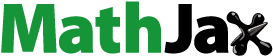
Abstract
Global droughts have become more frequent in recent years, posing a serious threat to human production and life. In order to overcome the limitations of traditional studies in quantifying drought risk, this study uses runs theory to extract drought duration, severity and kurtosis as drought characteristic variables from standardized precipitation index (SPI) in Henan Province. Drought risk analysis models based on multivariate copula function are constructed to reveal the response relationships between drought characteristic variables. The results show that the smaller the SPI scale, the higher the sensitivity to identify drought processes. In addition, the multivariate copula function shows good fitting performance for the optimal joint distribution of drought characteristic variables, with R2 values exceeding 0.9. Drought joint recurrence period is positively correlated with drought characteristic variables, and the recurrence period within the duration of 4–6 months occur frequently, indicating a higher probability of experiencing short-term droughts and cross-seasonal droughts. When the drought duration, severity and kurtosis are greater than 2.5, 2200 and 1300, respectively, the drought joint recurrence period reaches 20 months. The research results have provided new methods for drought risk analysis and data support for formulating drought mitigation strategies in Henan Province.
1. Introduction
Water resources are an invaluable and irreplaceable asset that supports sustainable development across the globe (Zhang et al. Citation2019). However, the water cycle on Earth is currently experiencing significant changes as a result of human activities and global climate change (Kogan et al. Citation2020). Almost half of the world’s countries are currently facing the threat of drought, posing a common challenge for humanity (Maeng et al. Citation2017; Bai et al. Citation2018). The 15th UN Convention to Combat Desertification’s "Drought Report" highlights that drought, which accounts for 15% of natural disasters, results in the highest number of deaths. Between 1970 and 2019, it caused approximately 650,000 deaths worldwide. Since 2000, the frequency and duration of global droughts have increased by 29%, affecting roughly 1.4 billion people. Between 1998 and 2017, the global economic losses attributed to drought reached a staggering $124 billion. The report suggests that by 2030, 700 million individuals will face the risk of displacement due to drought. The Intergovernmental Panel on Climate Change (IPCC) state (Lynn and Peeva Citation2021): most parts of the world will become more arid in future, and the degree of aridification will be remotely higher than the changes of the past millennium, highlighting the urgency of in-depth study of drought. Despite its severe implications, drought has become a major impediment to sustainable human development, owing to its intricate natural environment and multifarious impacts from various complex factors (Han et al. Citation2019). Drought risk analysis serves as a crucial metric to quantify the severity of drought to some extent and constitutes a crucial cornerstone for identifying and issuing early warnings.
Over the past decade, there has been a notable surge in research on drought risk, as evidenced by the explosive increase in the number of search results for "drought disaster risk" from 505,000 in 2016 to 6.13 million in 2020 (Liu et al. Citation2021). Drought and drought risk have become important research areas in the fields of natural disasters and sustainable development (Duan et al. Citation2022). For instance, Zhao Jing (Zhao et al. Citation2012) analyzed drought disaster risk and created a drought risk zoning graph using GIS, examining drought disasters in different regions and time periods. Meanwhile, Xu (Xu et al. Citation2015) developed a regional drought frequency analysis model based on copula functions by considering the spatial and temporal variability of drought events, and assessed and discussed the robustness of the drought frequency analysis as well as the extent of drought. In addition, Zhang (Zhang et al. Citation2015) constructed the joint distribution of drought characteristics by using the Archimedean Copula function. Based on the error analysis and tail dependence coefficient, the Gumbel-Hougaard copula is selected to analyse spatial distributions of the drought joint recurrence period. Wu (Wu et al. Citation2019) used the copula function to construct the joint distribution of drought risk management indicators in grassland ecosystems based on SPEI data, and the results indicated that the Frank Copula function could well simulate the joint dependence structure among the indicators. Zhong (Zhong et al. Citation2018) used on Bayesian maximum entropy method for optimal estimation of annual precipitation and calculated the annual standardized precipitation index. The hazard of drought was also assessed based on information diffusion and excess probability theory. Li (Li et al. Citation2020) proposed an overall framework and key technologies for quantitative evaluation of drought risk based on a scientific definition of drought risk. Richter (Richter and Semenov Citation2005) employed the Hadley Climate Center’s climate model and a stochastic weather generator to simulate the climate scenario for England in 2050 and evaluated the impact of future drought risk on wheat yield. Eleni (Kampragou et al. Citation2011) suggested that governments at all levels in the EU should shift from traditional drought crisis management to drought risk management at regional, watershed, and national levels. Kirono (Kirono et al. Citation2011) used global climate models (GCMs) to simulate future climate scenarios under high greenhouse gas emissions and analyzed drought characteristics in Australia from 2030 to 2050. Finally, Wilhite (Wilhite et al. Citation2000; Wilhite Citation2016; de Araujo et al. Citation2019) pointed out that current risk management strategies are inadequate to deal with the increasingly severe drought disasters, and argued for the need to manage drought as a risk in the context of changing climates. The copula function is flexible and versatile. On the one hand, it is possible to construct multidimensional joint distributions by considering the marginal distributions and correlation structures of different drought variables without loss of data information. On the other hand, the copula function model can account for the complex relationships between multiple variables, overcoming the limitations of traditional methods and providing a powerful framework for comprehensive drought risk analysis.
In summary, there are several shortcomings in the current analysis of drought risk. Firstly, the identification and analysis of drought risk often focuses on a single indicator, which is limited in scope. Secondly, there is a certain degree of missing data information in the processing and transformation of drought index data, which can lead to inaccurate analysis results. In addition, there is limited research on drought risk analysis using the construction of joint distributions of three or more dimensions. Therefore, it is crucial to establish a sound mechanism for drought risk analysis, reveal the response relationship between different drought characteristic variables, and accurately evaluate the drought situation in Henan Province.
This paper aims to construct a joint distribution among drought characteristic variables using a multivariate copula function to address the limitations of drought risk analysis in Henan Province. Firstly, the calculation of SPI based on precipitation data and the trend analysis of SPI values at different scales are carried out. Secondly, three drought characteristic variables, drought duration, drought severity, and drought kurtosis, were extracted from SPI1 based on runs theory. Then, the joint distribution among the drought characteristic variables is constructed based on the multivariate copula function. Finally, by calculating the conditional probability of the joint distribution among drought characteristic variables, the joint recurrence period is determined and the analysis of drought risk in Henan Province is realized. This study overcomes the information loss between data and achieves a comprehensive analysis of drought risk in Henan Province from a multivariate perspective.
2. Study area
Henan Province, known as Zhongyuan in ancient times, is located in the central and eastern regions of China, within the middle and lower reaches of the Yellow River. Henan is one of the major provinces in China with a large population and extensive agricultural production, covering an area of 165,500 square kilometers. shows the research area of Henan Province, where the terrain is mainly characterized by plains, complemented by hills and mountains. Specifically, hills account for 17.7% of the basin area, mountains account for 26.6%, and plains account for 55.7% of the basin area (Luo and Li Citation2023).
Henan Province has a diverse geological structure, which results in uneven precipitation distribution throughout the year and pronounced seasonal changes. Specifically, the winter season sees less precipitation, while the summer season experiences ample precipitation, and there are significant inter-annual variations (Wang et al. Citation2018). On average, the minimum precipitation is 500 mm, while the annual maximum precipitation is 900 mm. Additionally, there are notable differences in precipitation patterns across different regions, with a decreasing trend from south to north. Over the past 60 years (1951–2010), the average precipitation is 625.6 mm, and the precipitation trend rate is −4.542 mm/10a. The intergenerational characteristics of annual precipitation exhibit a downward trend from year to year, and the frequency of droughts has been as high as 58.2%. Given the constantly changing drought situation, the risk of drought is increasing every year.
2.1. Data used
Hydro-meteorological data forms the fundamental basis for analysis and calculation. In this study, the hydro-meteorological data for 18 cities in Henan Province from 1961 to 2010, including monthly precipitation, temperature, wind speed, relative humidity, and other observational data, were obtained from the China Meteorological Data Service Center (http://data.cma.cn).
In addition, the calculation and transformation of the multi-scale SPI based on the precipitation data were carried out, taking into account the current climate conditions. The analysis of drought risk in Henan Province was carried out at different time scales to improve the limitations of using only single precipitation data for risk analysis results.
3. Methodology
3.1. Calculation of the SPI
Proposed by McKee in 1993, the SPI (Belayneh et al. Citation2014; Li et al. Citation2020) describes the severity of drought with the probability of precipitation. This index applies to droughts on multiple time scales. Suppose precipitation x obeys gamma distribution. Then, the probability density of x, i.e. the SPI, can be described as:
(1)
(1)
where
is the shape factor;
is the scale factor;
is the gamma function. These parameters can be computed through maximum likelihood estimation (MLE):
(2)
(2)
The SPI-based drought classification standard () was established according to the Classification of Meteorological Drought (GB/T20481-2006) issued by the National Meteorological Center, CMA.
Table 1. SPI-base drought classification.
3.2. Runs theory
Based on the SPI 1, the runs theory (Zhang et al. Citation2015; Long et al. Citation2020) is used to extract three drought characteristic variables from them: drought duration, drought severity, and drought kurtosis. Three cut-off levels, namely are established. Drought occurs during a period when the standardized precipitation index sequence
for one or more periods is less than R1, indicating a negative run. The specific identification procedure is as follows: Drought duration (D, monthly) refers to the length of the period of two or more drought occurrences, which constitutes a negative run. Drought severity (S, 10,000 m3) is the area of the negative run. Drought kurtosis (K, 10,000 m3) refers to the extreme value of the negative run. The runs theory diagram is shown in .
3.3. Copula function
The copula function has a flexible and diverse form, and is easy to solve. In multivariate analysis, determining the marginal distribution of a single variable given the joint distribution of multiple variables is relatively straightforward. However, determining the joint distribution based on the marginal distributions of each variable can be challenging. Copula function theory has been proposed to address this problem. As a linking function (Li et al. Citation2021), it can transform the distribution between the two without compromising the data information of the random variables. According to the Sklar theorem (Afshar et al. Citation2016; Li et al. Citation2020), any multivariate joint distribution can be connected to single-variable marginal distribution functions and a copula function that describes the dependence structure between variables. The specific formula for this definition is as follows:
is the joint distribution of the marginal distribution
Let
then there exists an n-dimensional Copula function C. For any
we have
3.3.1. Two-dimensional copula functions
The Copula function is a uniform joint distribution function with marginal distributions restricted to the interval [0,1]. Let F(x) be the marginal distribution function of a one-dimensional random variable X, Let G(y) denote the marginal distribution function of a one-dimensional random variable Y, and H(x,y) denote their joint distribution function. The function C serves as a link function between the marginal and joint distribution functions. For fitting purposes in this study, an Archimedean Copula function is employed (see ).
Table 2. Two-dimensional Archimedean copula function.
3.3.2. Three-dimensional copula functions
The method for constructing a three-dimensional Copula function is similar to that of a two-dimensional Copula function. However, due to the tendency for drought data to exhibit skewed distribution, the drought characteristic variable data are fitted in this paper based on the Clayton Copula and Gumbel-Hougaard Copula functions (see ).
Table 3. Three-dimensional Archimedean copula function.
3.4. Goodness of fit test
Estimating Copula function parameters relies on the relationship between the rank correlation coefficient and parameter
Maximum likelihood estimation is commonly used to calculate Copula function parameters. However, it is still necessary to assess the goodness of fit of the joint distribution. Various test methods, such as the Ordinary Least Squares (OLS) and the Akaike information criterion method (AIC), can be employed to evaluate the goodness of fit. OLS is calculated as follows:
(3)
(3)
Where are the empirical and theoretical frequency value; n is the series length.
AIC is calculated as follows:
(4)
(4)
where m is the number of parameters.
The sizes of OLS and AIC reflect the degree to which the Copula function fits the original variable data, with smaller values indicating a better model fit.
3.5. Joint recurrence period
The joint recurrence period of drought is defined as the duration between the first and second occurrences of a drought. It is worth noting that the joint recurrence period for variables that exceed or equal a specific predetermined threshold is shown below (Li et al. Citation2022).
(5)
(5)
where Ti is the univariate recurrence period; F(Xi) is the marginal distribution function; N is the sample length; and n is the number of occurrences greater than a given sample.
Two-dimensional joint recurrence period expression is as follows:
(6)
(6)
Three-dimensional joint recurrence period expression is as follows:
(7)
(7)
where
is the joint recurrence period.
and
are the joint distribution function. u,v and w are the marginal distribution function of each variable.
In summary, this study uses precipitation data and multi-scale SPI values for 1961–2010 in Henan Province as the basis for analysing drought risk in Henan Province from a global perspective using the method described above, overcoming the loss of information during data conversion and the limitations of the analysis results. In addition, the data used in this paper were cleaned and validated for consistency and completeness.
4. Results and discussion
4.1. Mechanisms of drought formation
Anomalies in atmospheric circulation or insufficient water vapor can lead to decreased precipitation, which is a key indicator of the onset of drought (Wang T et al. Citation2023; Zhang et al. Citation2023). Droughts are typically characterized by five distinct stages, namely gestation, initial, buffer, development and relief. Anomalies in atmospheric circulation and insufficient water vapor serve as the gestation process for drought, while reduced precipitation is the direct cause of drought and marks the beginning of the process. If the rate of evaporation increases and water vapor is replenished in time, precipitation may increase. However, if water vapor cannot be replenished in time, precipitation will continue to decrease, and the drought process will continue for a longer period of time, which represents the buffering and development period of drought. After passing through the gestation period, the initial period, the buffer period, and the development period, with the cessation of abnormal circulation, the increase in precipitation and the supply of water vapor from external sources will lead to an increase in precipitation, which will eventually lead to the relief of drought. shows the process of drought formation and development.
The study of the processes that shape drought mechanisms can provide a fundamental understanding of drought. In addition, the influence factors of drought are complex, and its driving mechanism, dynamic response and propagation process are not very clear. Therefore, the study of the formation process, driving mechanism and dynamic response of drought can reveal the propagation mechanism of drought to a certain extent, and has certain guiding significance for drought early warning.
4.2. Multi-timescale analysis of drought
Given the complexity of the drought formation process and the multitude of factors involved, we calculate and transform drought characteristic indicator variables based on meteorological data from 1961–2010. Besides, we conduct a multi-scale analysis of drought in Henan Province using different SPI values (1, 3, 6, 9, 12, 24) to investigate the drought phenomenon. shows the fitted trend lines for SPI values at different time scales. The SPI is a representation of drought at different time scales and is one of the important indicators for characterizing drought, and SPI values at different time scales have different advantages. With smaller scale SPI values being more sensitive to drought response and have a higher sensitivity for identifying drought, which can characterize drought characteristics in a short period of time. In contrast, larger scale SPI values can accurately characterize the occurrence of drought over a longer time span. Therefore, using different scale SPI values in different analyses are crucial to improve the accuracy of drought research.
Table 4. SPI values fitted to trend lines.
From and , it can be seen that the SPI values at different time scales in Henan Province show an oscillatory process that varies over time, with a consistent trend of variation, with values fluctuating within the range of [-2.5, 2.5]. In particular, there are significant differences in the oscillation and changes of SPI values at different scales, with smaller scales showing more frequent and dramatic fluctuations. This observation suggests that the SPI values with smaller scales are more sensitive in identifying the occurrence of drought in Henan Province, and can promptly detect the process of drought occurrence within a short period. As the scale of the SPI value increases, the trend line of the SPI value oscillation becomes more stable, reflecting the cumulative effect of precipitation in the early stages on the process of drought occurrence.
Therefore, in studies, SPI values at the appropriate scale should be selected for the problem under study. At the same time, the application of multi-timescale SPI values not only allows for the timely identification of the degree of drought in the study area, but also provides an integrated picture of the effect of cumulative precipitation on drought. This has also been verified in the literature (Mehr and Vaheddoost Citation2020).
4.3. Drought evolution characterization
Based on the data of drought characteristic variables, the evolution law of drought duration, severity, and kurtosis is visualized in . It can be concluded that Zhumadian has a high frequency of drought occurrence with long duration and high intensity drought, followed by Pingdingshan, Luohe, and Zhoukou, while the drought intensity in Xinxiang, Puyang, Hebi, and Anyang is relatively lighter compared to other regions. Generally speaking, droughts are more likely to occur in central and southern Henan Province, while the frequency of droughts in northern Henan Province is relatively low. Dividing the region along the Yellow River, the frequency of drought occurrence is higher in the southern area of the Yellow River than in the northern area.
The evolution of the different drought characteristic variables visually demonstrates the drought evolution pattern in Henan Province. Through the analysis of this pattern, it is possible to grasp the dynamic evolution of drought in Henan Province, which can provide more comprehensive guidance to the relevant departments in their drought prevention work.
4.4. Drought risk analysis based on a two-dimensional copula function
4.4.1. Copula function preference
In order to improve the accuracy of drought risk analysis results in Henan Province, based on the analysis results, the SPI 1 with high drought identification sensitivity is selected as the data basis. The drought duration, severity, and kurtosis of 18 meteorological stations in Henan Province are extracted based on the runs theory, and they are set as random variables X, Y, and Z. Based on the two-dimensional Archimedean Copula function, the joint distribution of drought characteristic variables is constructed, and the optimal Copula function is selected based on the OLS and AIC, and a drought risk analysis model for Henan Province is established. The calculation results are shown in .
Table 5. Parameter estimation and goodness of fit results (Drought duration-severity).
Table 6. Parameter estimation and goodness of fit test results (Drought duration-kurtosis).
Table 7. Parameter estimation and goodness of fit test results (Drought severity-kurtosis).
According to the results are shown in , the Kendall and Spearman correlation coefficients of the joint distribution constructed by drought duration and severity fluctuate around 0.5, indicating a strong correlation between the two. In further calculations, the Frank Copula function has the smallest OLS and AIC among the three functions, with OLS = 0.0171 and AIC=−346.874. Therefore, the Frank Copula function is the most accurate choice for the joint distribution function of the two variables.
According to the results are shown in , it can be observed that the Kendall and Spearman coefficients for the joint distribution of drought duration and drought kurtosis show a slight fluctuation around 0.2. This indicates a weak correlation between the two. Additional calculations are performed to obtain a more precise selection of the joint distribution. Specifically, the OLS and the AIC for the Gumbel copula function were calculated and found to have minimum values of OLS = 0.065 and AIC = −347.104 respectively. As a result, it is recommended to use the Gumbel copula function in the joint distribution of the two variables for greater accuracy.
According to the results are shown in , the Kendall and Spearman coefficients of the joint distribution constructed by drought severity and drought kurtosis fluctuate around 0.6. In addition, the Spearman coefficient of the Frank Copula function reaches 0.8019, indicating an extremely strong correlation between drought severity and drought kurtosis. In further calculations, the OLS of the Gumbel copula function is 0.0269 and the AIC is −310.594. Both the OLS and AIC are the smallest of the three functions. Therefore, the Gumbel Copula function is selected to construct the joint distribution of drought severity and kurtosis for greater accuracy.
To further determine the fitting effect of the joint distribution of drought characteristic variables, a Q-Q plot is used to analyse the joint distribution between the variables. The Q-Q plot can intuitively express the fitting situation between the empirical and theoretical frequencies of the joint distribution. The empirical-theoretical joint distribution fitting plot for drought characteristic variables is shown in .
Figure 6. Fitting the empirical joint distribution to the theoretical joint distribution: (a), (c), (e) are QQ graphs of drought duration-severity, duration-kurtosis, and severity-kurtosis, respectively and (b), (d), (f) are theoretical and empirical frequency fits of drought duration-severity, duration-kurtosis, and severity-kurtosis, respectively.

According to , the Frank Copula function has a high goodness of fit between the theoretical and empirical frequencies of the joint distribution of drought duration and drought severity, with a correlation coefficient of R2=0.9985 and RMSE = 0.0098. Similarly, based on , the Gumbel Copula function has a high goodness of fit between the theoretical and empirical frequencies of the joint distribution of drought duration and drought kurtosis, with R2=0.9990 and RMSE = 0.0089. Furthermore, shows that the Gumbel Copula function has a high goodness of fit between the theoretical and empirical frequencies of the joint distribution of drought severity and drought kurtosis, with R2 = 0.9984 and RMSE = 0.0116. The correlation coefficients of the joint distribution of drought characteristic variables are all close to 1, and the error is less than 1%, indicating a high accuracy of different Copula functions in fitting the joint distribution of drought characteristic variables.
4.4.2. Joint probability distribution
In view of the above calculation results, the optimal joint distribution between drought duration and drought severity is the Frank Copula function with a parameter value of 6.309, while the optimal joint distributions between drought duration and drought kurtosis, and between drought severity and drought peak are both the Gumbel Copula function with parameter values of 1.256 and 2.524, respectively. On this basis, the probability density functions and empirical distribution functions of between the drought characteristic variables are shown in . The Frank Copula function has no restrictions on the correlation between random variables (Kavianpour et al. Citation2018; Fan et al. Citation2022), making it suitable for both positively and negatively correlated variables. The image feature of the Frank Copula function is symmetric in the tails and asymptotically independent between the upper and lower tails. The probability density function graph of the Gumbel Copula function has a "J"-shaped distribution, with a high upper tail and a low lower tail, and is not symmetric between the upper and lower tails.
Figure 7. The Probability density and empirical distribution function graphs: (a), (c), (e) are graphs of the joint density function between the drought characteristic variables, respectively and (b), (d), (f) are graphs of the joint distribution function between the drought characteristic variables, respectively

Based on , it can be observed that the probability density distribution of the two random variables of drought duration and severity have symmetrical tails and relatively stable upper and lower tail dependence, with the probability density concentrated around 4–5 months. On the other hand, shows that the probability density distribution of drought duration and kurtosis, drought severity kurtosis are highly sensitive to changes in the upper tail, and even subtle changes in the upper tail can be identified and captured, making it more sensitive for drought identification. Furthermore, in , the probability density is concentrated around 3–4 months, while in , it is concentrated around 10–15 months. In summary, the construction of a risk analysis model for drought characteristic variables using the Frank Copula and Gumbel Copula functions is of great significance for improving the accuracy of drought risk analysis in Henan Province.
Based on the joint distribution function of drought characteristic variables, it can be observed that the joint distribution of drought duration and severity, drought duration and kurtosis, and drought severity and kurtosis show consistent trends. As the probability of the marginal distribution of drought characteristic variables increases, the probability of the joint distribution also increases, and the growth trend is relatively stable. In summary, the probability values of droughts occurring in 3–5 months and 10–15 months are more concentrated. Therefore, it can be inferred that droughts in Henan Province are more likely to occur in 3–5 months and around 10–15 months, indicating a tendency towards medium-term and annual droughts, with a relatively stable development trend during the study period, and the frequency of cross-seasonal droughts is relatively high.
4.4.3. Joint recurrence period
The joint recurrence period of drought refers to a drought duration (severity, kurtosis) that is greater than or equal to the given drought duration (severity, kurtosis) value, which means that the drought of that duration (severity, kurtosis) is considered to have recurred once. In order to accurately understand the drought situation in Henan Province, the joint recurrence period and contour distribution graph were plotted based on the joint distribution function of the drought characteristic variables. The results are shown in .
Figure 8. Graphs of the joint recurrence period of drought characteristic variables: (a), (c), (e) are joint recurrence period graphs between drought duration-severity, duration-kurtosis, and severity-kurtosis, respectively and (b), (d), (f) are joint recurrence period contour graphs between drought duration-severity, duration-kurtosis, and severity-kurtosis, respectively.

Analysis of , it can be observed that for the joint distribution of drought duration and severity, when the drought duration and severity are less than 1 and 1000, respectively, the joint recurrence period is less than 4 months. When the drought severity is between 1000 and 1700, and the drought duration is between 1 and 1.8, the joint recurrence period is between 4 and 6 months. When the drought severity is between 1700 and 2200, and the drought duration is between 2 and 2.2, the joint recurrence period is approximately 7 months. When the drought severity is greater than 2500, and the drought duration is greater than 2.5, the joint recurrence period increases rapidly and reaches between 12 and 16 months.
From , we know that for the joint distribution of drought duration and drought kurtosis, when drought duration and kurtosis are less than 1 and 800, respectively, the joint recurrence period is between 0 and 4 months. When the drought kurtosis is between 800 and 1000 and the drought duration is between 1 and 2, the joint recurrence period increases to between 4 and 6 months. Furthermore, when the drought kurtosis increases to between 1200 and 1400, and the drought duration is between 2 and 2.5, the joint recurrence period further increases to approximately 9 months. Finally, for drought events with higher kurtosis and longer duration, the joint recurrence period increases rapidly, reaching a peak of between 12 and 16 months.
Based on , it can be seen that for the joint distribution of drought severity and drought kurtosis, when either the drought severity is less than 1000 or the drought kurtosis is less than 800, the joint recurrence period is less than 4 months. When the drought severity is between 1000 and 1800 and the drought kurtosis is between 800 and 1200, the joint recurrence period is between 4 and 6 months. When the drought severity is between 1800 and 2200 and the drought kurtosis is between 1200 and 1300, the joint recurrence period is 7 months. As the drought kurtosis value increases, the joint recurrence period increases rapidly and reaches 15 months.
In summary, the joint recurrence period of drought is closely related to the changes of drought characteristic variables, and the length of its period shows a positive correlation with the trend of these variables. The drought risk analysis model based on the Frank Copula and Gumbel Copula functions shows that when the drought duration is less than 1, and both the drought severity and kurtosis are less than 1000 and 800, respectively, the joint recurrence period of drought is less than 4 months, which means that droughts occur more frequently and short-term droughts are very likely to occur. When the drought duration is between 1 and 1.8, the drought severity is between 1000 and 1800, and the drought kurtosis is between 800 and 1000, the joint recurrence period of drought is 4–6 months. At this time, it is easy for medium and long-term drought to occur, and cross-seasonal drought is also likely to occur, which will significantly affect people’s lives. As the values of drought characteristic variables continue to increase, when the drought duration is greater than 2.5, the drought severity is greater than 2200, and the drought kurtosis is greater than 1300, the joint recurrence period of drought suddenly increases, which indicates that the probability of drought occurring during this period is very low.
Compared to risk analysis models based on grey theory and principal component analysis (Xu et al. Citation2021; Yu et al. Citation2021), the copula function can construct joint distributions between drought characteristic variables without loss of data information, while dealing with non-linear and non-normal dependencies between drought characteristic variables. This makes the model more applicable to drought risk analysis and provides greater accuracy when dealing with complex data. In addition, it can provide accurate data for disaster warning and economic loss estimation.
4.5. Drought risk analysis based on a three-dimensional copula function
Based on the extracted values of drought characteristic variables, with drought duration as the random variable X, drought severity as the Y, and drought kurtosis as the random variable Z, a joint distribution of drought characteristic variables is constructed using the three-dimensional Archimedean Copula function. Considering strong positive correlation between the drought characteristic variables in the aforementioned study, the three-dimensional Gumbel-Hougaard Copula function is chosen for the joint distribution construction. The results of the goodness of fit test and parameter estimation are shown in .
Table 8. Results of the goodness of fit parameter calculation.
According to , in the joint distribution of drought duration-severity-kurtosis constructed by the Gumbel-Hougaard Copula function, the Kendall and Spearman correlation coefficients are 0.55 and 0.74, respectively, both of which are greater than 0.5. This indicates a strong positive correlation between the drought characteristic variables. In addition, the OLS value of the joint distribution is 0.023, and the AIC value is −325.633. The joint distribution constructed by this function has a high goodness of fit.
Based on the above function formulas, a three-dimensional joint distribution is constructed, and a drought risk analysis model is established for risk analysis. The results are shown in and . From the analysis of the three-dimensional joint probability trend graphs (), we know that droughts have become more frequent in Henan Province between 1961 and 2010, with droughts occurring every year, and most of them are mild droughts. However, severe droughts occurred in 1972, 1980, 1987, 1992, and 2000. In addition, as the marginal distribution probability of any of the drought characteristic variables increases, the joint distribution probability shows a tendency to increase. When two drought characteristic variables have a certain probability value, the joint distribution probability of the three variables increases and tends to a specific value as the other variable increases, while the value of the joint distribution probability eventually tends to 1 when all three variables increase at the same time.
The analysis in shows a strong positive correlation between the three variables. When the joint probability of drought duration-severity is between 0 and 0.45, or the joint probability of drought severity-kurtosis is between 0 and 0.4, the drought recurrence period is 5 months, and droughts occur frequently during this period, mostly mild droughts. When the joint probability of drought duration-severity is between 0.45 and 0.78 or the joint probability of drought severity-kurtosis is between 0.45 and 0.8, the drought recurrence period is 10 months, and moderate droughts are more likely to occur during this period, but less frequent. Moreover, when the joint probability value of drought duration-severity is greater than 0.5, the joint recurrence period is at 45 months, and when the value of drought severity-kurtosis is greater than 0.7, the joint recurrence period is 35 months. Therefore, with an increase in the joint probability of drought duration-severity, duration-kurtosis and severity-kurtosis, the contour values of the joint recurrence period become larger and the spacing becomes smaller. This shows that as the joint probability of any two of the three drought characteristic variables increases to a certain extent, the drought density increases, and severe droughts are more likely to occur.
In summary, the three-dimensional copula function constructs a multidimensional joint probability distribution by considering the marginal distributions and correlation structure of arbitrary drought variables. It overcomes the limitations of traditional approaches in quantifying drought risk analysis in Henan Province. The flexible and diverse form of this function provides new means to study drought risk analysis (Wei et al. Citation2023). Furthermore, the traditional two-dimensional copula function has limitations in revealing the correlation between drought variables in Henan Province and drought risk. The three-dimensional copula function model can comprehensively consider more drought variables and fully reveal the response relationships between them (Seyedabadi et al. Citation2020).
Moreover, in the analysis of drought risk in Henan Province, the three-dimensional copula function has a better fit to the drought variables than the two-dimensional copula function. This indicates that the multivariate joint distribution has greater advantages in the analysis of drought risk in Henan Province. However, further research on parameter estimation and function selection is still needed to improve the model. Furthermore, by combining the results of the two-dimensional and three-dimensional copula function models, it is found that Henan Province is highly prone to seasonal drought, with mild drought dominating. This research result can provide theoretical support for the drought warning work of government departments.
5. Conclusions
Drought is influenced by many factors and its driving mechanisms are complex. In order to overcome the limitations of traditional studies in quantifying drought risk and to reveal the response relationships between different drought characteristic variables, this study uses runs theory to extract drought duration, severity and kurtosis from standardized precipitation index (SPI) in Henan Province and constructs drought risk analysis models based on multivariate Copula function. The results are as follows.
The SPI shows good sensitivity in drought identification, with higher sensitivity at smaller scales. Between 1961 and 2010, Henan Province was prone to mild drought. However, severe drought occurred in 1972, 1980, 1987, 1992, and 2000.
The optimal fitting functions for the joint distribution between drought duration-severity, duration-kurtosis, and severity-kurtosis are Frank copula and Gumbel copula, with R2 values of 0.9985, 0.9990, and 0.9984, respectively.
The drought recurrence period is positively correlated with the values of drought characteristic variables. There is a higher probability of drought recurrence period occurring between 4 to 6 months. This suggests that droughts are more frequent in Henan Province, with a high probability of short-term and cross-seasonal droughts.
Analysing the different areas in the study region, droughts are more frequent in central and southern Henan and less frequent in northern Henan, divided along the Yellow River, droughts are more frequent in areas south of the Yellow River compared to areas north of the Yellow River.
In summary, the three-dimensional Copula function fits the drought variables better than the two-dimensional Copula function in the analysis of drought risk in Henan Province, which indicates that the multivariate joint distribution has greater advantages in the analysis of drought risk in Henan Province. However, further research on parameter estimation and function selection process is still needed to improve the model.
Authors’ contributions
Yunliang Wen: investigation, conceptualization, methodology, data curation, software, formal analysis, writing-original draft, visualization. Liwei Zhou: conceptualization, methodology, data curation, software, formal analysis. Ling Kang: supervision, validation, resources, writing-review & editing, funding acquisition. Hao Chen: data curation, software, formal analysis, validation. Jinlei Guo: data curation, software, visualization
Disclosure statement
No conflict of interest exits in the submission of this manuscript, and manuscript is approved by all authors for publication.
Data availability statement
The data that support the findings of this study are available on request from the corresponding author, Yunliang Wen.
Additional information
Funding
References
- Afshar MH, Sorman AU, Yilmaz MT. 2016. Conditional copula-based spatial-temporal drought characteristics analysis – a case study over Turkey. Water. 8(10):426. doi:10.3390/w8100426.
- Bai X, Wang YM, Jin JL, Qi XM, Wu CG. 2018. Precondition cloud and maximum entropy principle coupling model-based approach for the comprehensive assessment of drought risk. Sustainability. 10(9):3236. doi:10.3390/su10093236.
- Belayneh A, Adamowski J, Khalil B, Ozga-Zielinski B. 2014. Long-term SPI drought forecasting in the Awash River Basin in Ethiopia using wavelet neural network and wavelet support vector regression models. J Hydrol. 508:418–429. doi:10.1016/j.jhydrol.2013.10.052.
- de Araujo WC, Esquerre KPO, Sahin O. 2019. Building a system dynamics model to support water management: a case study of the semiarid region in the Brazilian Northeast. Water. 11(12):2513. doi:10.3390/w11122513.
- Duan C, Zhang J, Chen Y, Lang Q, Zhang Y, Wu C, Zhang Z. 2022. Comprehensive risk assessment of urban waterlogging disaster based on MCDA-GIS integration: the case study of Changchun, China. Remote Sens. 14(13):3101. doi:10.3390/rs14133101.
- Fan JJ, Xu FF, Sun X, Dong W, Ma XJ, Liu GP, Cheng Y, Wang H. 2022. Construction and application of hydrometeorological comprehensive drought index in Weihe River. Atmosphere. 13(4):610. doi:10.3390/atmos13040610.
- Han Z, Huang S, Huang Q, Leng G, Wang H, Bai Q, Zhao J, Ma L, Wang L, Du M. 2019. Propagation dynamics from meteorological to groundwater drought and their possible influence factors. J Hydrol. 578:124102. doi:10.1016/j.jhydrol.2019.124102.
- Kampragou E, Apostolaki S, Manoli E, Froebrich J, Assimacopoulos D. 2011. Towards the harmonization of water-related policies for managing drought risks across the EU. Environ Sci Policy. 14(7):815–824. doi:10.1016/j.envsci.2011.04.001.
- Kavianpour M, Seyedabadi M, Moazami S. 2018. Spatial and temporal analysis of drought based on a combined index using copula. Environ Earth Sci. 77(22):769. doi:10.1007/s12665-018-7942-0.
- Kirono DGC, Kent DM, Hennessy KJ, Mpelasoka F. 2011. Characteristics of Australian droughts under enhanced greenhouse conditions: results from 14 global climate models. J Arid Environ. 75(6):566–575. doi:10.1016/j.jaridenv.2010.12.012.
- Kogan F, Guo W, Yang WZ. 2020. Near 40-year drought trend during 1981–2019 earth warming and food security. Geomatics Natural Hazards Risk. 11(1):469–490. doi:10.1080/19475705.2020.1730452.
- Li KW, Tong ZJ, Liu XP, Zhang JQ, Tong SQ. 2020. Quantitative assessment and driving force analysis of vegetation drought risk to climate change: methodology and application in Northeast China. Agric Meteorol. 282–283:107865. doi:10.1016/j.agrformet.2019.107865.
- Li J, Peng T, Dong X, Li Z, Wang G, Chang W, Lin Q, Wang J. 2022. Hydrological drought risk in the Hanjiang River Basin based on copula function. Res Soil Water Conserv. 29(3):179–188.
- Li YL, Gong YJ, Huang CY. 2021. Construction of combined drought index based on bivariate joint distribution. Alexandria Eng J. 60(3):2825–2833. doi:10.1016/j.aej.2021.01.006.
- Li YL, Wen YL, Lai HX, Zhao QQ. 2020. Drought response analysis based on cross wavelet transform and mutual entropy. Alexandria Eng J. 59(3):1223–1231. doi:10.1016/j.aej.2020.01.050.
- Li ZL, Shao QX, Tian QY, Zhang LI. 2020. Copula-based drought severity-area-frequency curve and its uncertainty, a case study of Heihe River basin, China. Hydrol Res. 51(5):867–881. doi:10.2166/nh.2020.173.
- Liu J, Huang L, Zuo Q, 1. School of Management Engineering, Zhengzhou University, Zhengzhou 450001, China, 2. Yellow River Institute for Ecological Protection & Regional Coordinated Development, Zhengzhou University, Zhengzhou 450001, China, 3. Center for Water Science Research, Zhengzhou 450001, China. 2021. Evaluation of harmonious development of economy-population-resource-environment in the lower reaches of the Yellow River. Res Sci. 43(2):412–422. doi:10.18402/resci.2021.02.18.
- Liu Y, Liu B, Cheng C, Qiu M, Wang K, Mao L, He Y. 2021. Bibliometric analysis on the effect of climate change to apple based on Citespace. J Fruit Sci. 38(10):1748–1759.
- Long R, Chang J, Zhang H, Wang Y. 2020. Risk of meteorological drought in Lancang River Basin as analyzed by Copula. J Beijing Norm Univ Nat Sci. 56(2):265–274.
- Luo D, Li LS. 2023. Spatiotemporal evolution analysis and prediction of drought in Henan Province based on standardized precipitation evapotranspiration index. Water Supply. 23(1):410–427. doi:10.2166/ws.2022.443.
- Lynn J, Peeva N. 2021. Communications in the IPCC’s sixth assessment report cycle. Clim Change. 169(1–2):18. doi:10.1007/s10584-021-03233-7.
- Maeng SJ, Azam M, Kim HS, Hwang JH. 2017. Analysis of changes in spatio-temporal patterns of drought across South Korea. Water. 9(9):679. doi:10.3390/w9090679.
- Mehr AD, Vaheddoost B. 2020. Identification of the trends associated with the SPI and SPEI indices across Ankara, Turkey. Theor Appl Climatol. 139(3–4):1531–1542. doi:10.1007/s00704-019-03071-9.
- Richter GM, Semenov MA. 2005. Modelling impacts of climate change on wheat yields in England and Wales: assessing drought risks. Agric Syst. 84(1):77–97. doi:10.1016/j.agsy.2004.06.011.
- Seyedabadi M, Kavianpour M, Moazami S. 2020. Multivariate drought risk analysis based on copula functions: a case study. Water Supply. 20(6):2375–2388. doi:10.2166/ws.2020.153.
- Wang F, Sun M, Lu S, Zhou Z. 2018. Analysis of spatial-temporal evolution of agricultural drought based on regional agricultural drought index. DWT. 112:351–356. doi:10.5004/dwt.2018.22344.
- Wang T, Tu XJ, Singh VP, Chen XH, Lin KR, Zhou ZL, Tan Y. 2023. Assessment of future socioeconomic drought based on CMIP6: evolution, driving factors and propagation. J Hydrol. 617:129009. doi:10.1016/j.jhydrol.2022.129009.
- Wei L, Yang XJ, Wang XD. 2023. Study on the wetness-dryness encountering for mainstream and tributary of Luanhe River Basin based on three-dimensional copula function. Haihe Water Res. 239(01):87–91.
- Wilhite DA. 2016. Managing drought risk in a changing climate. Clim Res. 70(2):99–102. doi:10.3354/cr01430.
- Wilhite DA, Hayes MJ, Knutson C, Smith KH. 2000. Planning for drought: moving from crisis to risk management. J Am Water Resources Assoc. 36(4):697–710. doi:10.1111/j.1752-1688.2000.tb04299.x.
- Wu RN, Zhang JQ, Bao YH, Guo EL. 2019. Run theory and copula-based drought risk analysis for Songnen Grassland in Northeastern China. Sustainability. 11(21, 6032). doi:10.3390/su11216032.
- Xu HF, Xu KX, Yang YJ. 2021. Risk assessment model of agricultural drought disaster based on grey matter-element analysis theory. Nat Hazards. 107(3):2693–2707. doi:10.1007/s11069-021-04681-1.
- Xu K, Yang DW, Xu XY, Lei HM. 2015. Copula based drought frequency analysis considering the spatio-temporal variability in Southwest China. J Hydrol. 527:630–640. doi:10.1016/j.jhydrol.2015.05.030.
- Yu J, Kim JE, Lee JH, Kim TW. 2021. Development of a PCA-based vulnerability and copula-based hazard analysis for assessing regional drought risk. KSCE J Civ Eng. 25(5):1901–1908. doi:10.1007/s12205-021-0922-z.
- Zhang BQ, Wu PT, Zhao XN. 2023. Integrating water deficit quantification, rainwater regulation, and precision irrigation for drought resistance. Sci Bull (Beijing). 68(1):48–52. doi:10.1016/j.scib.2022.12.028.
- Zhang D-D, Yan D-H, Lu F, Wang Y-C, Feng J. 2015. Copula-based risk assessment of drought in Yunnan province, China. Nat Hazards. 75(3):2199–2220. doi:10.1007/s11069-014-1419-6.
- Zhang S, Zhang H, Xin C, Nan Z, Li Z. 2019. A method for characterizing trends and morphological changes of hydrological series. Water Res Protect. 35(6):58–67.
- Zhong SB, Wang CL, Yang YS, Huang QY. 2018. Risk assessment of drought in Yun-Gui-Guang of China jointly using the standardized precipitation index and vulnerability curves. Geomat Nat Hazards Risk. 9(1):892–918. doi:10.1080/19475705.2018.1480537.
- Zhao J, Zhang J, Yan D, Tong Z, Liu X. 2012. Drought disaster risk zoning in the Northern Henan Province based on gridding GIS. J Catastrophol. 27(1):55–58.