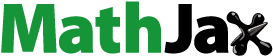
Abstract
The COVID-19 has caused adverse effects in various aspects, and its impact on population mobility cannot be ignored. In this study, we obtain mobility data for the Beijing–Tianjin–Hebei region during the Chunyun period of 2019–2021 and divide it into four stages. By developing directional weighted networks, the mobility patterns for pre- and post-epidemic are examined. Firstly, the migration scale declines significantly post-epidemic. The fourth stage migration scale exceeds 65% of 2019 in 2021 indicating the situation improves. Secondly, the structural characteristics are compared. The average degree values, clustering coefficients, and path lengths in the fourth stage of 2021 are higher than the values in 2020, showing the recovery of migration, but also efficiency degradation. Thirdly, community detection results show that three communities can be highlighted in the network, which followed Regional Development Pattern. Finally, the findings of Modified Alter-based Centrality and Alter-based Power models show that Beijing (27.78, 17.74) and Tianjin (14.92, 4.38) are typical cities, Tangshan (3.74, 0.03) and Langfang (3.40, 2.00) are gateway cities and others are general cities. A reasonable population distribution has not been formed. The study provides fresh perspectives for applying spatio-temporal data in health emergencies, and the results provide theoretical support for policy formulation of socio-economic recovery.
1. Introduction
The fast flow of production factors across global cities is rapidly transforming the conventional hierarchical city system. Cities are becoming increasingly interconnected. The city system evolves into network organization types with a focus on horizontal linkages, also known as urban networks. Kumar and Castells (Citation1997) proposed the concept of ‘flow space’, which is a dynamic networked space with two-way or multi-way movement of people, logistics, and information based on information technology. Since then, the study of urban networks has gradually evolved into a vital component of the study of urban systems (Yang et al. Citation2017; Sigler et al. Citation2021; Wang et al. Citation2021).
In urban networks, the nodes refer to cities, and the expression of interactions between nodes is necessary for the formation of urban networks. However, the representation of such interactions often uses static data, resulting in a limited research perspective (Derudder and Taylor Citation2005). Since Taylor and Derudder (Citation2015) proposed the Inter-locking Network Model, research on urban networks has begun to focus on the importance of relational data for network modeling (Losacker Citation2022; Malik Citation2022; Pan and Fang Citation2022). As a prerequisite and material basis for exchanging other production factors among cities, the population is the most important carrier to promote economic development and technological innovation, and is known as the ‘barometer of the rise and fall of cities’. Population mobility reflects the flow of capital, transportation, information, and other spatial relationships. The study of population mobility behavior, such as agglomeration and migration, is critical for revealing the functional placement of various cities, city connections, and the sustainable development level (Glaeser Citation2012; Lin et al. Citation2023).
Smart terminals and mobile communication technologies enable the collection of complete, dynamic, and real-time population flow data (Wu et al. Citation2022). Kraemer et al. (Citation2020) used data from Google location history for an analysis of global human mobility patterns; Pan and Lai (Citation2019) obtained Tencent migration data during the National Day and Mid-Autumn Festival to explore the spatial pattern of population mobility in Chinese cities; Li et al. (Citation2016) used multi-source tourism data from Baidu, Tencent, and Qihoo to analyze the spatial and temporal characteristics of population mobility during the Chinese Spring Festival. García-Palomares et al. (Citation2018) investigated the daily dynamics of the population in the city of Madrid utilizing geolocation data on Twitter. These studies, however, tended to focus on evaluating population mobility patterns within a country or city. Nowadays, urban agglomerations are gradually becoming the main form of modern urbanization. Urban agglomerations are the hub of social activity and resource aggregation, the rational layout of which can enhance the efficiency of resource allocation and play an important role in economic growth (Davis et al. Citation2014; Han et al. Citation2019). The construction of population mobility networks within urban agglomerations provides a powerful tool for exploring the rationality of urban agglomeration layouts.
In addition, for the construction of urban networks, the type chosen by most of the current studies is the undirected unweighted network, which generates a 0–1 matrix by considering the presence or absence of direct links between cities (Liu and Xiao Citation2021; Shen et al. Citation2021). This method, however, disregards the fact that linkages between cities differ significantly, leading to a mismatch between the constructed network and the actual situation. The direction and asymmetry of the flows must be quantified for the population network. It is preferable to construct the directional weighted network.
It has been shown that community hierarchies are commonly found in networks (Newman Citation2006; Fortunato Citation2010). Nodes within a community are tightly connected, while the connected edges between communities are sparse. The communities identified by the community detection algorithm represent multi-level spatial organization patterns. If these patterns are incorporated into regional development planning, it will help enhance the coordination linkage and functional complementarity between different cities. Current research tends to use community detection to identify subgroups from the national level (He et al. Citation2021; Liu et al. Citation2022), while community structure in urban agglomerations has received less attention.
Furthermore, identifying the characteristics of urban nodes is important to improve the efficiency of resource allocation in the network. Most of the research is based on centrality approaches (Liu et al. Citation2020; Shen et al. Citation2021). The higher the centrality of a node, the higher its position in the network and the stronger its control over resources. As urban network science continues to evolve, several scholars have distinguished the relevant concepts and revised the traditional approach. Neal (Citation2011) emphasized that centrality is not the same as the power of control in urban networks. Centrality is the unity of an urban node’s ability to aggregate and diffuse resources in the network, and control is the influence and dominance of a city in the resource flows, which is determined by its position and function in the network. These developments led to the theories of alter-based centrality and power (Neal Citation2013). However, this model does not apply to the directional weighting network. According to Zhao et al. (Citation2017), the degree centrality used for alter-based centrality and power measures the importance of nodes only from the unweighted network, which weakens the variability of weights among associated cities. Additionally, the strength of connections between cities used for the method does not reflect the directionality that resource flows have in urban networks. This suggests that more appropriate models are needed for the measurement of node centrality and power in directed weighted networks.
Meanwhile, cities face various uncertain risks during development. It is urgent to improve urban resilience, meaning the ability to resist external disturbances, to address the safety and growth of urban systems. Most relevant research focuses on qualitative and quantitative assessment of urban resilience levels. Serbanica and Constantin (Citation2023) used an empirical study to analyze the relationship between urban resilience and sustainable development; Ruan et al. (Citation2021) measured the evolution of the spatio-temporal pattern of urban resilience in Guangzhou under different scenarios based on a quantitative evaluation model; Zhao et al. (Citation2022) conducted an assessment of the level of resilience of Chinese cities by creating multidimensional indicators combined with quantitative assessment methods. However, these studies tend to involve individual cities only. With the growing agglomeration effect among cities, the consequences of any disaster damage in one area may spread to other areas, and it is critical to extend the concept of resilience to a regional scope (Xu et al. Citation2023). It has been shown that urban network structure and regional resilience are closely related (Boschma Citation2015). The change in network structure before and after a crisis is also a reflection of the ability of the region to handle unexpected crises and the degree of recovery, which coincides with the meaning of resilience. However, fewer studies have explored regional resilience through changes in urban networks. The breakout of the COVID-19 epidemic at the end of 2019 posed a severe test for every country (Qazi et al. Citation2021). Many countries have implemented strict measures to prevent and control the epidemic. One of these was the restriction of population movement to prevent the disease from spreading quickly. As a result, population mobility patterns have shifted (Liu Citation2020). Much research has used mobility data to explore the patterns during epidemics and anticipate the spread of epidemics. Bonaccorsi et al. (Citation2020) provided an analysis of the impact of the Italian embargo measures on socio-economic conditions based on real-time mobility data; Santamaria et al. (Citation2020) used anonymized location data to reveal the impact of outbreak restrictions on population mobility in Europe; Yang et al. (Citation2022) predicted the spread of the epidemic in China by using Baidu real-time dynamic surveillance system data. But few studies analyzed the impact of the epidemic on the status of population mobility networks over a relatively long-time scale.
By constructing the population mobility network in the Beijing–Tianjin–Hebei urban agglomeration, this paper aims to systematically analyze the spatio-temporal characteristics of the network both pre- and post-epidemic, and to investigate the differentiation pattern of population mobility under the influence of the epidemic, following the research sequence of ‘overall network-community structure-urban nodes’. The results obtained from the study can be utilized to optimize the resource allocation model of regions, which is conducive to exploring an effective path for coordinated regional development. Compared with previous studies, the main contributions of this paper are as follows:
First, the population mobility behavior is modeled using location-based service data to construct directional weighted networks. It offers new applications for spatio-temporal big data in public health emergencies. Second, based on the changes in urban network structure, the resilience of the region reflected by COVID-19 is assessed. This broadens the scope of regional resilience studies. Third, this study uses the community detection algorithm to explore the community structures that exist within the region, providing a new method for identifying population and resource concentration patterns. Fourth, the MAC-MAP model is proposed for measuring the functional characteristics of each urban node. The findings facilitate the formulation of targeted scientific development plans.
2. Methodology and data
This section initially presents the study area and data sources, and then describes the study methodology, including (1) construction of population mobility networks; (2) network characterization methods; (3) community detection algorithm; (4) the MAC-MAP model proposed to identify network node features. The flowchart of this study is shown in .
2.1. Study area
As China’s urbanization process accelerates, so does the expansion of urban agglomerations (Fang et al. Citation2022). The Beijing-Tianjin-Hebei (BTH) metropolitan agglomeration, which contains the capital Beijing and is near the Bohai Sea, is one of the most active and open sectors of China’s economy, absorbing a large population. The BTH urban agglomeration includes 13 cities: Beijing, Tianjin, Shijiazhuang, Tangshan, Qinhuangdao, Handan, Xingtai, Baoding, Zhangjiakou, Chengde, Cangzhou, Langfang, and Hengshui (). Beijing and Tianjin are municipalities, and Shijiazhuang is the provincial administrative center of Hebei Province (The three cities are defined in this study as the central cities of the BTH region). The other cities are prefecture-level cities. In the process of coordinated development, this region faces some dilemmas, including the problem of irrational population distribution patterns. This worsening problem is not only detrimental to the deconstruction of Beijing’s non-capital functions but also causes population loss in some cities in Hebei Province, which is not conducive to creating a demographic basis for sustainable economic growth. Meanwhile, the outbreak may reshape the pattern of population movement in this region, and there is a need to investigate the changes pre- and post-epidemic and devise treatments to support a balanced population distribution.
2.2. Data source
In this study, migration data are collected from the migration big data platformFootnote1 launched by Baidu Company in 2014, which is the location data statistics of Baidu Maps and third-party users. Baidu’s location service responds to hundreds of millions of location service requests from users every day and records migration paths.
The data collected in this study include (1) Migration scale index: including the in-migration and the out-migration scale index, which reflects the scale of a city’s migrating population on a given day. It makes the daily migration of a city a uniform standard in terms of size and comparable among different cities. (2) In-migration (out-migration) ratio: For each city, the platform provides the top 100 cities in terms of the number of migrants in (out) to that city per day and the ratio.
Baidu migration data for each city in the BTH region during the Spring Festival travel season (Chunyun period) in 2019, 2020, and 2021 are extracted. The Chunyun period is usually bounded by the Spring Festival and includes the 15 days before as well as the 25 days after, and is known as a rare global population mobility. To a significant extent, this short-term, large-scale mobility phenomenon can reflect a migration pattern and direction (Zhu et al. Citation2021). Due to the holiday policy and traditional customs during the Spring Festival, the Chunyun period has the obvious phase-specific characteristics of population mobility, and this study splits the period into four stages, defined as follows:
First stage: Hometown-returning Stage (December 16–December 29 of the lunar calendar)
Second stage: Chinese Spring Festival Holiday (December 30–January 9 of the lunar calendar)
Third stage: Workplace-returning Stage (January 10–January 16 of the lunar calendar)
Fourth stage: Daily Population Mobility Stage (January 17–January 25 of the lunar calendar)
2.3. Construction of population mobility network
The data provided by Baidu’s migration platform is a relative weighting ratio of total in-and out-migration for each city, rather than actual population flow data, so the data need to be processed before analysis. The daily population mobility connection strength is defined as follows:
(1)
(1)
Where, Rij is the population mobility connection strength from city i to city j;
MEij is the ratio of the population moving out of city i to city j to the population of all people moving out of city i;
MIij is the ratio of the population of city i moving into city j to the population of all people moving into city j;
GEi is the out-migration scale index for city i;
GIj is the in-migration scale index for city j.
MEij, MIij, GEi, and GIj are data obtained from the Baidu migration platform.
The matrix: R = (Rij) is constructed to characterize the daily population mobility, which is the basis for the population mobility network:
(2)
(2)
Where n denotes the nth city, and when the subscript numbers are the same indicates the same city. Migration data from December 16th to January 25th of the lunar calendar in 2020 and 2021 of 13 cities are selected and processed.Footnote2 Eighty 13 × 13 multi-valued asymmetric directed matrices with a temporal resolution of days are obtained.
The total migration scale TMi,t, and the net migration scale NMi,t for the city i over time are defined to characterize the migration scale of each city at each stage. The formulas are as follows:
(3)
(3)
(4)
(4)
When NMi,t is positive, it means that city i show net in-migration of the population at time t, conversely, city i show net out-migration.
2.4. Analysis of network characterization
The vertex strength, weighted average degree, weighted average clustering coefficient, and weighted average path length are selected to analyze the node and structure characteristics of the population mobility network, which are described in .
Table 1. Description of the calculation methods of network characteristics.
2.5. Community structure detection
In this study, the population mobility network of the BTH urban agglomeration is divided into community hierarchies using the Louvain method (Blondel et al. Citation2008). To begin, each node is allocated a unique community. The number of communities in this initial partition is equal to the number of nodes. The node j adjacent to node i is then analyzed for each node i and the gain of modularity obtained by removing i from its community and placing it in the community of node j is assessed. The above process is repeated. Until node i is placed in the community with the highest modularity gain. The node is retained in the original community if the gain of modularity is negative. The structure is considered to be optimally partitioned when the above process is applied sequentially to all nodes in the network.
2.6. The MAC-MAP model for identifying node features
This study considers the limitations of centrality measures in directed weighted networks, and chooses the theory of Alter-based Centrality (AC) and Alter-based Power (AP) proposed by Neal (Citation2013):
(12)
(12)
(13)
(13)
Where DCj is the degree centrality of city j. The product of Rij and DCj represents the ability of city i to obtain resources through city j, and ACi is the ability of city i to obtain resources in the total network; The ratio of Rij to DCj indicates the ability of city i as an interaction hub in the participation of city j in the circulation of resources throughout the network, while APi is the control role of city i in the network for the related cities.
Adaptations to this model, however, are required for the specific situation of this study: First, the network constructed in this study is the directional weighted network, in which the directionality of population mobility and the volume of flow should be reflected. Second, the distance decay effect should not be ignored. Based on these, we propose the Modified Alter-based Centrality (MAC) and Alter-based Power (MAP) models to assess the centrality and power of urban nodes. The calculation formula is as follows:
(14)
(14)
(15)
(15)
(16)
(16)
Where MACi is the modified alter-based centrality of the city i. When MACi is positive, city i is population-aggregation, conversely, city i is population-diffusion; MAPi is the modified alter-based power of city i. When MAPi is positive, city i is a control city with resources; conversely, city i is a controlled city; is the normalized distance between the two cities.
3. Results
3.1. Spatio-temporal characteristics of population mobility
3.1.1. Pre-epidemic
3.1.1.1. Temporal characteristics
and show curves of total and net migration scale during the Chunyun period in 2019. The reference lines of relevant time points are set to separate the period. The migration shows different variations at each stage. The total migration scale of cities increases steadily during the first stage, which is since as the Spring Festival approaches, most non-local workers begin to return to their hometowns. The total migration scale reaches its highest point on December 27th or 28th. The net migration scale curve also changes with the arrival of the Spring Festival. Beijing, Tianjin, Shijiazhuang, and Langfang show net out-migration of the population during this stage because these cities are labor-importing cities with high levels of economic development. Other cities mostly show net in-migration of the population.
In the second stage, the total migration scale of all cities drops to its lowest point on January 1st (the first day of the Spring Festival). Subsequently, the trend of the total migration scale of all cities shows essentially the same fluctuation trend. The type of population mobility in most cities shows the opposite phenomenon of the first stage. The three central cities show net in-migration of the population starting on January 3rd, as laborers begin to return to the workplaces. Other cities show net out-migration of population. The net migration scale for Qinhuangdao is essentially zero. This is because Qinhuangdao is a tourist city that attracts a large number of tourists in the Holiday. As people return to their places of employment, a fundamental balance between in- and out-migration is reached.
In the third stage, the curves of the total and net migration scales are fluctuating. The net migration scale of Beijing and Tianjin drops significantly on January 15th, because this day is the Chinese Lantern Festival and people often choose to return home. The fourth stage shows the normal state formed by inter-city business, work, and tourism activities. And the scale of net migration gradually decreases over time, and the curve of most cities gradually flattens out and approaches the coordinate axis, which indicates that the in-migration and out-migration of cities reach a relatively balanced state.
Furthermore, during the non-Chinese Festival holiday period, there are significant differences in the ‘weekend phenomenon’. Among the three central cities, Beijing’s net migration decreases suddenly on Saturday, generating a trough in the image, but Tianjin and Shijiazhuang are not obvious. This short-term migration fluctuation reflects the siphoning capacity of Beijing.
3.1.1.2. Network topology characteristics
The connection strength and vertex strength for the first stage data in 2020 are graded using the natural break method and plotted (). Since the connection strength has high dispersion, it is normalized to be in the range of 0–1. The epidemic has not yet affected the region at this stage, it reflects the pattern of population mobility during the hometown-returning stage under normal circumstances.
The overall structure depicts a distribution with the three central cities as the core and radiates outward. The central cities have the high-ranked vertex strength and they have strong connection strength with neighboring cities, which reflects their population radiation capacity. These cities are unevenly connected, with stronger connections between Beijing and Tianjin and looser connections between Shijiazhuang and the other two cities. Langfang and Baoding also have high vertex strength. Moreover, the core-periphery structure is obvious. Cities located at the periphery of the urban agglomeration (Qinhuangdao, Chengde, and Zhangjiakou) have low vertex strength. The density of population networks linking these cities to other cities is constrained by geographic factors and degrees of development.
3.1.2. Under the influence of the epidemic
3.1.2.1. Analysis of the total migration scale from 2019 to 2021
As shown in , the total migration scale in 2020 and 2019 exhibits some degree of coupling in the first stage, which continued into the initial second stage. This indicates that even the emergence of COVID-19 and related reports did not inhibit extensive migration. However, the outbreak of the epidemic and epidemic prevention policies greatly affected the population movement in the second stage, and the total migration scale significantly drops. The third and fourth stages also continue to hover at low levels.
Under the medium-term impact of the epidemic in 2021, the population migration rises slowly, and the peak of the first and third stages is much lower than that of the same period in 2019. It indicates that some people choose to stay in their workplaces for the Spring Festival in response to the appeal of the government. As a result, the workplace-returning migration (Third stage) is also influenced, and the curves of these two stages in are generally smooth. In the fourth stage, total migration in 2021 exceeds 65% of that in 2019, showing that people’s travel and family visits are gradually resuming during the normalized phase of epidemic prevention. Meanwhile, with sporadic epidemic situations in some cities, people’s concerns about health considerations and the still strict epidemic prevention policies have affected cross-city mobility. The scale of migration gradually stabilizes over time.
3.1.2.2. Network topology characteristics
shows the topology of the population mobility network in the first stage of 2021. Overall, compared to 2020 (), there is a significant decrease in connection strength between some cities in terms of rank, including as Chengde–Beijing, Baoding–Beijing, Shijiazhuang–Baoding, and Shijiazhuang–Xingtai. Because of the closure control policy and periodic outbreaks (Shijiazhuang experienced mass infections in late 2020), commuting and long-distance transit across the city remain impacted at this moment. People choose to stay at the workplace for health and policy reasons (e.g. the necessity for quarantine while arriving in another city). In addition to this, it can be observed that Beijing, Tianjin, and their surrounding cities remain the most active areas for migration and the core-periphery structure is strengthened. The intensity of the nodes in Tangshan and Langfang has instead gone up a rank. This is because the impact of the epidemic has strengthened their geographical advantage in absorbing the transfer of population, industry, and public services from the central cities. Other cities at the edge of the network are slower to recover from the epidemic and their migration behavior is affected due to government restrictions on public transportation and higher pressure on epidemic prevention caused by imperfect medical facilities.
3.1.2.3. Comparison of network characteristics
The structural indicators of the population mobility network for each stage in 2020 and 2021 are displayed in . The D value for the first stage in 2021 is only about 29.7% of that in 2020, and the second stage in both years is comparable and represents the mobility during the Spring Festival holiday affected by the epidemic. Entering the third and fourth stages, the migration level in 2021 is resuming because of the continued recovery of the city, and the D value exceeds the same period in 2020, reaching 51.18.
Table 2. Comparison of network characteristics between 2020 and 2021.
The increase in C values in the second stage of 2020 compared to the first stage may be due to the preventive policy, the limited movement of people across municipalities during the holidays, where people will only move within a certain range and the tendency to cluster within the network is evident. In the third and fourth stages, the C value falls back. This is because of the massive outbreak of the epidemic, which has suppressed the behavior of returning to work and daily mobility. The edge weights in the network are low and the connections are looser. In 2021, the C value exhibits a similar trend, with a considerable increase in the second stage but a decline in the third and fourth stages. However, the C value of the latter two stages is significantly higher than that of the same period in 2020, indicating that population mobility within a certain range is beginning to gradually recover with an increasing degree of clustering.
The L value shows a continuous upward trend, with the smallest L value of 1.646 in the first stage of 2020 and the largest L value of 2.01 in the fourth stage of 2021. This demonstrates the growing resistance to be overcome in the circulation of elements in the population mobility network. This also represents the higher cost and less effective circulation of cross-city population movements under the influence of COVID-19 because network efficiency is inversely proportional to path length. And this situation has not changed with the improvement of the epidemic situation.
3.2. Delineating community structure within the urban agglomeration
The fourth stage in 2021 is the daily stage of population mobility, which indicates the normal level of population mobility in the BTH region under the medium-term impact of the epidemic. shows the community detection results of the population mobility network for this stage. Cities with the same color in the figure are defined as the same community. The urban agglomeration is divided into three communities, with Beijing, Tianjin, and Shijiazhuang as the cores. Among them, the Beijing-cored community, distributed in the north and west of the BTH region, includes five cities and occupies the largest area; the Tianjin-cored community includes four cities, showing a strip distribution and concentrated along the Bohai Sea coast; Shijiazhuang-cored community, including four cities, is distributed in the south of Hebei Province. Combined with the previous analysis, cities within the same community are more closely connected. The communities with Beijing and Tianjin as the cores are frequently connected, relying mainly on the well-communicated role of the two cities and Langfang. However, the connection between the Shijiazhuang-cored community and the other two communities is weaker.
3.3. Centrality and power measurement of the urban nodes
The population migration data of the fourth stage in 2021 is selected, and by calculating the Modified Alter-based Centrality (MAC) and Modified Alter-based Power (MAP), aiming to determine the characteristics of each city. The results of the calculation according to EquationEquations (14)(14)
(14) and Equation(15)
(15)
(15) are presented in . gives the two-dimensional distribution characteristics of each urban node. is a close-up of . Beijing (27.78, 17.74) and Tianjin (14.92, 4.38) located in the first quadrant, are typical cities with high centrality and control. Meanwhile, Tangshan (3.74, 0.03) and Langfang (3.40, 2.00) also located in the first quadrant, have relatively high centrality and control. Shijiazhuang (0.54, −1.86), another central city, is located in the second quadrant (). Other cities in the third quadrant are general cities.
Table 3. The MAC and MAP of each node.
Except for the three central cities, only Tangshan and Langfang are population-aggregation cities, while the population-diffusion cities make up 81.8% of all the cities in Hebei Province. This indicates that Hebei Province has a significant issue of population loss. In terms of MAP, Beijing and Tianjin have an absolute influence on the flow of resources in the network. Langfang and Tangshan have high MAP due to their high level of economic development and proximity to the central cities, controlling the opportunities and possibilities of resource flow to a certain extent. Other cities in Hebei province have negative values of MAP and are in the controlled role.
4. Discussion
4.1. Impact of COVID-19 on population mobility networks in BTH urban agglomeration
4.1.1. Spatio-temporal effects
The total scale of population migration under the medium-term impact of the epidemic still has a large gap compared to the same period before the epidemic (). As the epidemic gradually stabilizes and enters a normalized phase of epidemic prevention, the scale of migration steadily increases. According to the topology of the mobility network, the network formed by Beijing, Tianjin, and neighboring cities shows a high degree of resilience (), which brings new development opportunities for Tangshan and Langfang. As the core-peripheral structure continues to be intensified, it is essential to notice that the peripheral cities, like Qinhuangdao, Chengde, and Xingtai, are weak links in the development’s synergy and that the pandemic makes connecting them to the central cities more expensive. However, the employment-driven population mobility patterns evident before the epidemic may change as a result, and these cities are likely to retain a portion of their labor force, facilitating the establishment of a post-epidemic demographic base for economic recovery. At the same time, sporadic outbreaks have a greater impact on migration networks in the southern part of Hebei province. This illustrates the possibility that the rebound of the epidemic leaves some regions in a state of prevention for a long time, which is harmful to the recovery of population movement and thus increases the development gap.
The changes in the structural characteristics of the network corroborate the above results to some extent (). While inter-city mobility behavior is gradually recovering (rising D value), internal clustering is becoming more clear (rising C value), and the impact of the epidemic is hindering the efficiency of population mobility (rising L value). On the one hand, it may aggravate population clustering in highly resilient cities and lead to negative consequences like urban overcrowding. On the other hand, population loss could widen the development gap in cities with low resilience.
4.1.2. Recovery of population mobility in three central cities
depicts the net migration of the three central cities in 2019 and 2021. All cities show net out-migration of the population in the first stage of the two years, which is related to their strong attractiveness to the laborer. And this attractiveness to the population remains evident under the medium-term impact of the epidemic. Entering the second stage in 2021, the net migration scale of Shijiazhuang is close to zero and continues until the fourth stage, due to the impact of the outbreak. On the contrary, Beijing and Tianjin have gradually narrowed their gap with the historical period over time, suggesting that both cities have recovered relatively well from the epidemic as engines of economic development in the BTH region. The above illustrates that even though the normalized epidemic prevention and control phase has been entered, the rate of recovery differs per city related to the probability of occasional epidemic cases and localized outbreaks, which have a substantial influence on migratory activities.
4.2. Community structure
The Hebei Provincial Government has suggested the ‘Regional Development Pattern’ in the 14th Five-Year PlanFootnote3 as part of the ongoing promotion of the Beijing–Tianjin–Hebei Cooperative Development Strategy, which primarily consists of:
Beijing-Tianjin core function area, including Baoding and Langfang.
Coastal Development Zone, including Tangshan, Qinhuangdao, and Cangzhou.
South Central Functional Expansion Zone of Hebei Province, including Shijiazhuang, Handan, Xingtai, and Hengshui.
This is generally consistent with the community division results obtained in this study (), which also demonstrates that the community organization within the urban agglomeration is mostly rational, but some issues persist. Baoding and Langfang are located in the Beijing-cored community, and the population siphoning capacity of Beijing for these two cities is stronger than Tianjin. In the context of the pandemic, the influx of labor into Beijing from the surrounding area will further increase. This is not conducive to solving the ‘Metropolitan Diseases’ like population overload and ecological degradation. In the Tianjin-cored community, Qinhuangdao is located in the northern region, with weak connection and a low economic development level compared to Tangshan and Cangzhou. In addition, the epidemic’s impact has hit tourism, one of the city’s key industries, which puts additional strain on economic growth. This city is currently on the ‘Coastal Development Zone’ short board. Moreover, the overall development level in the Shijiazhuang-cored community is weak. This is related to the lack of a distinct functional orientation of urban nodes within this community. At the same time, the ‘Regional Development Pattern’ mentioned above also proposes the establishment of the Northwest Hebei Ecological Conservation Zone, including Zhangjiakou and Chengde. The major function is to establish a green ecological system and promote ecological security in the urban agglomeration by maximizing the ecological resource endowment of the two cities. However, this study finds that the two cities had limited connection strength with other cities except for Beijing. Additionally, the impact of the epidemic has weakened the connection with Beijing (). The population, material, capital, and other resources of this region are poorly communicated, and its functionality of cities is not fully demonstrated.
4.3. Spatial grading characteristics of the urban nodes
shows the spatial distribution of the MAC and MAP features of the nodes. Cities in the network’s outer edges have greater costs to directly associate with the central cities due to factors like infrastructure construction and national policies. In general, the exchange is operated by first associating with the gateway cities and later central cities (Zhao et al. Citation2017). Tangshan and Langfang have high MAC and MAP, acting as the gateway cities in the region. Combined with , Tangshan belongs to the Tianjin-cored community and Langfang belongs to the Beijing-cored community. These two cities will take the lead in benefiting from the BTH Coordinated Development. Meanwhile, it indicates that the BTH region has developed a structure of ‘core city–gateway city–general city’, reflecting that the deconcentrated functions from Beijing and Tianjin have yielded preliminary results. But the present problem is that while Tangshan and Langfang have more frequent population movements with central cities in the post-epidemic period, they are less connected with general cities. Furthermore, Shijiazhuang-cored community lacks a clear gateway city, which will result in some limitations on economic growth and resource flows in southern Hebei Province.
5. Conclusion and policy implications
In this study, we build population mobility networks based on Baidu migration data of the BTH urban agglomeration in China. First, the population movement patterns pre- and post-epidemic are compared and analyzed. The findings demonstrate that the outbreak drastically decreased the level of population movement in this region. As the epidemic enters the normalized period, mobility behavior gradually resumes. However, sporadic outbreaks have a great impact on local mobility patterns. In addition, we observe that the epidemic exacerbates the core-periphery structure of the population mobility network. Beijing and Tianjin, as the economic development poles of the region, have strong recovery capacity and obvious demographic attractiveness. They form a highly resilient population mobility network with surrounding cities, but most of the cities in Hebei province have poor population aggregation functions and exist in population loss. And changes in the structural characteristics of the network imply that the tendency of clustering is becoming more obvious and that the epidemic raises the cost of population mobility between cities. This is highly likely to exacerbate the phenomenon of polarization of population mobility patterns. Subsequently, we use the Louvain algorithm to detect the community structure that exists within the network under the medium-term impact of the epidemic. Three communities exist in the BTH region, respectively Beijing, Tianjin, and Shijiazhuang as the core. Finally, the individual characteristics of urban nodes are explored based on the MAC-MAP model. According to the findings, urban nodes can be divided into three categories: Beijing and Tianjin, with high MAC and MAP, have the strongest power to control the population and resources in the region; Tangshan and Langfang, with relatively high MAC and MAP, are the gateway cities in the region and play the role of communicating; others are general cities, which are in the controlled position in the network. Combined with the results of the community division, located in the southern part of Hebei Province, the Shijiazhuang-cored community lacks a clear gateway city. This indicates that a reasonable population gradient distribution in the BTH region has not been fully developed.
Based on the primary findings, we propose several policy recommendations. The possibility of local epidemic outbreaks still exists, and robust emergency response mechanisms should be established. The spatio-temporal big data should be properly utilized to make timely assessments of the long-term impact caused by public health events. Precise policies that contribute to socio-economic recovery should be formulated for the characteristics of each city. The central cities of the region, Beijing, Tianjin, and Shijiazhuang, should play the leading and radiation-driven role in expanding the depth of inter-city cooperation in all aspects. The other cities need to improve their capacity for comprehensive carrying and service. Policymakers should take corresponding measures to encourage cooperation among multiple parties, promote the integration and complementation of industrial structures, and establish a reasonable urban division of labor system. For cities with serious population loss, reasonable spatial reallocation of public resources as well as increased fiscal spending are needed to attract the labor force to return. The excessive concentration of the population of Beijing and Tianjin should continue to be mitigated. An orderly deconcentrating of industries and population can be guided by measures in surrounding cities, such as increasing employment opportunities and improving the quality of public services.
Acknowledgements
We thank the editor and the anonymous reviewers for their constructive remarks that improved this paper.
Disclosure statement
No potential conflict of interest was reported by the author(s).
Data availability statement
The data that support the findings of this study are available from the corresponding author upon request.
Additional information
Funding
Notes
2 ME and MI data are not available for 2019.
3 https://www.ndrc.gov.cn/fggz/fzzlgh/dffzgh/202106/t20210611_1283092.html, visited in April 2023.
References
- Barrat A, Barthélemy M, Pastor-Satorras R, Vespignani A. 2004. The architecture of complex weighted networks. Proc Natl Acad Sci USA. 101(11):3747–3752. doi: 10.1073/pnas.0400087101.
- Blondel V, Guillaume J-L, Lambiotte R, Lefebvre E. 2008. Fast unfolding of communities in large networks. J Stat Mech Theory Exp. 2008(10):P10008.
- Bonaccorsi G, Pierri F, Cinelli M, Flori A, Galeazzi A, Porcelli F, Schmidt AL, Valensise CM, Scala A, Quattrociocchi W, et al. 2020. Economic and social consequences of human mobility restrictions under COVID-19. Proc Natl Acad Sci USA. 117(27):15530–15535. doi: 10.1073/pnas.2007658117.
- Boschma R. 2015. Towards an evolutionary perspective on regional resilience. Reg Stud. 49(5):733–751. doi: 10.1080/00343404.2014.959481.
- Davis MA, Fisher JDM, Whited TM. 2014. Macroeconomic implications of agglomeration. Econometrica. 82(2):731–764.
- Derudder BEN, Taylor P. 2005. The cliquishness of world cities. Glob Netw. 5(1):71–91. doi: 10.1111/j.1471-0374.2005.00108.x.
- Fang Y, Wang J, Fu S, Zhai T, Huang L. 2022. Changes in ecological networks and eco-environmental effects on urban ecosystem in China’s typical urban agglomerations. Environ Sci Pollut Res Int. 29(31):46991–47010. doi: 10.1007/s11356-022-19226-7.
- Fortunato S. 2010. Community detection in graphs. Phys Rep. 486(3–5):75–174. doi: 10.1016/j.physrep.2009.11.002.
- García-Palomares JC, Salas-Olmedo MH, Moya-Gómez B, Condeço-Melhorado A, Gutiérrez J. 2018. City dynamics through Twitter: relationships between land use and spatiotemporal demographics. Cities. 72:310–319. doi: 10.1016/j.cities.2017.09.007.
- Glaeser E. 2012. Triumph of the city: how our greatest invention makes us richer, smarter, greener, healthier, and happier.
- Han J, Gao M, Sun Y. 2019. Research on the measurement and path of urban agglomeration growth effect. Sustainability. 11(19):5179. doi: 10.3390/su11195179.
- He X, Yuan X, Zhang D, Zhang R, Li M, Zhou C. 2021. Delineation of urban agglomeration boundary based on multisource big data fusion—a case study of Guangdong–Hong Kong–Macao Greater Bay Area (GBA). Rem Sens. 13(9):1801. doi: 10.3390/rs13091801.
- Kraemer MUG, Sadilek A, Zhang Q, Marchal NA, Tuli G, Cohn EL, Hswen Y, Perkins TA, Smith DL, Reiner RC, et al. 2020. Mapping global variation in human mobility. Nat Hum Behav. 4(8):800–810. doi: 10.1038/s41562-020-0875-0.
- Kumar K, Castells M. 1997. The information age: economy, society and culture. Volume I. The rise of the network society. Br J Sociol. 48(3):524. doi: 10.2307/591145.
- Li J, Ye Q, Deng X, Liu Y, Liu Y. 2016. Spatial-temporal analysis on spring festival travel rush in China based on multisource big data. Sustainability. 8(11):1184. doi: 10.3390/su8111184.
- Lin J, Yang S, Liu Y, Zhu Y, Cai A. 2023. The urban population agglomeration capacity and its impact on economic efficiency in the Yangtze River Delta Urban Agglomeration. Environ Dev Sustain. 1–30. doi: 10.1007/s10668-023-03242-9.
- Liu L. 2020. Emerging study on the transmission of the Novel Coronavirus (COVID-19) from urban perspective: evidence from China. Cities. 103:102759. doi: 10.1016/j.cities.2020.102759.
- Liu W, Guo J, Wu W, Cao Y. 2022. The evolution of regional spatial structure influenced by passenger rail service: a case study of the Yangtze River Delta. Growth Change. 53(2):651–679. doi: 10.1111/grow.12601.
- Liu W, Hou Q, Xie Z, Mai X. 2020. Urban network and regions in China: an analysis of daily migration with complex networks model. Sustainability. 12(8):3208. doi: 10.3390/su12083208.
- Liu S, Xiao Q. 2021. An empirical analysis on spatial correlation investigation of industrial carbon emissions using SNA-ICE model. Energy. 224:120183. doi: 10.1016/j.energy.2021.120183.
- Losacker S. 2022. ‘License to green’: regional patent licensing networks and green technology diffusion in China. Technol Forecast Social Change. 175:121336. doi: 10.1016/j.techfore.2021.121336.
- Malik HAM. 2022. Complex network formation and analysis of online social media systems. Comput Model Eng Sci. 130(3):1737–1750.
- Neal Z. 2011. Differentiating centrality and power in the world city network. Urb Stud. 48(13):2733–2748. doi: 10.1177/0042098010388954.
- Neal Z. 2013. Does world city network research need eigenvectors? Urb Stud. 50(8):1648–1659. doi: 10.1177/0042098013477702.
- Newman MEJ. 2006. Modularity and community structure in networks. Proc Natl Acad Sci USA. 103(23):8577–8582. doi: 10.1073/pnas.0601602103.
- Onnela J-P, Saramäki J, Kertész J, Kaski K. 2005. Intensity and coherence of motifs in weighted complex networks. Phys Rev E Stat Nonlin Soft Matter Phys. 71(6 Pt 2):065103. doi: 10.1103/PhysRevE.71.065103.
- Opsahl T, Agneessens F, Skvoretz J. 2010. Node centrality in weighted networks: generalizing degree and shortest paths. Soc Netw. 32(3):245–251. doi: 10.1016/j.socnet.2010.03.006.
- Pan F, Fang C. 2022. Mapping global financial networks: a spatial analysis of Chinese companies’ overseas listings. Trans Plan Urb Res. 1(1–2):32–49. doi: 10.1177/27541223221116124.
- Pan J, Lai J. 2019. Spatial pattern of population mobility among cities in China: case study of the National Day plus mid-autumn festival based on Tencent migration data. Cities. 94:55–69. doi: 10.1016/j.cities.2019.05.022.
- Qazi A, Simsekler MCE, Akram M. 2021. Efficacy of early warning systems in assessing country-level risk exposure to COVID-19. Geomatics Nat Hazards Risk. 12(1):2352–2366. doi: 10.1080/19475705.2021.1962984.
- Ruan J, Chen Y, Yang Z. 2021. Assessment of temporal and spatial progress of urban resilience in Guangzhou under rainstorm scenarios. Int J Disaster Risk Reduct. 66:102578. doi: 10.1016/j.ijdrr.2021.102578.
- Santamaria C, Sermi F, Spyratos S, Iacus SM, Annunziato A, Tarchi D, Vespe M. 2020. Measuring the impact of COVID-19 confinement measures on human mobility using mobile positioning data. A European regional analysis. Saf Sci. 132:104925. doi: 10.1016/j.ssci.2020.104925.
- Serbanica C, Constantin DL. 2023. Misfortunes never come singly. A holistic approach to urban resilience and sustainability challenges. Cities. 134:104177. doi: 10.1016/j.cities.2022.104177.
- Shen W, Liang H, Dong L, Ren J, Wang G. 2021. Synergistic CO2 reduction effects in Chinese urban agglomerations: perspectives from social network analysis. Sci Total Environ. 798:149352. doi: 10.1016/j.scitotenv.2021.149352.
- Sigler T, Martinus K, Loginova J. 2021. Socio-spatial relations observed in the global city network of firms. PLOS One. 16(8):e0255461. doi: 10.1371/journal.pone.0255461.
- Taylor P, Derudder B. 2015. World city network: a global urban analysis. London: Routledge.
- Wang L, Xue X, Zhou X, Wang Z, Liu R. 2021. Analyzing the topology characteristic and effectiveness of the China city network. Environ Plan B Urb Anal City Sci. 48(9):2554–2573. doi: 10.1177/2399808320983011.
- Wu K, Wu J, Li Y. 2022. Mining typhoon victim information based on multi-source data fusion using social media data in China: a case study of the 2019 Super Typhoon Lekima. Geomatics Nat Hazards Risk. 13(1):1087–1105. doi: 10.1080/19475705.2022.2064774.
- Xu S, Wang X, Zhu R, Wang D. 2023. Spatio-temporal effects of regional resilience construction on carbon emissions: evidence from 30 Chinese provinces. Sci Total Environ. 887:164109. doi: 10.1016/j.scitotenv.2023.164109.
- Yang X, Derudder B, Taylor PJ, Ni P, Shen W. 2017. Asymmetric global network connectivities in the world city network, 2013. Cities. 60:84–90. doi: 10.1016/j.cities.2016.08.009.
- Yang H, Hu S, Zheng X, Wu Y, Lin X, Xie L, Shen Z. 2022. Population migration, confirmed COVID-19 cases, pandemic prevention, and control: evidence and experiences from China. Z Gesundh Wiss. 30(5):1257–1263. doi: 10.1007/s10389-020-01403-y.
- Zhao R, Fang C, Liu J, Zhang L. 2022. The evaluation and obstacle analysis of urban resilience from the multidimensional perspective in Chinese cities. Sustainable Cities and Society. 86:104160. doi: 10.1016/j.scs.2022.104160.
- Zhao Z, Wei Y, WS, Pang R. 2017. Measurement of directed alternative centricity and power of directed weighted urban network: a case of population flow network of China during “Chunyun” period. Geogr Res. 36(4):647–660.
- Zhu R, Wang Y, Lin D, Jendryke M, Xie M, Guo J, Meng L. 2021. Exploring the rich-club characteristic in internal migration: evidence from Chinese Chunyun migration. Cities. 114:103198. doi: 10.1016/j.cities.2021.103198.