catchment area method. Sustainability. 10. https://mdpi-res.com/d_attachment/sustainability/sustainability-10-00214/article_deploy/sustainability-10-00214.pdf?version=1516167317.
- Xiao Y, Wang Z, Li Z, Tang Z. 2017. An assessment of urban park access in Shanghai – Implications for the social equity in urban China. Land Urban Plann. 157:383–393.
- Xu W, Zhang F, Zeng B, Yang T, Wang H. 2019. Spatial access to medical services in the underdeveloped region of Northwestern China: a case study in Lanzhou City. Sustainability. 11(23):6786. https://mdpi-res.com/d_attachment/sustainability/sustainability-11-06786/article_deploy/sustainability-11-06786.pdf?version=1575031955.
- Yang N, Chen S, Hu W, Wu Z, Chao Y. 2016. Spatial distribution balance analysis of hospitals in Wuhan. IJERPH. 13(10):971. https://mdpi-res.com/d_attachment/ijerph/ijerph-13-00971/article_deploy/ijerph-13-00971.pdf?version=1475234916.
- Zhang W, Gao H. 2012. Vibration, structural engineering and measurement II. p. 2245–2248.
- Zhang Y, Haitao J. 2019. OpenStreetMap traffic data quality assessment and applicability analysis—case study of Henan Railway Network. Geomatics World. 26(4):112–116.
- Zhang X, Shanchu Y, Xiaofei Z. 2020. Optimized location model of resident emergency congregate shelter based on multi-objective constraints. Urban Stud. 27:59–66.
- Zhang ZJ, Wang YH, Chen Y, Houseman GA, Tian XB, Wang ER, Teng JW. 2009. Crustal structure across Longmenshan fault belt from passive source seismic profiling. Geophys Res Lett. 36(17):310–314. doi:10.1029/2009GL039580.
- Zhang PZ, Yang ZX, Gupta HK, Bhatia SC, Shedlock KM. 1999. Global Seismic Hazard Assessment Program (GSHAP) in continental Asia. Ann Geofis. 42:1167–1190.
- Zhao X, Ma Y, Liang P, Qin L, Zhou H, Yuan Y, Xu W. 2018. Analysis of earthquake emergency shelter location selection based on particle swarm optimization algorithm: Wenping of Ludian in Yunnan Province as a case. J Beijing Normal Univ Nat Sci. 54:217–223.
- Zhao X, Xu W, Ma Y, Hu F. 2015. Scenario-based multi-objective optimum allocation model for earthquake emergency shelters using a modified particle swarm optimization algorithm: a case study in Chaoyang District, Beijing, China. PLoS One. 10(12):e0144455. doi:10.1371/journal.pone.0144455
- Zhao X, Xu W, Ma Y, Qin L, Zhang J, Wang Y. 2017. Relationships between evacuation population size, earthquake emergency shelter capacity, and evacuation time. Int J Disas Risk Sci. 8(4):457–470.
- Zhao Y, Xu F, Wan Y. 2022. Spatial accessibility and supply-demand balance analysis method of Park Green Space based on modified gravity model. J Geo-Inform Sci. 24(10):1993–2003.
- Zhong G, Zhai G, Shang K, Chen W. 2022. A two-stage hierarchical model for spatial location and evacuation allocation problem of urban earthquake shelters: a case study in Central urban area of Yangbi county, Yunnan province, China. Geomatics Nat Hazards Risk. 13(1):1721–1761.
- Zhou X, Yijiang Z. 2020. Issues and advances of crowdsourcing geographic data quality. Geomatics World. 27(1):1–7.
- Zhuolin T, Yang C. 2016. Research progress of the two-step floating catchment area method and extensions. Progr Geogr. 35:589–599.