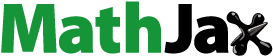
Abstract
To prevent water inrush hazards of mining under the Quaternary unconsolidated confined aquifer in North China coalfield, it is necessary to build a water inrush prediction model to guarantee coal safety production. The current method of evaluating mining hazards under unconsolidated confined aquifers is easy to fall into the problems of simple superposition of evaluation factors, large subjectivity of the factor selection, and low accuracy of prediction results. In this paper, an analytic hierarchy process (AHP) is improved according to contribution of information value method (IVM) to analyze the impact of water inrush factors. The factor weight values are considered in calculating the total information value of IVM to build a coupled AHP-IVM model. The information value of each factor is calculated through IVM, and area under curve (AUC) value is used to rank the factors, to exclude the subjective judgment of the importance of each factor. The geological and hydrogeological data of Qinan coal mine in Huaibei coalfield are analyzed and organized. The results suggest that the coupled AHP-IVM model has an AUC value of 0.8309, indicating its great potential and practical significance for future mining operations.
1. Introduction
A layer of unconsolidated confined aquifer composed of sandy soil and gravel as the frame exists at the bottom of the Quaternary unconsolidated soils in the concealed coalfields of North China. The anisotropic aquifer is directly covered on the top of the coal seam. The presence of such aquifers increases the risk of hydrogeological hazards (e.g. water inrush hazards) which is considered as one of the most important issues threatening the safe coal production in north China (Khan et al. Citation2018, Citation2020). It is the most important problem threatening the safety of coal mining operations (Wu Q and Zhou Citation2008; Wang XZ Citation2012; Sun WJ et al. Citation2016; Wu Q et al. Citation2017; Wang F et al. Citation2019; Xue et al. Citation2022; Xue et al. Citation2023). It is crucial to establish a scientific and practical model for predicting water inrush in mining under the unconsolidated confined aquifer. Reducing water inrush can effectively improve the efficiency of coal mining.
To effectively predict the water inrush hazard under unconsolidated confined aquifers, many experts have studied mining under unconsolidated confined aquifers controlled by various factors in recent years. For instance, Wu Q et al. (Citation2016) proposed three maps-two predictions method. Xu JL et al. (Citation2009) and Xu W et al. (Citation2013) concluded that water inrush hazard is related to overlying strata failure and proposed a method of predicting the water inrush hazard zone. Chen LW et al. (Citation2016) applied the multi-information superposition method to determine the water inrush areas. Lu et al. (Citation2019) proposed multi-type-four-double (MTFD) evaluation technology to predict water inrush from the roof. Ma, Duan, Zhang (Citation2022), Ma et al. (Citation2022) and (Li et al. Citation2015) proposed a one-dimensional radial three-phase flow model to predict water–silt inrush hazard of fault rock by numerical simulation. By GIS technology and entropy weight theory, a multi-source information evaluation method for the risk of water inrush from coal floor was proposed and verified by Li, Zhang, Luo, et al. (Citation2022) and Li, Zhang, Long, et al. (Citation2022). Zhang and Wang proposed a novel off-the-shelf two-step assessment approach including Fisher discriminant analysis and classification algorithm on the same transformed low-dimensional data to predict the water abundance level (Zhang and Wang Citation2021). No single method can address all issues associated with the water inrush phenomenon. Therefore, the prediction accuracy of water inrush risk can be effectively improved by combining different methods, and it is a crucial research direction to use AHP method combined with other methods for water inrush assessment.
AHP method is an effective tool to solve complex decision-making problems for multi-objectives, which is commonly used to calculate the weight of different factors as well as to combine it with other methods to assess water inrush risk. Wang et al. and Yang et al. (2012, Citation2017) constructed a secondary fuzzy comprehensive evaluation system and considered the impact of various factors on the water inrush from qualitatively to quantitatively. Li LP et al. (Citation2015) proposed an evaluation system of attribute synthetic, which comprehensively considered the weight and the membership of factors. Li B and Chen (Citation2016) put forward a coupled model based on the grey relational analysis (GRA) method and AHP method. Ruan et al. (Citation2019) thought the judgment matrix of AHP method determined by personal experience has great variations or even errors. Wang Q et al. (Citation2022) evaluated the results obtained from the certainty factor (CF) and logistic regression (LR) models for estimating water-inrush risk. Li JL et al. (Citation2021) established the fractal-AHP-VI model of floor water inrush based on the fractal theory. The combined weight (CW) method and the fuzzy matter-element analysis (FMEA) method were used to analyze and evaluate the control effect of subsiding land by Wang ZY et al. (Citation2021).
However, there are still some limitations to these coupled models, which do not emphasize the influence of geological background on each evaluation factor. Therefore, it is necessary to put forward an objective judgment method to compare the impact of each factor under specific geological background, and then improve AHP method and reduce the error caused by individual subjective judgment. In this study, IVM is selected as the effective method to improve AHP method.
Its advantage lies in the evaluation of AHP factors through IVM to obtain the weight of each factor, so that the importance of factors is not affected by subjectivity. IVM can transform the measured factor affecting the research object into the information value and evaluate the correlation between factor and research object, which can effectively reflect the influence on water inrush risk under a specific geological background (Wu Q et al. Citation2011; Hu et al. Citation2019).
Existing AHP methods still need to rely on personal experience to construct the judgment matrix. If ranking is based on experience, it is easy to be preconceived or poorly considered, resulting in inaccurate prediction results. Under different geological environments, the impact of each factor is different. The importance of the factors involved in the risk assessment was ranked using the IVM method. Subjective errors of the judgment matrix can be avoided by using the IVM method. This paper proposes a coupled model to evaluate the water inrush risk by the improved AHP method, and applies it to Qinan coal mine to verify the effectiveness of this model.
2. Hydrogeological and geological setting
The Huaibei coalfield is located in the northern part of Anhui Province, China, bordering Jiangsu and Henan Provinces in the northeast and west, respectively (). The multi-stage tectonic movements of Indosinian, Early Yanshanian and Late Yanshanian are superimposed, resulting in a series of complex network patterns of EW, NNE and NE trending structures interwoven in the study area, such as Banqiao-Guzhen fault, Subei fault, Xiayi fault, Fengwo fault and Guzhen-Changfeng fault () (Zhang et al. Citation2020).
Figure 1. Regional geological structure of the Qinan coal mine in the Huaibei coalfield (a) Location of the Huaibei coalfield; (b) Regional geological structure of the Huaibei coalfield; (c) Regional geological structure of the Qinan coal mine.

The Qinan coal mine is located in the southeast of Huaibei coalfield, which is a typical North China-type coal mine, and its mineable coal seams are directly covered by Cenozoic loose strata (). Constrained by the Sunan syncline, the Qinan coal mine is a monoclinic structure that strikes north–south and slopes eastward with a dip angle of about 15°. Furthermore, normal faults are the main structural features in the study area, accompanied by small-scale folds (). The coal mine covers an area of 35 km2 and contains three main minable coal seams named No. 32, 72 and 10, respectively.
According to the lithology, thickness, water-bearing medium and burial conditions of the formation, the unconsolidated Cenozoic formation can be divided into three aquifuges and four aquifers from top to bottom, which are the first aquifer, the first aquifuge, the second aquifer, the second aquifuge, the third aquifer, the third aquifuge and the fourth aquifer, respectively (). The physical parameters and hydrological parameters of the rock stratum are shown in . The parameters of these aquifers can be found in . The fourth aquifer is directly covered on the coal strata. The location and depth of water inrush points are shown in . Since the third aquiclude develops steadily and has excellent water-proof performance in the region, the fourth aquifer and the lower aquifers have no direct hydraulic connection with meteoric water and surface water under normal circumstances. The fourth aquifer usually has good water storage capacity, showing the characteristics of high pressure and strong seepage. Meanwhile, this aquifer directly overlays the Permian strata, which is the main source of water-inrush for shallow coal resources mining. As the research object of this paper, the coal seams under the unconsolidated confined aquifers in Qinan coal mine are No. 32, 72. According to the hydrogeological account of Qinan coal mine, there have been 21 water-inrush accidents during mining under the unconsolidated and confined aquifer. Therefore, a reasonable and effective water inrush risk assessment is of great significance to ensure safe mining operations.
Table 1. The physical parameters and hydrological parameters of the rock stratum.
Table 2. The parameters of the aquifers.
Table 3. The location and depth of water inrush points.
3. AHP method and its improvement
3.1. AHP method
AHP is an analytical method that combines qualitative and quantitative analysis to conduct a risk assessment and has been widely used in the coal mine field (Ruan et al. Citation2019). The weight value of each factor is calculated by AHP method, and the calculation process can be divided into four steps:
Establishing a comprehensive evaluation system of factors.
Constructing the judgment matrix D using the 1–9 scales, as shown in EquationEquation 1
(1)
(1) .
(1)
(1) Where xmn is the comparing result of the importance of the m th factor and the n th factor (Saaty Citation1980).
Consistency test of the judgment matrix, as shown in EquationEquations 2
(2)
(2) and Equation3
(3)
(3) .
(2)
(2)
(3)
(3) Where CR is the random consistency ratio, CI is the consistency check index, RI is the average random consistency index (), λmax is the largest eigenvalue of the judgment matrix, and n is the quantity of the assessment indices (Saaty Citation1980).
The consistency ratio (CR) is obtained by comparing the CI with the appropriate one of the following set of numbers, each of which is an average random consistency index (RI) derived from a sample randomly generated reciprocal matrix using the scale l/9, l/8, …, 1, . .8, 9 to see if it is about 0.10 or less. Saaty mentioned that the CR of less than 0.1 will be acceptable (Saaty Citation1980).
When CR < 0.1, the judgment matrix satisfies the consistency test; otherwise, the judgment matrix needs to be adjusted.
Under the condition that the judgment matrix satisfies the consistency test, the weight vector of the evaluation factors can be calculated.
Table 4. Values of random consistency index.
3.2. The shortcomings of AHP method and its improvement
AHP method quantifies the subjective judgment relationship between the indexes by means of the 1–9 scale method proposed by Saaty, constructs the quantity judgment matrix and makes consistency test (Saaty Citation1980). However, decision-makers assessed based on their professional knowledge, experience, and subjective judgment. Therefore, there have been many improved methods proposed to reduce the search costs caused by the original judgment matrix. Ruan et al. (Citation2019) used Dempster-Shafer (D–S) synthetic rule to improve the 1–9 scales method, which took full advantage of each expert’s opinion and reduced the subjective error caused by an individual decision-maker. Shi et al. (Citation2020) calculated the integrated weight by combining AHP with the entropy weight method. The reliability of the total weight is better than that calculated by the original judgment matrix. However, it is difficult to quantify the influence of geological conditions and establish risk-grade standards of water inrush. In general, the impact of each evaluation factor on water inrush is different due to the complexity of geological conditions.
The receiver operating characteristic curve (ROC) is a graphical technique used to assess the accuracy of model. AUC is defined as the area enclosed by the coordinate axis under ROC. The closer AUC is to 1.0, the higher the authenticity of the detection method is. When it is equal to 0.5, it has the lowest authenticity and no application value. The larger AUC is, the greater contribution of this factor to water inrush is (Fan et al. Citation2014).The subjective judgment of AHP method mainly depend on the division of the importance of different factors. In this study, the information value of each factor is calculated through IVM, and the value of AUC is used to rank the factors, to exclude the subjective judgment of the importance of each factor. In order to determine the importance of various evaluation factors on water inrush, the success rate curve method is selected to combine with IVM (Fan et al. Citation2014). The horizontal axis represents the area percentage of the information value from high to low, while the vertical axis represents the proportion of the water inrush hazards occurring within the corresponding information value. The larger AUC value is, the greater the importance of the factor has on water inrush. According to AUC value, the importance of factors on water inrush can be divided into four categories, named relatively important (I), medium important (II), important (III), absolutely important (IV), respectively, as shown in EquationEquation 3(3)
(3) as follows:
(4)
(4)
Where Xi is the evaluation factor, xi is AUC value of Xi, d1, d2, d3 is the classification threshold and calculated by EquationEquation 5(5)
(5) as follows:
(5)
(5)
Where xmax and xmin are the maximum and the minimum AUC value of the evaluation factor, respectively.
Based on this classification, the importance of the evaluation factors can be compared objectively, and the degree of importance is shown in .
Table 5. Comparison Table of importance degree.
3.3. IVM method
IVM developed from information theory is an effective quantitative evaluation method, which can transform the measured factor value affecting research object into the information value (Ruan and Huang Citation2001; Wu C and Qiao Citation2009; Xu W et al. Citation2013; Peng et al. Citation2021). The key point of IVM is to judge the possibility of water inrush hazards by calculating the total information value of the study area. In general, the greater the information value is, the greater the possibility of water inrush hazards has. The total information value calculation is mainly divided into two steps:
Calculating the information value of individual evaluation factors, as in EquationEquation 6
(6)
(6) .
Where Ii is the information value of evaluation factor xi, dimensionless; Ni is the total amount of distribution units of water inrush contain the factor xi, dimensionless; N is the total of distribution units of water inrush in the study area, dimensionless; Si is the total of the evaluation factors units containing the factor xi in the study area, dimensionless; S is the total of evaluation unit of study area, dimensionless.
2. Calculating the total information value of evaluation factors, as in EquationEquation 7
(7)
(7) .
Where I is the total information value, dimensionless.
3.4. The shortcomings of IVM and its improvement
By calculating the total information value of evaluation factors, IVM can judge the possibility of water inrush hazards in the different parts of the study area. However, the contribution rate of each factor for the water inrush hazard is different. If the factors are simply analyzed without considering their weight, the accuracy of evaluation model would be reduced. In order to determine the weight of factors, an improved AHP is chosen to describe the importance. In this model, the importance of AHP method is introduced to describe the importance of different factors in IVM method. But its importance is evaluated by calculating AUC value, and the process of calculating AUC value is objective. Thus, IVM is improved as EquationEquation 8(8)
(8) .
(8)
(8)
Where IW is the total information value considering the weight of the factor, dimensionless; wi is the weight value of evaluation factor xi, dimensionless; Ii is the information value of evaluation factor xi, dimensionless.
3.5. Establishment of the coupled AHP-IVM model
Risk assessment methods for water inrush consist of three parts: selecting the main evaluation factors, calculating the weight of evaluation factor, constructing the assessment model (Wu Q et al. Citation2017; Peng et al. Citation2021). Based on this framework, the establishment of the coupled AHP-IVM model can be divided into three steps ():
Construct the comprehensive evaluation factor system and calculate the information value of factors. Thematic maps of factors are generated based on the geographic information system (GIS). Then, the information value can be calculated by EquationEquation 6
(6)
(6) (Yang et al. Citation2018).
Calculate the weight of each factor by the improved AHP. According to AUC value of each factors, the importance of different factor to water inrush can be analyzed, and then the improved judgment matrix was established according to . Based on the improved AHP, the factor weight is determined.
Construct the coupled AHP-IVM model. Based on these weight values calculated in Step 2 and the information value of factors calculated in Step 1, the coupled AHP-IVM model is established by EquationEquation 8
(8)
(8) .
4. Application and results
4.1. The information value of controlling factors
Water inrush hazards in China are influenced by various factors (Wu Q and Zhou Citation2008; Chen PP and Liu Citation2010; Huang et al. Citation2012; Pang et al. Citation2021; Peng et al. Citation2021). The controlling factors can be divided into four aspects based on related studies of water inrush and the special geological and hydrogeological conditions in the Qinan coal mine:
Characteristics of the unconsolidated and confined aquifer. The characteristics of the aquifer are closely related to water inrush, which include the fully saturated of aquifer and coal mine pressure. The specific yield and the aquifer thickness are significant for judging the water abundance (Chen LW et al. Citation2018). In addition, coal mine pressure can be determined by the thickness of unconsolidated layer and the load transfer coefficient of the aquifer (Wang XZ Citation2012).
Bedrock lithology and its association. The bedrock lithology and its association are the key factors to determine the generation of water diversion channels (Palchik Citation2003; He et al. Citation2020). Four factors including the bedrock thickness, the thickest sandstone ratio in bedrock, the friable-plastic rocks ratio and the weathered bedrock thickness are selected to describe the bedrock lithology and its association.
Geological structure effects. Geological structures such as faults significantly contribute to the safety of coal mine production (Sun WB et al. Citation2019; Chen B et al. Citation2020). The development degree of the rock fracture shows a certain distance effect with the fault, and the closer the fault, the stronger the rock failure is, which provides conditions for water inrush. Thus, the distance from faults has an important influence on water inrush.
Mining activities. Under the influence of mining, the water flowing fracture zone is fully developed, which is the channel for groundwater to enter the working face, and its development height is the key to the occurrence of water inrush (Xu JL et al. Citation2009; He et al. Citation2020).
Based on the geological borehole and geological map information of the Qinan coal mine, the grading map of evaluation factors are generated by ArcGIS, as shown in .
Figure 4. Evaluation factor grading maps in the study area (a) the thickness of the unconsolidated soils; (b) the unconsolidated confined aquifer thickness; (c) the specific yield of the unconsolidated and confined aquifer; (d) the load transfer coefficient of the unconsolidated and confined aquifer; (e) the water pressure of the unconsolidated confined aquifer; (f) the thickness of the bottom clay layer; (g) the thickness of the bedrock; (h) the thickness ratio of friable rocks to plastic rocks; (i) the proportion of the thickest sandstone in the bedrock; (j) the depth of the weathered bedrock; (k) the distance from the faults; (l) the density of the faults; (m) the height of the water flowing fracture zone; (n) the thickness of coal seam; (o) the depth of the coal roof.


According to the grading maps, the information value of controlling factors are calculated by EquationEquation 6(6)
(6) , and the results are shown in .
Table 6. Information value of evaluation factors.
4.2. Weight calculation by the improved AHP method
Based on the improved AHP, the weight of each factor can be calculated. The importance of each factor to water inrush hazard is obtained by the success rate curve method. From the results presented in , AUC value of the thickness of the bedrock is 0.7409, meaning that the evaluation accuracy of the evaluation factor is 74.09%. AUC value of different evaluation factors according to the order from high to low is the height of the thickness of the bedrock (C1), the depth of the coal roof (C2), the height of the water flowing fracture zone (C3), the depth of the weathered bedrock (C4), the water pressure of the unconsolidated confined aquifer (C5), the thickness of the loose soils (C6), the density of the faults (C7), the distance from the faults (C8), the load transfer coefficient of the unconsolidated and confined aquifer (C9), the thickness of bottom clay layer (C10), the proportion of the thickest sandstone in the bedrock (C11), the thickness of coal seam (C12), the unconsolidated confined aquifer thickness (C13), the thickness ratio of friable rocks to plastic rocks (C14), the specific yield of the unconsolidated and confined aquifer (C15). According to AUC value of factors, a three-level hierarchical structure for water inrush () is established.
And then, based on AUC value of the evaluation factor, the second-grade factors (Ci) and the first-grade factor (Bi) can be divided into four categories: I (C13, C14, C15), II (C6, C7, C8, C9, C10, C11, C12), III (C4, C5, B1, B2, B3), IV (C1, C2, C3)), respectively. Thus, an improved judgment matrix of Bi (i = 1, 2, 3, 4) under A condition, Ci (i = 5, 6, 9, 10, 13, 15) under B1 condition, Ci (i = 1, 4, 11, 14) under B2 condition, Ci (i = 7, 8) under B3 condition, Ci (i = 2, 3, 12) under B4 condition are established by comparing the corresponding factors at the same level. In addition, the quantified relationships for the factor comparison at the same level can be found in .
The greatest characteristic root and corresponding eigenvectors of each judgment matrix are calculated algorithms in Matlab environment as shown in . The weight of each second-grade factor to the first-grade and the weight of each first-grade factor to the target layer are obtained. The CR value of each group of matrices can be calculated by EquationEquations 2(2)
(2) and Equation3
(3)
(3) , and the calculation results are all less than 0.1, which means the improving judgment matrix has satisfactory consistency and can pass the consistency test.
Table 7. Judgment matrix parameters of each grade factors.
Based on these local weight values, the weight of each second-grade factor to the target layer can be calculated. The calculation results are shown in .
Table 8. Results of the weight of the factors.
4.3. Risk assessment of mine water inrush using the coupled AHP-IVM model
Due to the information value and weight values of the evaluation factors (), the coupled AHP-IVM model is established as follows:
(9)
(9)
By calculating the information value of each unit in the grid and dividing all the results on average, the range of different water inrush areas can be obtained. The results are shown in .
Table 9. Information value of predicted water inrush areas.
Based on the model and GIS, the water inrush risk zoning map of the Qinan coal mine is presented in . Total information value (IW) in all units of the integrated map is classified as four levels by the Jenks natural breaks classification method, named risk area, relative risk area, relative safety area, and safety area, respectively (Jenks Citation1967).
As shown in , the overall risk of water inrush in the Qinan coal mine is relatively high, and the risk and relative risk areas are mainly concentrated in the central and northern parts of the study area. The west of the coal mine is the safe area. This trend is caused by the depth of the coal roof in the central and northern mining areas of the Qinan coal mine. In this model, the two most important water inrush factors are the depth of the coal roof and the height of the water flowing fracture zone. They had a weight of 0.24486. AUC value of the depth of the coal roof is 0.7230 and the height of the water flowing fracture zone is 0.7209. In this area, the average depth of the coal roof is more than 45 m, and the average development height of the water conducting fracture zone is found to 460 m–760 m. If the height of the water flowing fracture zone is greater than the distance between coal seam roof and aquifer, water inrush will occur. Therefore, this factor is very important for predicting water inrush (Xu et al. Citation2009) . It can be analyzed by comparing and that the height of the water flowing fracture zone provides the risk and relative risk areas. Therefore, it is more likely that the water flowing fracture zone will continue to develop and reaches the aquifer when mining under the unconsolidated and confined aquifer with complex geological conditions.
4.4. Comparison of the coupled AHP-IVM model and site situations
For comparison, the AHP and IVM models are also used for the risk assessment respectively. In this study, the AHP and IVM models are constructed using the same training data. Then, the corresponding water inrush risk zoning maps are produced, as shown in .
As shown in , the distribution trend of risk and relative risk areas predicted by the IVM model is similar to that of the coupled AHP-IVM model, and the AHP model is different from that of the coupled AHP-IVM model. However, the risk areas of the IVM model is larger than the coupled AHP-IVM model. It includes large areas without actual water inrush points, which indicates a poor discriminant ability of the two independent models.
In order to verify the prediction accuracy of the model, the prediction results are compared with the actual observation in the field. The distribution of the water inrush points under unconsolidated confined aquifers in the study area are divided into three levels: small water inrush points 0 < Q ≤ 60m3/h; medium water inrush points 60 m3/h < Q ≤ 600 m3/h; large water inrush points Q > 600m3/h, the result shown in .
Table 10. Summary of water inrush points distribution.
Table 11. Pearson’s chi squared test of predicted water inrush areas.
shows the advantages of the AHP-IVM method for the AHP method and the IVM method. The prediction accuracy of the coupled AHP-IVM model for all types of water inrush points is 100%. All the water inrush points are distributed in the risk area and relative risk area. The prediction accuracy of the AHP model for medium water inrush points is 100%, and for small water inrush points is 64.7%. The prediction accuracy of the IVM model for large and medium water inrush points is 100%, and for small water inrush points is 88.2%. The AHP-IVM model accuracy is higher than other models, which indicates the rationality of the model used in this study. In general, a risk zonation map included a large number of water inrush points in a small area of water inrush risk and relative risk area, indicating the model has a good discriminant ability and high prediction accuracy. The water inrush points of three models in different predicted water inrush areas were counted and analyzed Pearson’s chi-square test. The result is p < 0.01, indicating a statistically significant difference between the different models ().
shows that AUC value of the coupled AHP-IVM model is 0.83089, which is higher than that of the other two models. Thus, the coupling model for risk assessment of water inrush has a higher prediction accuracy and can effectively guide the safe and efficient production of coal mines under the unconsolidated and confined aquifer.
This study is aimed at the risk of mining under unconsolidated confined aquifers and is instructive for the production activities in Qinan coal mine. Water inrush can be prevented by advanced drainage, grouting reconstruction technology, curtain grouting, partial-filling technology and retaining safety coal pillar. If more detailed geological data is available, the accuracy of this method could be improved. This research is also valuable for coal mines with similar geological conditions.
5. Conclusions
Based on 15 factors, a risk assessment system of water inrush is established, and the weight of each factor is calculated by the coupled AHP-IVM method, which the judgment matrix establishment is improved by the information value method.
The information value of the evaluation factors and the total information value in the study area is calculated. The coupled AHP-IVM model is applied to evaluate the risk of water inrush when mining under the unconsolidated and confined aquifer in the Qinan coal mine. The evaluation result indicates that the overall risk of water inrush in the coal mine is relatively high, and the risk and relative risk areas are mainly concentrated in the southern, eastern and northern parts.
The evaluation results of the coupled AHP-IVM model are compared with the actual water inrush points in coal mining, and the actual water inrush points are all located in the risk area and relatively risk area. The success rate curve method is applied to evaluate the coupled AHP-IVM model, and AUC value is 0.83089. The prediction results of the coupled AHP-IVM model are consistent with the actual results, and the combination of the two different methods can improve the prediction accuracy of the model and effectively serve as general guidelines for mine operations.
Disclosure statement
The authors declare that they have no known competing financial interests or personal relationships that could have appeared to influence the work reported in this paper.
Data availability statement
The data used to support the findings of this study are available from the corresponding author upon request.
Additional information
Funding
References
- Chen B, Zhang S, Li Y, Li Z, Zhou H. 2020. Physical simulation study of crack propagation and instability information discrimination of rock-like materials with faults. Arab J Geosci. 13(18):1–12. doi: 10.1007/s12517-020-05966-8.
- Chen LW, Feng XQ, Xie WP, Xu DQ. 2016. Prediction of water-inrush risk areas in process of mining under the unconsolidated and confined aquifer: a case study from the Qidong coal mine in China. Environ Earth Sci. 75(8):1–17. doi: 10.1007/s12665-016-5533-5.
- Chen LW, Feng XQ, Xu DQ, Zeng W, Zheng ZY. 2018. Prediction of water inrush areas under an unconsolidated, confined aquifer: the application of multi-information superposition based on GIS and AHP in the Qidong coal mine, China. Mine Water Environ. 37(4):786–795. doi: 10.1007/s10230-018-0541-1.
- Chen PP, Liu XE. 2010. Research and application of water inrush early warning system to mine roof. Coal Sci Technol. 38(12):93–96.
- Fan Q, Ju NP, Xiang XQ, Huang J. 2014. Landslides hazards assessment with weights of evidence-a case study in Guizhou. China. J Eng Geo. 22(03):474–481.
- He X, Zhao YX, Zhang C, Han PH. 2020. A model to estimate the height of the water-conducting fracture zone for longwall panels in western China. Mine Water Environ. 39(4):823–838. doi: 10.1007/s10230-020-00726-2.
- Hu Y, Li W, Liu S, Wang Q, Wang Z. 2019. Risk assessment of water inrush from aquifers underlying the Qiuji coal mine in China. Arab J Geosci. 12(3):1–17. doi: 10.1007/s12517-019-4244-0.
- Huang HF, Mao XB, Yao BH, Pu H. 2012. Numerical simulation on fault water-inrush based on fluid-solid coupling theory. J Coal Sci Eng China. 18(3):291–296. doi: 10.1007/s12404-012-0312-8.
- Jenks GF. 1967. The data model concept in statistical mapping. Int Yearb Cartogr. 7:186–190.
- Khan MY, Xue GQ, Chen WY, Boateng CD. 2020. Investigation of groundwater in-rush zone using petrophysical logs and short-offset transient electromagnetic (SOTEM) data. JEEG. 25(3):433–437. doi: 10.32389/JEEG18-111.
- Khan MY, Xue GQ, Chen WY, Zhong HS. 2018. Analysis of long-offset transient electromagnetic (LOTEM) data in time, frequency, and pseudo-seismic domain. JEEG. 23(1):15–32. doi: 10.2113/JEEG23.1.15.
- Li B, Chen YL. 2016. Risk assessment of coal floor water inrush from underlying aquifers based on GRA–AHP and its application. Geotech Geol Eng. 34(1):143–154. doi: 10.1007/s10706-015-9935-z.
- Li B, Zhang HL, Luo YL, Liu L, Li T. 2022. Mine inflow prediction model based on unbiased Grey-Markov theory and its application. Earth Sci Inform. 15(2):855–862. doi: 10.1007/s12145-022-00770-2.
- Li B, Zhang WP, Long J, Fan J, Chen MY, Li T, Liu P. 2022. Multi-source information fusion technology for risk assessment of water inrush from coal floor karst aquifer. Geomat Nat Haz Risk. 13(1):2086–2106. doi: 10.1080/19475705.2022.2108728.
- Li JL, Wang SW, Wang Y, Wang XY, Wang XX. 2021. Water inrush risk assessment of coal floor after CBM development based on the Fractal-AHP-Vulnerlability index method. Geotech Geol Eng. 39(5):3487–3497. doi: 10.1007/s10706-021-01706-1.
- Li LP, Zhou ZQ, Li SC, Xue YG, Xu ZH, Shi SS. 2015. An attribute synthetic evaluation system for risk assessment of floor water inrush in coal mines. Mine Water Environ. 34(3):288–294. doi: 10.1007/s10230-014-0318-0.
- Li Q, Ma D, Zhang YD, Liu Y, Ma YJ. 2022. Insights into controlling factors of pore structure and hydraulic properties of broken rock mass in a geothermal reservoir. Lithosphere-Us. 2021(Special 5):1–17. doi: 10.2113/2022/3887832.
- Lu YG, Li HJ, Xia YJ, Liu HC, Han G. 2019. Prediction and evaluation study on coal seam roof water inrush based on multi-type four-double method. Coal Sci Technol. 47(09):219–228.
- Ma D, Duan HY, Zhang JX. 2022. Solid grain migration on hydraulic properties of fault rocks in underground mining tunnel: radial seepage experiments and verification of permeability prediction. Tunn Undergr Sp Tech. 126(08):1–14.
- Ma D, Duan HY, Zhang JX, Liu XW, Li ZH. 2022. Numerical simulation of water-silt inrush hazard of fault rock: a three-phase flow model. Rock Mech Rock Eng. 55(8):5163–5182. doi: 10.1007/s00603-022-02878-9.
- Palchik V. 2003. Formation of fractured zones in overburden due to longwall mining. Env Geol. 44(1):28–38. doi: 10.1007/s00254-002-0732-7.
- Pang L, Liu W, Qin Y. 2021. Analysis of main controlling factors of overburden failure in coal mining under thick coal seam geological conditions. Geotech Geol Eng. 39(2):883–896. doi: 10.1007/s10706-020-01529-6.
- Peng ZH, Chen LW, Hou XW, Ou QH, Zhang J, Chen YF. 2021. Risk assessment of water inrush under an unconsolidated, confined aquifer: the application of GIS and information value model in the Qidong coal mine, China. Earth Sci Inform. 14(4):2373–2386. doi: 10.1007/s12145-021-00702-6.
- Ruan SY, Huang RQ. 2001. Application of GIS-based information model on assessment of geological hazards risk. J Chengdu Univ Tech. 18(01):89–92.
- Ruan ZE, Li CP, Wu AX, Wang Y. 2019. A new risk assessment model for underground mine water inrush based on AHP and D-S evidence theory. Mine Water Environ. 38(3):488–496. doi: 10.1007/s10230-018-00575-0.
- Saaty TL. 1980. The analytic hierarchy process: planning, priority setting, resource allocation. New York: McGraw-Hill International Book Co.
- Shi LQ, Qiu M, Teng C, Wang Y, Liu TH, Qu XY. 2020. Risk assessment of water inrush to coal seams from underlying aquifer by an innovative combination of the TFN-AHP and TOPSIS techniques. Arab J Geosci. 13(14):1–18. doi: 10.1007/s12517-020-05588-0.
- Sun WB, Xue YC, Li TT, Liu WT. 2019. Multi-field coupling of water inrush channel formation in a deep mine with a buried fault. Mine Water Environ. 38(3):528–535. doi: 10.1007/s10230-019-00616-2.
- Sun WJ, Zhou WF, Jiao J. 2016. Hydrogeological classification and water inrush accidents in China’s coal mines. Mine Water Environ. 35(2):214–220. doi: 10.1007/s10230-015-0363-3.
- Wang F, Xu J, Xie J. 2019. Effects of arch structure in unconsolidated layers on fracture and failure of overlying strata. Int J Rock Mech Min Sci. 114(02):141–152. doi: 10.1016/j.ijrmms.2018.12.016.
- Wang Q, Lin Y, Li W, Yu X, Zhang Y. 2022. GIS-based evaluation of water-inrush risk from coal floor using logistic regression and certainty factor models. Arab J Geosci. 15(3):1–11. doi: 10.1007/s12517-022-09501-9.
- Wang XZ. 2012. Study on occurrence condition and prevention of support crushing and water inrush hazard during mining under unconsolidated confined aquifer [PhD dissertation]. Hefei: China Univ. Min. Tech.
- Wang Y, Yang WF, Li M, Liu X. 2012. Risk assessment of floor water inrush in coal mines based on secondary fuzzy comprehensive evaluation. Int J Rock Mech Min Sci. 52(04):50–55. doi: 10.1016/j.ijrmms.2012.03.006.
- Wang ZY, Zhang Q, Shao JL, Zhang WQ, Wu XT, Lei Y, Wu XA. 2021. Mathematical evaluation on the control of mining-induced ground subsidence in thick loose strata. ACS Omega. 6(50):34596–34605. doi: 10.1021/acsomega.1c04970.
- Wu C, Qiao J. 2009. Relationship between landslides and lithology in the Three Gorges Reservoir area based on GIS and information value model. Front for China. 4(2):165–170. doi: 10.1007/s11461-009-0030-6.
- Wu Q, Liu Y, Yang L. 2011. Using the vulnerable index method to assess the likelihood of a water inrush through the floor of a multi-seam coal mine in China. Mine Water Environ. 30(1):54–60. doi: 10.1007/s10230-010-0125-1.
- Wu Q, Xu K, Zhang W. 2016. Further research on “three maps—two predictions” method for prediction on coal seam roof water bursting risk. J China Coal Soc. 41(06):1341–1347.
- Wu Q, Zhao DK, Wang Y, Shen JJ, Mu WP, Liu HL. 2017. Method for assessing coal-floor water-inrush risk based on the variable-weight model and unascertained measure theory. Hydrogeol J. 25(7):2089–2103. doi: 10.1007/s10040-017-1614-0.
- Wu Q, Zhou W. 2008. Prediction of groundwater inrush into coal mines from aquifers underlying the coal seams in China: vulnerability index method and its construction. Environ Geol. 56(2):245–254. doi: 10.1007/s00254-007-1160-5.
- Xu JL, Wang XZ, Liu WT, Wang ZG. 2009. Effects of primary key stratum location on height of water flowing fracture zone. J Rock Mech Eng. 28(02):380–385.
- Xu W, Yu W, Jing S, Zhang G, Huang J. 2013. Debris flow susceptibility assessment by GIS and information value model in a large-scale region, Sichuan Province (China). Nat Hazards. 65(3):1379–1392. doi: 10.1007/s11069-012-0414-z.
- Xue Y, Liu J, Ranjith PG, Gao F, Xie HP, Wang J. 2022. Changes in microstructure and mechanical properties of low-permeability coal induced by pulsating nitrogen fatigue fracturing tests. Rock Mech Rock Eng. 55(12):7469–7488. doi: 10.1007/s00603-022-03031-2.
- Xue Y, Ranjith PG, Chen Y, Cai CZ, Gao F, Liu XG. 2023. Nonlinear mechanical characteristics and damage constitutive model of coal under CO2 adsorption during geological sequestration. Fuel. 331(P1):125690. doi: 10.1016/j.fuel.2022.125690.
- Yang B, Sui W, Duan L. 2017. Risk assessment of water inrush in an underground coal mine based on GIS and fuzzy set theory. Mine Water Environ. 36(4):617–627. doi: 10.1007/s10230-017-0457-1.
- Yang B, Yuan J, Ye Z. 2018. Risk assessment of coal mining above confined aquifer based on maximizing deviation in a GIS environment. Arab J Geosci. 11(12):299. doi: 10.1007/s12517-018-3651-y.
- Zhang KZ, Wang L, Cheng YP, Li W, Kan J, Tu QY, Jiang JY. 2020. Geological Control of Fold Structure on Gas Occurrence and Its Implication for Coalbed Gas Outburst: case Study in the Qinan Coal Mine, Huaibei Coalfield, China. Nat Resour Res. 29(2):1375–1395. doi: 10.1007/s11053-019-09511-7.
- Zhang Q, Wang ZY. 2021. Spatial prediction of loose aquifer water abundance mapping based on a hybrid statistical learning approach. Earth Sci Inform. 14(3):1349–1365. doi: 10.1007/s12145-021-00640-3.