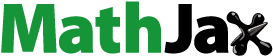
Abstract
Rapid determination of the most-affected areas after an earthquake can help with emergency relief efforts, which is also an essential part of postearthquake relief work. Mobile signaling data contains rich user information, which helps determine the most-affected areas. This study investigated the 2022 Mw 6.6 Luding earthquake in Sichuan, China; the relationship between mobile signaling data and the seismically most-affected area was explored using comparative, regression, and spatial interpolation analysis. The results revealed that mobile signaling data changed abnormally following the earthquake and differed across high- and low-intensity zones. There was a significant correlation between mobile signaling data and intensity. Additionally, the most-affected areas estimated using mobile signaling data were comparable to the actual seismic damage. Therefore, mobile signaling data can provide a reference for determining the most-affected areas and assist in emergency relief efforts after an earthquake.
1. Introduction
On September 5, 2022, an earthquake of Mw 6.6 occurred in Luding, Sichuan Province, China, with the epicenter (29.59°N, 102.08°E) located in the town of Moxi, Luding County, Ganzi Prefecture, Sichuan Province, at a depth of 16 km, the highest intensity of this earthquake reached IX degrees. And it was the strongest earthquake in Sichuan since the 2017 Jiuzhaigou Mw6.8 earthquake, which caused a more serious break in the ring. As of September 11, 2022, the earthquake had caused 93 deaths and 25 people were missing. The earthquake also caused significant damage to many roads, bridges, and other buildings and triggered several geological hazards.Footnote1 To minimize casualties, post-disaster emergency relief work has become a top priority.
Rapid identification of the most-affected areas is critical for carrying out emergency relief efforts following a disaster (Ma and Xie Citation2000; Wei et al. Citation2017), and earthquakes are often ‘information black box periods’ in the early stages (Nie et al. Citation2012). Determining the most-affected areas is a difficult task in postearthquake emergency relief efforts. At present, seismic intensity is the primary basis for determining the most-affected areas (Liu Citation1994; Kropivnitskaya et al. Citation2017). Seismic intensity is calculated based on ground-motion attenuation models to estimate the extent and impact of an earthquake (Wang et al. Citation2000; Wang and Song Citation2001). The determination of seismic intensity is mostly based on geological parameters, which leads to inaccuracies with actual seismic damage and influences assessments of the most-affected areas. Seismic hazards and their impacts are complex, increasing the possibility of inaccurate seismic intensity assessments and failure to accurately determine actual impact situations, which affects determination of the most-affected areas and obstructing rapid emergency relief efforts following earthquakes (Li et al. Citation2008; Lu et al. Citation2018). Therefore, further exploration of methods for quickly and accurately identifying the most-affected areas is still a major research task for us.
In recent years, as the internet and spatial information technologies have developed, LBS (location-based service) systems have gradually developed. LBS is a service system that uses positioning technology to locate mobile terminals; it analyzes the positioned information through internet technology and then responds to the user through the mobile terminal (Huang et al. Citation2018). Mobile signaling is the communication data between mobile phone users and transmitting base stations or microstations. Mobile signaling data begins to be generated as soon as the phone is turned on. After desensitization, further mining can help determine the most-affected areas and provide emergency relief.
In view of above, taking the 2022 Mw 6.6 Luding earthquake in Sichuan, China as an example, this study used the minute-by-minute mobile signaling data before and after the earthquake to determine the scope of the most-affected area, to provide a reference for postearthquake emergency relief. The main objective of this study include: (1) to analyze the temporal changes of mobile signaling data before and after the earthquake; (2) to explore the response of mobile signaling changes to the earthquake and its relationship with seismic intensity; and (3) to carry out an interpolation analysis using kriging interpolation and attempt to build an earthquake intensity assessment model to make more rapid and refined judgments on the most-affected areas.
This paper consists of six parts, with the following structure: In Section 2, we review relevant research related to the addressed topics; in Section 3, we describe the study area, methods and data; the results are presented in Section 4; we discuss the results in Section 5; and we provide the conclusions of this study in Section 6.
2. Literature review
Determination of the most-affected areas of the earthquake is a prerequisite for emergency relief work. To further improve the accuracy of assessing the most-affected areas, many scholars have tried to study various methods of assessing seismic intensity, such as the intensity attenuation relationship (Casado Citation2000; Pasolini et al. Citation2008), field investigation (Xu et al. Citation2013; Huang and Gartner Citation2018), instrumental intensity (Wu Citation2003; Jin et al. Citation2013). However, traditional methods for identifying the most-affected areas are mainly based on the earthquake itself, which may lead to inconsistencies between the determined areas and actual casualties and losses. Given that post-earthquake emergency rescue primarily aims to save lives and minimize casualties, many scholars have developed new methods to improve the accuracy of identifying the most-affected areas. For instance, remote sensing images have been used to analyze earthquake damage and pinpoint the locations of the most-affected areas (Zhang et al. Citation2002; Corbane et al. Citation2011; Xu et al. Citation2014; Wang et al. Citation2015), while artificial intelligence technology has been leveraged to assist with pre-earthquake preparedness, warning, and post-earthquake emergency rescue (Harirchian et al. Citation2022; Kumari et al. Citation2022; Abdalzaher et al. Citation2023).
Although these studies have made significant progress and have helped quickly and accurately identify the most-affected areas for emergency rescue, the ‘golden 72 h’ after an earthquake require even faster and more precise methods for determining the most-affected areas. With the development of information network technology and the increasing popularity of mobile phones. The LBS system contains rich location information about users, which many scholars have used to assess the human mobility and building damage in disaster events (Lu et al. Citation2016; Ercan et al. Citation2018; Marzuoli and Liu Citation2018; Acosta et al. Citation2020) and provide disaster early warning information (Moumni et al. Citation2013; Bossu et al. Citation2015; Yabe et al. Citation2018; Finazzi et al. Citation2022). Currently, the research on LBS in determining the affected areas is mainly divided into two types: social media data and mobile signaling data. Social media data mainly utilizes the rich location information contained in social platforms such as Twitter and Weibo to study the characteristics of social response changes, in order to reflect the assessment of the impact of earthquakes (Arapostathis et al. 2018; Xing et al. Citation2019). Social media data enable faster access to real-time disaster information, speeding up determination of the affected areas after an earthquake. However, the social media data are targeted at a single audience group, are unevenly distributed, and contain less spatial information with lower accuracy, thus affecting accuracy when determining the most-affected areas (Bengtsson et al. Citation2011; Dai et al. Citation2022).
Compared to social media, mobile signaling data provides a more complete and continuous tracking of cell phone users’ activities through base stations, offering high timeliness, spatial continuity, sufficient data volume, and accuracy. After desensitization, this data can be used to aid in rapid assessment and emergency rescue efforts following an earthquake. When combined with traditional empirical assessment models, mobile signaling data can provide more timely and powerful technical support for post-disaster emergency response, thereby enhancing the efficiency of rescue efforts (Huang et al. Citation2018; Balistrocch et al. Citation2020; Yabe et al. Citation2022). Many scholars have utilized mobile signaling data to investigate population changes and disasters, including earthquakes, demonstrating that it can be used to determine the most-affected areas. Although mobile signaling data cannot precisely represent the actual population size, it can reflect the real spatial and temporal changes of the population due to the widespread use of mobile devices (Guo and Karimi Citation2017; Li et al. Citation2017; Chen et al. Citation2018; Citation2019; Wei et al. Citation2023). As of the end of 2021, the number of mobile phone users in China had reached 1.643 billion, with a penetration rate of 116.3 phones per hundred people, providing a vast amount of data to explore the use of mobile signaling data in determining the most-affected areas. Mobile signaling data may be classified into two types based on the location information determination method: One type relies on telecom operators’ base stations or satellites (GPS and Beidou, etc.) to identify mobile phone location information, which mostly consists of phone bill data and signaling data given by telecom carriers. In this type, Yabe et al. (Citation2022) used mobile signaling data to estimate post-earthquake evacuation hotspots and judged the worst-hit areas by evacuation rates. Xing et al. (Citation2021) proposed a method to combine mobile phone signaling data with social media data to determine the worst-hit areas after an earthquake. Dai et al. (Citation2022) used a spatiotemporal clustering method to determine the worst-hit areas after the earthquake based on mobile signaling data before and after the earthquake. Another type is the determination of mobile phone location data using a Software Development Kit (SDK). This signaling data is less affected by base station distribution and immediately locates the phone with high precision. Thus, Li et al. (Citation2017, Citation2022) have applied it to the study of post-earthquake population response. In addition, some scholars have attempted to use mobile phone signaling data to study the seismic impact range in recent years. Pang et al. (Citation2019) demonstrated the feasibility of using mobile signaling data to determine the earthquake impact areas Xia et al. (Citation2019) analyzed the changes in mobile phone signaling data for eight earthquakes and attempted to establish an intensity assessment model. Guo et al. (Citation2022) used mobile phone signaling data to interpolate the seismic impact field and attempted to develop an intensity assessment model. Based on mobile signaling data, Li et al. (Citation2022) analyzed responses of crowds in high-rise buildings to far-field non-destructive earthquakes to determine the degree of earthquake impact. However, the application of mobile phone signaling data in disasters, especially earthquakes, is still in the exploratory stage. Further mining of mobile signaling data and combining it with traditional methods will help with planning and conducting postdisaster emergency relief efforts. Therefore, we still need to use empirical research to perfect the theoretical models and technical methods related to mobile signaling data. In this study, we attempted to use mobile signaling data to determine the most-affected areas of the Luding earthquake in Sichuan, China in order to further revise the official intensity impact field for rapid emergency relief.
3. Methods and data
3.1. Study area and 2022 Luding earthquake
The study area is the area that was affected by the Mw 6.6 Luding earthquake in Sichuan, China (). The Mw 6.6 Luding earthquake occurred at 12:52 on September 5, 2022. The epicenter (29.59°N, 102.08°E) was in the town of Moxi, Luding County, Sichuan Province, China, at a depth of 16 km, and the seismogenic fault was the Xianshui River Rift on the southeastern edge of the Qinghai-Tibet Plateau. The highest intensity of this earthquake reached IX degrees, and the study area was mainly above the VI intensity zones (). The topography of the study area has a large altitude gap (up to 7,556 m above sea level, with over 5,000 m above sea level from the lowest point), with a strong site amplification effect and serious slope instability problems. This earthquake caused a serious break due to the topographic conditions.
3.2. Data
The data in this study are minute-by-minute mobile signaling data from the GeoHash grid obtained by a third party. The GeoHash grid is a two-dimensional spatial latitude and longitude data grid encoded into a string using an address encoding method. The longer the string is, the more accurate the representation is. In this study, GeoHash encoding has 6-bit precision, indicating a rectangular area of 1.2 * 0.6 km. The mobile signaling data is a count of the number of mobile devices in each GeoHash square in the region range every minute, obtained using several location techniques for the service. If the number of devices in a GeoHash unit varies rapidly within a time slice, large-scale anomalies in the range and period may indicate that an earthquake or other abrupt calamity has happened in the region. And the data have been desensitized and do not affect personal privacy, as required by law.
Based on our preliminary research and the actual data we have collected, we have established that reviewing two hours of data before and after the earthquake is adequate to quantify the damage in the most severely impacted locations (Pang et al. Citation2019; Guo et al. Citation2022). Therefore, we selected mobile signaling data in 6-bit precision GeoHash grids acquired for 239 min before and after the earthquake occurred (10:53–14:51 on September 5, 2022), with a concentration on the Luding seismic intensity VI zone and above (). The numbers of GeoHash grids within intensity zones VI, VII, VIII, and IX were 2384, 493, 87, and 72, respectively. The area of each intensity zone revealed that the number of grids increased in proportion to their size. The total numbers of devices collected within intensity zones VI, VII, VIII, and IX during the study period were 149505, 49,939, 1501, and 1861, respectively. The number of devices gathered increases with the number of grids in the low-intensity region. However, in the high-intensity area, which was the most impacted, the number of devices collected decreases with the number of grids due to many factors.
To provide more precise assistance in postearthquake emergency response, this study defines the VIII and IX intensity zones as the most-affected areas, i.e. areas affected significantly by the earthquake with serious seismic damage. And the intensity levels are classified according to The Chinese seismic intensity scale (2020).Footnote2
3.3. Methods
3.3.1. Analysis of mobile signaling changes
Mobile signaling data for 119 min before the earthquake, i.e. 10:53–12:51 (later referred to as ‘preearthquake’), 119 min after the earthquake, i.e. 12:53–14:51 (later referred to as ‘postearthquake’), and the moment of the earthquake, i.e. 12:52, for a total of 239 min, were used to analyze the changes in mobile signaling data before and after the earthquake.
This study has established a mobile signaling change rate indicator C to quantify the change in mobile signaling data. The change rate could reflect the increase, decrease, and magnitude of change in the number of devices before and after the earthquake, thus reflecting the intensity and seismic damage. The change rate C in this study is the ratio of the number N of devices collected at t minutes after the earthquake relative to the number of devices collected at t minutes before the earthquake in the GeoHash square g. The change rate C can be calculated with the following formula:
(1)
(1)
where C gt is the change rate of mobile signaling at t minutes in GeoHash square g, Ngt is the number of devices at t minutes after the earthquake in GeoHash square g, and N’gt is the number of devices at t minutes before the earthquake in GeoHash square g.
If the change rate C is positive, the number of devices after the earthquake is smaller than that before the earthquake. The greater the change rate is, which tends to be one, the greater the decrease in the number of devices will be postearthquake compared to preearthquake, indicating a more severe earthquake. If the change rate C is negative, the number of devices increased after the earthquake compared to before. The more the change rate tendency is toward −1, the larger the change in the number of devices will be after the earthquake, suggesting a more severe earthquake. If the change rate C tends toward zero, the number of devices postearthquake is equivalent to the number preearthquake, and the seismic influence is not significant or diminished.
Additionally, to investigate the trend of the change rate of mobile signaling further, we calculated a sliding average of the change rate C, i.e. Q gt (the T-minute sliding average of the mobile signaling change rate at minute t in GeoHash square g). The specific equation is as follows:
(2)
(2)
To examine the trend of mobile signaling, we compared the trends after 2-, 3-, 5-, 10-, 15-, and 30-minute sliding averages. We finally decided to apply the best working 5-minute sliding average method in this study.
3.3.2. Analysis of the relationship between mobile signaling data and intensity
As the earthquake has a variable impact in different intensity areas, an analysis of the change in the number of devices at various intensities can help determine the most-affected areas. Pearson correlation coefficients can measure the degree of correlation between two variables (Rodgers and Nicewander Citation1988; Fan et al. Citation2014), so we used Pearson correlation analysis to explore the correlation between mobile signaling and intensity. In addition, multiple regression analysis is a statistical method of building a regression model between several independent variables and the dependent variable (Fu et al. Citation2014; Yang and Song Citation2022). We attempt to use regression analysis to establish an intensity assessment model based on the change rate of mobile signaling and the epicenter distance, therefore providing a reference to quickly determine the most-affected locations following the earthquake.
This study used SPSS software (IBM, Armonk, NY, USA) for correlation analysis and regression analysis. In addition, because the topography in the study area is mountainous and the population density distribution is uneven, the number of devices collected in some remote regions by mobile signaling is prone to abnormal values. To further improve the accuracy of the interpolation results, we remove Geohash grids with invalid change rate values and anomalies within the VI and VII intensity areas (meeting the two conditions of the change rate is 1 and the 5-minute average of the number of devices before the earthquake is less than 1). Finally, in intensity zones IX, VIII, VII, and VI, the GeoHash grids are 20, 19, 92, and 392, respectively.
3.3.3. Spatial interpolation analysis
Spatial interpolation analysis is a geostatistical method that uses information from sampled points to forecast information about unknown points or regions. Spatial interpolation analysis includes several methods. Kriging interpolation uses the distance and spatial relationship between the unknown point and a range of sampled points to fit a model to determine the weights and provides a linear unbiased, optimal estimate of the attributes of the unknown region. Kriging is essentially a method of estimation by local weighted averaging:
(3)
(3)
where
is the estimate of point Z(
is the measured value at point Z(
), n is the total number of sample points and
is the weights. The kriging interpolation method can represent the spatial variation in the attributes and is more accurate and realistic (Oliver and Webster Citation1990; Li et al. Citation2021).
Therefore, this study uses the Kriging interpolation approach to perform a spatial interpolation analysis based on the change rate C after removing anomalies. Simultaneously, we conduct an overlay study with the published intensity maps to further validate the interpolation results. And the shows the flowchart of this study.
4. Results
4.1. Analysis of changes in the number of devices collected by mobile signaling
4.1.1. Analysis of overall change’s
shows the minute-by-minute variation in the number of devices collected by mobile signaling for 239 min (10:53–14:51) before and after the Luding earthquake occurred. According to the changes, we can divide it into three phases. The first phase is from 10:53–12:51, which did not exhibit any effects from the earthquake, and the trend of the 5-minute sliding average showed that the change in this phase was relatively smooth. The second phase, from 12:52 to 12:57, was a mutation phase. The earthquake occurred at 12:52, and the number of devices increased dramatically in the first minute after the earthquake. The number of devices reached 1173 at 12:53, the highest value throughout the research period. Then, a sudden decrease occurred, dropping to 798 at 13:00, i.e. 8 min after the earthquake. In the first minute immediately following the earthquake, with the base stations still working in the least-hardest hit areas, the number of people checking their mobile phones simultaneously increased significantly, so the overall number of devices showed a sudden increase. As time passed, the number of people checking their mobile phones concurrently decreased rapidly, resulting in a sharp reduction from 12:53 to 13:00. The third phase, from 12:57 to 14:47, showed minor up-and-down fluctuations. The 5-minute sliding average change trended downward and was lower than the preearthquake level. Due to the earthquake’s significant impacts in intensity areas VIII and IX, where several base stations were out of service and were not repaired within 2 h following the earthquake, fewer devices were collected after the earthquake occurred than before.
Figure 3. Min-by-minute changes in the number of devices. On the horizontal axis, 1 represents 1 min after 12:52, i.e. 12:53; 2 represents 2 min after 12:52, i.e. 12:54, ……, and 119 represents14:51. -1 represents 1 min before12:52, i.e. 12:51; -2 represents 2 min after 12:52, i.e. 12:50, ……, and -119 represents10:53.

4.1.2. Analysis of changes in different intensity zones
shows the temporal variation in the number of devices collected by mobile signaling in the different intensity areas. The number of devices collected by mobile signaling varied by intensity, with different levels of seismic impact. The number of devices in intensity IX decreased abruptly 1 min after the earthquake and then tended to 0. At intensity VIII, there was also a sudden decrease 1 min after the earthquake occurred, but the magnitude of the decrease was less than that at intensity IX. At intensity VII, there was a slight increase 1 min after the earthquake and then a slight reduction 6 min after the earthquake, with both the increase and decrease being small. At Intensity VI, the number of devices increased abruptly, peaked 1 min after the earthquake, and then gradually dropped to the preearthquake level due to the earthquake’s minor effect. The GeoHash grids with data reported were 2384, 493, 87, and 72 in intensity areas VI, VII, VIII, and IX, respectively. Influenced by the size of the sampling area, the number of devices sampled in intensity zones VI and VII was much greater.
Figure 4. Time variation in change rates in different intensity areas. On the horizontal axis, 1 represents 1 min after 12:52, i.e. 12:53; 2 represents 2 min after 12:52, i.e. 12:54, ……, and 119 represents 14:51. -1 represents 1 min before12:52 i.e. 12:51; -2 represents 2 min after 12:52, i.e. 12:50, ……, and -119 represents10:53.

4.2. Analysis of time variation in the change rate of mobile signaling
4.2.1. Time variation analysis of the overall change rate
shows the temporal variation in the mobile signaling change rate. There were two stages, according to the 5-minute sliding average variation trend. The first phase, from the 1st minute to the 8th minute, was a mutation phase. The change rate dropped to −0.424 in the first minute and then climbed to 0.157 in the eighth minute. The change rate for the first 5 min was less than zero, while the change rate for the next 6–8 min was above zero. Consistent with the temporal change in the number of devices in , there was a sharp increase followed by a sharp decline in the first 5 min after the earthquake. Due to the impact of the earthquake disaster, some base stations were damaged or even withdrawn from service. Therefore, the overall number of devices after the earthquake was lower than before, and the change rate gradually increased from below zero to above zero in the first stage. The second phase, which took place from the 8th minute to the 119th minute, revealed modest fluctuations in the increasing magnitude, with the change rate fluctuating above and below zero. According to the 5-minute sliding average trend line, the change rate in this phase was often more than zero, indicating that the number of devices mainly decreased after the earthquake compared to the preearthquake period.
4.2.2. Temporal variation analysis of the change rate in different intensity zones
shows the temporal changes in the change rate of mobile signaling in different intensity zones. The trends in the four intensity areas were similar to the overall changes, but the trends in the four intensity areas differed. The change rates in intensity regions VIII and IX were all above zero, with 5-minute sliding averages ranging between 0.6 and 1. The number of devices was significantly reduced after the earthquake occurred compared to before the earthquake occurred because of the severe seismic effect in intensity zones VIII and IX, where base stations were severely damaged. In intensity areas VI and VII, the change rate in the early postearthquake period is less than 0, i.e. the number of devices collected by mobile signaling increased relative to the preearthquake period. As these two zones were less affected by the earthquake, mobile devices, such as mobile phones, would increase significantly, and the number of devices compared to the preearthquake period would increase, so the change rate was negative in the initial postearthquake period. From the 5-minute sliding average trend line, the average change rate in intensity zone VII rose to a positive value in the 5–9-minute period and then fluctuated upward in the range of 0–0.6, while in intensity zone VI, the change rate fluctuated near approximately 0. As a result, mobile signaling change rates varied across intensity zones. In the high-intensity zones, the change rate was above zero and fluctuated toward 1, while in the low-intensity zones, the change rate was below zero immediately following the earthquake. The lower the intensity was, the less noticeable the increasing tendency after the earthquake was, and the closer it remained to 0.
4.3. Analysis of the relationship between mobile signaling data and intensity
To some extent, using mobile signaling data can aid in determining the most-affect areas after an earthquake occurs based on the above research. The level of intensity reflects the degree of disaster damage. We analyzed the relationship between the intensity and the average value of the change rate in the first 5 min after the earthquake occurred to further explore determination of the most-affected areas by mobile signaling.
4.3.1. Variation in the mobile signaling change rate in different intensity areas
shows the average value of the change rate in different intensity zones, which can be divided into two types. One type is high-intensity areas, i.e. intensity zones VIII and IX, where the change rate is close to 1. In high-intensity zones, which were more affected by earthquakes, the damage was more serious, so the number of devices collected by mobile signaling decreased or could not be collected following the earthquake due to the destruction of base stations. The other type is the low-intensity areas, i.e. the VI and VII intensity zones. The earthquake had a minor impact on low-intensity zones. After the earthquake, the number of people accessing mobile phones increased during the same period, as did the number of mobile signaling collection devices, so the change rate in this type was less than 0. Thus, there was a relationship between the change rate of mobile signaling and the intensity magnitude.
4.3.2. Correlation analysis and regression analysis
Within the GeoHash square g, we performed correlation analyses of the average change rate in the first 5 min Cg5 and the epicentral distance Dg (distance from the GeoHash grid to the epicenter) with intensity Ig. At the 0.01 level of confidence, Ig is significant with Cg5 and Dg. The correlation between intensity and change rate was significantly positive, with a correlation coefficient of 0.225. The greater the change rate is, the higher the intensity is, i.e. the more severe the damage caused by the earthquake. The intensity and epicentral distance were significantly negatively correlated.
After removing the anomalies, we selected three-quarters of the data (392) as test data to build the intensity assessment model presents the results of the regression analysis. Model 1 was built based on the only variable that is the change rate C of mobile signaling. It can be seen from that the model 1 is significant at the confidence level of 0.01, indicating that its fitting result is effective, although the adjusted variance explanation (adj-R2) is only 4.8%. To further improve the fit effect of model 1 and enhance its applicability, we added a variable, epicentral distance D, which represents the distance from each signaling point to the epicenter, to build the intensity assessment model 2. We can find that the model 2 is also statistically significant at the confidence level of 0.01. The adjusted variance explanation (adj-R2) of model 2 reaches to 62.0%, showing that the variables mobile signaling and epicenter distance can more well fit the change of seismic intensity ().
Table 1. Results of regression analyses.
In addition, we selected another quarter of the data (131) as validation data to test the accuracy of the models. As can be seen in , the accuracy of model 1 is 63.36%; the accuracy of the model 2 can reach 77.86%. As a result, model 2 built based on mobile signaling change rate and epicentral distance performed better both in terms of fitting effect and validation accuracy. The model 2 can assist us in more accurately determining the most-affected areas in an earthquake.
4.4. Interpolation analysis using mobile signaling data
By comparing and analyzing the change rates within 10 min, we finally selected the first 5-minute average of the change rates as the interpolation sample points. We carried out kriging interpolation analysis using sample points, as shown in . The interpolation results in show clear divisions and a distinct range of extreme seismic zones. The interpolation results revealed two extreme seismic zones with change rates above 0.6. The two extremely severely damaged seismic zones were in the towns of Moxi and Detuo. As a result of the Luding earthquake, there were 97 deaths and 20 missing persons. The distribution of deaths was close to two extreme seismic zones. And the major secondary disasters after the disaster also occur in the two extremely severely damaged seismic zones. This finding further demonstrated that our interpolation results could accurately reflect the seismic damage situation. We can further refine determination of the most-affected areas in the interpolation results based on the information obtained from field surveys, such as the distribution of deaths and geological hazards.
Furthermore, by comparing the officially published intensity maps, our interpolation results matched the intensity maps. In general, the change rate was positive with intensity, and the change rate was above 0.4 in the high-intensity areas, i.e. intensity zones VIII and IX. The direction of change in our interpolation results was also comparable to the intensity map, running from northwest to southeast. In addition, the interpolation results provided a more detailed classification of the damage, especially for the VII intensity zone, which could be divided into two categories: a more severely damaged area in yellow and a less severely damaged area in blue. In short, mobile signaling data can provide a more solid indication of the extent of damage in the most-affected areas as well as a reference for rapid relief following an earthquake occurrence.
5. Discussion
5.1. Response of mobile signaling to earthquake effects
People’s work and relaxation routines influence the number of devices collected by mobile signaling (Chen et al. Citation2018; Wei et al. Citation2023). When there is no emergency event, it fluctuates within a particular range, while the number of devices changes abnormally after an emergency event, such as an earthquake. There was a sudden increase one minute after the Luding earthquake, followed by a sharp decrease. Immediately following the earthquake, people would check their phones or call friends and family, resulting in a sudden increase in the number of devices collected by mobile signaling. For the change rate, the 1-minute change rate was the lowest and negative (−0.184), indicating a substantial increase compared to the preearthquake period. The number of simultaneous accesses then decreased, and the number of devices collected also declined. The sudden reduction process lasted for 7 min. The change rate between the second and fifth minutes was negative, suggesting that the number of devices after the earthquake increased compared to the preearthquake period. The change rate turned positive between the sixth and eighth minutes, suggesting that the number of devices collected during the 6–8 min following the earthquake, although still declining, was already less than that preearthquake. After the mutation ended, the change rate fluctuated near approximately 0, and it was above 0 most of the time. The overall number of devices collected was less than the preearthquake levels due to the severe seismic effects in the VIII and IX intensity areas, where base stations were damaged and decommissioned. Therefore, the changes in mobile signaling data can be an indicator of emergencies, such as earthquakes. These findings are consistent with those of similar studies (Guo and Karimi Citation2017; Pang et al. Citation2019; Xia et al. Citation2019)
5.2. Response of mobile signaling to the degree of seismic damage
Because the intensity generally reflects the degree of seismic damage, we analyzed the change in the number of devices in various intensity areas. And the number of devices varied across different intensity zones. In the high-intensity zones, most of the base stations were severely damaged or even withdrawn from service and could not be repaired within 119 min following the earthquake, so the number of devices collected after the earthquake tended toward zero, with a significant decline compared to the prior period. In the low-intensity zones, the number of devices first increased and subsequently decreased following the earthquake. However, the magnitude of change differed in the two intensity zones. At intensity VII, the postearthquake increase was slight, with a downward trend in the postearthquake period. Similar to the overall change in intensity VI, there was a significant sudden increase at 1 min postearthquake, followed by a sudden decrease and a slight increase at 62 min postearthquake. For the change rate, the low-intensity zone had a negative value after the earthquake and fluctuated near approximately 0. The overall tendency of the VII intensity zone was above 0. Because intensity zone VII was the high- and low-intensity division and part of it was still more affected by the earthquake, there was no significant sudden change in the initial postearthquake period. In contrast, the intensity VI was farther away from the epicenter and was less affected by the earthquake, so the number of people accessing mobile phones increased greatly in the initial period after the earthquake, resulting in a considerable rise in the number of devices. In addition, due to slight earthquake damage, the affected base stations would be repaired within one hour following the earthquake, leading to a rebound in the number of devices collected in the Intensity VI zone one hour after the earthquake. In short, the change in mobile signaling data can reflect the degree of damage.
5.3. Determination of the degree of damage by mobile signaling data
We conducted an interpolation analysis based on the change rate of mobile signaling using kriging interpolation. According to the results, the change rate of mobile signaling could determine the degree of seismic damage and delineate the most-affected areas. The official intensity map is the primary way to determine the extent of earthquake damage. Our interpolation results were consistent with the published intensity map, with higher change rates in the high-intensity zones and lower change rates in the low-intensity zones. Both directions of change were northwest–southeast. Unlike the published intensity map, which used a more regular ellipse to divide the four intensity zones, our interpolation results showed a more precise classification into ten classes, with two extreme zones where the change rate was more than 0.6. Because the VII intensity zone was in the high- and low-intensity division zone, our interpolation results revealed that the VII intensity zone was divided into two sections, the high change rate in yellow and the low change rate in blue.
Furthermore, combining field surveys on the distribution of fatalities and geological hazards, we can more accurately classify the most-affected areas. The death toll in the Luding earthquake was 93, but 76 people died in geological disasters. Geological disasters are also an important cause of casualties in this earthquake (Deng et al. Citation2023; Huang et al. Citation2023). The rapid judgment of post-earthquake secondary disasters plays an important role in post-earthquake emergency rescue work and has important reference value in the study of determining most-affected areas. In summary, employing mobile signaling data can help assess the severity of seismic damage, more precisely pinpoint the most afflicted regions, and provide quick relief after an earthquake.
In addition, there was a significant correlation between intensity and the mobile signaling change rate. Many scholars have attempted to develop intensity assessment models to achieve a more rapid assessment of most-affected areas (Xia et al. Citation2019; Guo et al. Citation2022). However, this is still in the exploratory stage, and a large number of studies are needed for empirical research. Thus, we attempted to develop an intensity assessment model using the change rate of mobile signaling and the epicentral distance. The model can evaluate the intensity zones and provide a quick rating of the degree of seismic damage, which can aid in postearthquake damage assessments.
5.4. Advantages of mobile signaling data in determining most-affected areas
Mobile signaling data is more advantageous for determining the extent of the seismic most-affected areas. Firstly, the ‘golden 72 h’ best rescue window after a disaster has established strict requirements for determining the extent of the most-affected areas. As a product of the Internet era, mobile signaling data has the advantages of quick access and large data volumes. This study reveals that an evaluation of the seismic impact is possible when using mobile signaling data collected five minutes after an earthquake. It vastly accelerates the process of determining the most-affected area. Secondly, compared to traditional methods of determining the impact range of earthquakes, which put more emphasis on the research of earthquake formation conditions such fracture zones, mobile signaling data can somewhat reflect population distribution, directly examining the impact of earthquakes on hazard-affected bodies, therefore offering a more specific assessment range. The interpolation findings, for instance, specifically determined two severe seismic zones. And they provided a more detailed evaluation of the impact scenario in the affected areas. Lastly, the judgment on the distribution of casualties is more consistent with the actual situation since the mobile signaling data directly represents the hazard-affected bodies. According to this study, most deaths from the Luding earthquake were located in the yellow or red area of the interpolation results, i.e. the most-affected area.
The post-earthquake emergency rescue’s primary objective is to save as many lives as possible while minimizing deaths. Mobile signaling data includes a wealth of demographic information. As a result, mobile signaling data can not only respond to the earthquake’s effect, but also provide assessment results that are faster, more accurate, and more responsive to casualty distribution, which may play a critical role in determining the most-affected areas and providing post-earthquake casualty relief. Mobile signaling can be used to assist the officially published intensity map. For instance, two hours after the Luding earthquake, the intensity map had some deviation from the actual situation (Xu et al. Citation2022). And there is a large error between our findings and the intensity map generated after 2 h. Therefore, using mobile signaling can enable timely correction of the main areas of emergency relief.
5.5. Limitations and challenges
In this study, inaccuracies were prone to occur in areas with few base stations because the distribution of GeoHash stations was consistent with the base stations. The distribution of the most-affected areas in the interpolation results corresponded to the base stations. Furthermore, environmental factors, such as the terrain of the study area, influenced the collection of mobile signaling. The geological background of the study area is complex, with a large elevation gap and mountainous terrain. Due to topographic factors, many devices were difficult to capture during the acquisition process, particularly in the intensity VIII area, where the terrain was mainly mountainous, and the settlements were scattered. As a result, it was prone to anomalies in the change rate calculation, and we eliminated the anomalies to increase the precision of determining the most-affected areas.
Using mobile signaling to build an intensity assessment model enables a more rapid assessment of damage; however, building and validating the model were all about the Luding earthquake data. More regional research data still need to be applied. Furthermore, the attempts should be made to incorporate more other independent variables, such as magnitude and time, into the seismic intensity assessment model, to establish a more applicable general model for seismic intensity assessment in the future.
Mobile signaling data can rapidly and accurately identify the areas most affected by earthquakes, providing valuable assistance to the officially published intensity maps. As data precision improves, it will be possible to study more localized areas and refine the determination of the most affected regions, facilitating quicker emergency rescue efforts. However, while mobile signaling technology continues to advance, it must also address challenges related to privacy leakage. Therefore, it is crucial to establish a reasonable mechanism for utilizing mobile signaling data in future research (Yin et al. Citation2015; Wei et al. Citation2023). The appropriate use of mobile signaling data under such a mechanism will significantly benefit our society.
6. Conclusion
This study explored the temporal variations in the overall and various intensity areas using mobile signaling data for 239 min before and after the Luding earthquake. According to the results, mobile signaling data changed rapidly following the earthquake, and the number of postearthquake devices was lower than the number of preearthquake devices. The changes in mobile signaling data varied by intensity area, with a sudden decrease and a tendency toward zero in high-intensity areas after the earthquake. There was a sudden increase in low-intensity zones, followed by a downward trend. The number of devices rebounded one hour following the earthquake in intensity VI zones. Based on the change rate of mobile signaling, we carried out an interpolation analysis, which was consistent with the published intensity map. The results provided a more specific representation of the distribution of most-affected areas and a more detailed classification of earthquake damage. In addition, we used the change rate of mobile signaling with the epicenter distance to build a model for intensity estimation, and we verified the accuracy up to 77.86%. Therefore, mobile signaling can determine earthquake damage, assist in determining the most-affected areas and result in more rapid rescue following an earthquake.
As a product of the internet era, mobile signaling can acquire a large amount of data in a brief time, improving the efficiency of seismic damage assessment and aiding in more rapid rescue. Of course, mobile signaling is still not a substitute for traditional seismic damage assessment. However, as a product of the big data era, it is a novel idea and method for seismic damage assessment following desensitization, which can aid in emergency rescue work following earthquakes and other emergencies.
Author contributions
Conceptualization, B.W. and X.G.; methodology, X.G.; formal analysis, X.G. and W.Q.; investigation, X.G. and T.Z.; resources, B.W..; data curation, B.W.; writing—original draft preparation, X.G.; writing—review and editing, B.W. and G.S; supervision, B.W. and G.S.; project administration, B.W. All authors have read and agreed to the published version of the manuscript.
Data availability statement
The mobile signaling data and seismic fatality distribution data can be obtained by contacting the corresponding author under reasonable grounds.
Disclosure statement
No potential conflict of interest was reported by the authors.
Additional information
Funding
Notes
1 Ministry of Emergency Management of the People's Republic of China [EB/OL]. Available online: https://www.mem.gov.cn/xw/yjglbgzdt/202209/t20220911_422190.shtml (accessed on 16 November 2022).
References
- Abdalzaher MS, Elsayed HA, Fouda MM, Salim MM. 2023. Employing machine learning and IoT for earthquake early warning system in smart cities. Energies. 16(1):495. doi:10.3390/en16010495.
- Acosta RJ, Kishore N, Irizarry RA, Buckee CO. 2020. Quantifying the dynamics of migration after hurricane Maria in Puerto Rico. Proc Natl Acad Sci U S A. 117(51):32772–32778. doi:10.1073/pnas.2001671117.
- Arapostathis S, Lekkas E, Kalabokidis K, Xanthopoulos G, Drakatos G, Spirou N, Kalogeras I. 2018. Developing seismic intensity maps from twitter data, the case study of Lesvos Greece 2017 earthquake: assessments, improvements and enrichments on the methodology. ISPRS - International Archives of the Photogrammetry. Int Arch Photogramm Remote Sens Spatial Inf Sci. XLII-3/W4/(W4):59–66. doi:10.5194/isprs-archives-XLII-3-W4-59-2018.
- Balistrocch M, Metulini R, Carpita M, Ranzi R. 2020. Dynamic maps of human exposure to floods based on mobile phone data. Nat Hazards Earth Syst Sci. 20(12):3485–3500. doi:10.5194/nhess-20-3485-2020.
- Bengtsson L, Lu X, Thorson A, Garfield R, von Schreeb J. 2011. Improved response to disasters and outbreaks by tracking population movements with mobile phone network data: a post-earthquake geospatial study in Haiti. PLoS Med. 8(8):e1001083. doi:10.1371/journal.pmed.1001083.
- Bossu R, Laurin M, Mazet-Roux G, Roussel F, Steed R. 2015. The importance of smartphones as public earthquake-information tools and tools for the rapid engagement with eyewitnesses: a case study of the 2015 Nepal earthquake sequence. Seismol Res Lett. 86(6):1587–1592. doi:10.1785/0220150147.
- Casado CL. 2000. Attenuation of intensity with epicentral distance in the Iberian Peninsula. Bull Seismol Soc Am. 90(1):34–47. doi:10.1785/0119980116.
- Chen LN, Wu S, Chen J. 2018. The near-real-time prediction of urban populations based on mobile phone location data (in Chinese). J Geo-Inform Sci. 20(4):523–531.
- Chen W, Zhai GF, Zhang Y. 2019. High-precision spatial and temporal distribution of population based on mobile phone data: A case of Xiaobailou CBD area in Tianjin city (in Chinese). Resource Develop Market. 35(10):1266–1272.
- Corbane C, Saito K, Dell’Oro L, Gill S, Piard B, Huyck C, Kemper T, Lemoine G, Spenc R, Shankar R, Senegas O, Ghesquiere F, Lallemant D. 2011. A comprehensive analysis of building damage in the 12 January 2010 Mw7 Haiti earthquake using high-resolution satellite and aerial imagery. Photogramm Eng Remote Sensing. 77(10):997–1009. doi:10.14358/PERS.77.10.0997.
- Dai KX, Cheng CX, Shen S, Su K, Zheng XM, Zhang T. 2022. Postearthquake situational awareness based on mobile phone signaling data: an example from the 2017 Jiuzhaigou earthquake. Int J Disaster Risk Reduct. 69:102736. doi:10.1016/j.ijdrr.2021.102736.
- Deng JH, Wei X, Dai SG, Deng H. 2023. Characterization of triggered disasters in the Luding Earthquake[J/OL]. Advance Engng Sci. 1–11. doi:10.15961/j.jsuese.202201236.
- Ercan I, Mehmet I, Mehmet B. 2018. Web based evaluation of earthquake damages for reinforced concrete buildings. Earthquake Struct. 13:423–432. doi:10.12989/eas.2017.13.4.423.
- Fan R, Meng DZ, Xu DS. 2014. Survey of research procession statistical correlation analysis [J]. Math Modeling Appl. 3:1–12.
- Finazzi F, Bondár I, Bossu R, Steed R. 2022. A probabilistic framework for modeling the detection capability of smartphone networks in earthquake early warning. Seismol Res Lett. 93(6):3291–3307. doi:10.1785/0220220213.
- Fu YY, Yang GJ, Wang JH, Song XY, Feng HK. 2014. Winter wheat biomass estimation based on spectral indices, band depth analysis and partial least squares regression using hyperspectral measurements. Comput Electron Agr. 100:51–59. doi:10.1016/j.compag.10.010.
- Guo QL, Karimi HA. 2017. A novel methodology for prediction of spatial-temporal activities using latent features[J]. Comput Environ Urban Sys. 62:74–85. doi:10.1016/j.compenvurbsys.2016.10.006.
- Guo XX, Wei BY, Nie GZ, Su GW. 2022. Application of mobile signaling data in determining the seismic influence field: a case study of the 2017 Mw 6.5 Jiuzhaigou earthquake, China. IJERPH. 19(17):10697. doi:10.3390/ijerph191710697.
- Harirchian E, Jadhav K, Kumari V, Lahmer T. 2022. ML-EHSAPP: a prototype for machine learning-based earthquake hazard safety assessment of structures by using a smartphone app. Eur J Environ Civil Engin. 26(11):5279–5299. doi:10.1080/19648189.2021.1892829.
- Huang HS, Gartner G. 2018. Current trends and challenges in location-based services[J]. IJGI. 7(6):199. doi:10.3390/ijgi7060199.
- Huang Y, Xie C, Li T, Xu C, He X, Shao X, Xu X, Zhan T, Chen Z. 2023. An open accessed inventory of landslides triggered by the Ms 6.8 Luding earthquake, China on September 5, 2022. Earthquake Res Adv. 3(1):100181. doi:10.1016/j.eqrea.2022.100181.
- Huang Z, Ling X, Wang P, Zhang F, Mao Y, Lin T, Wang FY. 2018. Modeling real-time human, mobility based on mobile phone and transportation data fusion. Transport Res C Emerg Technol. 96:251–269. doi:10.1016/j.trc.2018.09.016.
- Jin X, Zhang HC, Li J. 2013. Preliminary study on seismic instrument intensity criteria. Prog Geophys. 28:2336–2351.
- Kropivnitskaya Y, Tiampo KF, Qin J, Bauer MA. 2017. Real-time earthquake intensity estimation using streaming data analysis of social and physical sensors. Pure Appl Geophys. 174(6):2331–2349. doi:10.1007/s00024-016-1417-6.
- Kumari V, Harirchian E, Lahmer T, Rasulzade S. 2022. Evaluation of machine learning and web-based process for damage score estimation of existing buildings. Buildings. 12(5):578. doi:10.3390/buildings12050578.
- Li DP, Huang LH, Chen P. 2017. Crowd flow analysis based on cell phone location data for the 7.0 magnitude earthquake in Jiuzhaigou, Sichuan[J]. Earthquake Res China. 33:602–612.
- Li DP, Tan QQ, Yin JF, Jian YB. 2022. Analysis on the impact of Taiwan far-field earthquakes on the disaster avoidance behavior of people in high-rise buildings in large cities in southeast China. Geomat Nat Haz Risk. 13(1):2006–2023. doi:10.1080/19475705.2022.2101945.
- Li Q, Wang W, Dan G, Zhang JF, Wang JF. 2021. Approach to evaluating seismic intensity by integrating InSAR Co-seismic deformation and far field observation data. Nat Hazards. 1(3):116–125. doi:10.1016/j.nhres.2021.06.004.
- Li ZQ, Yuan YF, Li XL, He P. 2008. Some insights into the macro-epicenter and meizoseismal region of Wen Chuan eartehquake [J]. Seismol Geol. 3:768–777.
- Liu HX. 1994. Outline of engineering standards for SI. Selected papers on Liu Huixian’s seismic engineering. Institute of Engineering Mechanics, State Seismological Administration. Seismic Publishing Press; p. 59–80.
- Lu X, Wrathall DJ, Sundsøy PR, Nadiruzzaman M, Wetter E, Iqbal A, Qureshi T, Tatem A, Canright G, Engø-Monsen K, et al. 2016. Unveiling hidden migration and mobility patterns in climate stressed regions: a longitudinal study of six million anonymous mobile phone users in Bangladesh. Global Environ Change. 38:1–7. doi:10.1016/j.gloenvcha.2016.02.002.
- Lu YX, Wang L, Wei L, Liu K, Che GF, Li SH. 2018. Study on relative variability of ground-motion in Min-Zhang region by using representative site parameters [J]. J Disaster Prevent Mitig Engng. 38(2):359–366. doi:10.13409/j.cnki.jdpme.
- Ma YH, Xie LL. 2000. A discussion on the factors of human casualties in earthquakes[J]. J Natural Disaster. 3:84–90.
- Marzuoli A, Liu F. 2018. A data-driven impact evaluation of hurricane Harvey from mobile phone data. In 2018 IEEE International Conference on Big Data (Big Data). 3442–3451. doi:10.1109/BigData.2018.8622641.
- Moumni B, Frías-Martínez V, Frias-Martinez E. 2013. Characterizing social response to urban earthquakes using cell-phone network data: the 2012 Oaxaca earthquake. UbiComp 2013 adjunct - adjunct publication of the 2013 ACM conference on ubiquitous computing. 1199–1208. doi:10.1145/2494091.2497350.
- Nie GZ, An JW, Deng Y. 2012. Advances in earthquake emergency disaster services[J]. Seismol Geol. 34:782–791.
- Oliver MA, Webster R. 1990. Kriging: a method of interpolation for geographical information systems. Int J Geogr Inf Sci. 4(3):313–332. doi:10.1080/02693799008941549.
- Pang XK, Nie GZ, Zhang X. 2019. Selection of earthquake disaster indicators based on cell phone location data[J]. Earthquake Res China. 35:144–157.
- Pasolini C, Albarello D, Gasperini P, D'Amico V, Lolli B. 2008. The attenuation of seismic intensity in Italy, Part II: modeling and validation. Bull Seismol Soc Am. 98(2):692–708. doi:10.1785/0120070021.
- Rodgers JL, Nicewander WA. 1988. Thirteen ways to look at the correlation coefficient. Am Stat. 42(1):59–66. doi:10.2307/2685263.
- Wang JL, Song ZF. 2001. Model for fast seismic disaster assessment [J]. J Seismol Res. 2:162–167.
- Wang SY, Yu YX, Gao AJ, Yan XJ. 2000. Development attenuation relations for ground motion in China[J]. Earthquake Res China. 2:5–12.
- Wang XQ, Dou AX, Wang L. 2015. RS-based assessment of seismic intensity of the 2013 Lushan, Sichuan, China MS7.0 earthquake. Geophys. 58:163–171.
- Wei BY, Nie GZ, Su GW, Qi WH. 2017. Advances on the assessment methods of buried personnel distribution in earthquake disaster[J]. J Catastrophol. 32:155–159.
- Wei BY, Su GW, Liu FG. 2023. Dynamic assessment of spatiotemporal population distribution based on mobile phone data: a case study in Xining City, China. Int J Disaster Risk Sci. doi:10.1007/s13753-023-00480-3.
- Wu YM. 2003. Relationship between peak ground acceleration, peak ground velocity, and intensity in Taiwan. Bull Seismol Soc Am. 93(1):386–396. doi:10.1785/0120020097.
- Xia CX, Nie GZ, Pang XK, Fan XW, Zhou JX, Li HY. 2019. Research on the application of phone location data in the rapid delimitation of the Meizoseismal Area. Bull Seismol Soc Am. 109:2470–2490. doi:10.1785/0120190010.
- Xing ZY, Su X, Liu J, Su W, Zhang X. 2019. Spatiotemporal change analysis of earthquake emergency information based on Mi-croblog Data: a case study of the “8.8” Jiuzhaigou earthquake. IJGI. 8(8):359. doi:10.3390/ijgi8080359.
- Xing ZY, Zhang X, Zan D, Xiao XL, Li C, Han B, Liu KK, Liu JM. 2021. Crowdsourced social media and mobile phone signaling data for disaster impact assessment: a case study of the 8.8 Jiuzhaigou earthquake. Int J Disaster Risk Reduct. 58:102200. ISSN 2212-4209, doi:10.1016/j.ijdrr.2021.102200.
- Xu C,Xu X,Yao X,Dai F. 2014. Three (nearly) complete inventories of landslides triggered by the May 12, 2008 Wenchuan Mw 7.9 earthquake of China and their spatial distribution statistical analysis. Landslides. 11(3):441–461. doi:10.1007/s10346-013-0404-6.
- Xu C, Xu X, Zhou B, Yu G. 2013. Revisions of the M 8.0 Wenchuan earthquake seismic intensity map based on co-seismic landslide abundance. Nat Hazards. 69(3):1459–1476. doi:10.1007/s11069-013-0757-0.
- Xu TR, Dai DQ, Yang ZG, Xi N, Deng WZ, Zhang JY, Liu FL. 2022. Preliminary study of emergency production and source Parameters of the M6.8 earthquake on September 05, 2022 in Luding, Sichuan Province[J]. Earthquake Res China. 38(03):412–424.
- Yabe T, Jones N, Rao P, Gonzalez M, Ukkusuri S. 2022. Mobile phone location data for disasters: a review from natural hazards and epidemics. Comput Environ Urban Syst. 94:101777. doi:10.1016/j.compenvurbsys.2022.101777.
- Yabe T, Tsubouchi K, Sekimoto Y. 2018. Fusion of terrain information and mobile phone location data for flood area detection in rural areas. In 2018 IEEE International Conference on Big Data (Big Data). 881–890. doi:10.1109/BigData.2018.8622156.
- Yang XW, Song JG. 2022. A study of vegetation biomass in the Yellow River Delta wetlands based on regression analysis[J]. Agriculture and Technol. 42:32–35.
- Yin L, Wang Q, Shaw SL, Fang ZX, Hu JX, Tao Y, Wang W. 2015. Re-identification risk versus data utility for aggregated mobility research using mobile phone location data. PLoS One. 10(10):e0140589. doi:10.1371/journal.pone.0140589.
- Zhang JF, Xie LL, Tao XX. 2002. Change detection of remote sensing image for earthquake-damaged buildings and its application in seismic disaster assessment. J Nat Disaster. 2:59–64.