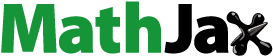
Abstract
Storm surge-induced flooding (SSIF) is a major hazard for coastal areas under intensified typhoons. Therefore, it is essential to assess the potential impacts of SSIF (SSPIA). This study proposes a multidisciplinary framework for refined SSPIA using an ocean model and a refined exposure estimation method. First, a finite-volume coastal ocean model (FVCOM) and a typhoon model were developed and validated. Then, five scenarios of varying intensity were defined and combined with FVCOM to identify inundation scenarios. Subsequently, machine learning was used to obtain the fine-scale gridded population and gross domestic product (GDP) maps based on the census and geospatial data. Finally, we assessed the magnitude of the affected population and GDP based on the inundation scenarios and refined exposure datasets. We selected Zhoushan Island as a study area to implement this framework. Our assessment results show that the lowest scenario (955 hPa) affected 2587 people and 323.745 million CNY of GDP, while the highest scenario (915 hPa) affected 259,516 people and 20,178.898 million CNY of GDP. Therefore, it is imperative to implement effective mitigation and adaptation measures to address the threat of SSIF. This framework will apply to all flood-prone areas for a refined assessment of the potential impacts of SSIF.
1. Introduction
Storm surges are extreme coastal events that can cause severe storm surge-induced flooding (SSIF) and damage in low-elevation coastal zones (LECZs) (Fang et al. Citation2017; Wang et al. Citation2021a). China has a long coastline that spans from south to north, with more than one-third of its county-level administrative regions exposed to SSIF (Xianwu et al. Citation2020). In recent years, SSIF has become more frequent and intense due to global climate change, sea level rise, and land subsidence, posing greater risks to people and assets in LECZs (Yin et al. Citation2013; Wang et al. Citation2018, Citation2021b; Nayak and Takemi Citation2023). Therefore, it is imperative to conduct a refined assessment of the potential impacts of storm surge-induced flooding (SSPIA) in China’s coastal cities or critical zones (Liu et al. Citation2015).
Hazards are defined as natural events that affect people or property, and their impact is determined by the depth and extent of inundation in the case of SSIF (Shepard et al. Citation2012). Common approaches for estimating SSIF include the bathtub method (BTM) and ocean model simulation (Yin et al. Citation2021). BTM is a geographic information system (GIS)-based method that is widely used to identify areas that are inundated below a certain water level (Rowley et al. Citation2007; Feng et al. Citation2018). However, this method has limitations, as it can incorrectly identify low-lying areas that are disconnected from the coast as flooded (Williams and Lück-Vogel Citation2020). As a result, ocean model simulations have become the preferred method for obtaining reliable coastal inundation information. Several robust ocean numerical simulation models, such as the Finite-Volume Coastal Ocean Model (FVCOM) and the Advanced Circulation Model (ADCIRC), have been developed to simulate SSIF (Chen et al Citation2003; Dietrich et al. Citation2012). These models can be used to estimate extreme storm surge inundation for typical typhoon classes, return periods, and probable maximum storm surge inundation (Shi et al. Citation2020; Wang, Guo, et al. Citation2021; Wang, Mu, Yao, et al. Citation2021). In China, these methods have been applied to estimate SSIF in many LECZs.
To assess the potential impact of SSIF on coastal socioeconomic systems, it is important to consider the potentially affected populations and economies. Many countries have established their flood damage estimation models (Scawthorn et al. Citation2006; Amadio et al. Citation2016; Aznar-Siguan and Bresch Citation2019). To effectively estimate the potential impact of SSIF, a detailed and accurate distribution of population or gross domestic product (GDP) is essential for exposure assessment. However, obtaining detailed information on the spatial distribution of population/GDP from census data can be challenging because the actual distribution is quite heterogeneous within the boundaries of these administrative boundaries, hindering the integration of natural and social sciences (Molinari et al. Citation2019; Chakraborty et al. Citation2022). In addition, the insufficient resolution of census data leads to a high degree of uncertainty in the prediction of potential fatalities and injuries (Mondal and Tatem Citation2012). Consequently, cross-disciplinary research by matching census data with these fine-scale hazard data (e.g. inundation depth and extent) is challenging. In other words, refined gridded population/GDP maps are essential for integrating census population/GDP data with disaster risk assessment variables (Merz et al. Citation2010). As a result, obtaining refined gridded population/GDP maps remains the most significant challenge in SSPIA.
Recently, scholars have developed various refined exposure estimation methods to decompose census population/GDP data into spatial grid units using social perception data, such as points of interest (POIs), nighttime light (NTL) data, and road network data (Dobson et al. Citation2000; Tatem Citation2017). For example, Ye et al. (Citation2019) used Random Forest (RF) and POIs to map the distribution of the population in China in 2010 with a resolution of 100 m. Chen et al. (Citation2021) used RF and POIs to map the distribution of three sub-sectors of GDP in China in 2010 with a resolution of 1000 m. Wang et al. (Citation2019) used the isolation forest machine learning algorithm and NTL to generate a distribution of summed GDP with a resolution of 1000 m. However, these previous population or GDP datasets are outdated and difficult to apply to SSPIA. Furthermore, their low resolution is too coarse for refined SSPIA (Dabbeek et al. Citation2021). In particular, detailed and accurate information on population/GDP distribution with a high spatial resolution (<50 m) is crucial for SSPIA in coastal flood-prone areas near the coastline to achieve effective refined SSPIA. Accordingly, the refined exposure estimation method could be combined with ocean model simulation, thus greatly realizing the possibility of a refined SSPIA.
In this study, we focus on Zhoushan City in eastern China, which is constantly threatened by storm surges. This city is one of the first prefecture-level cities in China to be built in the form of an archipelago. It is also an important crude oil transportation base, a prominent port with the world’s largest cargo throughput, and a famous fishing ground (Ma et al. Citation2020; Deng et al. Citation2023). However, due to its subtropical marine monsoon climate, this area is frequently hit by storms and typhoons (Li et al. Citation2021). Furthermore, islands are even more vulnerable to coastal flooding than continental coastal zones and thus require more attention to protect their socioeconomic systems from this hazard (Ma et al. Citation2020). In addition, human activities have intensified the development of these islands in recent years (Zhang et al. Citation2014), highlighting the importance of SSPIA in Zhoushan. Although many scholars (Zhilin et al. Citation2020; Shi et al. Citation2021; Huang et al. Citation2023) have conducted numerous studies on the hydrodynamic conditions in the sea around Zhoushan, the refined SSPIA of Zhoushan is still limited. Therefore, there is an urgent need to conduct a refined SSPIA based on the refined exposure estimation in Zhoushan.
The purpose of this study is to provide a multidisciplinary framework for refined SSPIA using an ocean model and a refined exposure estimation method. This study aims to solve the problem of poor resolution in SSPIA caused by the difficulty in obtaining fine-resolution population and GDP data, thereby greatly improving the possibility of refined SSPIA. This study is organized as follows: First, FVCOM and Jelesnianski typhoon models were developed and validated. Second, five typhoon scenarios with different intensities were defined and combined with FVCOM to identify extreme inundation scenarios. Third, GIS was used to visualize and assess SSIF hazard levels based on simulated inundation extent and depth. Fourth, fine-resolution population and GDP maps were generated using RF and the Dasymetric Mapping Method (DMM) to bridge the gap between census data and SSIF hazard data. Finally, storm surge inundation areas were overlaid with population and GDP density maps to estimate the magnitude of the affected population and GDP.
2. Study area and datasets
2.1. Study area
Zhoushan () is an archipelago of thousands of islands located near the confluence of the Yangtze River estuary and Hangzhou Bay in the East China Sea. Its geographical coordinates are 29°32′–31°04′N and 121°30′–123°25′E. The central city is located on the main island of the archipelago, which is the fourth largest island in China with an area of 468.7 km2. In 2020, Zhoushan had a residential population of more than 1,157,817 and a total GDP of 170,362 million CNY. Zhoushan fishing ground has rich marine fishery resources due to the marine upwelling system created by the conjunction of the coastal and Taiwan currents on the Zhejiang coast.
Figure 1. Study area: (a) map of China; (b) map of Zhejiang Province and the study area; (c) southeastern portion of Zhoushan Island with dikes.

According to the best track dataset from the Shanghai Typhoon Institute of the China Meteorological Administration (CMA-STI) (Lu et al. Citation2021) from 1949 to 2019, a total of 313 typhoons have affected Zhoushan, with an average of 4.47 typhoons per year. In recent decades, Zhoushan has reclaimed a large amount of land from the sea, resulting in the emergence of numerous low-lying coastal communities and industrial areas with high population and asset densities. This study focuses on four streets in the southeastern portion of Zhoushan Island: Lincheng, Qiandao, Donggang, and Shengjiamen Streets. These streets are characterized by high population density and vibrant economic activities. Although its land area is only 13% of Zhoushan’s total area, its residents account for 34% of Zhoushan’s total population in 2020 (see Table A1). Furthermore, according to the Zhoushan City Land Use Master Plan released by the Zhoushan Natural Resources and Planning Bureau, Lincheng and Qiandao Streets are the new areas with the highest development intensity in Zhoushan; Donggang and Shengjiamen Streets are the most famous tourist areas in Zhoushan.
Table 1. Typhoon intensity scenarios.
2.2. Datasets
This study used a variety of datasets, including observed datasets, such as historical tropical cyclone data, bathymetry, and water level records; survey datasets, such as storm surge dike information, population and GDP census data, administrative boundaries, coastline, and distance to the coastline; Raster datasets, such as NTL, Land Use/Land Cover (LULC), Land Surface Temperature (LST), Normalized Difference Vegetation Index (NDVI), Digital Elevation Model (DEM), and Slope; and Big Data geospatial datasets, such as POIs and road networks. Detailed information on the retrieval and preprocessing of these datasets can be found in Supplementary Material A2.
3. Methodology
This study proposes a multidisciplinary framework for refined SSPIA using an ocean model and a refined exposure estimation method. The framework has four components: (1) ocean model building and validation; (2) typhoon scenario definition and extreme flooding simulation; (3) refined exposure estimation; and (4) potential impact estimation. The process for carrying out this methodology is shown in .
Figure 2. Flowchart for conducting the potential impacts estimation of storm surge-induced flooding based on the refined exposure estimation.

3.1. Extreme inundation estimation
3.1.1. Storm surge model
FVCOM is used to simulate the SSIF in this study area. This model uses a non-overlapping, unstructured triangular grid in the horizontal to resolve the dynamics in complex regions, making it highly suitable for the present study with its irregular and complex coastlines. In addition, FVCOM includes a commonly used wet/dry scheme and a dike-groyne module (Chen et al. Citation2008; Ge et al. Citation2012). This model has been successfully applied to numerous estuaries and continental shelf areas for storm surge simulation (Chu et al. Citation2019; He et al. Citation2020; Li et al. Citation2022).
As shown in , the computational domain includes the coastlines and islands of the Bohai Sea, the Yellow Sea, and the East China Sea. The mesh resolution in the coastal lowland regions is maintained at about 30–50 m (see ). At the open boundary, the mesh resolution is about 20 km. Thus, the model mesh consists of 102,355 nodes and 197,865 triangular elements. Supplementary Material A2 provides more details on the bathymetry data and DEM. The dikes around Zhoushan provide a robust defense mechanism against coastal flooding. These dikes are included in FVCOM using the dike-groyne module. Detailed information on the dike defense was provided by the Second Surveying and Mapping Institute of Zhejiang Province. The Donggang dike has a defense standard of 50 years, and its height varies from 2.81 to 4.20 m (sampled at ∼200 m intervals). The Shenjiamen dike has a design high tide level of 3.14 m and its height varies from about 2.5 to 3.9 m. The dike height of Qiandao and Lincheng Streets varies from 3.1 to 5.2 m.
Figure 3. Mesh gird in the numerical model for evaluating storm surge risk: (a) computational domain; (b) domain over the study area; (c) domain over the Qiandao Street; (d) domain over the Shengjiamen Street; and (e) domain over the Donggang Street.

This FVCOM model is run in the three-dimensional mode where temperature and salinity are held constant. Four sigma uniform layers are used in the vertical direction. The TPXO 9 global model of ocean tides was used to determine water levels along the open boundaries (Egbert and Erofeeva Citation2002). Hourly tidal heights were predicted by thirteen tidal components (i.e. K1, K2, M2, M4, MF, MM, MN4, MS4, N2, O1, P1, Q1, and S2). Meanwhile, the Jelesnianski typhoon model was used to calculate the spatiotemporal variations of air pressure and wind field (Jelesnianski Citation1965). The Supplementary Material provides more details on the FVCOM parameter configuration and the typhoon model.
The performance of the ocean model was verified using three typhoon events (Figure A2), namely No. 9417 (Fred), No. 9711 (Winnie), and No. 1509 (Chan-Hom). The model-simulated water level was compared with the measured water level at several tide gauges along the coast (see the Supplementary Material for details on model validation, Figure A3 and Table A4). The results showed that the model can reasonably reproduce the observed water level, indicating its ability to simulate storm surges in the study area.
3.1.2. Scenario configuration
The typhoon field is determined by several typhoon parameters, including the typhoon central air pressure (P0), maximum wind speed (Vmax), maximum wind radius (Rmax), and typhoon tracks. According to CMA-STI, Zhoushan has experienced frequent typhoon events, with 313 occurrences from 1959 to 2019, an average of 4.47 times per year. About 36.7% of the typhoons had a typhoon central air pressure lower than 955 hPa at landfall (Figure A4). Typhoon No. 9711 (Winnie) was the most destructive storm surge disaster, causing 1482 deaths in Zhejiang Province and more than 14 million CNY in economic losses. The typhoon’s central air pressure at landfall reached 955 hPa with a maximum wind speed of 55 m/s. According to the Technical Guidelines for Risk Assessment and Zoning of Marine Disasters, Part 1: Storm Surge (Liu et al. Citation2018), this study considered typhoons with five different intensity levels (), which were calculated based on the typhoon central air pressure at landfall. The values of Vmax were taken according to the Beaufort wind scale table. This method has been widely used to estimate storm surge hazards (Shi et al. Citation2020; Yu et al. Citation2020; Zhilin et al. Citation2020).
The third parameter is Rmax. Accurate measurement of Rmax is extremely difficult (Fujii Citation1998). In this study, an empirical statistical relationship proposed by Cheung et al. (Citation2007) was adopted to calculate Rmax:
(1)
(1)
where Rmax is the maximum wind radius, km; P0 is the typhoon central air pressure, hPa; Rk is an empirical constant with a value of 50 km (Wang, Mu, Yao, et al. Citation2021), P0 is the typhoon central air pressure.
The typhoon track is the fourth typhoon parameter and is mainly determined by the typhoon approach direction (θ) and the typhoon movement speed (Vt). In this study, a coordinate system was constructed based on Zhoushan Island, with the y-axis designated south-north and the x-axis designated west-east. Holding other typhoon parameters constant, the peak storm surge in Zhoushan reaches a maximum when θ is between −45 and 90° (Zhilin et al. Citation2020). Therefore, five typical typhoon approach directions (i.e. −25, 5, 35, 65, and 95°) were selected. Vt is closely related to the latitude of the typhoon center (Chan Citation1984; Liang and Chan Citation2005), and was set at 22.5 km/h based on the latitude of the typhoon track near the study area (Li et al. Citation2014). In this study, the multi-track blending method was used to ensure that each location in the study area was adequately affected by the constructed typhoon tracks (Zhilin et al. Citation2020; Wang, Guo, et al. Citation2021). As a result, Zhoushan Island was covered by twelve parallel tracks in five directions, which were determined by translating at half the maximum wind radius, forming the most severe typhoon events in the study area ().
In addition, when an astronomical high tide occurs during a storm surge, water levels in coastal areas can rise rapidly. To simulate the maximum extent and depth of flooding, this study considered the monthly average high tide. The month-averaged high tides at representative tide stations in the local coastal area from June to October were selected as the astronomical tide associated with the storm surge during the peak storm surge period. The month-averaged high tide at the Zhoushan tide station was 178 cm from June to October from 2003 to 2011 (Table A5), and this value was used as the astronomical high tide in the ocean model for the SSIF simulation. Specifically, based on this monthly astronomical high tide, it was assumed that the peak storm surge occurred simultaneously. The encounter time and typhoon track were used to determine the start time of the SSIF simulation, and the worst-case SSIF was simulated using FVCOM and the Jelesnianski typhoon model (Shi et al. Citation2020).
3.1.3. Hazard assessment
Wind and pressure fields were generated using the Jelesnianski typhoon model based on the values of the above typhoon parameters. These fields were then provided to FVCOM to simulate SSIF. In this study, the envelope diagram of inundation areas and inundation depths were selected as evaluation indicators for SSPIA (Liu et al. Citation2018). Matrix Laboratory (MATLAB, version 2021a) and GIS were used to convert FVCOM outputs into inundation ranges and inundation depths. First, MATLAB was used to read the FVCOM output and extract the maximum water levels of each triangular node. The elevation at each location was then subtracted to obtain the maximum inundation depth of each node. Second, the model outputs of different scenarios were transformed into flood extent and inundation depth in raster format using spatial interpolation techniques in GIS. Finally, the inundation area was classified into different hazard levels based on the water depth of each grid in the raster layer. shows the different hazard levels, the upper and lower limits of water depths corresponding to the hazard levels, and the colors marked in the hazard maps. As a result, hazard maps were obtained that provide information on the spatial extent and hazard level of SSIF associated with each typhoon scenario.
Table 2. Hazard levels classification.
3.2. Refined exposure estimation
To conduct a refined SSPIA, high-resolution socioeconomic gridded data are required, which can be achieved by using a refined exposure estimation method to downscale census data (Ye et al. Citation2019; Chen et al. Citation2021). In this study, two types of exposure were considered for SSPIA: population and three sub-sectors of GDP. The GDP categories are divided by differentiated characteristics of economic activities, with agricultural, forestry, livestock, and fisheries being included in the primary sector (GDP1), mining, manufacturing, and transportation being included in the secondary sector (GDP2), and service industries being included in the tertiary sector (GDP3). The mapping of high-resolution population/GDP gridded data was performed in three steps: selection of covariates, the establishment of the relationship between covariates and census population/GDP data using RF, and spatialization using RF and DMM.
A variety of covariates derived from remote sensing and social perception data were used to map high-resolution population/GDP gridded data. For gridded population mapping, covariates related to human activities, such as NTL, road density, LULC (i.e. built-up areas), and POIs were used along with other covariates, such as NDVI, DEM, and slope. For gridded GDP1 mapping, covariates potentially related to agricultural productivity, such as LULC (i.e. cropland rate, forest rate, grassland rate, and water rate), NDVI, DEM, slope, and distance to the coastline, were used. For gridded GDP2 mapping, covariates potentially related to industrial activities, such as industrial-related POIs (e.g. factories and mining companies), LULC (i.e. built-up areas), NTL, and road network density were used, along with other covariates, such as LST, DEM, and slope. For gridded GDP3 mapping, covariates potentially related to the service activity, such as service-related POIs (e.g. commercial buildings, restaurants, and banks), LULC (i.e. built-up regions), NTL, and road network density were used, along with other covariates, such as NDVI, LST, DEM, and slope.
Four RF models were then built to identify complex associations between various covariates and census population/GDP data. RF is a powerful machine-learning technique proposed by Breiman (Citation2001). In this study, the ‘randomForest’ package in R was used to fit and predict population/GDP density (Liaw and Wiener Citation2015). For each RF model, the raster layers of predictors at 30 m resolution were grouped by street or county and then combined with the natural logarithm of the census population/GDP density to fit the specific RF models. To predict the 30-m resolution population/GDP distribution weight layers, all of the fitted RF models were used along with the corresponding covariate raster layers. Then, the census population/GDP data were disaggregated by weight layers into 30 m resolution grids, and the fine-scale population/GDP distribution maps were obtained using formulas (2) and (3). The three subsectors of GDP were summed to obtain the summed GDP density map.
(2)
(2)
where WPOP_grid is the population distribution weight for a 30 × 30 m gridded area; WPOP_street is the summed population distribution weight of a street; POPstreet represents the street census population; and POPgrid is the distribution population for the gridded area.
(3)
(3)
where WGDP_grid is the GDP distribution weight for a 30 × 30 m gridded area; WGDP_county is the summed GDP-distribution weight of a county; GDPcounty represents the county census GDP; and GDPgrid is the distribution GDP for the gridded area.
3.3. Potential impacts estimation
The affected population/GDP was estimated using high-resolution gridded population/GDP data exposed to the flooded area. The casualty population was calculated using the following equations:
(4)
(4)
where i is the serial number of elements exposed to SSIF; CP(i) is the number of casualties in the study area due to SSIF; n is the total number of elements exposed to SSIF; POP(i) is the population number of the i-th element; hi is the inundation depth of the i-th element; F is the percentage of the population being damaged, generally calculated by the depth-casualty function provided by Jonkman and Vrijling (Citation2008), as follows:
(5)
(5)
where h is the inundation depth, m. We obtained casualty rate maps based on the envelope diagrams of inundation using the raster calculator in GIS and formula (5). The casualty rate maps were then multiplied by the population density map to obtain the number of casualties in each grid.
4. Results
4.1. Inundation hazard assessment
The envelope diagrams of inundation extent and inundation depth for five scenarios were used to perform the SSIF hazard assessment (see Figure A5). shows the SSIF hazard assessment maps showing the maximum inundation extent and hazard levels for different typhoon intensities. shows the statistics of inundated areas at different hazard levels for the designed typhoon scenarios. These data show that the maximum inundation depth and extent increase as the typhoon’s central air pressure decreases. At the moderate typhoon intensity (955 hPa), inundated areas are mainly along the coast and in locations with few exposed populations and assets. The total flooded area is 1.2483 km2, which is 2.11% of the LECZ (59.14 km2). These dikes can effectively protect most of the coastal areas during moderate typhoons (955 hPa).
Figure 5. Hazard maps of storm surges for designed typhoon scenarios: (a) 915 hPa, (b) 925 hPa, (c) 935 hPa, (d) 945 hPa, and (e) 955 hPa.

Figure 6. The histogram statistic presents the inundated areas at different hazard levels for the designed typhoon scenarios.

At the strong typhoon intensity (945 hPa), the inundated areas are mainly behind the Donggang Street dike, and most of the inundated areas are at low or relatively low hazard levels (1.6776 and 1.0476 km2). The total flooded area is 4.6899 km2, accounting for 7.92% of the LECZ. This is because the highest water level at the front of the Donggang Street dike exceeds the elevation of the corresponding part of the dike under strong typhoon influence (945 hPa). In addition, many LECZ are located behind the Donggang Street dike, causing some areas to be submerged. In contrast, the highest water level of Shengjiamen is lower than the highest tide level in front of Donggang, and the land elevation behind Shengjiamen Street dikes is higher than Donggang Street. As a result, only the back of the Donggang dike is flooded. These dikes cannot effectively protect the coastal areas along Donggang Street during strong typhoons (945 hPa). However, they can effectively protect other streets (i.e. Qiandao, Shengjiamen, and Lincheng streets).
At the strong typhoon intensity (935 hPa), Shenjiamen, Lincheng, and Qiandao streets also began to flood, and the total flooded area increased sharply to 34.6941 km2, accounting for 58.66% of the LECZ. The moderate and relatively high hazard levels begin to exceed the low hazard levels. At the super typhoon intensity (925 hPa), the total inundation area increased to 42.4512 km2, accounting for 71.78% of the LECZ. The proportion of relatively high hazard levels accounted for 41.94% of the total submerged area. At the super typhoon intensity (915 hPa), most of the low-lying coastal areas were submerged, with a total submerged area of 47.8953 km2, accounting for 80.98% of the LECZ. The high hazard level increased significantly, accounting for 32.11% of the total submerged area. Thus, these dikes cannot effectively protect most coastal areas during strong or super typhoons (≤945 hPa) that generate storm surges.
4.2. Refined exposure assessment
4.2.1. Refined population exposure
A high-resolution population density map with a resolution of 30 m was generated for the study area in 2020 using open access multi-source data, RF, and DMM (see ). The RF population model was rigorously validated using a 10-fold cross-validation approach. The validation results were within acceptable limits (see Table A7). As shown in , the population density is higher along the coastline compared to the low-density inland areas. In particular, the south coast of Zhoushan Island emerged as a major population concentration center. There are clear urban-rural differences in population distribution, with high densities observed in urban areas and decreasing densities in rural areas. For example, coastal urban areas had higher population densities than inland mountainous areas. The population density map identified four distinct population aggregation areas located behind the Donggang Street dike, along Shenjiamen Street, in the zones between Lincheng Street and Qiandao Street, and in the zones between Donggang Street and Shengjiamen Street. These results are consistent with the actual situation on the ground. The area with the highest population density (25 persons/900 m2) is located along Shengjiamen Street, which is known as the most famous fishing port and tourist destination.
4.2.2. Refined GDP exposure
Similarly, we used multi-source open data, RF and DMM to generate high-resolution GDP density maps with 30 m resolution for three different sectors in the study area for 2020 (see ). The RF models of GDP were rigorously validated using a 10-fold cross-validation approach. The validation results were within acceptable limits (see Table A7). As shown in , GDP density is higher along the coastline compared to inland areas where GDP density is low. As with the population distribution, there are significant urban-rural differences in GDP distribution. For example, on Zhoushan Island, most of the GDP is located in the central urban area along the south coast. The density maps of GDP2 and GDP3 are more spatially heterogeneous than the density maps of GDP1. The distribution of summed GDP has four distinct agglomerations like the population distribution, with high values located in the central business district of this city, which is consistent with the actual situation. The highest values for the three sectors are 0.004, 0.45, and 1.5 million CNY/900 m2, respectively. The highest total GDP value (1.69 million CNY/900 m2) is located in the above four different agglomerations.
4.3. Potential impacts of inundation
4.3.1. Potentially affected population
Due to rapid socio-economic development, coastal regions are experiencing population concentration. As typhoon intensity increases, a greater proportion of the population will be exposed to SSIF. Spatially explicit results from overlaying SSIF layers with the population density map are shown in . Using GIS, we overlaid the casualty rate maps with the population density map to obtain the number of population casualties.
Figure 9. Potentially affected population distributions in scenarios corresponding to : (a) 915 hPa, (b) 925 hPa, (c) 935 hPa, (d) 945 hPa, and (e) 955 hPa (unit: person/900 m2).

The potentially affected population under five flooding scenarios is shown in . As the typhoon intensity increases, so does the number of affected people. The number of potentially affected people at moderate typhoon intensity (955 hPa) is about 2587 (0.66% of the total population of the four streets). At super typhoon intensity (915 hPa), the number of people affected is about 259,516 (66% of the total population of the four streets)—about 100 times higher than at moderate typhoon intensity (955 hPa). In addition, under strong typhoon intensity (945 hPa), 41,781 people (31% of the total population of Donggang Street) are exposed to SSIF in Donggang Street. Using the depth-casualty function provided by Jonkman and Vrijling (Citation2008), it is calculated that at moderate typhoon intensity (955 hPa), there are more than 400 casualties; at super typhoon intensity (915 hPa), there are more than 3300 casualties, almost 8.25 times the lowest intensity typhoon scenarios.
4.3.2. Potentially affected GDP
Similarly, an increase in typhoon intensity leads to an increase in GDP exposure. and show the explicit spatial distribution and amount of potentially affected GDP, respectively. As typhoon intensity increases, so does the impact on GDP. In all five typhoon scenarios, GDP3 was the most affected, followed by GDP2 and GDP1. At moderate typhoon intensity (955 hPa), about 323.745 million CNY of GDP is affected by SSIF. At super typhoon intensity (915 hPa), about 20,178,898 million CNY of GDP is affected by SSIF—about 63 times higher than at moderate typhoon intensity (955 hPa).
Figure 11. Potentially affected summed GDP distributions in scenarios corresponding to : (a) 915 hPa, (b) 925 hPa, (c) 935 hPa, (d) 945 hPa, and (e) 955 hPa (unit: million CNY/900 m2).

Figure 12. The histogram statistic presents the affected GDP exposed to inundation for the designed typhoon scenarios.

The ratio of each affected sub-sector of GDP to the affected summed GDP is shown in . At four typhoon intensities (915, 925, 935, and 955 hPa), affected GDP1, GDP2, and GDP3 account for about 1, 34, and 65%, respectively. However, at strong typhoon intensity (945 hPa), affected GDP1, GDP2, and GDP3 account for about 2, 24, and 74%, respectively. This is because, at strong typhoon intensity (945 hPa), only areas behind Donggang dike are flooded, which is the core tourist area of Zhoushan City. As a result, the share of GDP3 related to tourism services increases, while the share of GDP2 related to industrial activities decreases.
5. Discussions
5.1. The comparison of the maximum water level at dikes
Previous studies (Zhilin et al. Citation2020; Li et al. Citation2022; Shi et al. Citation2021; Huang et al. Citation2023) have investigated the storm surge risk near Zhoushan but did not consider inundation scenes after flooding. In this study, we simulated SSIF using FVCOM and the Jelesnianski typhoon model. To better evaluate SSIF and identify dike gaps, we compared the dike height with the maximum water level in front of the dikes for each scenario using detailed dike data and ocean model results (). The maximum water levels in front of the Donggang Street dike tend to decrease from north to south, with higher typhoon intensity resulting in a larger difference. Meanwhile, the maximum water levels on the east and west sides of the Shenjiamen Street dike are higher than in the middle part, with higher typhoon intensity also resulting in a larger difference. This is consistent with the report content of the ‘Technical Report on Storm Surge Hazard Risk Assessment and Zoning in Putuo District’ by the Natural Resources and Planning Bureau of Zhoushan City.
Figure 14. Comparison of the maximum water level and dike elevation for the designed typhoon scenarios.

To evaluate the effectiveness of the dikes in protecting this study area from SSIF, we compared the dike height and the maximum water level. As shown in , these dikes can effectively protect most of the study area from SSIF at moderate typhoon intensity (955 hPa). However, at strong typhoon intensity (945 hPa), the maximum water level exceeds the elevation of the corresponding parts of dikes at three locations. Behind the Donggang Street dike, there are large areas of low-lying flat land, resulting in massive flooding. In contrast, Shenjiamen Street will not be flooded due to the high terrain behind the Shenjiamen Street dike. At strong or super typhoon intensity (935 or 925 hPa), the maximum water level in many places is higher than the dike, resulting in the inundation of large areas of Shenjiamen, Lincheng, and Qiandao Streets. Finally, at the super typhoon intensity (915 hPa), the maximum water level is mostly higher than the dike height, and almost all the dikes lose their protective function.
To improve the resilience of the study area to SSIF, we recommend raising the elevation of the dikes in Donggang Street and increasing the social resilience of tourism and service industries (i.e. GDP3) in Donggang Street to withstand SSIF. After increasing the resilience of Donggang Street to strong typhoon intensity (945 hPa), we should further increase the physical or social resilience of the whole area to strong/super typhoon intensity (915–935 hPa). For better management at the village/community administrative unit, we calculated the average potentially affected population/GDP for each village/community at super typhoon intensity (915 hPa) using the zonal statistics tool in GIS. We then created a zoning map of the potential risk level in the study area based on the natural break method in GIS. As shown in Figure A6, the local government should focus on increasing coastal flood resilience in eight high-risk villages (i.e. Shengshan, Laoqi, Xidang, Waigoushan, Pudong, Zhongnong, Luxi, and Luxisha villages).
5.2. Uncertainty of exposure map
Quantifying disaster risk is fundamental to flood risk assessment. Some studies (Boyd Citation2010; Tang et al. Citation2013; Yan et al. Citation2016; Lianxiao et al. Citation2022) have directly used census data to investigate the potential impact of floods on the population. This is the most convenient method. However, census data do not provide detailed information on the spatial distribution of the population. In contrast, open-access datasets (e.g. WorldPop) are currently the most widely used population grid data in large-scale flood assessment studies (Tatem Citation2017; Kulp and Strauss Citation2019; Smith et al. Citation2019). However, there is no international standard for coastlines, and the administrative boundaries used in open-access datasets may not strictly match the physical reality of the coastline (Mcleod et al. Citation2010). This may result in the open-access dataset failing to distribute the population in areas near the coastline. Aligning the input spatial layers is paramount (Merz et al. Citation2010). That is, for estimation in coastal flood hazard assessment, it is important to align population density data with inundation layers in coastal areas. This is particularly important for the small island study area, where most of the land area is near the sea.
As shown in Figure A7, we estimated the potentially affected population for five SSIF scenarios using uniformly distributed 30 m resolution census data (CensusPop), 100 m resolution WorldPop and the 30 m resolution gridded population data used in this study (PoiPop). The potentially affected populations for all five scenarios for the four Streets estimated using CensusPop and WorldPop are similar. The affected populations estimated using CensusPop and WorldPop are smaller than those estimated using PoiPop. The main reason for the underestimation of the affected population estimated by CensusPop is that the non-homogeneity of the population distribution is not taken into account. This can lead to an underestimation of population density in densely populated coastal communities or commercial areas. There are two reasons why the affected populations predicted by WorldPop are underestimated. First, there is a significant difference between the total population of WorldPop and the actual total population on each street. We compared the total population from the latest 7th Census with the total population of different townships or streets in WorldPop (Figure A8) and found large discrepancies in the populations of Shenjiamen, Donggang, and Qiandao streets. Second, the administrative boundary data in WorldPop do not match the actual coastline. As shown in Figure A9, there is a large population deficit in the newly reclaimed area behind the embankment of Donggang Street. In summary, the multidisciplinary framework of combining the ocean model and the refined exposure estimation methods for potential impact assessment in this study can improve the underestimation of potential impacts caused by the direct use of census data or open data.
5.3. Limitations
Our results are subject to several uncertainties arising from the flood modeling, exposure elements, and vulnerability analysis. First, different parameter settings, such as mesh resolution, land elevation, local bathymetry, and typhoon parameters, may lead to variations and uncertainties in hazard results (Lin and Chavas Citation2012; Chu et al. Citation2019). In fact, a DEM with structures and buildings removed can lead to an overestimation of flooding. A more detailed Digital Surface Model (including buildings and structures) would contribute to a more realistic flood simulation (Li et al. Citation2021). Besides, the construction of dikes may make the damage worse. Dikes may block water from draining and capture behind them (Ke et al. Citation2021). In addition, precipitation runoff, rising waves, and more can also affect inundation levels during typhoon events (Pandey et al. Citation2021). Second, the use of a probabilistic approach to determine typhoon intensity will help in the analysis of expected annual damages, as opposed to the use of specific typhoon levels (Wang, Mu, Qi, et al. Citation2021). Third, the results of population/GDP spatialization will vary depending on the forecast model and covariates (Yang et al. Citation2019). Uncertainty can be reduced by using fine-resolution population/GDP data based on novel social perception data (Chaoxu et al. Citation2019). Fourth, a major source of uncertainty is the depth-casualty function. This curve should be further calibrated with more localized damage data. Due to the lack of vulnerability curves for GDP in the economic risk assessment, we only calculated values of GDP exposure to different levels of SSIF. We will consider these issues as future work.
6. Conclusions
The frequency and intensity of SSIF are expected to increase due to climate change and sea level rise. As economic activities and assets in coastal cities continue to grow, the risks posed by SSIF are also increasing. However, there are few studies on refined SSPIA in China due to the lack of high spatial resolution exposure element datasets. This study proposed a multidisciplinary framework for refined SSPIA using FVCOM with the Jelesnianski typhoon model and a refined exposure estimation method. Zhoushan City is an exemplary case study due to its large amount of port and tourist attractions and high population density. Its capabilities and performance were demonstrated in this exemplary case study.
The spatially explicit assessment results show that dikes can effectively reduce risk at moderate typhoon intensity (955 hPa), but not at strong or super typhoon intensity (915–945 hPa). Some parts of the densely populated communities in the east of Donggang Street were at high and very high risk for the strong typhoon intensity (945 hPa). In addition, the strong or super typhoon intensity scenario (915–935 hPa) induced a very high risk to the entire coastal area. The results of the impact estimation statistics show that even at moderate typhoon intensity (955 hPa), more than 2587 people were exposed to flooding. At super typhoon intensity (915 hPa), the affected population reached about 259,516 people—about 100 times higher than at moderate typhoon intensity. Similarly, at moderate typhoon intensity (955 hPa), flooding affected about 323.745 million CNY of GDP. At super typhoon intensity (915 hPa), about 20,178,898 million CNY of GDP is affected by SSIF—about 63 times higher than at moderate typhoon intensity.
Our study provides a refined pattern for estimating potential impacts, which has significant implications for risk assessment of climate change adaptation and flood resilience. It is expected that the approach and modeling tools proposed in this study, together with the predictions for Lincheng, Qiandao, Donggang, and Shengjiamen Streets, will be useful for future evacuation and flood risk reduction planning. This multidisciplinary framework will apply to all coastal flood-prone areas around the world for a more refined assessment of the potential impacts of SSIF.
Supplemental Material
Download MS Word (20.5 MB)Acknowledgments
The authors would like to thank the comments and suggestions by anonymous reviewers for improving the original manuscript.
Data availability statement
All relevant data and their access are in the manuscript and its Supplementary Material files.
Additional information
Funding
References
- Amadio M, Mysiak J, Carrera L, Koks E. 2016. Improving flood damage assessment models in Italy. Nat Hazards. 82(3):2075–2088. doi: 10.1007/s11069-016-2286-0.
- Aznar-Siguan G, Bresch DN. 2019. CLIMADA v1: a global weather and climate risk assessment platform. Geosci Model Dev. 12(7):3085–3097. doi: 10.5194/gmd-12-3085-2019.
- Boyd EC. 2010. Estimating and mapping the direct flood fatality rate for flooding in greater New Orleans due to Hurricane Katrina. Risk Hazards Crisis Public Policy. 1(3):87–110. doi: 10.2202/1944-4079.1017.
- Breiman L. 2001. Random forests. Mach Learn. 45(1):5–32. doi: 10.1023/A:1010933404324.
- Chakraborty L, Rus H, Henstra D, Thistlethwaite J, Minano A, Scott D. 2022. Exploring spatial heterogeneity and environmental injustices in exposure to flood hazards using geographically weighted regression. Environ Res. 210:112982. doi: 10.1016/j.envres.2022.112982.
- Chan JC. 1984. An observational study of the physical processes responsible for tropical cyclone motion. J Atmos Sci. 41(6):1036–1048. doi: 10.1175/1520-0469(1984)041<1036:AOSOTP>2.0.CO;2.
- Chaoxu X, Gaozhong N, Xiwei F, Junxue Z, Xiaoke P. 2019. Research on the application of mobile phone location signal data in earthquake emergency work: a case study of Jiuzhaigou earthquake. PLOS One. 14(4):e0215361. doi: 10.1371/journal.pone.0215361.
- Chen C,Liu H,Beardsley RC. 2003. An unstructured grid, finite-volume, three-dimensional, primitive equations ocean model: application to coastal ocean and estuaries. J Atmos Oceanic Technol. 20(1):159–186. 10.1175/1520-0426(2003)020<0159:AUGFVT>2.0.CO;2.(Chen et al., 2003)
- Chen C, Qi J, Li C, Beardsley RC, Lin H, Walker R, Gates K. 2008. Complexity of the flooding/drying process in an estuarine tidal‐creek salt‐marsh system: an application of FVCOM. J Geophys Res. 113(C7):C07052. doi: 10.1029/2007JC004328.
- Chen Q, Ye T, Zhao N, Ding M, Ouyang Z, Jia P, Yue W, Yang X. 2021. Mapping China’s regional economic activity by integrating points-of-interest and remote sensing data with random forest. Environ Plan B Urban Anal City Sci. 48(7):1876–1894. doi: 10.1177/2399808320951580.
- Cheung KF, Tang L, Donnelly JP, Scileppi EM, Liu KB, Mao XZ, Houston SH, Murnane RJ. 2007. Numerical modeling and field evidence of coastal overwash in southern New England from Hurricane Bob and implications for paleotempestology. J Geophys Res. 112(F3):F03024. doi: 10.1029/2006JF000612.
- Chu D, Zhang J, Wu Y, Jiao X, Qian S. 2019. Sensitivities of modelling storm surge to bottom friction, wind drag coefficient, and meteorological product in the East China Sea. Estuar Coastal Shelf Sci. 231:106460. doi: 10.1016/j.ecss.2019.106460.
- Dabbeek J, Crowley H, Silva V, Weatherill G, Paul N, Nievas CI. 2021. Impact of exposure spatial resolution on seismic loss estimates in regional portfolios. Bull Earthquake Eng. 19(14):5819–5841. doi: 10.1007/s10518-021-01194-x.
- Deng Z, Hu S, Tang L, Jiang L, He J, Shen K, Xu Y, Jiang R, Li T, Chen C, et al. 2023. Carbazole and polyhalogenated carbazoles in the marine environment around the Zhoushan Archipelago: distribution characteristics, environmental behavior, and sources. J Hazard Mater. 442:129999. doi: 10.1016/j.jhazmat.2022.129999.
- Dietrich JC, Tanaka S, Westerink JJ, Dawson CN, Luettich RA, Zijlema M, Holthuijsen LH, Smith JM, Westerink LG, Westerink HJ. 2012. Performance of the unstructured-mesh, SWAN + ADCIRC model in computing hurricane waves and surge. J Sci Comput. 52(2):468–497. doi: 10.1007/s10915-011-9555-6.
- Dobson JE, Bright EA, Coleman PR, Durfee RC, Worley BA. 2000. LandScan: a global population database for estimating populations at risk. Photogramm Eng Remote Sens. 66(7):849–857.
- Egbert GD, Erofeeva SY. 2002. Efficient inverse modeling of barotropic ocean tides. J Atmos Oceanic Technol. 19(2):183–204. doi: 10.1175/1520-0426(2002)019<0183:EIMOBO>2.0.CO;2.
- Fang J, Liu W, Yang S, Brown S, Nicholls RJ, Hinkel J, Shi X, Shi P. 2017. Spatial-temporal changes of coastal and marine disasters risks and impacts in Mainland China. Ocean Coastal Manag. 139:125–140. doi: 10.1016/j.ocecoaman.2017.02.003.
- Feng A, Gao J, Wu S, Liu L, Li Y, Yue X. 2018. Assessing the inundation risk resulting from extreme water levels under sea-level rise: a case study of Rongcheng, China. Geomatics Nat Hazards Risk. 9(1):456–470. doi: 10.1080/19475705.2018.1447026.
- Fujii T. 1998. Statistical analysis of the characteristics of severe typhoons hitting the Japanese main islands. Mon Weather Rev. 126(4):1091–1097. doi: 10.1175/1520-0493(1998)126<1091:SAOTCO>2.0.CO;2.
- Ge J, Chen C, Qi J, Ding P, Beardsley RC. 2012. A dike–groyne algorithm in a terrain-following coordinate ocean model (FVCOM): development, validation and application. Ocean Modell. 47:26–40. doi: 10.1016/j.ocemod.2012.01.006.
- He Z, Tang Y, Xia Y, Chen B, Xu J, Yu Z, Li L. 2020. Interaction impacts of tides, waves and winds on storm surge in a channel-island system: observational and numerical study in Yangshan Harbor. Ocean Dyn. 70(3):307–325. doi: 10.1007/s10236-019-01328-5.
- Huang J, Hu X, Ding J, Gui J, Zhang R. 2023. Berthing capacity evaluation of fishing port prone to typhoons: a case study of Shengsi fishing port. J Waterway Port Coastal Ocean Eng. 149(2):05022006. doi: 10.1061/JWPED5.WWENG-1913.
- Jelesnianski CP. 1965. A numerical calculation of storm tides induced by a tropical storm impinging on a continental shelf. Mon Weather Rev. 93(6):343–358. doi: 10.1175/1520-0493(1993)093<0343:ANCOS>2.3.CO;2.
- Jonkman SN, Vrijling JK. 2008. Loss of life due to floods. J Flood Risk Manag. 1(1):43–56. doi: 10.1111/j.1753-318X.2008.00006.x.
- Ke Q, Yin J, Bricker JD, Savage N, Buonomo E, Ye Q, Visser P, Dong G, Wang S, Tian Z, et al. 2021. An integrated framework of coastal flood modelling under the failures of sea dikes: a case study in Shanghai. Nat Hazards. 109(1):671–703. doi: 10.1007/s11069-021-04853-z.
- Kulp SA, Strauss BH. 2019. New elevation data triple estimates of global vulnerability to sea-level rise and coastal flooding. Nat Commun. 10(1):1–12.
- Li L, Li Z, He Z, Yu Z, Ren Y. 2022. Investigation of storm tides induced by super typhoon in macro-tidal Hangzhou Bay. Front Mar Sci. 9:970. doi: 10.3389/fmars.2022.890285.
- Li W, Liu B, Hu P, He Z, Zou J. 2021. Porous shallow water modeling for urban floods in the Zhoushan City, China. Front Earth Sci. 9:687311. doi: 10.3389/feart.2021.687311.
- Liang X, Chan JC. 2005. The effects of the full Coriolis force on the structure and motion of a tropical cyclone. Part I: effects due to vertical motion. J Atmos Sci. 62(10):3825–3830. doi: 10.1175/JAS3545.1.
- Lianxiao, Morimoto T, Siqin T, An C, Yuhai B, Fei G. 2022. Evaluating regional flood disaster resilience based on the DROP model and TOPSIS method: a case study of the Katsushika Ward, Tokyo. Nat Hazards Rev. 23(3):04022018. doi: 10.1061/(ASCE)NH.1527-6996.0000551.
- Liaw A, Wiener M. 2015. randomForest: Breiman and Cutler’s random forests for classification and regression. R Package Version. 4:14.
- Li Y, Fang W, Lin W, Ye Y. 2014. Parameterization of synthetic tropical cyclones at various scales for probable maximum storm surge risk modeling. Mar Sci. 38(4):71–80.
- Lin N, Chavas D. 2012. On hurricane parametric wind and applications in storm surge modeling. J Geophys Res. 117(D9):D09120. doi: 10.1029/2011JD017126.
- Liu Q, Shi X, Guo Z. 2018. Technical guidelines for risk assessment and zoning of marine disaster part 1: storm surge. Beijing: Standards Press of China.
- Liu J, Hertel TW, Diffenbaugh NS, Delgado MS, Ashfaq M. 2015. Future property damage from flooding: sensitivities to economy and climate change. Clim Change. 132(4):741–749. doi: 10.1007/s10584-015-1478-z.
- Lu X, Yu H, Ying M, Zhao B, Zhang S, Lin L, Bai L, Wan R. 2021. Western North Pacific tropical cyclone database created by the China Meteorological Administration. Adv Atmos Sci. 38(4):690–699. doi: 10.1007/s00376-020-0211-7.
- Ma X, de Jong M, Sun B, Bao X. 2020. Nouveauté or Cliché? Assessment on island ecological vulnerability to tourism: application to Zhoushan, China. Ecol Indic. 113:106247. doi: 10.1016/j.ecolind.2020.106247.
- Mcleod E, Poulter B, Hinkel J, Reyes E, Salm R. 2010. Sea-level rise impact models and environmental conservation: a review of models and their applications. Ocean Coastal Manag. 53(9):507–517. doi: 10.1016/j.ocecoaman.2010.06.009.
- Merz B, Kreibich H, Schwarze R, Thieken A. 2010. Review article: assessment of economic flood damage. Nat Hazards Earth Syst Sci. 10(8):1697–1724. doi: 10.5194/nhess-10-1697-2010.
- Molinari D, De Bruijn KM, Castillo-Rodríguez JT, Aronica GT, Bouwer LM. 2019. Validation of flood risk models: current practice and possible improvements. Int J Disaster Risk Reduct. 33:441–448. doi: 10.1016/j.ijdrr.2018.10.022.
- Mondal P, Tatem AJ. 2012. Uncertainties in measuring populations potentially impacted by sea level rise and coastal flooding. PLOS One. 7(10):e48191. doi: 10.1371/journal.pone.0048191.
- Nayak S, Takemi T. 2023. Statistical analysis of the characteristics of typhoons approaching Japan from 2006 to 2019. Geomatics Nat Hazards Risk. 14(1):2208722. doi: 10.1080/19475705.2023.2208722.
- Pandey S, Rao AD, Haldar R. 2021. Modeling of coastal inundation in response to a tropical cyclone using a coupled hydraulic HEC‐RAS and ADCIRC model. J Geophys Res Oceans. 126(7):e2020JC016810. doi: 10.1029/2020JC016810.
- Rowley RJ, Kostelnick JC, Braaten D, Li X, Meisel J. 2007. Risk of rising sea level to population and land area. Eos Trans AGU. 88(9):105–107. doi: 10.1029/2007EO090001.
- Scawthorn C, Blais N, Seligson H, Tate E, Mifflin E, Thomas W, Murphy J, Jones C. 2006. HAZUS-MH flood loss estimation methodology. I: overview and flood hazard characterization. Nat Hazards Rev. 7(2):60–71. doi: 10.1061/(ASCE)1527-6988(2006)7:2(60).
- Shepard CC, Agostini VN, Gilmer B, Allen T, Stone J, Brooks W, Beck MW. 2012. Assessing future risk: quantifying the effects of sea level rise on storm surge risk for the southern shores of Long Island, New York. Nat Hazards. 60(2):727–745. doi: 10.1007/s11069-011-0046-8.
- Shi X, Chen B, Liang Y, Zhang B, Ye T. 2021. Inundation simulation of different return periods of storm surge based on a numerical model and observational data. Stoch Environ Res Risk Assess. 35(10):2093–2103. doi: 10.1007/s00477-021-02010-3.
- Shi X, Yu P, Guo Z, Sun Z, Chen F, Wu X, Cheng W, Zeng J. 2020. Simulation of storm surge inundation under different typhoon intensity scenarios: case study of Pingyang County, China. Nat Hazards Earth Syst Sci. 20(10):2777–2790. doi: 10.5194/nhess-20-2777-2020.
- Smith A, Bates PD, Wing O, Sampson C, Quinn N, Neal J. 2019. New estimates of flood exposure in developing countries using high-resolution population data. Nat Commun. 10(1):1814. doi: 10.1038/s41467-019-09282-y.
- Tang HS, Chien SIJ, Temimi M, Blain CA, Ke Q, Zhao L, Kraatz S. 2013. Vulnerability of population and transportation infrastructure at the east bank of Delaware Bay due to coastal flooding in sea-level rise conditions. Nat Hazards. 69(1):141–163. doi: 10.1007/s11069-013-0691-1.
- Tatem AJ. 2017. WorldPop, open data for spatial demography. Sci Data. 4(1):1–4. doi: 10.1038/sdata.2017.4.
- Wang J, Yi S, Li M, Wang L, Song C. 2018. Effects of sea level rise, land subsidence, bathymetric change and typhoon tracks on storm flooding in the coastal areas of Shanghai. Sci Total Environ. 621:228–234. doi: 10.1016/j.scitotenv.2017.11.224.
- Wang K, Yang Y, Reniers G, Huang Q. 2021a. A study into the spatiotemporal distribution of typhoon storm surge disasters in China. Nat Hazards. 108(1):1237–1256. doi: 10.1007/s11069-021-04730-9.
- Wang K, Yang Y, Reniers G, Huang Q. 2021b. Understanding spatiotemporal patterns of typhoon storm surge disasters based on their tropical cyclone track clusters in China. Geomatics Nat Hazards Risk. 12(1):2736–2754. doi: 10.1080/19475705.2021.1973120.
- Wang S, Mu L, Yao Z, Gao J, Zhao E, Wang L. 2021. Assessing and zoning of typhoon storm surge risk with a geographic information system (GIS) technique: a case study of the coastal area of Huizhou. Nat Hazards Earth Syst Sci. 21(1):439–462. doi: 10.5194/nhess-21-439-2021.
- Wang S, Mu L, Qi M, Yu Z, Yao Z, Zhao E. 2021. Quantitative risk assessment of storm surge using GIS techniques and open data: a case study of Daya Bay Zone, China. J Environ Manage. 289:112514. doi: 10.1016/j.jenvman.2021.112514.
- Wang X, Sutton PC, Qi B. 2019. Global mapping of GDP at 1 km2 using VIIRS nighttime satellite imagery. Int J Geo-Inf. 8(12):580. doi: 10.3390/ijgi8120580.
- Wang Y, Guo Z, Zheng S, Zhang M, Shu X, Luo J, Qiu L, Gao T. 2021. Risk assessment for typhoon-induced storm surges in Wenchang, Hainan Island of China. Geomatics Nat Hazards Risk. 12(1):880–899. doi: 10.1080/19475705.2021.1899060.
- Williams LL, Lück-Vogel M. 2020. Comparative assessment of the GIS based bathtub model and an enhanced bathtub model for coastal inundation. J Coast Conserv. 24(2):23. doi: 10.1007/s11852-020-00735-x.
- Xianwu S, Ziqiang H, Jiayi F, Jun T, Zhixing G, Zhilin S. 2020. Assessment and zonation of storm surge hazards in the coastal areas of China. Nat Hazards. 100(1):39–48. doi: 10.1007/s11069-019-03793-z.
- Yan B, Li S, Wang J, Ge Z, Zhang L. 2016. Socio-economic vulnerability of the megacity of Shanghai (China) to sea-level rise and associated storm surges. Reg Environ Change. 16(5):1443–1456. doi: 10.1007/s10113-015-0878-y.
- Yang X, Yao C, Chen Q, Ye T, Jin C. 2019. Improved estimates of population exposure in low-elevation coastal zones of China. Int J Environ Res Public Health. 16(20):4012. doi: 10.3390/ijerph16204012.
- Ye T, Zhao N, Yang X, Ouyang Z, Liu X, Chen Q, Hu K, Yue W, Qi J, Li Z, et al. 2019. Improved population mapping for China using remotely sensed and points-of-interest data within a random forests model. Sci Total Environ. 658:936–946. doi: 10.1016/j.scitotenv.2018.12.276.
- Yin J, Lin N, Yang Y, Pringle WJ, Tan J, Westerink JJ, Yu D. 2021. Hazard assessment for typhoon‐induced coastal flooding and inundation in Shanghai, China. J Geophys Res Oceans. 126(7):e2021JC017319. doi: 10.1029/2021JC017319.
- Yin J, Yu D, Yin Z, Wang J, Xu S. 2013. Modelling the combined impacts of sea-level rise and land subsidence on storm tides induced flooding of the Huangpu River in Shanghai, China. Clim Change. 119(3–4):919–932. doi: 10.1007/s10584-013-0749-9.
- Yu F, Fu C, Guo H, Liu Q. 2020. Modern technologies and application in storm surge forecasting. Beijing: Science Press.
- Zhang X, Pan D, Chen J, Zhao J, Zhu Q, Huang H. 2014. Evaluation of coastline changes under human intervention using multi-temporal high-resolution images: a case study of the Zhoushan Islands, China. Rem Sens. 6(10):9930–9950. doi: 10.3390/rs6109930.
- Zhilin S, Shanhong Z, Chen W. 2020. Simulation and analysis of storm surge at Zhoushan fishing port. Haiyang Xuebao. 42(1):136–143.