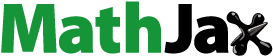
Abstract
This manuscript aims to develop a new multivariate composite index for monitoring agricultural drought. To achieve this, the AVHRR, VIIRS, CHIRPS data series over a period of 40 years, rainfall and crop yield data as references were used. Variables include parameters for vegetative stress (SVCI, PV, SMN), water stress (PCI, RDI, NRDI), and heat stress (SMT, TCI, STCI), and a new variable related to environmental conditions was calculated through a normalized rainfall efficiency index. Then, random forest algorithm was used to determine the weights of each component of the model by considering interannual fluctuations in cereal yields as an impact variable. The multivariate composite model was compared to the VHI, NVSWI and SPI-12 indices for validation. The results show a large spatiotemporal concordance between the MDCI and the validation indices with a maximum correlation of 0.95 with the VHI and a highly significant p value (< 2.2e-16). Validation of the MDCI model by SPI-12 shows a significantly higher statistically significant relationship than that observed between SPI and VHI and NVSWI. P value range from 3.531e-05 to 6.137e-06 with correlations that vary between 0.6 and 0.64 depending on the station. It is also highly correlated with the Palmer drought severity index (PDSI) and climatic water deficit index (CWDI), with R = 0.85 and p value < 5.8e-10 and R = 0.72 and p value < 1.9e-6, respectively. Finally, the study provides a new direction for multivariate modeling of agricultural drought that should be further explored under various agroclimatic conditions.
1. Introduction
Agricultural drought is a recurrent intraseasonal phenomenon in several regions of the world (Sharara et al. Citation2022). It is a natural feature of the climate that can occur in any climate regime (Svoboda and Fuchs Citation2016). Unlike other types of droughts, agricultural drought is characterized by a lack of sufficient moisture in the surface layers of the soil to support crop and forage growth (Gao et al. Citation2021; Das et al. Citation2021). In addition to the precipitation deficit, the soil moisture deficit that can affect agricultural productivity is often associated with a poor spatiotemporal distribution of seasonal precipitation and the simultaneous occurrence of heat waves. The concept of agricultural drought has its origins in these multifactor interrelationships that do not depend solely on the deficit of rainfall. Thus, the monitoring and evaluation of agricultural drought is more demanding in terms of variables and processes than meteorological or hydrological drought.
Traditionally, drought monitoring is based on climate data provided by a network of weather stations (Klein Citation2009; Nam et al. Citation2018). Indices of precipitation standardized or by a combination of climatological and hydroclimatic data such as the Palmer Drought Severity Index are the most widely used. In most countries, weather station networks are sometimes poorly or unrepresentative of the spatial diversity of agricultural and climatic landscapes (Li and Heap Citation2014; Hazaymeh and Hassan Citation2016; Nam et al. Citation2018); therefore, local variability related to topography or latitude is rarely considered (Lebourgeois and Piedallu Citation2005). The other disadvantage of this approach is that some indices used are only relevant when calculated on long data series of at least 30 years (Szczypta Citation2012). For example, hydroclimatic indices such as SPI, PDSI, and SPEI have been widely used to assess drought conditions (Palmer Citation1965; Liu et al. Citation2018; Tian et al. Citation2018; Al Shoumik et al. Citation2023). However, they are often calculated on a large spatial scale, which does not allow for effective monitoring of drought conditions at the agricultural plot level, and their accuracy is mixed when calculated on incomplete or inaccurate datasets. In addition, they are sometimes calculated at monthly or seasonal time scales, which does not allow for the monitoring of rapid changes in crop water stress conditions and makes it difficult to assess short-term agricultural drought (Liu et al. Citation2016). To overcome these limitations, univariate biophysical indices, such as the temperature condition index (TCI), precipitation condition index (PCI), soil moisture condition index (SMCI), and vegetation condition index (VCI), as well as bivariate indices, such as the vegetation health index (VHI) and normalized vegetation supply water index (NVSWI), have been introduced in the scientific literature since the work of Kogan (Citation1995). They have been successfully used for various applications to assess the impacts of weather on vegetation and soils (Zhuo et al. Citation2016; Gidey et al. Citation2018; Teweldebirhan et al. Citation2019; Kukunuri et al. Citation2022). However, while these indices are valuable tools for assessing drought conditions, changes in agricultural and land use practices can significantly affect their comparability across different time periods and geographic regions. This means that no single simple index taken individually can satisfy all aspects of agricultural drought (Tian et al. Citation2018; Wu et al. Citation2023; Nugraha et al. Citation2023). For this reason, multivariate composite models that include other environmental characteristics specific to each geographic condition may be better suited to describe the drought state conditions of an agrosystem.
Indeed, according to the number of variables considered in the formulation of drought indices, three main families can be distinguished: monovariate indices, bivariate indices, and composite indices. Monovariate indices are the simplest to implement because they require a single biophysical or hydroclimatic variable. However, this characteristic makes them more sensitive to the influence of sometimes nonclimatic factors that can affect their ability to reproduce drought conditions properly. Much of it has been developed under specific geographical conditions (Gao et al. Citation2021; Alahacoon and Edirisinghe Citation2022); therefore, its performance in other geographical regions has been shown to be limited (Shamshirband et al. Citation2020; Hanadé et al. Citation2022a). Indeed, the use of certain monovarietal indices by remote sensing, such as the VCI and the TCI, presents some biases. On the one hand, they do not take into account climatic variables such as variation in precipitation, which is one of the factors influencing drought in semiarid areas (Du et al. Citation2013), and on the other hand, negative anomalies in the vegetation condition expressed in the NDVI data record can be related to many types of stress (e.g. excessive humidity, heat stress, pest infestation, change in agricultural practices such as irrigation or not.) on plants in addition to drought (Nam et al. Citation2018). To minimize these sources of imprecision, several previous studies have suggested that the combination of several biophysical variables tends to improve the concordance between climate and remote sensing indices (Cao et al. Citation2019; Han et al. 2021; Hanadé et al. 2022). Therefore, reliable monitoring of agricultural drought would require the integration of multiple climatic, hydroclimatic, and biophysical covariables and/or indices from different sources to monitor several aspects of drought. It is in this sense that we are increasingly witnessing the emergence of new approaches combining both data from different sources, particularly those of remote sensing, and the use of advanced matrix intelligence algorithms in multivariate drought modeling (Bayissa et al. Citation2019; Ghazaryan et al. Citation2020; Jiménez-Donaire et al. Citation2020; Wang and Yu Citation2021; Mokhtar et al. Citation2021; Hara et al. Citation2021; Han et al. Citation2021b; Chandrasekara et al. Citation2021). Due to the increasing availability of multisource data, particularly those of multisensory remote sensing, a wide variety of composite indices have been proposed to approach the requirements of operational monitoring of the state of drought (Rajsekhar et al. Citation2015; Waseem et al. Citation2015; Senapati and Das Citation2022; Yang et al. Citation2022; Naderi et al. Citation2022; Ali et al. Citation2022). Thus, multivariate composite models have progressed in popularity because of their multidimensionality, which offers them the ability to summarize the complex multifactorial processes underlying the occurrence and worsening of agricultural drought intensity. In recent literature, to adequately link the interrelationships of drought variables, the use of machine learning (ML) for agricultural drought monitoring is the most emerging approach to constructing drought indices (Rahmati et al. Citation2020; Han et al. 2021a). This is due to several advantages of ML over other methods, such as statistical, probabilistic and time series modeling. One of the success factors of using ML models is that they allow for many of the variables and complex multisource data, which can significantly improve the accuracy and reliability of the results obtained. Thus, unlike statistical, chronological, and probabilistic methods, the use of ML makes it possible to take into account together variables whose spatiotemporal variability changes instantaneously and the static determinants of drought status (Park et al. Citation2019; Prodhan et al. Citation2021, Saha et al. Citation2021). For example, time series of in situ meteorological variables at several scales (precipitation, temperatures) and remotely sensed biophysical variables that are causal factors with dynamic variability are often associated in LMs with other factors that can be described as static factors of worsening drought status, such as topographical factors and soil types for a better assessment of the state of drought. However, statistical, time and probabilistic methods require historical time series of at least 30 years to achieve the best results (Jiang et al. Citation2023), which makes it difficult to integrate static factors such as topographic moisture, topographic heterogeneity, soil water retention and physiographic diversity of landforms. In general, statistical methods are only suitable for linear applications, and their applications to unstable data are limited. In contrast, the mathematical underpinnings of ML algorithms such as neural networks and decision trees give them the ability to detect interrelationships between covariates, hidden trends, and complex patterns in data even when these are not obvious or difficult to identify with conventional statistical methods. Additionally, due to recent developments in information technology and computing, including connected IoT platforms, ML models can be continuously fed with meteorological, environmental, and remotely sensed data to ensure real-time and constant monitoring of drought conditions (Kaur and Sood 2020a; Kaur and Sood Citation2020b; Hoang et al. Citation2020). However, it should be emphasized that the use of traditional methods may be more reliable when one is interested in the analysis of the dynamics of the frequency intensity of drought or the historical retrospective of drought parameters without highlighting the biophysical and environmental impacts of drought. Thus, the above suggests that the best approach to drought modeling may emerge from a combination of traditional and smart methods.
In this regard, Dixit and Jayakumar (Citation2022) developed the copula-based probabilistic multivariate drought index (MDI) to adequately estimate three types of droughts by incorporating simulated precipitation, evapotranspiration, soil moisture and flow data. MDI is an excellent framework for monitoring the multivariate future dynamics of drought conditions, although early findings of the approach are mixed, as they contradict global climate trends in most climate models that predicted exacerbation of extreme weather conditions.
Due to the stochastic and multifactorial dimension of drought, multivariate indices that simultaneously provide information on different types of droughts tend to provide a more reliable representation of drought status than univariate indices (Hao and Singh Citation2015; Hanadé et al. Citation2022b). The MDI is a multivariate index that considers the hydrological dimension of drought through information on flows and evapotranspiration, agricultural drought through information on soil moisture and meteorological drought through information on rainfall impacts of climate change, and its performance in integrated assessment. The MDI approach has the advantage that it is applicable for prospective and future assessments of integrated drought conditions. Similarly, the use of the Archimedean copula makes it possible to integrate the intensity of dependence of drought covariates into the assessment of drought severity, which is not the case in other multivariate models based on statistical methods such as principal component analysis (Li et al. Citation2015; Mansour Badamassi et al. Citation2020; Ali et al. Citation2022). However, MDI does not include the sociohuman dimension of drought severity or physiographic and environmental determinants that are likely to aggravate drought severity. These elements reflect the intrinsic sensitivity of agrosystems in most drought-prone regions. For short-term monitoring of agricultural drought parameters, Prodhan et al. (Citation2021) proposed a deep learning-based approach to model the SMDI index as a key agricultural drought response variable by incorporating twelve variables of causes and worsening of drought status. The approach seems particularly suitable for monitoring the severity of drought according to different phenological stages. However, the approach does not involve the use of other environmental covariables, such as rainfall efficiency, groundwater storage, topographical variables, and land use practices, that are likely to affect the quantitative estimation of drought status. In this direction, Bageshree et al. (Citation2022) recently developed a holistic framework for quantifying and classifying the state of seasonal agricultural drought in the central state of Maharashtra in India called a multivariate joint drought index (JDI) based on copula and PCA. It is one of the few approaches that also suggests remotely sensed indices, hydroclimatic indices (SPEI), hydrological indices (SSI), groundwater and runoff. However, despite a possible improvement in the detection of combined drought variability with the JDI, vegetation response variables to drought, such as NDVI and VCI, are not considered in the JDI model configuration. However, vegetation response to different levels of drought stress intensity is an essential component in multivariate descriptive modeling of agricultural drought. Another scientific advance in multivariate drought modeling is the approach developed by Schwartz et al. (Citation2022) combining ten environmental variables related to water supply and demand. In terms of the number of subindicators, the approach covers several drought processes; however, the drought detection performance of the CCDI model is relatively low, especially in irrigated agrosystems. Two other composite models, namely, a novel Comprehensive Agricultural Drought Index (CADI) proposed by Tian et al. (Citation2022) and a multivariate drought response index (ADRI) developed in the Marathwada region by Singh et al. (Citation2022), showed slightly better performance than the CCDI model. Using machine learning models, many other evidence-based studies have focused on the predictive dimension of agricultural drought severity, agricultural drought vulnerability modeling or integrated quantitative modeling of agricultural drought parameters, i.e. combining multisource data from remote sensing, climate modeling and in situ data. In northeastern Bangladesh, Al Kafy et al. (Citation2023) proposed a predictive drought severity assessment approach based on the historical vegetation health index and Cellular Automa (CA)-Artificial Neural Network (ANN) algorithms. The study suggests a significant increase in drought severity from 2% in 1996 to 24% by 2031. Nevertheless, although the VHI used can provide valuable information on vegetation health and stress, it has some limitations that may be a source of uncertainty. Indeed, the VHI is a bivariate index that combines normalized vegetation anomalies through the vegetation condition index (VCI) and land surface temperature anomalies through the temperature condition index (TCI). However, the approach based on maximum and minimum value anomalies can be biased, as the extreme values of NDVI and LST are often aggravated by nonclimatic conditions, including sensitivity to cloud cover on multidate images and variability in agricultural practices over the analysis period. To minimize these sources of uncertainty, other researchers have highlighted the value of multivariate drought severity modeling approaches (Han et al. Citation2021a; Tian et al. Citation2022; Ali et al. Citation2022). Recently, using four machine learning models (SVR, RF, XGBR, KNNR), Kafy et al. (Citation2023) proposed the Standard Integrated Drought Index (SIDI) in the Tibetan Plateau by adopting a descriptive drought modeling approach that does not involve the use of anomalies based on maximum and minimum values. Thirteen predictors related to weather, vegetation conditions, soil properties and topographic conditions were used to predict drought conditions with a maximum accuracy of RMSE = 0.11 and MAE = 0.08 with the XGBR model. Nevertheless, despite this relatively high performance, other studies, such as Saha et al. (Citation2023), have suggested that the use of deep learning models performs better in multivariate drought modeling than traditional machine learning models.
Unlike these multivariate composite models, which undoubtedly constitute major scientific advances in the detection, monitoring, and classification of drought intensity, in this study, we propose a new approach that integrates for the first time a new variable related to the principles of rainfall efficiency. To our knowledge, no multivariate composite model in the previous study has yet explored the integration of variables derived from rainfall efficiency. The hypothesis behind our approach is to believe that rainfall efficiency is a function that is both climatic, environmental and footprints of previous droughts. Therefore, considering new variables in addition to the variables commonly used in multivariate drought modeling should improve the quantitative estimation and quality of agricultural drought parameters, particularly under Sahelian conditions. At the same time, it must be noted that very few previous studies have exploited the historical time series of products over forty years provided by the NOAA AVHRR and VIIRS satellites. Most multivariate composite models for drought monitoring rely on data from the latest series of sensors with medium spatial resolution sensors, including MODIS and climate model data. However, in addition to accurately estimating drought intensity, information on the climatic dynamics of drought parameters is essential to understand future dynamics, particularly in the highly heterogeneous agrosystems of the central Sahel and climate sensitivity to seasonal rainfall variability.
In the Central Sahel region, the footprint of local forcings, as well as the cumulative multifactorial impacts of previous droughts and climate change, have significantly exacerbated the sensitivity of natural and anthropogenic units (Balhane et al. Citation2022). Since the 1980s, the agroecosystems of the central Sahel have experienced a complex environmental dynamic that is very contrasted and irreversible in places. Extreme climatic hazards such as prolonged dry sequences are the main drivers of this dynamic. By greatly affecting crop and agricultural production, severe drought sequences are the leading cause of food insecurity in sub-Saharan Africa (Loulli and Hadjimitsis Citation2018; Nooni et al. Citation2021). The development of effective tools to monitor, assess and provide relevant early warning information on agricultural drought is a crucial imperative to address the multiple challenges that hinder the development of this part of the world. In the literature, three approaches are commonly used in the development of composite indices for monitoring and assessing agricultural drought conditions. These include composite models developed based on local knowledge (expert opinion), (Bijaber et al. Citation2018; Bezdan et al. Citation2019; Kulkarni et al. Citation2020), composite models built on purely statistical approaches (Hao et al. Citation2015; Liu et al. Citation2020; Kulkarni et al. Citation2020; Chen et al. Citation2020; Wang and Yu Citation2021; Le et al. Citation2021; Kim et al. Citation2021; Ali et al. Citation2022) and very recently models based on ML algorithms (Park et al. Citation2019; Han et al. Citation20121a; Son et al. Citation2021;Hanadé et al. 2022b; Bageshree et al. Citation2022). The development of robust tools for accurate drought monitoring and early warning has been a remarkable success in several regions of the world (Cammalleri et al. Citation2021; Sultana et al. Citation2021). The current development of new AI and multisensor remote sensing technologies offers a very favorable framework to meet this challenge in any geographical region.
In the Sahel region, many previous studies have been conducted on drought (Leroux et al. Citation2019; Noureldeen et al. Citation2020; Chen et al. Citation2020; Nooni et al. Citation2021; Aiyelokun et al. Citation2021; Abdourahamane et al. Citation2022). They are, however, essentially approaches devoted to the characterization of the spatiotemporal dynamics of drought based on the use of time series of weather stations (Lebel and Ali 2009; Chen et al. Citation2020; Nooni et al. Citation2021). Studies on multivariate descriptive modeling of drought conditions are very marginal (Petersen Citation2018; Fall et al. Citation2021). According to Adedeji et al. (Citation2020), existing drought monitoring mechanisms in the sub-Saharan African region depend mainly on conventional methods. The joint use of climate indices and remote sensing is still a good perspective (Noureldeen et al. Citation2020; Nooni et al. Citation2021), but the assessment and classification of drought parameters by machine learning techniques are very limited. Factual and technologically contemporary studies are therefore needed for better quantification of the drought parameters that periodically plague Sahelian agroecosystems. In other parts of the world, such as South Asia, to account for the impacts of drought sequences at the scale of smallholder farmers, Neeti et al. (Citation2021) recently developed the effective weather drought index (EMDI). EMDI is an integrated, multisensor approach based on the random forest algorithm for CHIRPS downscaling data.
In the same year, in northern Punjab, Pakistan, Qaiser et al. (Citation2021) developed the composite drought index (CDI), which is particularly suitable for monitoring agrometeorological drought in rainfed crop areas. Similarly, Son et al. (Citation2021) proposed the vector drought projection index (VPID) in the US CONUS based on a correlation approach between dependent and nondependent variables. The VPID has a very high correlation with the US Drought Monitor but has not yet been validated with commonly used remote sensing indicators such as VCI, TCI, VHI, or NVSWI. In northern China, Wu et al. (Citation2021) proposed a modeled real evapotranspiration approach for monitoring agricultural drought. The evapotranspiration deficit index (ETDI) was found to be more consistent with the composite agricultural yield reduction index than drought indices such as the SPI and PDSI. In addition to remote sensing data, other approaches use climate model simulation data in the construction of agricultural drought monitoring indices. In this regard, Han et al. (Citation2019) recently developed the standardized soil moisture index (SSMI) based on root soil moisture data from the Global Land Data Assimilation System (GLDAS). The SSMI captured erasing the spatiotemporal dynamics of agricultural drought in the Loess Plateau. In South Korea, Sur et al. (Citation2019) developed the Agricultural Dry Condition Index (ADCI) by combining the VCI, TCI, and Soil Water Saturation Index (WSIS). It should be noted that the ADCI is a rare composite model whose formulation does not include precipitation.
In addition to these approaches, drought monitoring models based on deep learning techniques have emerged around the world, suggesting that deep learning would be effective in exploring the complex relationships of drought variables (Feng et al. Citation2019; Liu et al. Citation2020; Saha et al. Citation2021; Han et al. Citation2021a). For example, Shen et al. (Citation2019) developed a drought monitoring model in Henan Province, China, using data from multisource remote sensing and a deep learning algorithm. The model has a significant correlation with soil relative humidity at a depth of 10 cm. Such a relationship is particularly useful for monitoring drought stress, especially in areas where agriculture is highly dependent on rainfall. Therefore, certain measures to adapt to water stress, such as complementary irrigation, can be recommended based on a mapping of the prevalence of stress. In another study, Liu et al. (Citation2020) proposed an integrated agricultural drought index (IDI) strongly correlated with soil moisture in situ. IDI is an approach based on the backpropagation neural network and multisource remote sensing and seems better suited for monitoring agricultural drought parameters due to the better consideration of the effect of NDVI shift and precipitation. However, the statistical relationship between the IDI and yield anomalies has not been established to better assess the performance of the IDI.
In the Sahel, in the Nigerien context, Mansour Badamassi et al. (Citation2020) developed the Agricultural Drought Index (ADCI) from an approach combining four commonly used drought indices (VCI, TCI, ETCI, PCI). ADCI is a principal component analysis (PCA)-based approach to assigning weights to each variable, and its applicability has been tested on millet yields in southern Niger. However, the statistical relationship of ADCI and VHI is not very high (R = 0.62) compared to other models found in the literature. For example, the ADCI developed by Sur et al. (Citation2019) in South Korea recorded a correlation of 0.8 with potato and soybean yields. These differences in correlative performance could stem from the difference in approaches used to the choice and weighting of variables. Currently, learning techniques can be used to achieve much better performance, thus allowing an accurate assessment of drought conditions in the Sahelian context. Recent studies that have used ML algorithms for variable weighting have resulted in significantly higher performance. Recently, it has been shown that intelligent data-driven models can, in addition to establishing nonlinear relationships between variables, be effective in predicting the short-term impacts of an expected or developing drought (Sutanto et al. Citation2020; Han et al. Citation2021a). However, the ability to detect drought parameters depends greatly on the availability of historical multisource data on impacts. Therefore, it should be noted that accurate, reliable, and operational drought monitoring applications still require additional efforts. The desired compromise on early warning, particularly at the local level, has not yet been reached. The latter should include both high spatial and temporal resolution monitoring and accurate quantification of all drought parameters.
Thus, it can be seen in the current literature on agricultural drought intensity mapping that apart from anomalies in commonly used biophysical and/or climatic variables, previous and/or environmental conditions are rarely taken. Past and cumulative impacts of historical droughts are often omitted from the construction of drought assessment indices. This can lead to an overestimation or underestimation of the intensity of agricultural drought, particularly in climate-sensitive agrosystems. Evidence-based studies are therefore needed to explore the cause-and-effect interconnections of meteorological, biophysical, environmental, and anthropogenic variables. The joint use of environmental condition indices with climate and biophysical indices is one of the avenues to be explored to improve the performance of multivariate models for descriptive assessment of agricultural drought conditions because the specific sensitivity of an agrosystem to drought is a function of the dynamics of previous conditions and present conditions. For example, Jiao et al. (Citation2021) demonstrated that postdrought recovery is strongly influenced by the duration, frequency, and intensity of previous droughts, postdrought moisture conditions and the bioclimatic setting. In most composite models proposed in the literature, anomalies in biophysical (vegetation, moisture, soil temperature) and climatic (precipitation, evapotranspiration) parameters are aggregated as simple indices without the anomalies of bivariate relationships between them being included in the multivariate configuration of agricultural drought assessment models. Variances in bivariate relationships between biophysical and climatic parameters may be an ideal marker for accounting for the footprints of past conditions in drought assessments. For example, anomalies in the linear relationship between vegetation conditions and precipitation anomalies known as rainfall efficiency would be potentially representative of direct and past impacts of water stress better than precipitation and/or NDVI individually. Rainfall efficiency is one of the most important environmental factors in highlighting the productive potential of precipitation in ecosystems where rainfall is the only source of water supply (San Emeterio et al. Citation2012). The addition of this variable to descriptive modeling of agricultural drought could reduce uncertainties associated with a spatiotemporal misdistribution of precipitation intensity and concentration during the rainfed crop growing season.
In this study, in addition to the simple indices traditionally used in multivariate drought modeling, based on the history of drought impacts, we tested the addition of a new biophysical variable that relies on the principle of rainfall efficiency to develop a multivariate composite model for agricultural drought monitoring. Our basic assumption is that precipitation efficiency is a variable that highlights the efficiency of precipitation in ecosystems where precipitation is the only source of water. Therefore, integrating it into the configuration of the multivariate model should allow a better quantification of agricultural drought parameters and an objective classification of drought severity. Thus, the research questions underlying this approach include the following:
Will the addition of standardized rainfall efficiency anomalies have a beneficial effect on the performance of the new multivariate agricultural drought assessment model?
Does the use of unsupervised learning techniques with random forest allow a better use of the history of cumulative and/or delayed impacts of previous droughts in estimating the weights of the components of the multivariate model?
Does the historical dynamics of the agricultural drought parameters (start, duration, intensity, and cessation) of the new multivariate model corroborate with those of the other drought indices (VHI, NVSWI, SPI, PDSI).
There is a statistically significant relationship between the new multivariate drought monitoring model (MDCI) and climate water deficit (CWD).
2. Materials and methods
2.1. Study area
The Central Sahel River Basin is a segment of the Niger River Basin covering three countries: Niger, Mali, and Burkina Faso (). It extends from its topographical limits on the borders of the Sahara in the north (Algeria) to the south, where the climatic conditions are humid tropical. The study area is located between 10°57'5.40″N and 17° 5'14.76″N latitude and 1°59'4.34″W and 6° 0'31.33″E longitude. It was chosen because it is one of the main cereal production areas in West Africa. However, in the current context of insecurity linked to terrorism in this so-called ‘three-border’ zone, there is no possibility of collecting field information. The objective is therefore to evaluate the seasonal productivity of agrosystems in this insecure area using only multisource remote sensing data without any ground truth. This watershed is inactive on its topographical boundaries that extend to Algeria by the fossil networks of the Azaouagh. It drains 340,723 km2, but the active and exploited part of the basin remains very small compared to its geographical extent. It fully covers the two administrative regions of southwest Niger, part of the northern regions of Mali and Burkina Faso. The climatic conditions of the basin are between the Saharan and Sudanese domains which are most often considered the hotspots of global climate influence on several scales. It records a well-contrasting alternation between a short-wet season under the influence of the ITCZ and a long dry season under the influence of the trade winds. Further north, the Sahelo-Saharan zone records an annual rainfall of between 150 and 250 mm, the typical Sahelian zone in the central zone of the basin reaches 250 to 500 mm/year, and the Sahelo-Sudanian zone further south reaches 500 to 750 mm/year. Closely linked to the very gradual south–north bioclimatic transition, the dominant vegetation is a steppe and herbaceous savannah dotted with trees in the south and shrubs in the north.
2.2. Characteristics of production systems
The study area is very diverse in terms of agro-climatic conditions. It is mainly made up of three types of agrosystems: hyper arid agrosystems in the extreme north of the basin (rainfall <100 mm/year), pastoral agrosystems within the limit of 100 to 350 mm and agricultural production systems essentially in the southern part of the watershed, which records rainfall between 350 and 1000 mm/year. The agricultural production system is essentially rainfed (approximately 90%) and is characterized by a low technological capacity with only 4% of land irrigated, which limits the climatic resilience of the production systems (Sanou 2002). Productivity per hectare is one of the lowest in the world, approximately 1179 kg/ha compared to 6 to 12 tons in Asia and Europe (https://reca-niger.org/spip.php?article142). shows the updated spatial distribution of production systems and the main land use and land cover units. The red color of the map corresponds to production systems with supplementary irrigation. This production system clearly occupies a very small part of the watershed. The different levels of intensity of the green color correspond to rainfed production systems that are very dominant compared to semi-irrigated systems. This includes the main rainfed crop species, which are cereals (millet, sorghum, fonio and maize) and the dominant cash crops (cowpeas, groundnuts, voadzou and sesame).
2.2. Data sources
2.2.1. NOAA and VIIRS sensor image data
To develop the new multivariate index over a forty-year period, the NOAA experimental database was used to assess the spatiotemporal dynamics of agricultural drought parameters in central Sahel agrosystems. This historical database is obtained from the https://www.ncei.noaa.gov/ website. It consists of the restated data series (2019 version) of the noise-standardized difference vegetation index (SMN), noise brightness temperature (SMT), vegetation condition index (VCI), state of temperature index (TCI), and vegetation health index (VHI) of the AVHRR sensor (1981-2012) and VIIRS (since 2013). The database was acquired at a resolution of 4 km with a temporality of 7 days and is available in three formats (Geotiff, HDF, and NetCDF). We used the NDVI MODIS 250 m resolution grid to resample all products at 250 m resolution. Note that this historical database is being tested. Few case studies have evaluated the quality of multisensor time series products in the Sahelian zone. In this respect, our reference time series are therefore the products and indices of the MODIS sensors (2002 to 2003) used for the purpose of comparison or resampling at an average spatial resolution of 250 m.
2.2.2. CHIRPS (InfraRed precipitation with station data)
The meteorological component of the approach used was represented by CHIRPS satellite precipitation uploaded to the FEWSNET/USAID website. The monthly rainfall database was acquired for the period 1981 to 2021. This product integrates thermal infrared satellite estimates at a resolution of 0.05° and in situ observations to create a precipitation time series for trend analysis and seasonal drought monitoring. In a context marked by the exacerbation of the demand for atmospheric water due to climate change, rainfall remains the most important factor in the development of drought, particularly in the semiarid tropical agrosystems of the central Sahel.
2.2.3. MODIS sensor data (2002_2003)
To make a comparison and assess the quality of the multisensor time series, data from the MODIS NDVI and LST sensors were downloaded https://lpdaac.usgs.gov/products/mcd12q1v006/ for the period 2002 and 2021. The MODIS sensor offers the ability to track changes in vegetation water stress and anomalies in ground surface temperature at a spatial resolution of 250 m, which is much better than the NOAA and VIIRS sensors. However, the advantage of the latter is that it offers the possibility of mapping the history of drought parameters over forty years and therefore a sufficient and necessary database for predictive modeling ().
Table 1. Data characteristics and biophysical index.
2.2.4. Reference data
In this study, two types of reference data were used for the development and validation of the new multivariate index for agricultural drought monitoring. These are annual cereal yield data covering the period 1981 to 2018 acquired from the FAO website. These data were normalized according to Equationequation 9(9)
(9) to generate a reference variable that represents the interannual impact of drought. Then, this variable related to the variability of agricultural yield anomalies was considered as a response variable to determine the relative importance of each variable using a random forest model. The second set of reference data is the monthly and annual rainfall time series from two meteorological stations in the watershed (Niamey and Dori) that were used to calculate the SPI for the final model validation (Equationequation 12
(12)
(12) ). These data cover the period 1981 to 2021 and are available on the NASA website: https://power.larc.nasa.gov/docs/methodology/. In addition, historical time series of seasonal averages of the Palmer drought severity index (PDSI) and climatic water deficit from the TerraClimate database were acquired on the Google Earth engine platform to refine the evaluation statistics of the MDCI_RF model. This database is open access and can be accessed via the link https://developers.google.com/earthengine/datasets/catalog/IDAHO_EPSCOR_TERRACLIMATE.
2.2.5. Crop zone mask and agricultural calendar
Drought in the agronomic sense is to be distinguished from meteorological or hydrological drought. The impacts of drought factors, in particular the rainfall deficit, on crop growth and productivity are mainly linked to the spatiotemporal distribution of drought factors throughout the phenological cycle of crops. Even when rainfall is at the turn of the climatic average, its poor distribution in time and space is the most increased risk factor, especially in agrosystems where rainfall is the only source of water supply. Therefore, the scale of analysis of the dynamics of agricultural drought parameters must consider the agricultural calendar of rainfed crops. In this study, since we are particularly interested in agricultural drought, a seasonal crop mask was applied to extract the pixel values of the agricultural areas for statistical analysis. The seasonal crop mask was taken from the website https://www.mapspam.info/data/.
2.3. Method
In this study, the parameters of agricultural drought (occurrence, intensity, duration, and severity) are obtained by the objective combination of anomalies in the parameters of vegetative stress (VCI, PV, SVCI), heat stress (SMT, TCI, STCI), climate stress (PCI, RDI, IDP) and an indicator related to environmental conditions (RUE, NRUE). The objective combination of explanatory parameters assumes an approach based on the drought impact variable and the selection of the most relevant variables in the final model. In this study, the impact variable considered is the anomalies of cereal yields at the national level, and highly correlated redundant variables were not considered in the final model.
2.3.1. Processing steps
After the acquisition of the database of different variables, the processing chain includes four steps. The first step is the cleaning of the missing pixels on each image. The number of missing pixels is likely to affect the reliability of the results. Thus, this step aims to clean up the missing pixels (NoData) and replace the average values of the neighboring pixels by using on ArcGIS 10.4.1 the functions Con(IsNull(‘raster’), FocalStatistics(‘raster’, NbrRectangle(5,5, ‘CELL’), ‘MEAN’), ‘raster’) in ArcGIS 10.4.1. For Blended-VHP from NOAA/AVHRR and VIIRS sensors, the missing pixels correspond to the numbers −9999, and this step resulted in rasters without missing values. More information on this step is available at https://support.esri.com/en/technical-article/000004792. The second step is the reconstruction of the seasonal time series from 1981-2021 considering the availability of 7-day images between May and September of each year. This step allows applying the Combine function to switch from multidate images to a single image that corresponds to the pixel average values of the number of available images. The third step of the methodology consists of a resampling of the time series images to 250 m. To do this, all the images were resampled using the resample function of ArcGIS considering as reference the pixel grid of the NDVI MODIS, which is 250 m. In the fourth processing step, the per-pixel statistical zonal function was applied to the raster bricks to extract the Max, Min, STD, and Mean values on each image series to calculate the anomalies of the biophysical variables (univariate indices). These will be considered as a new database on which the variable relative importance assessment described in the next section was made.
2.3.2. Identification of potential variables (model input)
To determine the relative importance of the variables, 11 explanatory factors derived from multisensor remote sensing were calculated and compared to retain the most important variables in the configuration of the final model. To achieve this, after calculating the different indices, the average values of the seasonal pixels were extracted. The pixel values were extracted under the environment of the ENVI image processing software by applying the crop mask. This made it possible to build an Excel table with 12 columns including the reference variable, which is the anomaly of agricultural yields. This table was later used as input to the random forest algorithm in R 4.3.0 software for prioritizing relationships between covariates and crop yield anomalies. The calculations of the different indices and the description of random forest algorithm are presented in the following sections.
2.3.2.1. Parameters related to climate stress (IDP, PCI, NRDI)
Water stress related to a deficit of precipitation, or its poor spatiotemporal distribution can be obtained through several indices based on the deviation from the maximum, minimum, or standard deviation values and the average of the climate series. In this study, the precipitation concentration index (PCI), Equationequation (2)(2)
(2) ; the precipitation deficit index (IDP), Equationequation (3)
(3)
(3) ; and the normalized rainfall deficit index (NRDI), Equationequation (1)
(1)
(1) , were calculated and compared to identify the best indices that would represent climate stress anomalies.
(1)
(1)
(2)
(2)
(3)
(3)
IDP: Rainfall deficit index (in percentage); Pi: annual precipitation (in mm); Pm: average precipitation (in mm)
2.3.2.2. Abnormalities in parameters related to vegetative stress (VCI, PV, SVCI)
Several biophysical indicators related to vegetation are representative of vegetation stress conditions. In this study, we explored the relevance of four vegetation stress indicators: the proportion of vegetation (Equationequation 4(4)
(4) ), the SVCI scaled vegetation condition index derived from smoothed NDVI (Equationequation 5
(5)
(5) ), the noise-standardized difference vegetation index (SMN), and the Vegetation Condition Index (VCI) at the origin of the work of (Kogan Citation1990). PV is an index that determines the proportion of vegetation cover originally proposed by Yu et al. (Citation2014) as a necessary variable for estimating LST from Landsat thermal bands. The SVCI is indeed the same as the VCI proposed by Kogan (Citation1990), and the only difference is that it was calculated using the smoothed NDVI (SMN). Therefore, to differentiate the two indices, we added the term scaled.
(4)
(4)
PV is the proportion of vegetation derived from NDVI (smoothed).
(5)
(5)
SVCI is a vegetation condition index derived from smoothed NDVI (SMN).
SMN (i), SMNmax, and NSMN min are the pixel values of SMN and its maximum and minimum, respectively, in the time series considered.
2.3.2.3. Heat stress-related parameters (TCI, STCI, SMT)
The temperature condition index (TCI) led to Kogan’s work at NOAA in 1995 in the United States. It is an indicator that is based on the temperature of brightness and is applicable at the local, regional, or continental level, instantaneously or over periods of up to a year (Mohammed Citation2008). The index determines the stress that temperature and excessive humidity due to soil saturation with water cause to vegetation (Kogan, Citation1997). The conditions are estimated in relation to the maximum and minimum temperatures and are adapted according to the different reactions of the vegetation to the temperature. In this study, in addition to the corrective treatments applied to the NOAA sensor’s TCI product, the scaled temperature conditions index (STCI) was calculated from the noise-free brightness temperature (SMT), Equationequation (6)(6)
(6) . The STCI is indeed the same as the TCI proposed by Kogan (Citation1997); the only difference is that it was calculated using the smoothed LST (SMT). Thus, to differentiate the two indices, the term scaled has been added.
(6)
(6)
STCI(i), SMTmax and SMTmin are the pixel values of SMT and its maximum and minimum, respectively, in the time series considered.
2.3.2.4. Parameters related to rain use efficiency (RUE)
The effectiveness of rainfall is one of the most important environmental factors that makes it possible to highlight the efficiency of rainfall, especially in environmental conditions where rainfall is the only source of water supply. Taking this variable into account in descriptive drought modeling aims to reduce the influence of the poor spatiotemporal distribution of rainfall intensity and concentration during the crop growth period. In the context of the central Sahel, the beneficial effect of cumulative rainfall on agrosystem productivity is highly dependent on a good distribution of rainfall over the five months of the agricultural calendar. This variant of rainfall is particularly significant in the context of the Sahel, where the complementary irrigation system is poorly developed or even nonexistent in places. RUE (rain use efficiency) is an indicator of rain efficiency. It was originally calculated by considering the ratio of net primary production to precipitation. In this study, we considered the ratio of the vegetation condition index (VCI) and precipitation to estimate the RUE. NRUE (normalized rain use efficiency) is an indicator that represents the normalized form of the RUE index (Equationequation 7(7)
(7) ). It was developed in this study to represent the sensitivity of agrosystems to the cumulative impacts of past droughts.
(7)
(7)
NRUE is the Normalized Rainfall Efficiency Index, and Max and Min are the extreme values of the RUE pixels.
2.3.3. Relative importance of relevant variables using random forest algorithm
Estimating the importance of each variable in the composite model is a key step in the multivariate modeling of drought parameters. Variable-importance evaluation functions that use model information have the advantage of using a model-based approach and may be able to incorporate the correlation structure between predictors in calculating the importance of explanatory variables. In this category, ML models based on decision trees have the advantage that they are less sensitive to order relationships in training data. For this reason, based on the approach used by Han et al. (Citation2019) for the development of the combined drought monitoring index (CDMI) model, the random forest algorithm was used to determine the relative importance of the components of the MDCI model. Random forest is one of the ML algorithms based on decision trees particularly effective for identifying the links between a variable to be explained and explanatory variables. It classifies the explanatory variables according to their relationship to the variable to be explained by creating several decision trees and then combines the output generated by each of the decision trees. The most common result for each observation is used to determine the relative importance of each variable (). It was chosen because its performance is relatively less sensitive to low input datasets and missing data (Zhou et al. Citation2016; Shaikhina et al. Citation2019; Li and Xu Citation2021). By aggregating the results of many trees, it can improve its accuracy and reduce the risk of overfitting, even when it comes to small data sets. The model calculates the importance of the impact of each variable on the errors of the predictions and thus determines the weights of each variable. In our case, interannual variability in cereal yield anomalies was considered as the target variable and PCI, TCI, VCI, SMN and NRUE as explanatory variables. The weights obtained were compared with those obtained on the correlative analysis of the variables. The random forest package used is available via http://mehdikhaneboubi.free.fr/random_forest_r.html. It is a semi-supervised machine learning model that uses only a quantitative data as input and does not involve the use of labeled data or the separation of data into training, calibration, and validation samples. The model fit parameters and model evaluation indicators are presented in the following section.
For the three weighting cases, all weights of the relative importance of the variables were normalized to the same scale (0 to 1), Equationequations (8)(8)
(8) .
(8)
(8)
4.3.4. Model performance evaluation
The performance of random forest was evaluated using the mean square residual (MSE) of the out-of-bag errors (OOB) using the smallest value to select the optimal model. Out-of-bag errors or test error is a method of measuring the prediction error of random forests. The goal is to obtain the lowest OOB by adjusting the model parameters, the number of trees (ntree) and the number of variables tested at each division (mtry). The smallest MSE value was obtained with random forest using parameters ntree= 2000 and mtry = 2. presents the MSEs, and the variance explained by each variable in the random forest model.
Table 2. Performance measures of the random forest model.
2.3.5. Yield anomaly
The impacts of extreme droughts can significantly affect cereal productivity in areas where rainfall is the only source of water supply for crops. For this purpose, agricultural yield anomalies are often used in the development and/or validation of drought indices (Patel and Yadav Citation2015; Anderson et al. Citation2016; Hendrawan et al. Citation2022). However, to minimize the effect of several factors that influence agricultural productivity, farm yield data were standardized according to the following formula (9):
(9)
(9)
where Ya is the yield anomaly, Ymin is the minimum value in the series, and Ymax is the maximum in the series.
2.3.6. Reference indices for the validation of the MDCI composite model
To validate the multivariate composite model developed for agricultural drought monitoring, the vegetation health index (VHI) and the normalized vegetation supply water index (NVSWI) were used. The VHI and the NVSWI are the two best-known indices of agricultural drought and are validated in many climatic zones. The Normalized Vegetation Water Supply Index (NVSWI) is calculated as follows:
(10)
(10)
NDVI and LST of the same month or year, VSWImin, and max are the maximum and minimum values of the time series.
The VHI used in this study for validation is the mixed vegetation health product (official mixed VHP), covering the period 1981 to 2021. It is a multisensor set of retired vegetation health data derived from VIIRS (2013-present) and AVHRR (1981-2012) data. Although the product has been improved over the GVI-x VH system, the operations of pixel outlier corrections, normalization (0 to 1), re-enticing at 250 m, and statistical zonal correction have been applied to VHP products. Seasonal and monthly VHI averages were calculated using the available 7-day image collections. In addition, VHI was calculated from TCI and VCI biophysical indices (Equationequation 11(11)
(11) ).
(11)
(11)
In this equation, the VHI of month (i) or year (i) is equal to the sum of VCI (i) *0.5 + TCI (i)* 0.5 at the time scale considered.
2.3.7. Concordance of MDCI vs. PCA, VHI and NVSWI time trend magnitudes
In addition to the statistical and cartographic comparison, the per-pixel temporal trend regression of the multivariate composite model was compared to the pixel temporal trend regressions of the principal component analysis, VHI, and NVISWI over the period 1981 to 2021. Principal component analysis was applied to three simple indices: the VCI, TCI, and NRUE. shows the main steps in the study methodology.
To classify the different drought intensity levels of the MDCI model, we adapted the classification threshold that was used by Han et al. (Citation2019) in China for the CDMI model. These intensity thresholds used for the cartographic comparison of reference drought indices (VHI, NVSWI) and the MDCI model are provided in .
Table 3. Classification of MDCI and the reference remote sensing drought indices.
2.3.8. Validation by the standardized precipitation index (SPI)
Developed by researchers at the University of Colorado (McKee et al. Citation1993), the SPI is a versatile index that can be calculated on any time scale and quantifies the deviation of a period’s precipitation, deficit or surplus, from the historical average precipitation of the period. In this study, the annual SPI was used from the time series of data from two metrological stations. These are the station of Niamey airport (13.48°N | 2.17°E, 223 m altitude) and the station of Dori in northern Burkina Fasso (14.03°N | 0.03°W, 276 m altitude). These two stations are spatially well distributed in the watershed; the Niamey station is slightly in the center of the basin, and the Dori station is slightly in the wateriest southwestern part of the watershed. SPI has been widely used as a benchmark in the development and validation of composite indices (Sepulcre-Canto et al. Citation2012; Alfred and Jürgen Citation2015; Bayissa et al. Citation2019). It is calculated by the formula:
(12)
(12)
where P is the total precipitation of a period (mm); Pm is the historical mean precipitation of the period (mm); and σP is the historical standard deviation of precipitation of the period (mm).
2.3.9. Validation by Palmer drought severity index (PDSI)
The PDSI is an M, Wagdy severity index widely used to monitor different types of droughts (Yan et al. Citation2016; Wu et al. Citation2021; Jiang et al. Citation2021; Du et al. Citation2022). It was developed by Palmer in 1965. The PDSI uses monthly data on temperature, precipitation and soil water holding capacity as input parameters. Given the information on rainfall and temperature, the PDSI is a more comprehensive index than the SPI for assessing agricultural drought (Huang et al. Citation2015). The index is standardized to be comparable between different climatic zones (Mishra and Singh Citation2010). The severity of drought is provided ranging from −10 (dry) to +10 (wet). In this study, historical records of the PDSI of TerraClimate data were used to assess its static relationship with the developed MDCI model.
2.3.10. Validation by climatic water deficit index (CWDI)
In addition to validation by SPI, PDSI and yield anomalies, the Climate Water Deficit Index (CWDI) was also used to assess MDCI performance. CWDI is a measure of potential evapotranspiration minus actual evapotranspiration and integrates climate, energy load, drainage, and soil moisture changes into a single variable (Flint et al. Citation2014). It is therefore an integrated variable that can provide information on local climate stress (https://www.aquaportail.com/definition-13134-deficit-hydrique.html) conditions. For this reason, we considered the CWDI from the one-dimensional soil water balance model of the TerraClimate database (Abatzoglou et al. Citation2018) to establish the statistical relationship between the CWDI and MDCI.
2.3.11. Statistical models and results visualization
In addition to comparative mapping, Pearson’s parametric correlation test (equation) and relationship significance test were used to evaluate the performance of the MDCI_RF model. For this purpose, the values of the seasonal variability of the RF MDCI_ and the validation indices (SPI, PDSI, CWDI, VHI and NVSWI) were considered to establish the statistical analyses. The Pearson correlation coefficient is one of the very popular statistical metrics in the evaluation of statistical relationships of spatiotemporal variability of drought indices (Jiang et al. Citation2015; Zhao et al. Citation2022). In this study, correlation matrices including regression lines, Pearson parametric correlation test and p value significance test were run automatically in the R environment version 4.2.0 using the library(ggpubr) and library(‘PerformanceAnalytics’). More information is accessible via the http://www.sthda.com/english/wiki/correlation-matrix-a-quick-start-guide-to-analyze-format-and-visualize-a-correlation-matrix-using-r-software site. Similarly, using R software, the Raster Library was used to generate and compare the pixel trend magnitudes of the reference indices and those of the developed MDCI_RF model. The p value per pixel was calculated to hide the values > 0.05 and achieve a 95% confidence level. Thus, only pixels of significant trends were considered for comparison of trend magnitudes. This method is inspired by the work of Brandt et al. (Citation2014), who applied temporal regression on NDVI and FAPAR time series to assess changes in local vegetation trends in Sahelian ecosystems https://matinbrandt.wordpress.com/2013/11/15/pixel-wise-time-series-trend-anaylsis-with-ndvi-gimms-and-r/. For the comparative visualization of the distribution of index densities (), the Seaborn 0.12.2 library was used. Seaborn is a Python library for statistical data visualization based on matplotlib. The notebook used for this analysis can be accessed via the following link https://seaborn.pydata.org/generated/seaborn.pairplot.html.
where n is the series number and xi and yi are the values of series x and y, respectively. x̅ and y̅ represent the average values of the respective series.
3. Results
3.1. False break of multisensor time series
highlights the disadvantage of the multisensor time series used in this study and the opportunities for effective improvement. At first glance, a break in the series has been observed since 2013. However, an advanced diagnosis has made it possible to understand that this break in the series is closely related to the difference in the data qualities of the NOAA sensor (1981 to 2012) and the VIIRS sensor (2013 to 2021). Such a result is very valuable given that the database used is being tested. Future studies can build on such results to understand the disadvantages of multisensor time series. The corrected result is shown in .
3.2. Analysis of the monthly distribution of VHI, TCI, VCI, and SMN
The comparative analysis of the clusters of the distribution of monthly index values (VHI, TCI, VCI) shows little difference for the first three months of the agricultural season except for the smoothed vegetation index (SMN). The SMN indicates a well-contrasted distribution between the months of June, July, and August (). Since the latter is an index based solely on the state of vegetation, this distribution corroborates the NDVI values throughout the agricultural season. Similarly, comparing the distribution of the other indices, it appears that the values of the temperature condition index (TCI) distribution record the lowest class separability for the first three months of crop growth.
3.3. Historical interannual variability of the MDCI_RF multivariate composite model
shows the multivariate mapping of drought conditions obtained by the combination of five drought variables. The proposed multivariate composite model for agricultural drought monitoring (MDCI) traced the history of drought parameters (occurrence, intensity, severity, and cessation). The climatology of drought conditions is provided at six levels of drought intensity. This result shows the irregular succession of both similar normal years and a very long period of drought that began in 1982 until 1989. Since then, the resurgence of the exceptional drought of the same intensity has not been observed in the Central Sahel watershed. This period is characterized by an exceptional intensity of drought and extreme severity considering its magnitude and duration. On the other hand, it should be noted that the succession of wet years in this part of the world hardly exceeds two consecutive years. However, a long series of relatively normal years characterize the last decade. These years are marked by the absence of pockets of exceptional and extreme droughts but a dominance of severe to moderate sequences.
The correspondence between the severity of exceptional droughts and the impact on yields per hectare () shows the ability of the MDCI model to capture the impacts of the exceptional droughts of 1984 and 1987. For these two years, which record the extreme intensity of the drought, the corresponding impacts in terms of reduced yields were −142 kg/ha and −72.6 kg/ha, respectively, compared to the average yield per hectare over 38 years. Similarly, the wettest years according to the MDCI result are characterized by their rainfall surplus compared to the climatic average precipitation. This is the case for 1999, with a surplus of +48.8 mm, and the year 2005, with a surplus of 25.5 mm. In other words, when the rainfall deficit reaches −24 mm/moy, the severe to extreme drought increases. This is the case illustrated by the year 2000.
3.4. Analysis of the frequency distribution of the MDCI_RF
Of the entire multivariate composite model (MDCI) time series, the frequency proportion of wet pixels (>0.5) is the lowest. The proportion of pixels of severe to moderate droughts (0.3 to 5) is most dominant for all years except 1987. 1987 was the year with the most extreme conditions (<0.3). Comparing the frequency distribution of drought intensity in 1985 with conditions in 1981, we can accept the occurrence of very contrasting conditions, both wet and extremely dry. However, similarities and interannual differences in the distribution of wet and dry pixels should be highlighted (). This is the case for the years 19881, 1995, and 96 (wet). Similarly, drought years that are similar in the distribution of dry pixels are easily identifiable (1982 to 1990). This decade is undoubtedly the driest of the series from 1981 to 2021.
3.5. Validation of MDCI by VHI, NVSWI and PCA
3.5.1. Analysis of cartographic correspondences with the VHI
The spatiotemporal mapping of drought conditions by the vegetation health index shown in the figure reflects a high agreement with the mapping result of the multivariate composite model shown in above. Unlike the cartographic result provided by the supply vegetation water index (), which shows an amplifying and contrasting effect of water stress, the spatialization of drought conditions by VHI () corresponds well to that of MDCI. The MDCI is a composite model that incorporates several factors related to the development and worsening of drought conditions, and the VHI is an index that integrates only two components (VCI and TCI). In the same way as the MDCI, the VHI made it possible to highlight the particularly dry conditions that prevailed between 1982 and 1990. However, it should be noted that the VHI was reclassified into six drought intensity classes to make the map visualization comparable to the six intensity levels of the proposed MDCI model.
3.5.2. Analysis of cartographic correspondences with the NVSWI
maps the historical dynamics of the distribution of agricultural drought parameters in the Sahel watershed by the NVSWI index. Although the NVSWI reflects a good temporal concordance with the VHI and MDCI, the spatial distribution of stress conditions by the NVSWI is characterized by a very contrasting amplifying effect. Nevertheless, this spatially contrasting amplifying effect is quite comparable to the cartographic results of the Normalized Rainfall Efficiency Index (NRUE), with a few exceptions (2018 to 2021). However, it should be noted that the statistical correspondence of the NVSWI with VHI and MDCI is significantly better than that of NRUE. On the mapping of NVSWI and NRUE, one can easily see the differentiated grip of extreme drought better than with VHI and MDCI. However, over the past five years, the normalized rainfall efficiency index has put forward a succession of adverse conditions, unlike NVSWI's mapping. This is understandable because NRUE is proposed to reflect the relationship between the vegetation condition index and precipitation (useful precipitation), while NVSWI relies on the relationship between NDVI and land surface temperature.
3.5.3. MDCI_RF vs VHI and NVSWI temporal consistency
shows the temporal evolution of the seasonal average values of the MDCI, VHI, and NVISWI. On this graph, the temporal variability of MDCI accurately reflects the seasonal variability of the most popular agricultural drought indices (VHI and NVSWI). VHI and NVSWI are considered in this study as benchmarks because they are the best-known indices of agricultural drought by remote sensing. The chronological comparison of the latter and the MDCI shows a consistent and highly synchronized interannual variability over a historical period of 40 years. The peaks of the extreme condition convergences with the minimum and maximum values of these three indices, whose low values indicate dry conditions and values above 0.5 reflect the absence of drought. In fact, on this basis, we can see that the period of return of wet years such as 1981, 1991, 1998, 1999 and 2005 is very variable from one decade to the next. The first decade of the time series (1981 to 19990) had only one wet year, while the decade 1990 to 2000 had five wet years.
3.5.4. Statistical validation of the multivariate model for drought monitoring
The multivariate composite model for monitoring drought conditions (MDCI) shows a highly significant statistical relationship with the other indicators (). Its correlation with the VHI vegetation health index is the highest at 0.95 with VHI and a p value < 2.2e-16. Similarly, the MDCI has a correlation of 0.94 and one with the water stress index (NVSWI). However, the correlative relationship between the VHI and NVSWI is 0.89, which is much lower than those between the MDCI and VHI and NVSWI. These statistics illustrate the synchronous chronological evolution mentioned above by the figure of the evolution of temporal coherences. In general, compared to the indices, the MDCI is distinguished by the best correlative compromise with 10 biophysical indicators.
3.5.5. Comparison of MDCI and PCA, NVSWI and VHI trend magnitude
In addition to the dynamic mapping of drought parameters, the magnitude of the trends of significant changes in the reference indices and the PCA were compared with that of the MDCI model over the period 1981 to 2021. Based on the obtained result (), we can see a very significant agreement between the trend magnitude of the MDCI and that of the VHI. Similarly, a very high agreement is observed between the trend magnitude of the PCA and the NVSWI. Overall, on the four maps, the highest agreement is observed for areas marked by trends of little or no significant change (green colors in the legend).
3.5.6. MDCI performance statistics vs. the SPI index, PDSI and CWDI
shows the comparative statistical relationships at the seasonal scale (seven months) between the MDCI_RF model and SPI, PDSI, CWDI, NVSWI, and VHI. The results show that MDCI_RF is statistically highly correlated with the Palmer drought severity index (PDSI) better than with the standardized precipitation index (SPI) and the climate water deficit index (CWDI). However, the correlative relationships of the MDCI_RF with the bivariate biophysical indices (VHI) and NVSWI are better than those with the hydroclimatic indices (SPI, PDSI, CWDI). Similarly, it was observed that the seasonal mean values for MDCI_RF are significantly better correlated with SPI −12 than with SPI and NVSWI. The p values between the MDCI and the SPI of the Niamey station and the Dori station in northern Burkina Faso are statistically very significant. They are between 3.531e-05 and 6.137e-06 with correlations that vary between 0.6 and 0.64. These values are slightly better when considering the average cumulative precipitation of two stations over the period 1981 to 2021. In view of the geographical extent of the basin, this suggests that by considering more stations better distributed throughout the basin, strong correlations are established between the MDCI_RF model and the meteorological drought indices.
3.5.7. Proportional occurrences of drought intensity MDCI vs PCA
The comparative analysis of proportional occurrences of the three levels of drought intensity according to the result of the MDCI model and the PCA () shows a good consistency in the interannual evolution of drought intensity in the watershed. Unquestionably, both results indicate that the decade from 1981 to 1990 is the driest and the last decade is the least dry in the Central Sahel region. Between the two very contrasting periods, the occurrence of moderate drought conditions was largely dominant over the period 1988 to 2010. The proportional occurrence of extreme conditions shows a significant downward trend. This downward trend in the intensity of drought in the central Sahel zone is linked to the increase in rainfall in this zone. Indeed, unlike other regions of Africa, notably East Africa and the Mediterranean basin, in West Africa, several studies have highlighted a factual trend of humidification over the last two decades (San Emeterio et al. Citation2012; Panthou et al. Citation2014; West et al. Citation2017; Chen et al. Citation2020; Porkka et al. Citation2021). Studies such as Biasutti (Citation2019) suggest an increase in rainfall in the central and eastern Sahel and a decrease in the more western regions. These findings corroborate the historical dynamics of agricultural drought parameters extracted from the seasonal variability of the developed MDCI model.
3.5.8. Temporal variability of MDCI models and grain yield anomalies
The interannual variability of the time series of the seasonal mean values of the MDCI models accurately reflects that of the anomalies in yields per hectare over the period 1981 to 2018 (). This result makes it easy to identify the number of wet, deficit, and excess years. Thus, these time series show 13 years in deficit, and unsurprisingly, 1984 corresponds to the minimum peak. There are 17 excess wet years, and the rest of the series are normal years. The other characteristic that should be highlighted is the succession of years that are distinguished by their low variability (1990 to 1997, 1985 and 1986) and years (1998 and 1999).
4. Discussions
Over the past two decades, from multisensor remote sensing and machine learning techniques, several approaches have been devoted to the development of many composite indices. However, it should be noted that most of these composite models have been developed over short periods that do not allow a climate study of drought parameters in the agricultural sense. In general, the composite analyses hitherto established are limited to short time series that cover periods of 10, 15 to 20 years (Feng et al. Citation2019; Guo et al. Citation2019; Ali et al. Citation2022). Studies such as those by Vicente-Serrano et al. (Citation2015) established the possible influence of drought trends on the SPEI index over a relatively long period of time from 1981 to 2011 without a multivariate modeling approach to drought conditions being provided. Drought conditions have been reduced to NDVI anomalies.
Climate and composite studies of agricultural drought by remote sensing remain the only effective way to extract spatiotemporal trends from all drought parameters over large areas. This study proposes a composite and multivariate approach over 40 years to trace the spatiotemporal footprint of historical droughts in the Central Sahel area. Such an approach makes it possible to establish climatic analyses of all drought parameters (occurrence, duration, severity, cessation, and intensity). The approach, therefore, provides a better understanding of the historical dynamics of water stress in the central Sahel area and provides the necessary basis for predictive modeling of agricultural drought parameters. The multivariate composite model for drought monitoring (MDCI) developed has a very high adequacy with the Vegetation Health Index (VHI) and the Crop Water Supply Index (NVSWI). This performance is comparable to that of many other composite models developed, including the composite model developed by Mansour Badamassi et al. (Citation2020) and the composite model for drought monitoring proposed by Han et al. (Citation2019) in Shaanxi Province, China. The correlative relationship of the ADCI with the VHI at the seasonal scale is 0.62, slightly lower than that of the MDCI, and the VHI is 0.95.
This correlative difference can be understood by the fact that MDCI was developed from machine learning models for variable weighting, while ADCI is an approach based on principal component analysis. The other strength of the MDCI is that it incorporates for the first time in its configuration a new index that can incorporate the specific sensitivity of each agrosystem and the cumulative effect of previous droughts called the normalized rainfall efficiency index (NRUE). This index is based on the principle of rainfall efficiency coefficient. The only difference is that in place and instead of NDVI or primary productivity, we tested several combinations of several variables, and the ratio between VCI and precipitation was found to be the most relevant and comparable to other biophysical indices. This approach made it possible to integrate the efficiency of the latter into the model in addition to the precipitation component. The efficiency of rainfall is closely related to previous and environmental conditions. The proposed normalized rainfall efficiency index proved particularly useful in multivariate modeling of agricultural drought parameters with an explanatory score of 23% compared to the climate component of the model, which is 20% by machine learning. Furthermore, it should be noted that the MDCI_RF was statistically validated by considering auxiliary hydroclimatic indices (SPI, PDSI, CWDI) that are not included in the MDCI_RF model development process. The temporal variability of MDCI_RF was very strongly correlated and statistically significant with that of the commonly used remote sensing drought indices VHI and NVSWI (R > 0.9 and p value < 2.2e-16) as well as with the hydroclimatic indices SPI, PDSI, and CDWI with an R between 0.6 and 0.85 and a p value < 5.8e-10. This ensures its reliability as a new multivariate model for agricultural drought monitoring.
In addition, the results of the multivariate mapping of the composite model developed corroborate the results of numerous studies on drought in the Central Sahel region (West et al. Citation2017; Chen et al. Citation2020). The driest decade in the Sahel’s climate history was highlighted, as were the impacts of the memorial droughts of 1984, 1985 and 1987. The composite approach has proven to be very useful in minimizing the effect of differences in data quality due to the use of multisensor time series. However, the MDCI was developed from global low-resolution spatial experimental data. Landsat, MODIS, and sentinel data can be used for the full evaluation of the MDCI model.
5. Limitations and future scope
In this research, a new approach for multivariate modeling of agricultural drought parameters of agrosystems in the central Sahel was proposed. Although it is widely accepted in the scientific literature that multivariate models are better suited to capture the spatiotemporal and multifactorial complexity of drought, we recognize here some limitations and sources of inaccuracies that can affect MDCI_RF performance. The MDCI was built by considering the history of multisensor biophysical variables. This results in several levels of data qualities of the time series of biophysical variables. For example, the MDCI was developed over the period 1981 to 2021, yet the quality of the time series data of the input variables over the period 1981 to 2013 of the NOAA AVHRR sensor was found to be different from that of the last period 2014 to 2021 covered by the VIIRS sensor. Thus, despite the very promising performance of the MDCI model potentially due to the addition of the NRUE variable compared to traditional indices, the quality of the experimental database (NOAA/VIIRS) used seems to have a reducing effect on the actual performance of the MDCI model. Despite data quality improvement treatments and variable normalization, these differences in different data quality levels are a potential source of imprecision. In addition, resampling data at 250 m resolution can add an additional bias to data quality. To assess the effectiveness of the MDCI model, it is necessary to test this approach on better quality data by exploring the contribution of spatiotemporal fusion of MODIS data with Sentinel-2 and Landsat data. Furthermore, it should be noted that the MDCI_RF does not include variables that provide information on the hydrological dimension of drought, namely, subsurface moisture and evapotranspiration information. However, the integration of hydrological drought variables and physiographic sensitivity factors, including the soil quality index and topographic factors, into the current MDCI_RF configuration could be beneficial for improving model performance. In this regard, GRACE's groundwater storage anomaly products and soil moisture from DInSAR (differential interferometric synthetic aperture radar) measurements need to be studied.
In other words, in the development of the MDCI_RF, the contributions (weights) of the different components of the model were calculated in relation to the interannual variability of cereal yields at the national level. However, in addition to climatic water stress, several other factors can affect yields per hectare. To circumvent this limitation, future studies can explore the use of in situ measurements of critical variables such as soil moisture measurements in rainfed agrosystems or climate water deficit to determine the relative contributions of each component of the MDCI_RF model. However, despite these sources of potential uncertainties, the results of the model validation MDCI_RF by several other independent indices, including its statistically significantly removed relationship with the Palmer Severity Index (PDSI), support its performance as a new multivariate index for drought monitoring.
6. Conclusion
This study proposes a multivariate composite index for monitoring agricultural drought. The Multivariate Drought Composite Index (MDCI) has effectively captured the climate dynamics footprint of the parameters (occurrence, duration, intensity, and aggravation) of drought in the central Sahel River basin. The multivariate composite model for drought monitoring (MDCI) developed has very high adequacy with the vegetation health index (VHI) and the normalized vegetation supply water index (NVSWI). The coefficients of determination are R2 = 0.91 and R2 = 0.88, respectively. The validation of the MDCI model shows a statistically significant relationship with the annual SPI. The values of the p value with the SPI are between 3.531e-05 and 6.137e-06 with correlations that vary between 0.6 and 0.64 depending on the station. The statistical relationship between MDCI and SPI-12 is significantly higher than that observed between IPS and VHI and NVSWI. In addition, MDCI_RF was found to be highly correlated with the Palmer drought severity index (PDSI) and climatic water deficit index, with R = 0.85 and p value < 5.8e-10 and R = 0.72 and p value < 1.9e-6, respectively. This suggests that the random forest model used have made it possible to effectively incorporate the historical spatiotemporal variability of anomalies of several factors related to the development and worsening of agricultural drought in the agrosystems of the central Sahel. The results of the index related to environmental conditions developed, were found to be comparable to other biophysical indices with an explanatory score of 23% slightly higher than the climate component of the model (PCI), which stands at 20%. The MDCI and NRUE were developed for the semiarid Sahelian tropical context to test and evaluate the applicability of this approach in other regions with different agroclimatic characteristics.
Abbreviations | ||
CDI | = | Composite Drought Index |
CDMI | = | Composite Drought Monitoring Index |
VIIRS | = | Visible Infrared Imaging Radiometer Suite |
AVHRR | = | Advanced Very High-Resolution Radiometer |
LST | = | Land Surface Temperature |
CHIRPS | = | Climate Hazards Group InfraRed Precipitation with Station data |
VCI | = | Vegetation Condition Index |
TCI | = | Temperature Condition Index |
NDVI | = | Normalized Difference Vegetation Index |
MDI | = | Multivariate Drought Index |
SMDI | = | Soil Moisture Deficit Index |
PCA | = | Principal Component Analysis |
JDI | = | Joint Drought Index |
SSI | = | Standardized Streamflow Index |
CCDI | = | Customized Composite Drought Index |
CADI | = | Comprehensive Agricultural Drought Index |
NOAA | = | National Oceanic and Atmospheric Administration |
PDSI | = | Palmer Drought Severity Index |
ADRI | = | Multivariate Drought Response Index |
MODIS | = | Moderate Resolution Imaging Spectroradiometer |
EMDI | = | Effective Weather Drought Index |
RF | = | Random Forest |
SPEI | = | Standardized Precipitation Drought Evapotranspiration Index |
SPI | = | Standardized Precipitation Index |
VHI | = | Vegetation Health Index |
VSWI | = | Vegetation Supply Water Index |
CDI | = | Composite Drought Index |
VPID | = | Vector Drought Projection Index |
ETDI | = | Evapotranspiration Deficit Index |
SSMI | = | Standardized Soil Moisture Index |
GLDAS | = | Data Assimilation System |
ADCI | = | Agricultural Dry Condition Index |
WSIS | = | Soil Water Saturation Index |
IDI | = | Agricultural Drought Index |
ML | = | Machine Learning |
ITCZ | = | Intertropical Convergence Zone |
SMT | = | Smoothed Brightness Temperature |
SMN | = | Smoothed Normalized Difference Vegetation Index |
FEWSNET | = | Famine Early Warning Systems Network |
USAID | = | US Agency for International Development |
RUE | = | Rain Use Efficiency |
NRUE | = | Normalised Rain Use Efficiency |
IDP | = | Precipitation Deficit Index |
NRDI | = | Normalized Rainfall Deficit Index |
PCI | = | Precipitation Concentration Index |
PV | = | Proportion of Vegetation |
SVCI | = | Vegetation Condition Index |
STCI | = | Scaled Temperature Conditions Index |
MDCI | = | Multivariate Dought Composite Index |
Acknowledgments
We gratefully thank the following research structures: the laboratory of UMR CNRS ESPACE 7300 (AMU France), the laboratory (UR 18) of the Department of Geodesy and Topography (IAV Hassan II), and the Regional Center for Agronomic Research of Marrakech (INRA, Morocco) for their contributions and collaborations in this research. We also thank the Islamic Development Bank for its financial support (scholarship).
Data availability statement
The data that support the findings of this study are available from the corresponding author, [IHH], upon reasonable request. They were however hosted on the Aix Marseille University platform and accessible via the https://filesender.renater.fr/?s=download&token=132c94c6-1cad-40a1-80ba-3ae274e3d3ab. After the publication of the manuscript the database of the final results will be made available in open access on ZENODO. The raw data is also available on the site: https://www.ncei.noaa.gov/.
Disclosure statement
All authors contributed to the production of the manuscript and declare no conflict of interest.
Additional information
Funding
References
- Abatzoglou JT, Dobrowski SZ, Parks SA, Hegewisch KC. 2018. Terraclimate, a high-resolution global dataset of monthly climate and climatic water balance from 1958-2015. Sci Data. 5:170191. doi:10.1038/sdata.2017.191.
- Abdourahamane ZS, Garba I, Boukary AG, Mirzabaev A. 2022. Spatiotemporal characterization of agricultural drought in the Sahel region using a composite drought index. J Arid Environ. 204:104789. doi:10.1016/j.jaridenv.2022.104789.
- Adedeji O, Olusola A, James G, Shaba HA, Orimoloye IR, Singh SK, Adelabu S, 2020. Early warning systems development for agricultural drought assessment in Nigeria. Environ Monit Assess. 192:1–21.
- Aiyelokun O, Ogunsanwo G, Ojelabi A, Agbede O. 2021. Gaussian Naïve Bayes Classification Algorithm for Drought and Flood Risk Reduction. In: deo R. Samui P. Kisi O. Yaseen Z. (eds) Intelligent Data Analytics for Decision-Support Systems in Hazard Mitigation. Springer Transactions in Civil and Environmental Engineering. Springer Singapore.
- Al Shoumik BA, Khan MZ, Islam MS. 2023. characteristics of meteorological and agricultural drought indices and their dynamic relationships during the premonsoon season in drought-prone region of Bangladesh. Environ Challeng. 11:100695. doi:10.1016/j.envc.2023.100695.
- Alahacoon N, Edirisinghe M. 2022. A comprehensive assessment of remote sensing and traditional based drought monitoring indices at global and regional scale. Geomat Nat Hazard Risk. 13(1):762–799. doi:10.1080/19475705.2022.2044394.
- Alfred LdJ, Jürgen V. 2015. Analyzing the Combined Drought Indicator (CDI): demonstration and Analysis of its Evolution during Spring and Summer 2013- 2014. Vogt/Agric Agric Sci Proced. 4:222–231. doi:10.1016/j.aaspro.2015.03.026.
- Ali M, Ghaith M, Wagdy A, Helmi AM. 2022. Development of a New Multivariate Composite Drought Index for the Blue Nile River Basin. Water. 14(6):886. doi:10.3390/w14060886.
- Anderson MC, Zolin CA, Sentelhas PC, Hain CR, Semmens K, Tugrul Yilmaz M, Gao F, Otkin JA, Tetrault R. 2016. The Evaporative Stress Index as an indicator of agricultural drought in Brazil: an assessment based on crop yield impacts. Remote Sens Environ. 174:82–99. doi:10.1016/j.rse.2015.11.034.
- Bageshree K. Abhishek, Kinouchi T. 2022. A multivariate drought index for seasonal agriculture drought classification in semiarid regions. Remote Sens. 14(16): 3891. doi:10.3390/rs14163891.
- Balhane S, Driouech F, Chafki O, Manzanas R, Chehbouni A, Moufouma-Okia W. 2022. Changes in mean and extreme temperature and precipitation events from different weighted multimodel ensembles over the northern half of Morocco. Clim Dyn. 58(1–2):389–404. doi:10.1007/s00382-021-05910-w.
- Bayissa Y, Tadesse T, Demisse G. 2019. Building A High-Resolution Vegetation Outlook Model to Monitor Agricultural Drought for the Upper Blue Nile Basin, Ethiopia. Remote Sens. 11(4):371. doi:10.3390/rs11040371.
- Bezdan J, Bezdan A, Blagojević B, Mesaroš M, Pejić B, Vranešević M, Pavić D, Nikolić-Đorić E. 2019. SPEI-Based Approach to Agricultural Drought Monitoring in Vojvodina Region. Water. 11(7):1481. doi:10.3390/w11071481.
- Biasutti M. 2019. Rainfall trends in the African Sahel: characteristics, processes, and causes. Wiley Interdiscip Rev Clim Change. 10(4):e591.
- Bijaber N, El Hadani D, Saidi M, Svoboda M, Wardlow B, Hain Christopher, Poulsen C, Yessef M, Rochdi A. 2018. Developing a remotely sensed drought monitoring indicator for Morocco. Geosciences. 8(2):55. doi:10.3390/geosciences8020055.
- Brandt M, Verger A, Diouf AA, Baret F, Samimi C. 2014. Local vegetation trends in the Sahel of Mali and Senegal using long time series FAPAR satellite products and field measurement (1982–2010). Remote Sens. 6(3):2408–2434. doi:10.3390/rs6032408.
- Cammalleri C, Arias-Muñoz C, Barbosa P, de Jager A, Magni D, Masante D, Mazzeschi M, McCormick N, Naumann G, Spinoni J, et al. 2021. A revision of the Combined Drought Indicator (CDI) used in the European Drought Observatory (EDO). Nat Hazards Earth Syst Sci. 21(2):481–495. doi:10.5194/nhess-21-481-2021.
- Cao Y, Chen S, Wang L, Zhu B, Lu T, Yu Y. 2019. An agricultural drought index for assessing droughts using a water balance method: a case study in Jilin Province, Northeast China. Remote Sens. 11(9):1066. doi:10.3390/rs11091066.
- Chandrasekara SSK, Kwon H-H, Vithanage M, Obeysekera J, Kim T-W. 2021. Drought in South Asia: a Review of Drought Assessment and Prediction in South Asian Countries. Atmosphere. 12(3):369. doi:10.3390/atmos12030369.
- Chen T, Zhou S, Liang C, Hagan DFT, Zeng N, Wang J, Shi T, Chen X, Dolman AJ. 2020. The Greening and Wetting of the Sahel Have Leveled off since approximately 1999 in Relation to SST. Remote Sens. 12(17):2723. doi:10.3390/rs12172723.
- Das AC, Noguchi R, Ahamed T. 2021. An Assessment of Drought Stress in Tea Estates Using Optical and Thermal Remote Sensing. Remote Sens. 13(14):2730. doi:10.3390/rs13142730.
- Dixit S, Jayakumar KV. 2022. Spatio‐temporal analysis of copula‐based probabilistic multivariate drought index using CMIP6 model. Int J Climatol. 42(8):4333–4350. doi:10.1002/joc.7469.
- Du L,Tian Q,Yu T,Meng Q,Jancso T,Udvardy P,Huang Y. 2013. A comprehensive drought monitoring method integrating MODIS and TRMM data. Int J Appl Earth Obs Geoinf. 23:245–253. doi:10.1016/j.jag.2012.09.010.
- Du C, Chen J, Nie T, Dai C. 2022. Spatial–temporal changes in meteorological and agricultural droughts in Northeast China: change patterns, response relationships and causes. Nat Hazards. 110(1):155–173. doi:10.1007/s11069-021-04940-1.
- Fall CMN, Lavaysse C, Kerdiles H, Dramé S, Roudier P, Gaye AT. 2021. Performance of dry and wet spells combined with remote sensing indicators for crop yield prediction in Senegal. Clim Risk Manage. 33:100331. doi:10.1016/j.crm.2021.100331.
- Feng P, Wang L, De L, Yu Q. 2019. Machine learning-based integration of remotely sensed drought factors can improve the estimation of agricultural drought in South-Eastern Australia. Agric Syst. 173():303–316. doi:10.1016/j.agsy.2019.03.015.
- Flint AE, Flint AL, Thorn JH. 2014. Climate change: evaluating your local and regional water resources. US Geol. Surv.(USGS) Fact Sheet. 3098:1–6.
- Gao Y, Gao M, Damdinsuren B, Dorjsuren M. 2021. Early drought warning based on chlorophyll fluorescence and normalized difference vegetation index in Xilingol League of China. J Appl Rem Sens. 15(03):032006. doi:10.1117/1.JRS.15.032006.
- Ghazaryan G, Dubovyk O, Graw V, Kussul N, Schellberg J. 2020. Local-scale agricultural drought monitoring with satellite-based multisensor time-series. GIScience Remote Sens. 57(5):704–718. doi:10.1080/15481603.2020.1778332.
- Gidey E, Dikinya O, Sebego R, Segosebe E, Zenebe A. 2018. Analysis of the long-term agricultural drought onset, cessation, duration, frequency, severity and spatial extent using Vegetation Health Index (VHI) in Raya and its environs, Northern Ethiopia. Environ Syst Res. 7(1):18. doi:10.1186/s40068-018-0115-z.
- Guo H, Bao A, Liu T, Ndayisaba F, Jiang L, Zheng G, Chen T, De Maeyer P. 2019. Determining variable weights for an Optimal Scaled Drought Condition Index (OSDCI): evaluation in Central Asia. Remote Sens of Environ. 231:111220. doi:10.1016/j.rse.2019.111220.
- Han H, Bai J, Yan J, Yang H, Ma G. 2021a. A combined drought monitoring index based on multisensor remote sensing data and machine learning. Geocarto Int. 36(10):1161–1177.
- Han Z, Huang Q, Huang S, Leng G, Bai Q, Liang H, Wang L, Zhao J, Fang W. 2021b. Spatial-temporal dynamics of agricultural drought in the Loess Plateau under a changing environment: characteristics and potential influencing factors. Agric Water Manage. 244:106540. doi:10.1016/j.agwat.2020.106540.
- Hanadé HI, El Mansouri L, Gadal S, Garba M, Hadria R. 2022a. Modeling agricultural drought: a review of latest advances in big data technologies. Geomat Nat Hazards Risk. 13(1):2737–2776. doi:10.1080/19475705.2022.2131471.
- Hanadé HI, El Mansouri L, Hadria R. Emran A, Chehbouni A. 2022b. Retrospective analysis and version improvement of the satellite-based drought composite index. A Semiarid Tensift-Morocco application. Geocart Int.37(11): 3069–3090.
- Hao C, Zhang J, Yao F. 2015. Combination of multisensor remote sensing data for drought monitoring over Southwest China. Int J Appl Earth Obs Geoinf. 35(): 270–283. doi:10.1016/j.jag.2014.09.011.
- Hao Z, Singh VP. 2015. Drought characterization from a multivariate perspective: a review. J Hydrol. 527:668–678. doi:10.1016/j.jhydrol.2015.05.031.
- Hara P, Piekutowska M, Niedbała G. 2021. Selection of independent variables for crop yield prediction using artificial neural network models with remote sensing data. Land. 10(6):609. doi:10.3390/land10060609.
- Hazaymeh K, Hassan Q. 2016. Remote sensing of agricultural drought monitoring: a state of art review. AIMS Environ Sci. 3(4):604–630. doi:10.3934/environsci.2016.4.604.
- Hendrawan VS, Kim W, Touge Y, Ke S, Komori D. 2022. A global-scale relationship between crop yield anomaly and multiscalar drought index based on multiple precipitation data. Environ Res Lett. 17(1):014037. doi:10.1088/1748-9326/ac45b4.
- Hoang VP, Nguye MH, Do TQ, Le DN, Bui DD. 2020. A long range, energy efficient Internet of Things based drought monitoring system. IJECE. 10(2):1278–1287. doi:10.11591/ijece.v10i2.pp1278-1287.
- Huang S, Huang Q, Chang J, Leng G, Xing L. 2015. The response of agricultural drought to meteorological drought and the influencing factors: a case study in the Wei River Basin, China. Agric Water Manage. 159:45–54. doi:10.1016/j.agwat.2015.05.023.
- Jiang T,Su X,Zhang G,Zhang T,Wu H. 2023. Estimating propagation probability from meteorological to ecological droughts using a hybrid machine learning copula method. Hydrol Earth Syst Sci. 27(2):559–576. doi:10.5194/hess-27-559-2023.
- Jiang R, Xie J, He H, Luo J, Zhu J. 2015. Use of four drought indices for evaluating drought characteristics under climate change in Shaanxi, China: 1951–2012. Nat Hazards. 75(3):2885–2903. doi:10.1007/s11069-014-1468-x.
- Jiang T, Su X, Singh VP, Zhang G. 2021. A novel index for ecological drought monitoring based on ecological water deficit. Ecol Indic. 129:107804. doi:10.1016/j.ecolind.2021.107804.
- Jiao T,Williams CA,De Kauwe MG,Schwalm CR,Medlyn BE. 2021. Patterns of post-drought recovery are strongly influenced by drought duration, frequency, post-drought wetness, and bioclimatic setting. Glob Chang Biol. 27(19):4630–4643. doi:10.1111/gcb.15788.34228866
- Jiménez-Donaire M, Giráldez JV, Vanwalleghem T. 2020. Evaluation of Drought Stress in Cereal through Probabilistic Modeling of Soil Moisture Dynamics. Water. 12(9):2592. doi:10.3390/w12092592.
- Kafy AA, Bakshi A, Saha M, Al Faisal A, Almulhim AI, Rahaman ZA, Mohammad P. 2023. Assessment and prediction of index based agricultural drought vulnerability using machine learning algorithms. Sci Total Environ. 867:161394. doi:10.1016/j.scitotenv.2023.161394.
- Kaur A, Sood SK. 2020a. Cloud-centric IoT-based green framework for smart drought prediction. IEEE Internet Things J. 7(2):1111–1121. doi:10.1109/JIOT.2019.2951610.
- Kaur A, Sood SK. 2020b. Artificial intelligence-based model for drought prediction and forecasting. Computer J. 63(11):1704–1712. doi:10.1093/comjnl/bxz105.
- Kim J-S, Park SY, Lee J-H, Chen J, Chen S, Kim T-W. 2021. Integrated Drought Monitoring and Evaluation through Multi-Sensor Satellite-Based Statistical Simulation. Remote Sens. 13(2):272. doi:10.3390/rs13020272.
- Klein T. 2009. Comparaison des sécheresses estivales de 1976 et 2003 en Europe occidentale à l’aide d’indices climatiques. Bull Soc Géograph Liège. 53:75–86.
- Kogan FN. 1997. Global Drought Watch from Space. Bull Amer Meteor Soc. 78(4):621–636. doi:10.1175/1520-0477(1997)078<0621:GDWFS>2.0.CO;2.
- Kogan FN. 1990. Remote sensing of weather impacts on vegetation in nonhomogeneous areas. Int J Remote Sens. 11(8):1405–1419. doi:10.1080/01431169008955102.
- Kogan FN. 1995. Application of vegetation index and brightness temperature for drought detection. Adv Sp Res. 15(11):91–100. doi:10.1016/0273-1177(95)00079-T.
- Kukunuri AN, Murugan D, Singh D. 2022. Variance based fusion of VCI and TCI for efficient classification of agriculture drought using MODIS data. Geocarto Int. 37(10):2871–2892. doi:10.1080/10106049.2020.1837256.
- Kulkarni SS, Wardlow BD, Bayissa YA, Tadesse T, Svoboda MD, Gedam SS. 2020. Developing a Remote Sensing-Based Combined Drought Indicator Approach for Agricultural Drought Monitoring over Marathwada, India. Remote Sens. 12(13):2091. doi:10.3390/rs12132091.
- Le T, Sun C, Choy S, Kuleshov Y. 2021. Regional drought risk assessment in the Central Highlands and the South of Vietnam. Geomat Nat Hazards Risk. 12(1):3140–3159. doi:10.1080/19475705.2021.1998232.
- Lebel T, Ali A. 2009. Recent trends in the Central and Western Sahel rainfall regime (1990–2007). J Hydrol. 375(1-2):52–64. doi:10.1016/j.jhydrol.2008.11.030.
- Lebourgeois F. and Piedallu C. 2005. Appréhender le niveau de sécheresse dans le cadre des études stationnelles et de la gestion forestière à partir d’indices bioclimatiques. Rev For Fr 57(4):331–356.
- Leroux L, Castets M, Baron C, Escorihuela M, Bégué A, Seen DL. 2019. Maize yield estimation in West Africa from crop process-induced combinations of multidomain remote sensing indices. Eur J Agron. 108:11–26. doi:10.1016/j.eja.2019.04.007.
- Li J, Heap AD. 2014. Spatial interpolation methods applied in the environmental sciences: a review. Environ Model Softw. 53:173–189. doi:10.1016/j.envsoft.2013.12.008.
- Li Q, Li P, Li H, Yu M. 2015. Drought assessment using a multivariate drought index in the Luanhe River basin of Northern China. Stoch Environ Res Risk Assess. 29(6):1509–1520. doi:10.1007/s00477-014-0982-4.
- Li S, Xu X. 2021. Study on remote sensing monitoring model of agricultural drought based on random forest deviation correction. INMATEH. 64(2):413–422. doi:10.35633/inmateh-64-41.
- Liu Q, Zhang S, Zhang H, Bai Y, Zhang J. 2020. Monitoring drought using composite drought indices based on remote sensing. Sci Total Environ. 711:134585. doi:10.1016/j.scitotenv.2019.134585.
- Liu X, Zhu X, Pan Y, Bai J, Li S. 2018. Performance of different drought indices for agriculture drought in the North China Plain. J Arid Land. 10(4):507–516. doi:10.1007/s40333-018-0005-2.
- Liu X, Zhu X, Pan Y, Li S, Liu Y, Ma Y. 2016. Agricultural drought monitoring: progress, challenges, and prospects. J Geogr Sci. 26(6):750–767. doi:10.1007/s11442-016-1297-9.
- Loulli E, Hadjimitsis DG. 2018. Remote Sensing-Based Indices for Drought Assessment in the East Mediterranean Region," Proc. SPIE 10783, Remote Sensing for Agriculture, Ecosystems, and Hydrology XX, 1078314. doi:10.1117/12.2325331.
- Mansour Badamassi MB, El-Aboudi A, Gbetkom PG. 2020. A new index to better detect and monitor agricultural drought in Niger using multisensor remote sensing data. Profess Geograph. 72(3):421–432. doi:10.1080/00330124.2020.1730197.
- McKee TB, Nj D, Kleist J. 1993. The relationship of drought frequency and duration of time scales. Eighth Conference on Applied Climatology, American Meteorological Society, Jan 17-23, 1993, Anaheim CA, p. 179–186.
- Mishra AK, Singh P. 2010. A review of drought concepts. J Hydrol. 391(1-2):202–216. doi:10.1016/j.jhydrol.2010.07.012.
- Mohammed L. 2008. Calcul des indicateurs de sécheresse à partir des images NOAA/AVHRR: rapport CRTS projet de mise en place d’un système d’alerte précoce à la sécheresse dans trois pays de la rive sud de la méditerranée: algérie, Maroc, et Tunisie.
- Mokhtar A, Jalali M, He H, Al-Ansari N, Elbeltagi A, Alsafadi K, Ghassan Abdo H, Sammen S, Gyasi-Agyei Y, Rodrigo-Comino J. 2021. Estimation of SPEI Meteorological Drought Using Machine Learning Algorithms « in. IEEE Access. 9:65503–65523. doi:10.1109/ACCESS.2021.3074305.
- Naderi K, Moghaddasi M, Shokri A. 2022. Drought Occurrence Probability Analysis Using Multivariate Standardized Drought Index and Copula Function Under Climate Change. Water Resour Manage. 36(8):2865–2888. doi:10.1007/s11269-022-03186-1.
- Nam W, Tadesse T, Wardlow B, Hayes M, Svoboda M, Hong E, Pachepsky Y, Jang M. 2018. Developing the vegetation drought response index for South Korea (VegDRI-SKorea) to assess the vegetation condition during drought events. Int J Remote Sens. 39(5):1548–1574. doi:10.1080/01431161.2017.1407047.
- Neeti N, Arun Murali CM, Chowdary VM, Rao NH, Kesarwani M. 2021. Integrated meteorological drought monitoring framework using multisensor and multitemporal earth observation datasets and machine learning algorithms: a case study of central India. J Hydrol. 601:126638. doi:10.1016/j.jhydrol.2021.126638.
- Nooni IK, Hagan DFT, Wang G, Ullah W, Li S, Lu J, Bhatti AS, Shi X, Lou D, Prempeh NA, et al. 2021. Spatiotemporal Characteristics and Trend Analysis of Two Evapotranspiration-Based Drought Products and Their Mechanisms in Sub-Saharan Africa. Remote Sens. 13(3):533. doi:10.3390/rs13030533.
- Noureldeen N, Mao K, Mohmmed A, Yuan Z, Yang Y. 2020. Spatiotemporal Drought Assessment over Sahelian Countries from 1985 to 2015. J Meteorol Res. 34(4):760–774. doi:10.1007/s13351-020-9178-7.
- Nugraha ASA, Kamal M, Murti SH, Widyatmanti W. 2023. Development of the triangle method for drought studies based on remote sensing images: a review. Remote Sens Appl: Soc Environ. 29:100920. doi:10.1016/j.rsase.2023.100920.
- Palmer WC. 1965. Meteorological drought, U.S. Weather Bureau Research Paper 45. pp. 58.
- Panthou G, Vischel T, Lebel T. 2014. Recent trends in the regime of extreme rainfall in the Central Sahel. Int J Climatol. 34(15):3998–4006. doi:10.1002/joc.3984.
- Park JH, Lee JH, Kim TW, Kwon HH. 2019. A development of multivariate drought index using the simulated soil moisture from a GM-NHMM model. J Korea Water Resour Assoc. 52(8):545–554.
- Patel NR, Yadav K. 2015. Monitoring spatiotemporal pattern of drought stress using integrated drought index over Bundelkhand region, India. Nat Hazards. 77(2):663–677. doi:10.1007/s11069-015-1614-0.
- Petersen LK. 2018. Real-Time Prediction of Crop Yields from MODIS Relative Vegetation Health: a Continent-Wide Analysis of Africa. Remote Sens. 10(11):1726. doi:10.3390/rs10111726.
- Porkka M, Wang-Erlandsson L, Destouni G, Ekman AML, Rockström J, Gordon LJ.,. 2021. Is wetter better? Exploring agriculturally relevant rainfall characteristics over four decades in the Sahel. Environ Res Lett. 16(3):035002. doi:10.1088/1748-9326/abdd57.
- Prodhan FA, Zhang J, Yao F, Shi L, Pangali Sharma TP, Zhang D, Cao D, Zheng M, Ahmed N, MOHana HP. 2021. Deep Learning for Monitoring Agricultural Drought in South Asia Using Remote Sensing Data. Remote Sens. 13(9):1715. doi:10.3390/rs13091715.
- Qaiser G, Tariq S, Adnan S, Latif M. 2021. Evaluation of a composite drought index to identify seasonal drought and its associated atmospheric dynamics in Northern Punjab, Pakistan. J Arid Environ. 185:104332. doi:10.1016/j.jaridenv.2020.104332.
- Rahmati O,Falah F,Dayal KS,Deo RC,Mohammadi F,Biggs T,Moghaddam DD,Naghibi SA,Bui DT. 2020. Machine learning approaches for spatial modeling of agricultural droughts in the south-east region of Queensland Australia. Sci Total Environ. 699:134230 doi:10.1016/j.scitotenv.2019.134230.
- Rajsekhar D, Singh VP, Mishra AK. 2015. Multivariate drought index: an information theory-based approach for integrated drought assessment. J Hydrol. 526:164–182. doi:10.1016/j.jhydrol.2014.11.031.
- Saha S, Gogoi P, Gayen A, Paul GC. 2021. Constructing the machine learning techniques based spatial drought vulnerability index in Karnataka state of India. J Clean Prod. 314:128073. VolumeISSN 0959-6526. doi:10.1016/j.jclepro.2021.128073.
- Saha S, Kundu B, Saha A, Mukherjee K, Pradhan B. 2023. Manifesting deep learning algorithms for developing drought vulnerability index in monsoon climate dominant region of West Bengal, India. Theor Appl Climatol. 151(1-2):891–913. doi:10.1007/s00704-022-04300-4.
- San Emeterio JL, Lacaze B, Mering C. 2012. Détection des changements de la couverture végétale au Sahel durant la période 1982-2002 à partir des données NDVI et précipitation. Télédétection. 10(2-3):135–143.
- Schwartz C, Ellenburg WL, Mishra V, Mayer T, Griffin R, Qamer F, Matin M, Tadesse T. 2022. A statistical evaluation of Earth-observation-based composite drought indices for a localized assessment of agricultural drought in Pakistan. Int J Appl Earth Obs Geoinf. 106:102646. doi:10.1016/j.jag.2021.102646.
- Senapati U, Das TK. 2022. Geospatial assessment of agricultural drought vulnerability using integrated three-dimensional model in the upper Dwarakeshwar river basin in West Bengal, India. Environ Sci Pollut Res.1–28. doi:10.1007/s11356-022-23663-9.
- Sepulcre-Canto G, Horion S, Singleton A, Carrao H, Vogt J. 2012. Development of a Combined Drought Indicator to detect agricultural drought in Europe. Nat Hazards Earth Syst Sci. 12(11):3519–3531. doi:10.5194/nhess-12-3519-2012.
- Shaikhina T, Lowe D, Dag S, Briggs D, Higgins R, Khovanova N. 2019. Decision tree and random forest models for outcome prediction in antibody incompatible kidney transplantation. Biomed Signal Process Control. 52:456–462. doi:10.1016/j.bspc.2017.01.012.
- Shamshirband S, Hashemi S, Salimi H, Samadianfard S, Asadi E, Shadkani S, Kargar K, Mosavi A, Nabipour N, Chau K-W. 2020. Predicting Standardized Streamflow index for hydrological drought using machine learning models. Eng Appl Comput Fluid Mech. 14(1):339–350. doi:10.1080/19942060.2020.1715844.
- Sharara A, Shekede MD, Gwitira I, Masocha M, Dube T. 2022. Fine-scale multitemporal and spatial analysis of agricultural drought in agro-ecological regions of Zimbabwe. Geomat Nat Hazards Risk. 13(1):1342–1365. doi:10.1080/19475705.2022.2072774.
- Shen R,Huang A,Li B,Guo J. 2019. Construction of a drought monitoring model using deep learning based on multi-source remote sensing data. Int J Appl Earth Obs Geoinf 79:48–57. doi:10.1016/j.jag.2019.03.006.
- Singh TP, Deshpande M, Das S, Kumbhar V. 2022. Drought pattern assessment over Marathwada, India through the development of multivariate advance drought response index. Int Arch Photogramm Remote Sens Spatial Inf Sci. XLIII-B3-2022:1173–1180. doi:10.5194/isprs-archives-XLIII-B3-2022-1173-2022.
- Son B, Park S, Im J, Park S, Ke Y, Quackenbush LJ. 2021. A new drought monitoring approach: vector Projection Analysis (VPA). Remote Sens Environ. 252:112145. doi:10.1016/j.rse.2020.112145.
- Sultana MS, Gazi MY, Mia MB. 2021. Multiple indices based agricultural drought assessment in the northwestern part of Bangladesh using geospatial techniques. Environ Challenges. 4:100120. doi:10.1016/j.envc.2021.100120.
- Sur C, Park SY, Kim T-W, Lee JH. 2019. Remote Sensing-based Agricultural Drought Monitoring using Hydrometeorological Variables. KSCE J Civ Eng. 23(12):5244–5256. doi:10.1007/s12205-019-2242-0.
- Sutanto SJ, Van der Weert M, Blauhut V, Van Lanen HAJ. 2020. Skill of large-scale seasonal drought impact forecasts. Nat Hazards Earth Syst Sci. 20(6):1595–1608. doi:10.5194/nhess-20-1595-2020.
- Svoboda M, Fuchs B. 2016. Handbook of Drought Indicators and Indices; World Meteorological Organization (WMO): Geneva, Switzerland, 2016.
- Szczypta C. 2012. Hydrologie Spatiale pour le suivi des sécheresses du bassin méditerranéen. Thèse de doctorat, Ecole doctorale: SDU2E (Sciences de l’Univers, de l’Environnement et de l’Espace). 197 pages
- Teweldebirhan TD, Uddameri V, Forghanparast F, Hernandez EA, Ekwaro-Osire S. 2019. Comparison of meteorological-and agriculture-related drought indicators across Ethiopia. Water. 11(11):2218. doi:10.3390/w11112218.
- Tian L, Yuan S, Quiring SM. 2018. Evaluation of six indices for monitoring agricultural drought in the south-central United States. Agric for Meteorol. 249:107–119. doi:10.1016/j.agrformet.2017.11.024.
- Tian Q, Lu J, Chen X. 2022. A novel comprehensive agricultural drought index reflecting time lag of soil moisture to meteorology: a case study in the Yangtze River basin, China. Catena. 209:105804. doi:10.1016/j.catena.2021.105804.
- Vicente-Serrano SM, Cabello D, Tomás-Burguera M, Martín-Hernández N, Beguería S, Azorin-Molina C, Kenawy AE. 2015. Drought Variability and Land Degradation in Semiarid Regions: assessment Using Remote Sensing Data and Drought Indices (1982–2011). Remote Sens. 7(4):4391–4423. doi:10.3390/rs70404391.
- Wang J, Yu Y. 2021. Comprehensive drought monitoring in Yunnan Province China using multisource remote sensing data. J Mt Sci. 18(6):1537–1549. doi:10.1007/s11629-020-6333-7.
- Waseem M, Ajmal M, Kim TW. 2015. Development of a new composite drought index for multivariate drought assessment. J Hydrol. 527:30–37. doi:10.1016/j.jhydrol.2015.04.044.
- West CT, Moody A, Nébié EK, Sanon O. 2017. Ground-Truthing Sahelian Greening: Ethnographic and Spatial Evidence from Burkina Faso. Hum Ecol Interdiscip J. 45(1):89–101. doi:10.1007/s10745-016-9888-8.
- Wu B, Zhang M, Zeng H, Tian F, Potgieter AB, Qin X, Yan N, Chang S, Yan Z, Dong Q, et al. 2023. Challenges and opportunities in remote sensing-based crop monitoring: a review. Natl Sci Rev. 10(4):nwac290. doi:10.1093/nsr/nwac290.
- Wu R, Liu Y, Xing X. 2021. Evaluation of evapotranspiration deficit index for agricultural drought monitoring in North China. J Hydrol. 596:126057. doi:10.1016/j.jhydrol.2021.126057.
- Yan H, Wang SQ, Wang JB, Lu HQ, Guo AH, Zhu ZC, Myneni RB, Shugart HH. 2016. Assessing spatiotemporal variation of drought in China and its impact on agriculture during 1982–2011 by using PDSI indices and agriculture drought survey data. J Geophys Res Atmos. 121(5):2283–2298. doi:10.1002/2015JD024285.
- Yang X, Li YP, Huang GH, Zhang SQ. 2022. Analyzing spatial–temporal change of multivariate drought risk based on Bayesian copula: application to the Balkhash Lake basin. Theor Appl Climatol. 149(1-2):787–804. doi:10.1007/s00704-022-04062-z.
- Yu X, Guo X, Wu Z. 2014. Land surface temperature retrieval from Landsat 8 TIRS—Comparison between radiative transfer equation-based method, split window algorithm and single channel method. Remote Sens. 6(10):9829–9852. doi:10.3390/rs6109829.
- Zhao X, Xia H, Liu B, Jiao W. 2022. Spatiotemporal comparison of drought in Shaanxi–Gansu–Ningxia from 2003 to 2020 using various drought indices in google earth engine. Remote Sens. 14(7):1570. doi:10.3390/rs14071570.
- Zhou X, Zhu X, Dong Z, Guo W. 2016. Estimation de la biomasse du blé à l‘aide d‘un algorithme de régression forestière aléatoire et de données de télédétection. Crop J. 4(3):212–219.
- Zhuo W, Huang J, Zhang X, Sun H, Zhu D, Su W, … Liu Z. 2016. July. Comparison of Five Drought Indices for Agricultural Drought Monitoring and Impacts on Winter Wheat Yields Analysis. In 2016 Fifth International Conference on Agro-Geoinformatics (Agro-Geoinformatics) (pp. 1-6). IEEE.