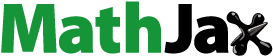
Abstract
Karakoram Himalayas are vulnerable to glacial lake outburst floods (GLOFs), which cause catastrophic floods in the surrounding areas. The increasing natural and anthropogenic activities, especially in the Indo-Gangetic Plains at the southern flank of the towering Himalayas, could be the cause of climate change affecting the frequency of the natural hazards in the Himalayas. In the present study, a detailed analysis of the Shisper Lake breach of 7 May 2022 is carried out using satellite remote sensing. A decreasing trend in the glacial mass balance is observed between 2017 and 2021; in this period, frequent GLOF episodes occurred. A pronounced decline in the snow cover and glacial debris (before the cascade) and an abnormal increase in land surface temperature (LST) retrieved from satellite data could be responsible for the enhanced snow/glacial melting prior to the breach. Alteration in glacial mobility (cm/12 days) is observed at the locations where the glacier melts due to an increase in LST with increased debris cover. In the paper, we have studied changes in water quality and land cover along the stream after the GLOF. An increment in normalized difference turbidity index (NDTI) is observed before the flood showing turbulence and sediment load. Increased glacial melt contribution into the stream results in increased flow rate and decreased water quality as observed through normalized difference water index (NDWI) and normalized difference chlorophyll index (NDCI), near the Karakoram Highway Bridge. Sentinel-1 backscatter coefficient indicates increased discharge from the melting of glaciers. A significant reduction in co-flood coherence is observed, attributed to the bridge collapse and severe bank erosion.
1. Introduction
The Himalaya-Karakoram (HK), a 2500 Km long mountain range, covers many glaciers. The mega river systems, Brahmaputra, Ganges, and Indus originate from the Himalaya-Karakoram glaciers (Immerzeel et al. Citation2010). The human civilization of more than 750 million is, directly and indirectly, dependent on these river systems (Pritchard Citation2017). Many scientists have used both field and satellite-based techniques to study the Himalayan cryosphere in terms of source endmembers to the perennial river systems, spatio-temporal fluctuations in annual precipitation, site-specific characteristics of agricultural practices as well as adaptation strategies (Winiger et al. Citation2005; Nüsser et al. Citation2019; Mondal et al. Citation2023). Scientists from different parts of the world started showing interest to study the Karakoram glaciers after the Intergovernmental Panel on Climate Change (IPCC) indicated an alarming loss of glaciers in the area by 2035 (Cogley et al. Citation2010) which was corrected by the IPCC (Vaughan et al. 2013). Bolch et al. (Citation2012) assessed the condition of glaciers in the Himalaya-Karakoram (HK) region and emphasized the importance of conducting on-site mapping as the glacial bodies of the region exhibit significant variations. Weather station data show that the Karakoram experienced less warming as compared to other parts of the Himalayan Range (Shekhar et al. 2010). Also, the famous ‘Karakoram Anomaly’ (Hewitt Citation2005; Kääb et al. Citation2012) explains the presence of advancing or stable glaciers mostly around the western Kunlun Shan region (Kääb et al. Citation2015) as compared to other receding glaciers worldwide (Zemp et al. 2015). In fact, recent study conducted to understand the long-term glacial dynamics of Nanga Parbat shows similitude with the Karakoram Anomaly (Nüsser and Schmidt Citation2021).
The glacial hazards in high mountain areas are frequent over the globe, recent disasters (Chamoli landslides, floods, forest fires, earthquakes, and landslides) in the Himalayas are evident that cause loss of human lives and infrastructural damages, affecting day-to-day activities (Qin et al. Citation2018, Meena et al. Citation2021a,Citation2021b,Citation2021c). Natural hazards, such as snow avalanches, glacial surges, glacial lake outburst floods (GLOFs), glacial collapse, and debris flow (Richardson and Reynolds Citation2000) occur in this region. Glacial recession, particularly in the Karakoram Mountain region, led to the formation of several glacial lakes with high prospective risk due to GLOF (Nie et al. Citation2013). Himalayas have evidenced GLOF events of varying degrees of severity once every three to ten years (Bajracharya et al. Citation2007). Detailed investigation of GLOF events and risk assessment of glacial lakes to future GLOFs is of paramount importance (Schmidt et al. Citation2020; Mondal and Bharti, Citation2022a) especially under the climate change scenarios. Especially the Hindu Kush-Karakoram-Himalayan region has witnessed numerous outbursts/flash floods in recent decades (Wester et al. Citation2019). Out of these unfortunate incidents, most of the GLOFs occurred in the Gilgit-Baltistan region of Karakoram especially due to severe rainfall, abrupt rise in temperature, and heatwaves (Ashraf et al. Citation2021). Some of the recent incidents include the 2007 and 2008 GLOF on Passu glacier (Ashraf et al. Citation2014), the 2013 GLOF in Reshun valley (Riaz et al. Citation2014), and GLOF events during 2008 - 2017 due to the sudden breach of the supraglacial lake on Ghulkin glacier and many more. The Hassanabad basin is another region that is highly susceptible to outburst floods attributed to the active movement of the Shisper and Muchowar glaciers (Khan et al. Citation2021). The Hassanabad ravine witnessed five minor GLOF events during 1890 − 1905 (Hewitt and Liu Citation2010). Recently, three major GLOF events have been observed in 2019, and 2020 (Muhammad et al. Citation2021). The recent Chamoli disaster occurred on 7 Feb 2021 which took more than 150 lives (Pandey et al. Citation2022, Mao et al. 2021, Meena et al. Citation2021a, Citation2021b), the surrounding rivers were flooded that affected river water quality (Meena et al. Citation2021a, Citation2021b, Citation2021c; Mao et al. 2021).
The towering Himalaya is located at the northern flanks of the Indo-Gangetic Plains (IGP), the outflow emissions from the IGP due to growing anthropogenic activities and industrial emissions affect the Himalayan region (Singh and Chauhan, 2021). The long-term growing greenhouse emissions are the drivers for climate change affecting the snow/glaciers of the Himalayas and affecting the outburst of the glacial lakes in the Himalayan region. In this regard, satellite remote sensing has proved to be one of the important tools in mapping and monitoring the state of art of snow/glacier surfaces, glacial lakes, and their parent glaciers (Wang et al. Citation2015; Patel et al. Citation2019). The Himalayan region contains 15000 glacial bodies and 9000 glacial lakes (Mool et al. 2005), within the Hindu-Kush region, 8790 glacial lakes have been identified by Ives et al. (Citation2010) out of which 203 lakes were identified as the most vulnerable for the future GLOFs. In fact, numerous naturally formed isolated lakes are located far from the glacial bodies devoid of any glacial origin. Some of these lakes situated above 3500 m a.s.l are seen to be the remnants of those glacial lakes left due to glacial retreat (Campbell, 2005). In the past few years, the retreat of glaciers has not only affected the hydrological processes but also led to the volumetric expansion of glacial lakes (Yao et al. 2010). Watanabe et al. (Citation1994) observed the increase in the volume of glacial lakes in Khumbu Himalaya and used it to assess the potential of GLOF. Lichtenegger et al. (Citation2008) employed microwave remote sensing to detect the variations of Imja glacial lake. Kumar et al. (Citation2020) used optical remote sensing data (Landsat-7/8 and Sentinel-2) to develop an inventory of glacial lakes in the Karakoram Himalayan region. However, there are certain limitations in using optical remote sensing for mapping glacial lakes which include obscuring cloud cover, shadow effect from mountains, seasonal snow, degree of turbidity of lake water, and frozen lake surfaces (Schmidt et al. Citation2020). The use of synthetic aperture radar (SAR) data is considered robust for identifying glacial lakes that are partially frozen, however, noise removal relies on manual corrections and auxiliary datasets (Wangchuk et al. Citation2019).
The optical and microwave remote sensing tools have proved to be important in monitoring the glacial lakes and associated changes especially in the mountainous region. Where an individual satellite system can provide information in a specific configuration (temporal, spatial, spectral and radiometric resolution), studying hazard cascades using multiple satellite systems can improve the monitoring process and investigation for plausible causative factors affecting substantially. Moreover, where optical remote sensing may face challenges due to long revisit time and obscuring cloud coverage, using the datasets of satellite constellations (Landsat-8/9 and Sentinel-2A/B) along with space-borne radar system (Sentinel-1) can be much more informative. Recently combined optical and radar data have provided information about the changes in the land cover, water bodies, water quality, liquid water content, and snow/glaciers (Meena et al. Citation2021a,Citation2021b). The change in the spectral response of water to changing sediment load, turbidity, and chlorophyll (Chlo) contamination are being monitored qualitative as well as quantitative (Ritchie et al. Citation2003, Meena et al. Citation2021a, Citation2021b, Citation2021c). In this study, an attempt has been made to use optical, thermal, and SAR data prior and after the breach of Shisper glacial lake occurred on 7 May 2022. We have discussed in detail, possible factors responsible for the fast melting of the Shisper and Muchowar glaciers and associated changes after the breach.
2. Study area
Shisper Glacier is the western component of the Hassanabad glacier, with the Muchuwar glacier in the east (Muhammad et al. Citation2021). The glacier belongs to the group of Passu mountain glaciers that lies in the upper Indus basin within the western Karakoram belt of the Himalayan Mountain range. Shisper and Muchuwar glaciers share a common tongue with an ice-dammed glacial lake at the snout of the Shisper glacier. Lying between 36° 15′ to 36° 30′ N latitudes and 74° 28′ to 74° 39′ E longitudes, the Shisper glacier acquires a total area of 54.7 km2. Similar to the other valley glaciers of Karakoram, Shisper and Muchuwar glacier has a main trunk lying between the elevation range of 5267 and 7611 m a.s.l, the glacier delivers its melt contribution into the Hunza River, as shown in . The region receives 125 mm of annual precipitation with a mean average temperature of 11 °C. Snow and glacial melt water serve as the primary source for agrarian practices influencing landuse patterns and irrigation networks (Parveen et al. Citation2015). However, the steady mobility of the glacier leads to blockage of the outlet from the Muchuwar glacial snout. There have been several episodes of outburst floods associated with the ice-dammed glacial lake at the snout of Shisper Glacial body since 1890. Some of the recent GLOFs occurred in the month of June 2019 and May 2020, affecting the downstream areas.
Figure 1. Study area map showing satellite view of the region and Shisper glacial lake: (A) major locations, glacial bodies, road networks and lake (B) Lake after the breach of 7 May 2022 (lake area: 0.21 km2), (C) Lake before the breach on 17 April 2022 (lake area: 0.26 km2), (D) 30 April 2022 (lake area: 0.30 km2), (E) 2 May 2022 (lake area: 0.37 km2), and (F) 5 May 2022 (lake area: 0.38 km2).

It was on 7 May 2022 that a massive outburst of flood occurred due to a breach of Shisper glacial lake. Experts stated that an anomalous increment in the melting rate of the parent glacier due to an abrupt rise in temperature by around 5 °C (heat waves) resulted in a more than 40% hike in the lake volume in the past 20 days, thus resulting in a breach. The melt contribution from the parent glacier into the lake reached up to 5000 cusecs (1 cusec = 28.32 litres/sec) before the collapse. The International Centre for Integrated Mountain Development (ICIMOD) revealed that the pre-breach size of Shisper lake had been 15% more than the recorded size in the past three years (https://www.icimod.org/increasing-risk-of-glacial-lake-outburst-floods-in-hunza-river-basin/). The outburst flood led to the loss of life and property along with the destruction of the Hassanabad bridge built on the Karakoram highway over the Hunza river.
3. Data and methods
In the present study, an attempt has been made to utilize optical and microwave remote sensing data to study the possible triggering mechanism of the glacial lake breach. Landsat-8/9 Operational Land Imager (OLI), Sentinel-2 Multi-Spectral Instrument (MSI), and Moderate Resolution Imaging Spectroradiometer (MODIS) products of surface spectral reflectance (MOD09GA) have been used to study the anomalous region. Landsat-8/9 provides a resolution of (30 m) in eleven spectral channels between 0.43 µm and 12.51 µm, covering the visible, near-infrared (NIR), shortwave infrared (SWIR), and thermal infrared (NIR) region of the electromagnetic (EM) spectrum. Sentinel-2 provides information in thirteen spectral channels devoid of thermal bands between 0.43 µm and 2.28 µm capturing visible (10 m), NIR (20 m), and SWIR (60 m) regions of the EM spectrum. As Landsat-8 and Landsat-9 are identical twins in terms of their spectral, spatial, radiometric, and temporal resolution, utilizing both datasets improve the time interval of data acquisition (<16 days) as shown in . This is an advantage in studying natural hazards such as floods associated with the melting of snow/glaciers. The Atmospheric Infra-Red Sounder (AIRS) skin temperature data is taken through NASA Giovanni portal for the months of May 2021 and May 2022 over the Shisper glacier location in the box size 10×1° to study the variations in the daily temperature. AIRS is a high ground resolution infrared sounder launched on Earth Observing System (EOS) Aqua acquiring geophysical parameters. Sentinel-1 level-I ground range detection (GRD) and single look complex (SLC) datasets have been used to derive the surface mobility of glacial bodies and alterations along the Hunza river stream. Sentinel-1 mission is equipped with two satellites, namely Sentinel-1A/B revolving in near-polar orbits providing datasets within 12 and 6 days as independent and pair satellites respectively. The satellites acquire C-band synthetic aperture radar (SAR) data in 5.405 GHz (central frequency) in single (HH, HV) as well as dual (VV + VH, HH + HV) polarization schemes (Filipponi Citation2019). Moreover, a high-resolution digital elevation model (DEM) in 12.5 m spatial resolution from Advanced Land Observing Satellite (ALOS) polarimetric Phased Array L-band Synthetic Aperture Radar (PALSAR) has been utilized to derive the stream networks around the Hassanabad region of Hunza river valley. PALSAR is a microwave sensor operating in L-band and acquiring data in all weather conditions. The DEM derived using PALSAR is transformed from orthometric height to ellipsoidal height to match the elevation profile with other available DEMs. The glacial boundaries in the area including Shisper and Muchuwar glaciers have been used from Randolph glacial inventory (RGI 6.0).
Table 1. Datasets used in the study (* denotes the polarization used in the study).
Using multi spectral satellite data, we have developed several indices providing information about the several plausible factors/parameters associated with the GLOF event. Our detailed analysis based on several indices using optical, thermal, and microwave remote sensing data () shows pronounced changes in several parameters of snow and water.
Figure 2. Flowchart of the optical (Landsat-8/9 & Sentinel-2) and SAR (Sentinel-1) datasets used and adopted methods to investigate the triggering factors and after effects of Shisper glacial lake breach.

3.1. Accumulation area ratio (AAR)
The rugged and complex topography and day-to-day variability in the weather conditions restrict scientists to carry out limited ground-based mass balance monitoring to very few glaciers for a few years. Some discrete data are available which may be of poor quality due to limited logistics and severe climatic conditions. The Accumulation-Area-Ratio (AAR) method was established to compute the mass balance of glaciers through satellite-based observations (Kulkarni Citation1992). The regression equation for the Karakoram Himalayas is given below:
(1)
(1)
NDSI (Normalized Difference Snow Index) threshold of 0.75 ∼ 0.96 can be used for the extraction of the firn line for the given region (Zhau et al. 2020). Using NDSI and firn line threshold, the accumulation area of the glacier can be extracted during the end of the ablation period i.e. in the month of Mid-September to Early November. The accumulation area ratio is calculated using following equation:
(2)
(2)
3.2. Normalized Difference Snow Index (NDSI)
NDSI (Nolin and Liang Citation2000) is defined as the difference in the reflectance in the visible (green band) and SWIR (1.57–1.65 µm) band divided by the sum of reflectance in the two bands (Equationequation 3(3)
(3) ).
(3)
(3)
NDSI distinguishes snow from clouds and other non-snow areas. Moreover, this band ratio minimizes the influence of viewing geometry and atmospheric effects (Salomonson and Appel Citation2004). A threshold of 0.4 has been considered to mask out surficial snow from other surface features (Hao et al. Citation2008)
3.3. Normalized difference dust index (NDDI)
NDDI (Qu et al. Citation2006) is devised as the difference in the reflectance of the 2.13 μm (band 7) band and 0.469 μm (band 3) band of MOD09GA (Equationequation 4(4)
(4) ).
(4)
(4)
NDDI helps differentiate between dust, surface features, and obscuring cloud coverage. NDDI < 0.0 represents cloud cover because of lower reflectance at band 7 and higher reflectance at band 3. The NDDI threshold of 0.28 helps in distinguishing between surface features (NDDI < 0.28) and dust (NDDI ≥ 0.28).
3.4. Normalized difference water index (NDWI)
NDWI (Equationequation 5(5)
(5) ) is developed by Gao et al. (1996) for the detection of water content using MODIS data and surface water features (McFeeters Citation1996).
(5)
(5)
In the case of floods, NDWI is based on the reflectance of bands 5 and 3, for assessing water quality as positive pixel values represent clear water in NDWI output that might shift towards negative values due to mud/debris contamination (Meena et al. Citation2021b).
3.5. Normalized difference turbidity index (NDTI)
NDTI (Equationequation 6(6)
(6) ) has been used as a supportive band ratio to the NDWI.
(6)
(6)
where
and
are the reflectance response in the green and red bands of Landsat-8/9 datasets, respectively. The positive values of this band ratio represent turbid water. Pixels showing NDTI values in the range of −0.03 and 0.03 indicate arable land cover with vegetation (Solovey Citation2019).
Even though NDWI can distinguish between clean and muddy water after an outburst flood, NDTI uses the reflectance response of green and red bands to detect turbid/muddy water in satellite imagery.
3.6. Normalized difference chlorophyll index (NDCI)
Over the past few years, several algorithms have been designed to quantify chlorophyll (Chlo-a) concentration in turbid productive water bodies particularly using NIR bands (Mishra and Mishra Citation2012). The Chlo-a concentration of river water gets altered soon after a flood event that can be easily detected using following NDCI equation.
(7)
(7)
where
and
are the reflectance in the red edge (0.698–0.713 µm) and red (0.65–0.68 µm) band for Sentinel-2 MSI datasets respectively. Once NDCI is derived, the Chal-a concentration (µg/L) can be derived using following Equationequation 8
(8)
(8) (Mishra and Mishra Citation2012).
(8)
(8)
Higher the value of NDCI, the higher is the Chlo-a concentration; thus, the poorer is the water quality (USEPA, Citation2008). NDCI value of 0.06 corresponds to a 20 µg/L Chlo-a concentration which is the lower contamination limit. A negative range of NDCI represents overflow conditions followed by lower Chlo-a concentration.
3.7. Debris index
Glaciers are composed of debris especially the Himalayan Glaciers (Hewitt Citation2009). Debris index (Equationequation 9(9)
(9) ) is widely used automated algorithms to distinguish debris-covered ice from clean snow/ice is the band ratio technique (Pfeffer et al. Citation2014).
(9)
(9)
where
is the reflectance in SWIR (1.565–1.655 μm) band for Sentinel-2 MSI datasets used. The threshold of 1.8 is considered to distinguish clean ice glacier areas from debris-covered areas.
3.8. Land surface temperature (LST)
In order to understand the impact of sudden increase in temperature (heat waves) resulting in enhanced melting of glacial bodies, it is important to analyse the surface temperature of the glaciers. The thermal infrared bands of Landsat-8/9 are widely used in deriving the LST of an area as shown in . The first step involves obtaining top of the atmosphere (TOA) spectral radiance (Lλ) (Equationequation 10(10)
(10) ) in watt m−2 srad−1 µm−1 of the TIR band (band 10).
(10)
(10)
where M and A represent the multiplicative and additive rescaling factors for Landsat-8/9 datasets as mentioned in and represents band 10 digital number (DN) value. The next step involves determining the TOA brightness temperature (BT) which is the microwave radiation radiance from the top of the atmosphere as shown in Equationequation (11)
(11)
(11) (USGS, 2013; Mondal and Bharti, 2021).
(11)
(11)
where K1 and K2 are the thermal constants for band 10 given in .
Table 2. Coefficients used in deriving LST from band-10 of Landsat-8/9.
This study adopted the normalized difference vegetation index (NDVI) threshold method to derive the emissivity (Avdan and Jovanovska Citation2016). The importance of calculating NDVI (Equationequation 12(12)
(12) ) is crucial in obtaining the proportion of vegetation (Pv) which has a relation to emissivity (ε).
(12)
(12)
Pv is calculated using the NDVI threshold for soil (0.5) and vegetation (0.2) as shown in Equationequation 13(13)
(13) , (Wang et al. Citation2015; Avdan and Jovanovska Citation2016). Further, Pv is used to derive the emissivity (Equationequation 14
(14)
(14) ). Finally, the emissivity corrected LST is calculated as shown in Equationequation 15
(15)
(15) , (Stathopoulou and Cartalis, 2007).
(13)
(13)
(14)
(14)
(15)
(15)
where λ is taken as 10.895 which is the wavelength of emitted radiance for band 10 and ρ is calculated using Equationequation 16
(16)
(16) .
(16)
(16)
where
is the Planck’s constant (6.626 × 10−34 Js), c is the velocity of electromagnetic radiation (2.998 × 108 ms−1), and σ is the Boltzmann constant (1.38 × 10−23 JK−1).
3.9. Intensity offset tracking
In the present study, SAR sub-pixel intensity offset tracking has been used. The method of cross-correlation optimization has been adopted for the GRD products of Sentinel-1 and processed in the Sentinel Application Platform (SNAP) designed by European Space Agency (ESA) as shown in . The first step involves assigning the precise orbit vectors to the pair of SAR imagery which is followed by thermal noise removal (TNR) since it disturbs the intensity of the SAR pairs. The TNR reduces this disturbance in inter-sub-swath texture, thus normalizing the backscatter signal resulting in minimum discontinuities between the sub swaths (Filipponi Citation2019). The next step is radiometric calibration which helps in acquiring radiometrically calibrated backscatter for the SAR imagery. The sub-pixel offset tracking has been applied over the radiometrically corrected pairs. The matching kernel (feature kernel) of 128 × 128 pixels (azimuth × range) and searching kernel of 10 × 10 pixels have been used. Oversampling factor and correlation coefficient threshold of 16 and 0.3 has been assigned respectively. The cross-correlation threshold helps mask out unreliable offsets as a result of pixel distortions and sharp surface changes (Zhu et al. 2021). Finally, the derived velocity vectors are checked for the user-defined threshold of maximum velocity. From previous studies conducted over the western Himalayan glaciers, a 15 m/day velocity threshold has been set (Amitrano et al. Citation2019). The Shisper glacier is a region of glacial surging; therefore, maximum velocity threshold has been assigned to capture anomalous displacements if any and prevent any underestimation of surface velocity. Finally, the obtained surface velocity outputs have been corrected for geometric distortions such as foreshortening and shadows due to topography through range doppler terrain correction (RDTC) using the Shuttle Radar Topography Mission (SRTM) 1 arcsec DEM.
3.10. Interferometric coherence
Interferometric coherence has applications in various types of natural hazards such as earthquakes, landslides and GLOFs for monitoring topographic changes (Zhang et al. Citation2020). However, coherence being a statistical value, the magnitude of ground displacements cannot be quantified (Closson and Milisavljevic Citation2017). Coherence is produced using differential interferometry (DInSAR) as shown in and represents the quality of the produced interferogram. The coherence image shows similarity in reflected radar signals between a pair of SAR patches. Processing begins with considering an SLC pair where one image serves as a master/reference image (pre-date file) and the other image as a slave/test image (post-date file). This is followed by modifying the orbit state vector of both datasets using precise orbit ephemeris (https:/qc.sentinel1.eo.esa.int/). One SLC file is composed of three sub-swaths. Therefore, in order to minimize the data bulk and computational time, interferometric wide swath sub-swath-1 (IW-1) has been extracted using TOPSAR split. Back geocoding has been performed through which the SAR coordinates are transferred to general cartesian coordinates with the help of SRTM 1 arcsec DEM and very precise orbits. This is followed by enhanced spectral diversity (ESD) which rectifies the residual errors during co-registration. It uses the phase differences between SAR pair and derives azimuth errors in co-registration from differential phase. The next step is the formation of interferogram by cross-multiplication of the reference image with the complex conjugate of the test image. This way, the phase and amplitude difference between SAR pair is generated. To emphasize phase signatures associated with surficial deformation, removing topographic phase contributions using DEM is necessary. The upcoming step is TOPSAR deburst which helps to merge the burst related to the region of interest. Further, multi-look helps eradicate speckle and improves the output quality (Cantalloube, 2014). The phase noise is further wrapped using Goldstein phase filter (5 × 5 kernel size). Finally, RDTC is executed to derive terrain corrected interferometric coherence of the area.
3.11. Backscatter coefficient (dB)
The backscatter coefficients derived from Sentinel-1 products indicate the reflective strength of the surface (Perrou et al. Citation2018, Meena et al. Citation2021a,Citation2021b). Stendardi et al. (2019) explained that the range of backscatter coefficients can be used to classify different surface features (). Once the SLC datasets are corrected for orbit and sub-swath (IW-1), it is extracted through TOPSAR split (as shown in ), followed by the radiometric calibration. The next step is TOPSAR deburst and multi-look followed by Linear to dB scale conversion to derive backscatter intensity products. Finally, RDTC is done to obtain terrain-corrected backscatter coefficients in decibels.
Table 3. Classification of Sentinel-1 derived backscatter coefficient (Stendardi et al. 2019).
4. Results and discussion
4.1. Plausible factors triggering breach
In order to understand the mechanism that might have triggered the Shisper glacial lake breach, a series of methods have been adopted. Firstly, the region has been analysed for the variation in the glacier accumulation area and AAR of the Shisper glacier. A decreasing accumulation (dotted line shows a trend of the AAR) can be seen in recent years during 2017 and 2021 (). The highest mass gain (0.417 m w e) in 2017 and the lowest (0.172 m w e) in 2021 can be seen. Mean mass fluctuation of 0.308 ± 0.098 m w e is observed between 2017 and 2021 when two major GLOF events occurred (Muhammad et al. Citation2021). The specific mass balance decreased during the years 2019–2021. From the optical datasets of Landsat-8/9, NDSI results show a significant reduction in the snow coverage of the area since 17 April 2022 as shown in . The threshold of 0.4 has been used to monitor the temporal changes in surficial snow. The NDSI output of 17 April shows 59.28% of the area under snow reduces to 52.11% by 2 May 2022 and further significantly reduces to 38.51% by 10 May 2022. Such reduction in NDSI is likely to be associated with the long-term transport of dust over the snow/glaciers which are common over the western Himalayas (Prasad and Singh Citation2007a,Citation2007b). The NDDI result of 17 April 2022 shows 671.2 km2 of area characterized as dusty region that further increases to 738.6 km2, and 946.2 km2 on 2 May 2022 and 10 May 2022 respectively (). The earlier studies conducted over the Karakoram Himalayas reveal a gradual accumulation of dust between April and June (due to enhanced convection) with greatest influence of dust during May-June (before the onset of summer monsoon) (Gautam et al. Citation2013) as also observed in the present study. Since the GLOF incident occurred during the month of May, the end of winter/accumulation period in the Karakoram Himalayas, NDSI results are insufficient to understand the phenomenon of the fast melting of Shisper glacial mass. However, a steady decrease in the NDSI value indicates a reduction in the surficial snow mainly due to melting. The likely cause of the melting could be associated with the anthropogenic emissions from the local and outflow from the IGP and long-range transport of airmass reaching over the Shisper and surrounding regions which is clearly seen from the HYSPILT back trajectories (). The airmass trajectories are observed after the 120 min run of HYSPILT model in different months in the year 2022. showing airmass trajectories reaching from the local, outflow from IGP and long-range transport. These airmass blanket the snow/glaciers, the airmass could be dust or carbon soot from the anthropogenic activties that affect the albedo of snow/glaciers, and thus the accelerated melting occurs causing outburst of the frozen lakes also.
Figure 7. Temporal changes in the (A) AAR (the dotted line shows a trend) and fluctuations in the (B) mass balance of Shisper glacier.

Figure 8. NDSI results showing snow coverages on (A) 17 April 2022 (1523.4 km2), (B) 2 May 2022 (1339.1 km2), (C) 10 May 2022 (989.6 km2) as well as debris cover within the glacial body on (D) 30 April 2022 (36.3 km2 accounting for 27.2%) and (E) 10 May 2022 (43.1 km2 accounting for 32.3%).

Figure 9. NDDI results showing dust coverages on (A) 17 April 2022 (671.2 km2), (B) 2 May 2022 (738.6 km2) and (C) 10 May 2022 (946.2 km2).

Figure 10. Airmass trajectories from HYSPILT model on (A) 6 May 2022, (B) 23 May 2022, and (C) 6 November 2022.

Snow has a much higher albedo compared to the firn/glacial ice (Winther Citation1993). The radiative heat flux plays a significant role in snow/glacial melting therefore, it is essential to understand the role of radiative heat flux in the fast-melting process of Shisper glacier. From the debris map derived from Sentinel-2 datasets, an increment in the debris coverage within the Shisper (rectangular zone) and Muchowar (oval zone) glacial bodies can be observed (). The exposed debris coverage within the glacial body increases by 6.8 km2 between 30 April and 10 May 2022, accounting for 5.1%. Such increase in the debris coverage or decrement in the fresh/clean snow cover (total glacial area – debris cover) corroborates well with the snow cover decrease as observed from NDSI (). Also, debris/contaminated ice has much lower albedo as compared to fresh/clean snow. Thus, increment in the debris cover supports more absorption of solar radiation by the glacial body, thus increasing the temperature profile, leading to enhanced melting.
Snow/glacial melting is a complex phenomenon that occurs due to changes in a set of fluxes such as radiative heat flux, sensible heat flux, precipitation heat flux, and latent heat flux (Che et al. Citation2019). Significant changes in the temperature profile around a glaciated region are indicative of rapid melting. From the LST derived using TIR band 10 of Landsat-8/9, a sudden increment in the surface temperature can be clearly observed (). The minimum and maximum temperature within the glacial body lies in the range of −26.1 °C and 26.9 °C respectively on 17 April. This increases drastically to −21.5 °C and 32.4 °C on 2 May, showing an increase in the surface temperature range by > 5 °C. Abrupt changes in the LST is indicative of rapid snow/glacial melting. Such changes occurred within 15 days between 17 April and 2 May and reached 34.1 °C as shown in the LST map () of 10 May 2022. The LST difference map () shows a maximum increase in LST (>12 °C) within the rectangular and oval zone of Shisper and Muchowar glaciers, respectively. These zones are the glacial trunk’s elevated regions that transfer the glacial melt discharge towards the snout where the glacial lake is located. The variations in the daily surface skin temperature of Shisper glacial region (at 1°x1°) for the month of May 2022 in comparison with May 2021 data shows a temperature offset (), the temperature varies in the range −3.5 °C in the month of May 2021 and 14.9 °C on 14 May and 27 May respectively, the maximum (23.4 °C) and minimum (1.9 °C) temperature, respectively on 12 and 24 May 2022. Before the glacier outburst (7 May 2022), a high temperature 17.5 °C was observed on 4 May 2022. The monthly mean temperature of May 2022 was 12 °C which was more than 4 °C compared to the mean monthly temperature of May 2021 (7.9 °C). The Chamoli deadly disaster occurred on 7 Feb 2021 due to sudden changes in the surface temperature (Meena et al. Citation2021a,Citation2021b). An increase in debris cover has also been observed within these zones, as shown in . Since debris coverage has a much lower albedo compared to the fresh snow cover, the overall absorption of solar radiation (watt/m2) increases within the glacial body, leading to a localized increase in the surface temperature, resulting in the fast melting of glacial ice. The low albedo shows the melting of snow/glaciers which could be also associated with the long-term transport of dust or black carbon from the outflow of the IGP (Chauhan et al. Citation2023).
Figure 11. Map showing major fluctuations in the LST around main trunk of Shisper glacier (rectangle) and Muchowar glacier (oval) as mapped on (A) 17 April 2022, (B) 2 May 2022, (C) 10 May 2022, and (D) LST difference between 2 May and 17 April 2022.

Figure 12. Fluctuation in the daily surface skin temperature (ºC) of Shisper glacial region in May 2021 and May 2022.

shows the changes in the pattern of short-term surface velocity (cm/12 days) of the glaciers. The surficial displacements of Shisper and Muchowar glaciers are represented on pre-flood () and post-flood () basis. The maximum surface velocity is observed around the glacial flanges bifurcating from the main trunk. shows the locations marked along the glacial trunks to capture the fluctuations in the glacial mobility as moving away from the proglacial glacial lake. From it can be clearly seen that the glacial mobility near the Shisper lake (Point-1) is around 5 cm (4 April to 16 April 2022) that increases abruptly to 13 cm in the next 12 days (16 April to 28 April 200) later restabilized to 7 cm between 28 April and 10 May 2022. Even though surface velocity of a glacier is a complex phenomenon which depends on several parameters such as topographic slope, glacial thickness and season (Mondal and Bharti, 2022b), a sudden incremental trend in the surface velocity of glacial snouts can be indicative of short-term changes in the glacial dynamics. Also, the abrupt hike in surface displacement is observed at point-6, 9 and 10 at Shisper glacier and Point-17 and 18 at Muchowar glacier () where a maximum increase in LST (>120C) is captured.
Figure 13. Map showing (A) the glacial boundary of Shisper and Muchowar glacier and locations considered along the main trunk and surface velocity between (B) 4 April and 16 April 2022, (C) 16 April and 28 April 2022 and (D) 28 April and 10 May 2022.

Figure 14. Indicating the velocity trend away from the snout for (A) Shisper glacial trunk and (B) Muchowar glacial trunk.

Hazards triggered at high-altitude glaciated environments are complex in terms of their controlling parameters and governing dynamics. Where rapid melting of a glacial body can result in substantial volumetric load of melt-water to a connected ice-dammed glacial lake, it is critically important to study the causative factors behind enhanced glacial melting in a small duration. The present study investigates the changes in various properties (glacial mobility, LST and debris cover) in synchronization with dust or carbon shoot due to anthropogenic activities (NDDI and HYSPILT back trajectories). Where glacial hazards are sudden and ground-based monitoring is challenging due to rugged topography and harsh weather conditions, utilizing satellite constellations and different domains of space-borne remote sensing (optical, thermal and microwave) can be effective.
4.2. After-effects
The after-effects of a flood event comprise several detectable alterations through satellite remote sensing such as changes in the water quality, flood plains, debris and sediment deposition, etc. Since, the proglacial lake lies close to the human settlements of Hassanabad region, it is essential to conduct a post-event analysis. The pre-and post-flood assessment of water quality has been carried out using optical and SAR datasets and changes at the five locations are shown in . The first three locations have been considered before the Karakoram highway bridge that collapsed due to the outburst flood. One location has been considered just after the stream crosses the bridge. The last location has been marked at the meltwater stream point of convergence with the Hunza river stream.
Figure 15. (A) Map showing locations considered for the assessment of after-effects of GLOF event and changes in (B) NDTI, (C) NDWI and (D) Chal-a concentration along the meltwater stream.

The collapse of the Karakoram highway bridge due to a glacial lake outburst indicates a highly turbulent discharge that washed away the bridge along. represents the NDTI in various locations during pre-and post-flood. It can be clearly seen that the NDTI values along the stream lie below 0.03 that get disturbed due to outburst flood. Along the stream, NDTI is comparatively higher before the flood in all the locations. A peak in the NDTI is observed at location 3 prior to the flood, just before the water crosses the Karakoram bridge. However, the value gets stabilized at location 5, where the stream meets the Hunza river. Also, the increment in NDTI throughout the stream on 2 May 2022 can be attributed to decreased water quality. A possible reason behind such anomalous rise in NDTI could be an increase in glacial melt contribution into the stream, leading to increased water activity in terms of discharge and turbulence, thus a hike in sediment load. shows the changes in NDWI values along the stream. The negative values of NDWI until point 4, just after the Karakoram bridge, represent muddy water that shifts towards positive at point 5 once the stream meets Hunza river. At locations 3 and 4, NDWI values further fall, symbolizing decreased water quality that corroborates well with NDTI's observations. At point 5 before the flood occurred, NDWI is positive, which indicates the presence of clean water. This later fall to negative after the flood indicating lowered water quality. The stream is checked for contamination using derived Chlo-a concentration as shown in . The values are less than 20 µg/L and appear to be almost uniform throughout the stream. Such lesser values of Chlo-a concentration indicate no contamination of water along the stream with a sufficiently higher flow rate. Increased value of Chlo-a concentration points towards contamination in water quality with a lowered flow rate which is not observed in this case.
The backscatter coefficient obtained from Sentinel-1 SAR datasets can capture alteration in the concentration of sediment load in water and various types of surfaces based on their roughness. The backscatter coefficient along the stream (Location-1 to 5) is shown in . The values exceed −5dB which indicates highly rough topography. On 16 April 2022, the backscatter coefficient is negative throughout the stream and shifts towards positive on 28 April at Location-2. Such a shift in the backscattering indicates the flow of increased discharge into the stream that attributes to more waves in the stream, similar results are reported by Feng et al. (Citation2022). High backscatter coefficient at Location-1 and 2 on 10 May (post-flood) shows a further discharge increase. As location-1 and 2 lie close to the glacial lake, a continuous positive shift in the backscattering can be attributed to the rapid melting rate of the glacial bodies contributing a significant amount of meltwater discharge into the stream. The backscattering tends to get stabilized (reduced to <0 dB) after Location-2 as the stream approaches the Hunza river. Locations- 3, 4 and 5 do not show a contrasting shift in backscattering.
Figure 16. Changes in backscatter coefficient (dB) at different locations along the meltwater stream.

A GLOF event delivers land cover changes along the stream it propagates, which can be mapped using the C-band SAR system of Sentinel-1 which could be related to the Shisper glacial lake outburst flood that could be associated with the changes in the form of bank erosions and the collapse of Hassanabad highway bridge. shows the pre-flood and co-flood coherence for ascending SLC data pairs. The warm color indicates a high correlation between SLC data pairs before and after the flood. The coherence values range between 0.996 and 0.03 for pre-flood () and 0.986 and 0.01 for co-flood (), respectively as the study region has been masked from the used SLC scene. However, it is important to understand that the decorrelation captured pre- and co-flood comprises of three kinds of effects that need to be considered (Equationequations 17(17)
(17) and Equation18
(18)
(18) ) (Zebker and Villasenor, 1992).
(17)
(17)
(18)
(18)
Figure 17. Map showing coherence derived between (A) 16 April and 28 April 2022 (B) 28 April and 10 May 2022 (C) Coherence difference between (B) and (A) and (D) Reduced coherence around Karakoram highway bridge (zoomed from (C)) and down the stream.

Here,
and
represents registered decorrelation due to sensor noise, geometric coherence attributed to perpendicular baseline component and correlation response due to changes in surface cover and/or vegetation respectively. Since, the SLC datasets have been obtained from the same satellite sensor (Sentinel-1), the effect of
is good to be neglected. Moreover, considering Sentinel-1 observation over a flat surface and scatterers distributed uniformly within a pixel, the effect of
obtained from the perpendicular baseline of the used SAR system remains almost identical and gets eliminated while computing the difference in coherence between two SLC pairs. Therefore, changes in
can be attributed to only changes in the surface cover and/or vegetation. shows a significant reduction in the co-flood coherence along the stream as the discharge crosses the location of the Karakoram highway bridge and continues downstream. This indicates geometric deformation/collapse of man-made structures such as the Karakoram highway bridge and variation of land surfaces due to severe bank erosions.
5. Conclusion
The Karakoram Himalaya comprises numerous moraine- and ice-dammed glacial lakes with a high perspective risk of GLOF. This study extensively investigated the recently triggered Shisper glacial lake outburst flood of 7 May 2022 through different domains of satellite remote sensing (optical, thermal and microwave) utilizing the satellite constellations. The study has been conducted in two stages, where the first stage deals with finding the plausible factors behind the GLOF, and the next stage captures the after-effects. The salient points of the investigation are as follows:
The accumulation area and the AAR of the Shishper glacier reflect a decreasing trend between 2017 and 2021. NDSI shows a significant reduction in the snow coverage of Hassanabad glaciated terrain between 17 April and 10 May 2022 followed by increased debris cover around the elevated regions of the glacial trunk between 30 April and 10 May 2022. A sudden increase in LST within the glacial body (>12 °C) within 15 days at the time of GLOF, as well as a rise in the skin temperature (4 °C) within the Shisper glacier region in the month of May 2022 as compared to May 2021, could be responsible for the breach of glacial lake.
The investigation of short-term surface velocity (cm/12 days) of glacial trunks shows hike in the surface mobility just before the outburst flood between 16 April and 28 April 2022. Moreover, an abrupt rise in surficial displacement is observed near the locations of increased debris cover and LST for both the glacier.
In analyzing the after-effects of the outburst flood, the water quality of the meltwater stream is checked using band ratio algorithms, namely NDTI, NDWI, and NDCI, respectively. Increments in the NDTI values are observed just before the flood in all the locations along the stream. This explains increased glacial melt contribution into the stream before flood, thus increased turbulence and sediment load. The results corroborate well with the reduction in NDWI values, particularly before and after the Karakoram Highway Bridge, indicating lowered water quality. The stream water seems to be devoid of Chlo-a contamination (<20 µg/L) with a sufficiently higher flow rate along the stream before and after the flood event.
The backscatter coefficient captured through the C-band Sentinel-1 SAR system shows a shift from negative on 16 April to positive on 28 April and 10 May 2022 along the stream close to the glacial lake. A possible reason behind such anomalous change in backscattering could be the enhanced melting rate of glacial bodies, thus increasing discharge into the meltwater stream resulting in more waves. However, this further gets stabilized (<0 dB) as the stream water moves downstream towards the Hunza river.
Reduction in the co-flood coherence is captured as the meltwater crosses the Karakoram highway bridge location. This can be attributed to the impact of the outburst flood in downstream, resulting in a bridge collapse and severe bank erosion episodes.
Based on the detailed analysis, it can be concluded that a noticeable reduction in the snow cover within a short span of time (within weeks) has led to increased exposure of debris within the glaciers that might have resulted in increased absorption of solar radiation by the glacial bodies. High absorption of solar radiation could be related to the increase in surface temperature of glaciers, thus leading to enhanced melting. As the meltwater of the Shisper glacier feeds a proglacial lake located at its snout, a sudden increase in the meltwater contribution might have led to increased pressure over the ice-dammed structure confining the lake water and thus breaching.
Acknowledgment
We are grateful to European Space Agency, Alaska Satellite Facility, USGS, and Randolph Glacier Inventory for their tremendous efforts to make the data available for the current study. We are grateful to the anonymous Reviewers and to Dr. Sridhara Nayak and Dr. Vincenzo Lapenna, two Associated Editors, for their valuable comments and suggestions to improve earlier versions of the manuscript. The authors thank the NASA Giovanni team for the AIRS skin temperature product.
Data availability statement
The data used for the study has been collected from different agencies that can be assessed using the following links.
SENTINEL-1:https://search.asf.alaska.edu/#/
SENTINEL-2 MSI:https://scihub.copernicus.eu/dhus/#/home
LANDSAT-8/9 OLI:https://earthexplorer.usgs.gov/
ALOS PALSAR:https://search.asf.alaska.edu/#/?dataset=ALOS
MODIS DATA (MOD09GA):https://lpdaac.usgs.gov/products/mod09gav006/
RANDOLPH GLACIER INVENTORY:https://www.glims.org/RGI/rgi60_dl.html
Disclosure statement
The authors reported no potential conflict of interest.
References
- Amitrano D, Guida R, Di Martino G, Iodice A. 2019. Glacier monitoring using frequency domain offset tracking applied to sentinel-1 images: a product performance comparison. Remote Sensing. 11(11):1322. doi: 10.3390/rs11111322.
- Ashraf A, Iqbal MB, Mustafa N, Naz R, Ahmad B. 2021. Prevalent risk of glacial lake outburst flood hazard in the Hindu Kush–Karakoram–Himalaya region of Pakistan. Environ Earth Sci. 80(12):1–12. doi: 10.1007/s12665-021-09740-1.
- Ashraf A, Roohi R, Naz R, Mustafa N. 2014. Monitoring cryosphere and associated flood hazards in high mountain ranges of Pakistan using remote sensing technique. Nat Hazards. 73(2):933–949. doi: 10.1007/s11069-014-1126-3.
- Avdan U, Jovanovska G. 2016. Algorithm for automated mapping of land surface temperature using LANDSAT 8 satellite data. J Sens. 2016:1–8. doi: 10.1155/2016/1480307.
- Bajracharya SR, Mool PK, Shrestha BR. 2007. Impact of climate change on Himalayan glaciers and glacial lakes: case studies on GLOF and associated hazards in Nepal and Bhutan. International Centre for Integrated Mountain Development (ICIMOD).
- Bolch T, Kulkarni A, Kääb A, Huggel C, Paul F, Cogley JG, Frey H, Kargel JS, Fujita K, Scheel M, et al. 2012. The state and fate of Himalayan glaciers. Science. 336(6079):310–314., doi: 10.1126/science.1215828.
- Campbell JG, Pradesh H. 2005. Inventory of glaciers, glacial lakes and the identification of potential glacial lake outburst floods (GLOFs) affected by global warming in the mountains of India, Pakistan and China/Tibet Autonomous Region. International Centre for Integrated Mountain Development, GP O. Box, Asia-Pacific Network for Global Change Research (APN). p. 3226.
- Cantalloube HM, Nouvel JF, Azarian S, Cheraly A, Roques S, Oriot H. 2016. June. Rail-borne SAR interferometry for Disaster Prevention. In Proceedings of EUSAR 2016: 11th European Conference on Synthetic Aperture Radar (pp. 1–5). VDE.
- Chauhan PK, Kumar A, Pratap V, Chaubey SK, Singh AK. 2023. Dust storm characteristics over Indo-Gangetic basin through satellite remote sensing. In Atmospheric Remote Sensing (p. 373–392). Elsevier. doi: 10.1016/B978-0-323-99262-6.00007-9.
- Che Y, Zhang M, Li Z, Wei Y, Nan Z, Li H, Wang S, Su B. 2019. Energy balance model of mass balance and its sensitivity to meteorological variability on Urumqi River Glacier No. 1 in the Chinese Tien Shan. Sci Rep. 9(1):13958. doi: 10.1038/s41598-019-50398-4.
- Closson D, Milisavljevic N. 2017. InSAR Coherence and Intensity Changes Detection. Mine Action-The Research Experience of the Royal Military Academy of Belgium. Croatia: InTech.
- Cogley JG, Kargel JS, Kaser G, van der Veen CJ. 2010. Tracking the source of glacier misinformation. Science. 327(5965):522–522. doi: 10.1126/science.327.5965.522-a.
- Feng Y, Chen J, Huang Z, Wan H, Xia R, Wu B, Sun L, Xing M. 2022. A lightweight position-enhanced anchor-free algorithm for SAR ship detection. Remote Sensing. 14(8):1908. doi: 10.3390/rs14081908.
- Filipponi F. 2019. Sentinel-1 GRD preprocessing workflow. In Multidisciplinary Digital Publishing Institute Proceedings. 18(1):11. (
- Gao BC. 1996. NDWI—A normalized difference water index for remote sensing of vegetation liquid water from space. Remote Sens Environ. 58(3):257–266. doi: 10.1016/S0034-4257(96)00067-3.
- Gautam R, Hsu NC, Lau WKM, Yasunari TJ. 2013. Satellite observations of desert dust‐induced Himalayan snow darkening. Geophys Res Lett. 40(5):988–993. doi: 10.1002/grl.50226.
- Hao XH, Wang J, Li HY. 2008. Evaluation of the NDSI threshold value in mapping snow cover of MODIS—A case study of snow in the Middle Qilian Mountains. J. Glaciol. Geocryol. 30(1):132–138.
- Hewitt K, Liu J. 2010. Ice-dammed lakes and outburst floods, Karakoram Himalaya: historical perspectives on emerging threats. Physical Geography. 31(6):528–551. doi: 10.2747/0272-3646.31.6.528.
- Hewitt K. 2005. The Karakoram anomaly? Glacier expansion and the ‘elevation effect,’Karakoram Himalaya. Mt Res Dev. 25(4):332–340. doi: 10.1659/0276-4741(2005)025[0332:TKAGEA.2.0.CO;2]
- Hewitt K. 2009. Rock avalanches that travel onto glaciers and related developments, Karakoram Himalaya, Inner Asia. Geomorphology. 103(1):66–79. doi: 10.1016/j.geomorph.2007.10.017.
- Immerzeel WW, Van Beek LP, Bierkens MF. 2010. Climate change will affect the Asian water towers. Science. 328(5984):1382–1385. doi: 10.1126/science.1183188.
- Ives JD, Shrestha RB, Mool PK. 2010. Formation of glacial lakes in the Hindu Kush-Himalayas and GLOF risk assessment (p. 10–11. Kathmandu: ICIMOD.
- Kääb A, Berthier E, Nuth C, Gardelle J, Arnaud Y. 2012. Contrasting patterns of early twenty-first-century glacier mass change in the Himalayas. Nature. 488(7412):495–498. doi: 10.1038/nature11324.
- Kääb A, Treichler D, Nuth C, Berthier E. 2015. Brief Communication: contending estimates of 2003–2008 glacier mass balance over the Pamir–Karakoram–Himalaya. The Cryosphere. 9(2):557–564. doi: 10.5194/tc-9-557-2015.
- Khan G, Ali S, Xiangke X, Qureshi JA, Ali M, Karim I. 2021. Expansion of Shishper Glacier lake and recent glacier lake outburst flood (GLOF), Gilgit-Baltistan, Pakistan. Environ Sci Pollut Res Int. 28(16):20290–20298. doi: 10.1007/s11356-020-11929-z.
- Kulkarni AV. 1992. Mass balance of Himalayan glaciers using AAR and ELA methods. J Glaciol. 38(128):101–104. doi: 10.3189/S0022143000009631.
- Kumar R, Bahuguna IM, Ali SN, Singh R. 2020. Lake inventory and evolution of glacial lakes in the Nubra-Shyok basin of Karakoram Range. Earth Syst Environ. 4(1):57–70. doi: 10.1007/s41748-019-00129-6.
- Lichtenegger J, Gurung DR, Mool PK, Bigot J. 2008. June. Near real-time monitoring of glacial lake Imja (Khumbu-Everest region). In Proceeding of the ESA Living Planet Symposium, Bergen, Norway (Vol. 28).
- Mao W, Wu L, Singh RP, Qi Y, Xie B, Liu Y, Ding Y, Zhou Z, Li J. 2022. Progressive destabilization and triggering mechanism analysis using multiple data for Chamoli rockslide of 7 February 2021. Geomatics Nat Hazards Risk. 13(1):35–53. doi: 10.1080/19475705.2021.2013960.
- McFeeters SK. 1996. The use of the Normalized Difference Water Index (NDWI) in the delineation of open water features. Int J Remote Sens. 17(7):1425–1432. doi: 10.1080/01431169608948714.
- Meena SR, Bhuyan K, Chauhan A, Singh RP. 2021a. Changes in the flood plains and water quality along the Himalayan rivers after the Chamoli disaster of 7 February 2021. Int J Remote Sens. 42(18):6984–7001. doi: 10.1080/01431161.2021.1944696.
- Meena SR, Chauhan A, Bhuyan K, Singh RP. 2021b. Chamoli disaster: pronounced changes in water quality and flood plains using Sentinel data. Environ Earth Sci. 80(17):601. doi: 10.1007/s12665-021-09904-z.
- Meena S, Bhuyan K, Chauhan AK, Singh RP. 2021c. Snow covered with dust after Chamoli rockslide: inference based on high-resolution satellite data. Remote Sensing Letters. 12Issue(7)Page:704–714. volume doi: 10.1080/2150704X.2021.1931532.
- Mishra S, Mishra DR. 2012. Normalized difference chlorophyll index: a novel model for remote estimation of chlorophyll-a concentration in turbid productive waters. Remote Sens Environ. 117:394–406. doi: 10.1016/j.rse.2011.10.016.
- Mondal SK, Bharti R. 2021b. July. Smoulder Detection Using Split-Window Algorithm: a Case Study from Baghjan Oilfield, Assam, India. In 2021 IEEE International Geoscience and Remote Sensing Symposium IGARSS (p. 8590–8593). IEEE. doi: 10.1109/IGARSS47720.2021.9554837
- Mondal SK, Bharti R, Kumar S. 2023. Spatio-temporal Variations in Oxygen and Deuterium Isotope of Different Water Sources in Sikkim Himalayas: an Understanding Towards Regional Scale Basin Hydrology. J Hydrol. 621:129613. doi: 10.1016/j.jhydrol.2023.129613.
- Mondal SK, Bharti R. 2022a. Glacial burst triggered by triangular wedge collapse: a study from Trisul Mountain near Ronti glacier valley. Geomatics Nat Hazards Risk. 13(1):830–853. doi: 10.1080/19475705.2022.2042402.
- Mondal SK, Bharti R. 2022a. July. Seismic Impact Around Himalayan Snow-Melt Fed Lake Using InSAR: a Case Study for 20 March 2020 MW5. 7 Tibet Earthquake. In IGARSS 2022-2022 IEEE International Geoscience and Remote Sensing Symposium (p. 2522–2525. IEEE.
- Mool PK, Bajracharya SR, Shrestha BR. 2005a. Inventory of Glaciers and Glacial Lakes and the Identification of Potential Glacial Lake Outburst Floods (GLOFs) Affected by Global Warming in the Mountains of India, Pakistan and China/Tibet Autonomous Region (APN 2004-03-CMY–Campbell). In APN Newsletter. 11(4):6–7.
- Muhammad S, Li J, Steiner JF, Shrestha F, Shah GM, Berthier E, Guo L, Wu LX, Tian L. 2021. A holistic view of Shisper Glacier surge and outburst floods: from physical processes to downstream impacts. Geomatics Nat Hazards Risk. 12(1):2755–2775. doi: 10.1080/19475705.2021.1975833.
- Nie Y, Liu Q, Liu S. 2013. Glacial lake expansion in the Central Himalayas by Landsat images, 1990–2010. PLoS One. 8(12):e83973. doi: 10.1371/journal.pone.0083973.
- Nolin AW, Liang S. 2000. Progress in bidirectional reflectance modeling and applications for surface particulate media: snow and soils. Remote Sensing Reviews. 18(2-4):307–342. doi: 10.1080/02757250009532394.
- Nüsser M, Dame J, Parveen S, Kraus B, Baghel R, Schmidt S. 2019. Cryosphere-fed irrigation networks in the northwestern Himalaya: precarious livelihoods and adaptation strategies under the impact of climate change. Mt Res Dev. 39(2):R1–R11. doi: 10.1659/MRD-JOURNAL-D-18-00072.1.
- Nüsser M, Schmidt S. 2021. Glacier changes on the Nanga Parbat 1856–2020: A multi-source retrospective analysis. Sci Total Environ. 785:147321. doi: 10.1016/j.scitotenv.2021.147321.
- Pandey VK, Kumar R, Singh R, Kumar R, Rai SC, Singh RP, Tripathi AK, Soni VK, Ali SN, Tamang D, et al. 2022. Catastrophic ice-debris flow in the Rishiganga River, Chamoli, Uttarakhand (India). Geomatics Nat Hazards Risk. 13(1):289–309. doi: 10.1080/19475705.2021.2023661.
- Parveen S, Winiger M, Schmidt S, Nüsser M. 2015. Irrigation in Upper Hunza: evolution of socio-hydrological interactions in the Karakoram, northern Pakistan. Erdkunde. 69(1):69–85. doi: 10.3112/erdkunde.2015.01.05.
- Patel P, Mondal SK, Bharti R. 2019. July. Snow Area Mapping using Feature-oriented Principal Component Analysis. In IGARSS 2019-2019 IEEE International Geoscience and Remote Sensing Symposium (p. 4088–4090. IEEE.
- Perrou T, Garioud A, Parcharidis I. 2018. Use of Sentinel-1 imagery for flood management in a reservoir-regulated river basin. Front Earth Sci. 12(3):506–520. doi: 10.1007/s11707-018-0711-2.
- Pfeffer WT, Arendt AA, Bliss A, Bolch T, Cogley JG, Gardner AS, Hagen J-O, Hock R, Kaser G, Kienholz C, et al. 2014. The Randolph Glacier Inventory: a globally complete inventory of glaciers. J Glaciol. 60(221):537–552., doi: 10.3189/2014JoG13J176.
- Prasad AK, Singh RP. 2007a. Changes in aerosol parameters during major dust storm events (2001–2005) over the Indo‐Gangetic Plains using AERONET and MODIS data. J Geophys Res. 112(D9):D09208. doi: 10.1029/2006JD007778.
- Prasad AK, Singh RP. 2007b. Changes in Himalayan snow and glacier cover between 1972 and 2000. EoS Transactions. 88(33):326–326. doi: 10.1029/2007EO330002.
- Pritchard HD. 2017. Asia’s glaciers are a regionally important buffer against drought. Nature. 545(7653):169–174. doi: 10.1038/nature22062.
- Qin D, Ding Y, Xiao C, Kang S, Ren J, Yang J, Zhang S. 2018. Cryospheric science: research framework and disciplinary system. National Science Review. 5(2):255–268. doi: 10.1093/nsr/nwx108.
- Qu JJ, Hao X, Kafatos M, Wang L. 2006. Asian dust storm monitoring combining Terra and Aqua MODIS SRB measurements. IEEE Geosci Remote Sensing Lett. 3(4):484–486. doi: 10.1109/LGRS.2006.877752.
- Reynolds JM. 1992. The identification and mitigation of glacier-related hazards: examples from the Cordillera Blanca, Peru. In Geohazards (p. 143–157. Springer, Dordrecht.
- Riaz S, Ali A, Baig MN. 2014. Increasing risk of glacial lake outburst floods as a consequence of climate change in the Himalayan region. Jàmbá: journal of Disaster Risk Studies. 6(1):1–7. doi: 10.4102/jamba.v6i1.110.
- Richardson SD, Reynolds JM. 2000. An overview of glacial hazards in the Himalayas. Quat Int. 65-66:31–47. doi: 10.1016/S1040-6182(99)00035-X.
- Ritchie JC, Zimba PV, Everitt JH. 2003. Remote sensing techniques to assess water quality. Photogramm Eng Remote Sensing. 69(6):695–704. doi: 10.14358/PERS.69.6.695.
- Salomonson VV, Appel I. 2004. Estimating fractional snow cover from MODIS using the normalized difference snow index. Remote Sens Environ. 89(3):351–360. doi: 10.1016/j.rse.2003.10.016.
- Schmidt S, Nüsser M, Baghel R, Dame J. 2020. Cryosphere hazards in Ladakh: the 2014 Gya glacial lake outburst flood and its implications for risk assessment. Nat Hazards. 104(3):2071–2095. doi: 10.1007/s11069-020-04262-8.
- Shugar DH, Jacquemart M, Shean D, Bhushan S, Upadhyay K, Sattar A, Schwanghart W, McBride S, de Vries MVW, Mergili M, et al. 2021. A massive rock and ice avalanche caused the 2021 disaster at Chamoli, Indian Himalaya. Science. 373(6552):300–306., doi: 10.1126/science.abh4455.
- Singh C, Chauhan N, Upadhyay SK, Singh R, Rani A. 2021. The Himalayan natural resources: challenges and conservation for sustainable development. Journal of Pharmacognosy and Phytochemistry. 10(1):1643–1648.
- Solovey T. 2019. An analysis of flooding coverage using remote sensing within the context of risk assessment. Geologos. 25(3):241–248. doi: 10.2478/logos-2019-0026.
- Stathopoulou M, Cartalis C, Petrakis M. 2007. Integrating Corine Land Cover data and Landsat TM for surface emissivity definition: application to the urban area of Athens, Greece. Int J Remote Sens. 28(15):3291–3304. doi: 10.1080/01431160600993421.
- USEPA. 2008. “National Coastal Condition Report III,” p. 329. Office of Research and Development/Office of Water. United StatesEnvironmental Protection Agency. EPA/842-R-08-002, Washington, DC 20460.
- Usgs (u S. 2013. Geological Survey).2013. Earthexplorer.Available: http://earthexplorer.usgs.gov/. [accessed 27 May ].
- Wang W, Xiang Y, Gao Y, Lu A, Yao T. 2015. Rapid expansion of glacial lakes caused by climate and glacier retreat in the Central Himalayas. Hydrol Process. 29(6):859–874. doi: 10.1002/hyp.10199.
- Wangchuk S, Bolch T, Zawadzki J. 2019. Towards automated mapping and monitoring of potentially dangerous glacial lakes in Bhutan Himalaya using Sentinel-1 Synthetic Aperture Radar data. Int J Remote Sens. 40(12):4642–4667. doi: 10.1080/01431161.2019.1569789.
- Watanabe T, Ives JD, Hammond JE. 1994. Rapid growth of a glacial lake in Khumbu Himal, Himalaya: prospects for a catastrophic flood. Mt Res Dev. 14(4):329–340. doi: 10.2307/3673729.
- Wester P, Mishra A, Mukherji A, Shrestha AB. 2019. The Hindu Kush Himalaya assessment: mountains, climate change, sustainability and people (p. 627). Springer Nature. doi: 10.1007/978-3-319-92288-1
- Winiger M, Gumpert M, Yamout H. 2005. Karakorum–Hindukush–western Himalaya: assessing high‐altitude water resources. Hydrological Processes: An International Journal. 19(12):2329–2338. doi: 10.1002/hyp.5887.
- Winther JG. 1993. Short-and Long-Term Variability of Snow Albedo: paper presented at the 9th Northern Res. Basin Symposium/Workshop (Whitehorse/Dawson/Inuvik, Canada-August 1992). Hydrology Research. 24(2-3):199–212. doi: 10.2166/nh.1993.0022.
- Zhang M, Chen F, Tian B, Liang D, Yang A. 2020. Characterization of Kyagar Glacier and lake outburst floods in 2018 based on time-series Sentinel-1A data. Water. 12(1):184. doi: 10.3390/w12010184.
- Zhao Y, Zhao J, Yue X, Wang Y. 2020. Comparison of remote sensing extraction methods for glacier firn line-considering Urumqi Glacier No. 1 as the experimental area. In E3S Web of Conferences (Vol. 218, p. 04024). EDP Sciences.