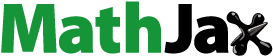
Abstract
One of the most important aspects of the ‘sub-tropical’ monsoon-influenced environment is the issue of ‘soil erosion’ and its related ‘land degradation’. On the other hand, the climate in this area has become quite extreme. According to this viewpoint, it is important to research a future ‘soil erosion’ scenario in front of the probable effects of climate change and land use change. For the objective of assessing the extent of soil erosion in this area, this study took into account both the USLE and the RUSLE. Compared to the USLE that has been validated, RUSLE has a comparatively greater quantitative efficiency. In RUSLE, the ‘very high’ (>20) and ‘high’ (15–20) ‘soil erosion’ zones tend to be associated with the ‘north-western, western, south-western, and southern’ regions of the river basin. The ‘soil erosion’ that will occur in the future has been estimated by taking into account the projected rainfall, land use and land cover (LULC). ‘Soil erosion’ has increased from the previous time to the projected time. Predicted R factor values for SSP 585 range from 399.92 to 493.72. In addition, a growing erosion tendency associated with increased shared socio-economic pathways (SSPs) has been found.
1. Introduction
Changes in intense precipitation has a major impact on soil erosion under climate change (Eekhout et al. Citation2018). A warmer atmosphere’s increased ability to store moisture is expected to lead to an increase in extreme precipitation and a more active hydrological cycle (Allan et al. Citation2020). Globally, long-term measurements already indicate an increase in severe precipitation, and future forecasts from climate models point to a continuation of this trend (Fischer et al. Citation2013). Extreme rainfall has an influence on soil erosion both through the impact of raindrops on the soil and through runoff that separates soil particles (Eekhout and De Vente Citation2020). The likelihood of climate change increasing infiltration and excess surface runoff increases the likelihood of rill and (ephemeral) gully erosion, which are said to account for the majority of the overall sediment production (Anderson et al. Citation2021).
Thirty-eight percent of the world’s land is used for agriculture, and contemporary society depends on this cultivated environment (Hazell et al. Citation2010). The capacity of the soil to sustain agricultural and animal production, which together account for more than 95% of the ‘global food supply’, is of primary importance to humans (Keating et al. Citation2014). The process of soil erosion is a natural process, though it is directly impacted by various anthropogenic activities. In this perspective, agricultural production is directly negatively linked with soil erosion and its associated nutrient losses. The agricultural outputs that underlie them are important contributors to biologically active ‘greenhouse emissions’ as well as key sources of soil and ‘environmental degradation (Aryal et al. Citation2022)’. According to the most recent assessment made by the ‘United Nations (UN)’ on the ‘state of global soil resources’, the quality of the soil on the majority of the planet is either merely fair, poor, or extremely bad. This was found in the report on the ‘status of global soil resources’. It emphasises that soil erosion is a major issue for agriculture and the environment worldwide. Plowing, poor farming practices, deforestation, and animal grazing are the most important cause of human-induced soil erosion (Borrelli et al. Citation2020). As a consequence, the ecosystem suffers from cumulative effects such as nutrient loss, reduced carbon storage, diminished diversity, and soil and ecological instability. At the worldwide scale, modelling attempts to forecast the influence of ‘climate’ and ‘land use change’ on soils are growing, but they are restricted (Dignac et al. Citation2017). The goal of this research is to progress our capacity to anticipate erosion in light of these factors.
Rural regions have seen major changes as a result of ‘agricultural LU’, mainly extensive cropland usage, during the last several decades (Pongratz et al. Citation2008). The potential for soils to be eroded by wind and water has significantly risen due to anthropogenic factors such as shifts in LU and selected crop sequences, the use of large agricultural utensils, and considerable land consolidation (Lin et al. Citation2023). Increasing air temperatures have an impact on soil evaporation and transpiration, which changes the water balance in the soil (Eekhout and de Vente Citation2022). Additionally, when growth circumstances vary due to climate change, soil erosion is mostly negatively impacted (Li and Fang Citation2016). Relevant parameters include root development, soil organic carbon content, and soil cover by canopy of plants and plant residues are the important parameters that directly influence overall soil loss (Etehadi Abari et al. Citation2017). Although land use might remain the same, higher rainfall erosivity alters the hydrological situation, leading to surface runoff and soil loss (Ouyang et al. Citation2018).
Under climate change, strong precipitation have the significant immediate effect on soil erosion (O'Neal et al. Citation2005). Because a warmer atmosphere is better able to store moisture, it is projected that extreme precipitation would rise and the hydrological cycle will become more dynamic. Global severe precipitation already seems to be trending upward over the long run, however, predictions made by climate models point to a more rise in the next decades (Walsh et al. Citation2016). Extreme rainfall influences soil erosion through the contact of raindrops on the soil (splash erosion)and runoff, separating soil (soil detachment) particles. Increased infiltration and excessive surface runoff, both of which are virtually surely brought on by climate change, are thought to encourage rill and (ephemeral) gully formation and erosion, which are said to contribute the most to overall sediment production (Li and Fang Citation2016).
It is generally known that changes in land use (LU) is one of the important diverse factors that have significant effects on soil erosion as well as how they interact with climate change (Lal Citation2004). However, predicting how LU will change under future climatic conditions is not a simple undertaking because to the many forces that work at local to global scales. It is expected, given these complications, that only a small number of studies take land use change, plant growth, and the effect of climate change (CC) on soil erosion (SE) into account (Smith et al. Citation2016). Promotion of soil conservation initiatives, which could involve altering land use, such as reforestation programme, and a variety of on-site and its associated off-site methods, is common as a response to the anticipated rise in SE caused by CC (Smith et al. Citation2016). In addition to reducing SE, soil conservation practices may also offer other related ecosystem services, such like the storage of carbon and nitrogen, which helps to mitigate CC and preserve biodiversity.
According to earlier research, the techniques used in CC effect assessments may considerably impact the expected change in soil erosion (Doulabian et al. Citation2021). Due to the uncertainty in the climate projections, it has been shown that climate models have a considerable impact on predictions for soil erosion. Positive as well as negative increases in soil erosion are frequently found using the climate model used (Segura et al. Citation2014). In general, multiple studies reveal notable heterogeneity within the ensembles of climate models. In addition, it is shown that various predictions of future soil erosion are provided by emission scenarios.
In order to employ climate model data in impact evaluations of soil erosion, downscaling and correction for bias are employed. Data from coarse-scale climate models are converted to fine-scale model domains via the process of downscaling, which may include downscaling in time or space (Giorgi Citation2019). Dynamic downscaling, which is connected to regional climate models, and quantitative downscaling are two types of downscaling. By using bias-correction techniques, biases between historic model output and observed are often produced by climate models (Wang and Tian Citation2022). Despite the fact that many researchers use downscaling and associated bias correction approaches after processing climate model data, research has demonstrated that these techniques have an influence on the projections for SE. At the very least, SE models additionally add to the variability in future estimates, for example, because of the time-step employed in conceptualizing soil erosion models and rainfall triggering (Alewell et al. Citation2019). SE is expected to become worse over the next several years due to the projected increase in heavy rainfall and the probable negative effects of changing LU. The few current global modeling studies forecast a rise in SE of between 9 to 56%, but they suffer from several conceptual oversights (Borrelli et al. Citation2017).
The ‘general circulation models (GCMs)’ have been extensively employed for research on the potential impacts of CC worldwide (Rajbanshi and Bhattacharya Citation2022). However, the lower resolution of the GCMs often restricts their usefulness to conduct evaluations of the impacts of CC and adaptations for small areas to catchment sizes. As a result, numerous studies have used ‘regional climate models (RCMs)’ to provide future climate estimates that are very accurate. Because of the sub-grid scale phenomena accessed via the various parametrization techniques, the RCMs are believed to be a more accurate portrayal of regional climate forecasts. RCMs perform better, according to Falco et al. (Citation2020), especially in regions with varying terrain and intense or small-scale climatic phenomena. Similar to this, Kalognomou et al. (Citation2013) also discovered a trustworthy simulated rainfall pattern in their research region using the ensemble of 10 RCMs. RCM simulations still retain systemic model mistakes, sometimes known as biases, despite their better performance. The shape of the distribution is also changed by these biases in RCM simulation, which makes it difficult to use these simulations as input data for further impact research. By using an appropriate bias correction strategy, these systematic mistakes or biases may be rectified. Using the gamma distribution-based bias reduction technique, for instance, (Switanek et al. Citation2017) demonstrated that the method is effective in correcting both the mean and the intensity of the heavy rainfall distribution. Using an empirical quantile mapping bias reduction approach, (Casanueva et al. Citation2020) discovered a trustworthy spatial connection among the actual and RCM-predicted seasonal and yearly rainfall. However, not all RCMs may exhibit identical bias correction performance under all climate change scenarios. As a result, in order to serve a particular research aim, it is important to assess their performance. Eekhout and de Vente (Citation2019) contend that reliable assessments of the effects of CC on SE under various emission scenarios depend on the evaluation of bias correction methodology.
Considering all of the prior studies, it is clear that the sediment yield (SE) and SY, which are expected to rise in the future, are directly impacted by the changing rainfall caused by modifying the R factor. Furthermore, the chosen RCMs and the bias correction approach have a large impact on the expected change in SE and SY. To comprehend how Indian precipitation may change in the future, multiple investigations have been carried out, starting with the ‘coupled model inter-comparison project (CMIP3)’ GCMs and continuing with its upgraded version, CMIP5. The main research question of this work is: what is the potential impact of climate and LULC change on future soil erosion? Therefore the primary objective of this study is to assess the potential impact of CC and LU change on soil erosion in Kangsabati river basin, eastern India.
2. Materials and methods
2.1. Study area
The upper portion of the ‘Kangsabati river’ basin in the lower ‘Ganga’ basin, between 85°57'08’ east and 86°48'56" east, and 22°42'54" north and 23°28'29" north, constituted the study area (). The areal coverage is about 5796 km2. The Kasai and Cossye are other names for the Kangsabati River (Bhattacharya and Das Chatterjee Citation2021). The Bhagarathi Hooghly River’s right-hand secondary tributary is often a non-perennial river by nature. Its source is the Ghoramara hill in Jhalda, which is located at 23°32'30"N and 85°56'30"E on the Eastern Chotonagpur plateau. It then runs east across the districts of Puruliya, Bankura, and undivided Midnapore (Mandal et al. Citation2021). Near Ghatal (Bandar), the Kangsabati River merges into the Rupnarayan River. Keleghai is the name for the Rupnarayan and Kangsabati merged flow (Chakraborty and Chakraborty Citation2021).
The river rises in Jhalda on the Chhotanagpur Plateau in the Purulia district and flows through the Bankura district’s Khatra and Ranibandh before entering Paschim Medinipur in the Binpur region. At Sijua, the Bhairabbanki and Taraphini rivers are merged to form the Kangsabati River. At Palaspai, the Rajnagar segment—also known as the Rupnaryan branch—and the Panskura segment—also known as the Khelaghai branch—are separated. Whereas the Panskura portion travels in a southerly direction and joins the Kaliaghai River, the Rajnagar segment goes through the Daspur region and joins the Rupnaryan River. The most significant right-hand tributary, Kumari, meets the main stem river in Ambikanagar, close to the Kangsabati-Kumari or Mukutmonipur dam. This alluvial river has ‘meandering, straight, and braided’ channel patterns in addition to dendritic, rectangular, trellised, and parallel drainage patterns. The whole Khatra and Raipur subbasins are composed of a plateau fringe region and a series of undulating plains that provide a dendritic drainage structure. The existence of a consistent, resistant rock structure is indicated by this pattern. A trellis drainage system formed in the Lalgarh subbasin as a result of the area’s high, undulating plain area and ridge and valley topography. In the Lalgarh subbasin, this structure was discovered to consist of alternating bands of relatively strong and weak rock. Due to the existence of an intense bedrock joint in the thin soil layer, the Kumari-Trapheni Rivers exhibit a rectangular drainage pattern, which is also significant. In contrast, the extensive alluvial flood plain with silt content along the lower course of the basin displays a pinnate drainage structure. This pattern suggests the presence of a stable, durable rock structure. The high, undulating plain and ridge and valley terrain of the Lalgarh subbasin resulted in the formation of a trellis drainage structure. This structure was found to consist of alternating bands of fairly strong and weak rock in the Lalgarh subbasin. The Kumari-Trapheni Rivers have a rectangular drainage pattern, which is also notable as a result of the presence of an intensive bedrock joint in the thin soil layer. On the other hand, the large, silt-rich alluvial flood plain throughout the basin’s lower course has a pinnate drainage system.
2.2. Database
The following dataset was utilized to fulfil the goals of this research study: ‘ALOS PALSAR Digital Elevation Model (DEM)’ by the ‘Japan Aerospace Exploration Agency’ topographic map (‘Survey of India’), Sentinel 2a satellite data, various soil samples, and first-hand field observation. provides detailed information on the large datasets, suppliers, and intended uses.
Table 1. Database and its sources.
2.3. Methodology
In , the exact process for assessing how ‘climate’ and ‘LULC’ change affects ‘soil erosion’ is shown. This work relates to the following goals:
To determine the current rate of ‘soil erosion’ in this region.
To develop a reliable model that can estimate ‘average annual soil erosion’.
Predicting how ‘climate’ and ‘LULC’ change in the future will impact ‘soil erosion’.
2.4. Soil erosion
To estimate ‘average annual soil erosion’, many criteria have been taken into account as ‘USLE’ and ‘RUSLE’ components ().
2.4.1. ‘Rainfall and runoff erosivity factor (R)’
The storm kinetic energy is multiplied by the maximum storm depth to generate the rainfall-runoff erosivity factor in RUSLE, and the results are then added for all storms in a year (Kinnell Citation2017). The input that powers the sheet and rill erosion processes is represented by the R-factor. Changes in the erosivity of the climate are reflected in changes in R values. The weighting of the soil erodibility value, K, and the cover-management component, C, in the R-factor calculation, is done using a seasonal distribution. For climatically homogenous regions, climate data have been created to simplify these computations.
The following equation represents the ‘MFI’:
(1)
(1)
(2)
(2)
Using the following equation, ‘the R factor is then expressed on the basis of MFI’ (Plangoen et al. Citation2013; Tiwari et al. Citation2016):
(3)
(3)
‘Where is an arbitrary, normally dispersed error and a and b are experiential factors’ (Plangoen et al. Citation2013; Tiwari et al. Citation2016).
2.4.2. ‘Soil erodibility factor (K)’
The K factor measures how easily a certain soil will erode under typical USLE plot maintenance conditions, which call for continual fallow land (Alewell et al. Citation2019). Values are greater for soils with a high silt percentage, whereas values are lower for soils with a high sand and clay concentration. The RUSLE process differs significantly from the USLE in that K also fluctuates seasonally. K is not constant, according to experimental data, and it changes with the season, reaching its maximum point in early spring and its lowest point in mid-fall or during frozen soil.
The following equation has been used to calculate the K factor while taking into consideration a number of physical and chemical parameters:
(4)
(4)
‘San is the proportion of sand, Sil is the proportion of silt, Cla is the proportion of clay, and SN1 is the 1-San/100, where K is the soil erodibility (Teng et al. Citation2018)’.
2.4.3. ‘Slope length and steepness factor (LS)’
The calculation of the LS factor includes considerable subjectivity since different users want various slope lengths for circumstances that are otherwise identical. To increase user uniformity, RUSLE has updated recommendations for selecting the slope length parameters. In terms of the L-factor, the slope length is the USLE characteristic that has the least impact on soil loss. A 10% inaccuracy in the slope length measurement causes a 5% error in the estimated soil loss under typical slope conditions. RUSLE employs four distinct slope length relationships. Three depend on the slope steepness as assessed by USLE, the soil’s susceptibility to rill erosion in relation to interrill erosion. The LS factor may be determined using the slope and length provided as follows:
The ‘LS factor’ for this site was calculated using the equation shown below:
(5)
(5)
(6)
(6)
(7)
(7)
(8)
(8)
where ‘L is the slope length factor, β is the slope length, m is the potential of erosion in relation to the amount of gradient in percentage terms, is the slope angle (in degrees) in the GIS environment, and flowacc is the flow accumulation. F is the ratio of rill and inter-rill erosion’.
(9)
(9)
(10)
(10)
where ‘LS is the combination of the slope length and steepness’ and ‘L is the slope length, S is the slope steepness’ (Nearing et al. Citation2017).
2.4.4. ‘Cover and management factor (C)’
Given that it identifies variables that may be changed most quickly to prevent erosion, the vegetative cover component of the RUSLE is perhaps the most significant. The values of C may range from virtually 0 for soil that is extremely well protected to 1.5 for a surface that has been finely tilled and ridged, which generates a lot of runoff and makes the soil especially sensitive to rill erosion (Thomas et al. Citation2018).
A unit plot is a fallow land that has been clean-tilled. The values of C indicate the weighted average of the soil loss ratios, which show how much soil has been lost over time compared to the unit plot in a particular circumstance (M.A. Nearing et al. Citation2017). As soil and cover conditions fluctuate throughout the year, so do soil loss ratios. ‘Soil loss ratios (SLR)’ are weighted in accordance with the distribution of erosivity throughout a year to get C. The C-factor in RUSLE takes into account how differences in soil erosion from losses that happen in bare fallow areas are caused by land cover, crops, and management of crops. Here, for the base period, the vegetation indices have been considered for estimating the C factor for this region. In projecting the C factor for the predicted period, we consider LULC prediction.
2.4.5. ‘Support practice factor (P)’
The erosion management practice element largely illustrates how surface characteristics have an influence on flow routes and flow hydraulics. For instance, runoff runs along channels created by tillage while contouring the slope. When compared to up-and-down hill flow pathways, the gradient and flow velocities may be much lower. The reliability of the P-factor values is the lowest of any of the other factors. The impact of contouring is influenced by a number of interrelated factors. In the few field studies that have addressed contouring, these interactions have made it difficult to record. Due to interactions between the intensity of the storm, antecedent soil water, and tillage, a contouring factor may vary considerably from storm to storm and field to field. Applying RUSLE makes it difficult to spot these minor traits in the field. The P-factor values, therefore, show wide, all-encompassing impacts of techniques like contouring.
The RUSLE P-components are seen as the sum of all the individual factors that were computed according to the methodology utilized in the landscape (Stefanidis et al. Citation2022). RUSLE has looked at a large quantity of data (both model-based and field-based) to assess the impacts of contouring (Tanyaş et al. Citation2015). As a consequence of the findings, factor values for contouring have been created as a function of ridge height, furrow slope, and climate erosivity. Even while RUSLE takes a wider range of strip cropping situations into consideration, new P-factor estimations for the impact of terracing take the slope along the terrace into account (Xiong et al. Citation2019). Not to add that RUSLE P-factors have been created to represent conservation efforts on a range areas. Estimates of surface roughness and runoff reduction are needed for the practices (Tamene et al. Citation2017). Here, different support practice measures have been incorporated,considering different slopes for estimating the P factor of this region.
(11)
(11)
The validation of the models has been done with the help of estimated soil erosion and measured soil erosion. The correlation has been made between measured soil erosion and estimated soil erosion.
2.5. Climate change
The study took into account the latest set of SSP scenarios created by CMIP6 of the World Climate Research Programme (WCRP) (SSP1-2.6, SSP2-4.5, SSP3-7.0, and SSP5-8.5). These additional scenarios were taken into account since they show various socioeconomic trends and paths for atmospheric greenhouse gas concentrations. SSPs are projections of expected worldwide socioeconomic trends through 2100. They are applied to create scenarios for greenhouse gas emissions under various climate policies.
The cumulative distribution function (CDF) of data and raw predictions are matched by quantile method (QM). Matching occurs at the level of specific combined members for ensemble predictions. Let FX and FO stand for the CDFs of the raw predictions and observations, and let and stand for the raw and post-processed projections, accordingly. QM is defined as
(12)
(12)
Applying FO to EquationEquation (1)(1)
(1) 's left and right sides results in
(13)
(13)
A fresh raw forecast number is postprocessed in two phases in accordance with EquationEqs. (12)(12)
(12) and Equation(13)
(13)
(13) . The location of the raw prediction in the CDF of (preceding) forecasts is used to compute the raw forecast’s quantile percentage (or cumulative likelihood). In the following step, ‘looking up’ the quantile in the data’s CDF updates the predicted ensemble member.
Conceptually, QM is straightforward and implementable. FX and FO can be derived either parametrically (Gudmundsson et al. Citation2012) or nonparametrically (Bennett et al. Citation2014) using a ‘lookup table’, ‘empirical distribution function’, or ‘kernel density estimation’. In particular, when dealing with tiny samples, the use of parametric models has the benefit of being less prone to sampling mistakes. Furthermore, the mapping functions that are generated by these models are often more stable (Lafon et al. Citation2013). They also make it simple to extrapolate when freshly predicted values are higher than the capabilities of the sample data that was used to construct the CDFs. This is because the CDFs are generated using sample data. The QM setup is being used here for the query. The CDFs take on the appearance of a mixed Bernoulli-gamma distribution, with the gamma distribution reflecting precipitation amounts larger than zero and the Bernoulli distribution representing rainfall probabilities. In this context, ‘precipitation amounts greater than zero’ refers to quantities of precipitation that are greater than zero. When there are many outliers and the mixed distribution cannot be fitted to the data, a nonparametric empirical cumulative distribution function is built from the data. This function is based on the data itself (Zhao et al. Citation2017).
2.6. LULC prediction
2.6.1. Markov model
The Markov model is a theory that was developed for the goals of optimal control theory and prediction. This theory is based on the way in which Markov random process systems are formed. The Markov model has the capability of displaying the transfer rate across the various categories of land use in addition to quantifying the steps involved in converting one land use type to another. It is often employed in the forecasting of geographic features without an associated event, and as a consequence, it has developed into a major forecasting tool in the field of geographic studies. The following computation forecasts changes in LU using the conditional probability formula—Bayes (Sang et al. Citation2011):
(14)
(14)
‘The system state at time t or time t + 1 is S(t), S(t + 1), and Pij is the state’s transfer likelihood matrix, which is derived as follows’ (Hou et al. Citation2004):
(15)
(15)
(16)
(16)
2.6.2. Cellular automaton (CA) model
The behavior of CA models may be affected by the uncertainty that results from interactions between model components and structures, as well as the quality of information sources that are utilized as model input. It focuses primarily on the local interactions of cells that have specific temporal and spatial connection qualities, as well as the tremendous computational capacity of space, which makes it particularly suitable for dynamic modeling and presentation along with distinctive networks that self-regulate. The use of geographic cellular automata for land use change simulations takes into account not only all of the natural factors, such as soil and climate and topography and other natural factors, but also all of the human factors, such as policy and economy and technology and other human factors, as well as the historical patterns of land use, all of which are very useful. The CA may be stated in the following way (Hou et al. Citation2004):
(17)
(17)
where ‘t and t + 1 denote the various periods, S is the set of finite and discrete cellular states, N is the cellular field, and f is the rule for transforming cellular states in local space’.
2.6.3. CA–Markov model
The CA-Markov land cover projection system combines ‘Cellular Automata, Markov Chain, Multi-Criteria, and Multi-Objective Land Allocation (MOLA)’ with a spatial contiguity component and knowledge regarding the predicted geographical pattern of changes. CA-Markov is referred to as a ‘Cellular Automata, Markov Chain, Multi-Criteria, and Multi-Objective Land Allocation (MOLA)’ land cover projection system.When making predictions about changes in land use, the Markov model emphasizes quantity. The spatial variables included in this model are insufficient and do not account for the many ways that land use has changed geographically (Wickramasuriya et al. Citation2009). The CA model has a robust concept of space, which enables it to manage the space-time dynamic growth of complex space systems. By combining the concepts of CA and Markov, the CA-Markov model concentrates on time series and space for the purpose of benefit prediction. It may be possible to develop more accurate models of the temporal and spatial patterns of change in LU. The CA-Markov module is able to anticipate the states in which land use changes will occur by combining the capabilities of cellular automaton filters with those of Markov processes. It’s possible that simulating changes in land usage might be more beneficial.
In this particular piece of research, shifts in land use have been modeled using the CA-Markov framework. Before achieving the geographical distribution of land use, vector data are first transformed into raster data. The Markov model assessment of land use patterns and the application of CA modeling, both of which are based on the CA-Markov module—a GIS and image processing module—achieve the desired results. The specific actions to take are as follows:
Markov chain analysis may be used to forecast future changes in land usage by beginning with the previous state. In order to make accurate predictions about the future, it is possible to use a matrix that contains the observed transition probabilities between maps from the past and the present. The transition probability matrix and the transfer region of the matrix may both be determined using the process of spatial overlay modeling. The transfer zone of the matrix illustrates how the predicted area will transition from one type of land use to another during the following time period. The transformation of the various land use categories into other forms of likelihood is represented by the change in the probability matrix between them. Remember that the baseline is the map of current land usage, which is positioned on the map before. Testing of the CA-Markov model will make use of the transition probability matrix that was produced as its change rules.
When CA filters are used, the space weighting factor is made readily apparent and is subject to alterations at any given time based on the characteristics of the cells that are located in the surrounding area. The neighborhood is determined in this study using the standard 55 contiguity filter. A matrix region composed of 55 cellular units surrounds each cellular center to drastically affect cellular alterations.
The current year will serve as the basis for the investigation. The number of iterations of CA was set at 15, and this was done so that the landscape spatial pattern could be replicated at the research site in the period of time that was projected. As a result, the CA-Markov model was used for this work to simulate and forecast LULC change. This procedure created transition area matrices by performing Markovian chain evaluation on the prior and base LULC maps. Next, transitional area maps of LULC were created. Finally, the model’s accuracy was assessed in order to simulate potential alterations and predict the geographic distribution of LULC within 2100.
3. Results
3.1. Soil erosion
This region’s ‘average annual soil erosion’ has been predicted while taking into account USLE and RUSLE. For a precise knowledge of the nature of erosion across the area, the spatial variance has been measured using several classifications. In USLE, the north-western, western, and southern portions of this river basin are mostly related to the geographical coverage for the ‘very high’ (>20) and ‘high’ (15-20) ‘soil erosion’ zones. The remainder of this basin has soil erosion zones that are classified as ‘moderate’ (10–15), ‘low’ (5–10), and ‘very low’ (<5) ().
The ‘north-western, western, south-western, and southern’ portions of the river basin in RUSLE are mostly connected with the ‘very high’ (>20) and ‘high’ (15-20) ‘soil erosion’ zones. The remaining portions of this basin are characterized by ‘soil erosion’ zones that are ‘moderate’ (10–15), ‘low’ (5–10), and ‘very low’ (<5) () (). For a clear underestimation of the nature of erosion, careful fieldwork has been done to identify the main erosion-prone locations. depicts a few of the main ‘erosion prone areas’ in this region.
Table 2. Areal coverage of different soil erosion zones.
3.2. Validation
The actual measured ‘soil erosion’ was taken into account while validating the models. Actual measured ‘soil erosion’ and USLE have an R2 value of 0.804, whereas RUSLE has an R-squared (R2) value of 0.883 (). In terms of quantitative efficiency, the RUSLE's optimum capacity in this area is superior to the USLE. Long rains have the potential to saturate the soil, which lowers input and increases erosional discharge, which RUSLE is better able to estimate than USLE. In contrast to USLE, RUSLE can handle convergent and divergent topography and accounts for regions with net deposition. The RUSLE has also changed since it now takes into consideration rock fragments in and on the soil. The K-factor corrects for rock in the soil layer to account for its impacts on permeability and, therefore, runoff. The C-factor considers rock particles on the soil’s surface as mulch.
3.3. Impact of climate and LULC change on soil erosion
Using predicted rainfall and ‘LULC’ data, the impact of CC on ‘soil erosion’ has been determined (). The estimated R factor values when taking SSP 126 into account vary from 369.34 to 461.07. In SSP 245, the expected R factor values vary from 376.36 to 473.85. The estimated R factor values while taking into account SSP 370 vary from 392.03 to 484.54. In the instance of SSP 585, predicted R factor values vary from 399.92 to 493.72. R factor has a propensity to rise in correlation with greater SSPs.
Figure 8. R factor in the projected period with considering SSP 126 (a), SSP 245 (b), SSP 370 (c) and SSP 585 (d).

By taking into account the ‘CA-Markov chain’ model for calculating the influence of ‘LULC’ change on ‘soil erosion,’ the future ‘LULC’ of this area has been forecasted. The 2100s are chosen as the expected time period in this viewpoint. In this light, the ‘LULC’ change from the base period has been chosen. This location has been linked to a number of ‘LULC’ categories, including ‘waterbody, dense vegetation, mixed vegetation, agricultural area, built-up area, and bare terrain’. There is a trend for thick vegetation and waterbodies to decline throughout the forecast timeframe ().
With expected rainfall and ‘LULC’ into account, the average annual ‘soil erosion’ for the predicted period has been calculated (). With greater SSPs, ‘soil erosion’ is anticipated since there is a growing tendency. In compared to the base period, the ‘very high’ and ‘high’ ‘soil erosion’ regions mostly show an increase in the projected period. In addition, the similar situation has been seen in relation to the higher SPPs. We may thus conclude from this research that ‘climate and LULC change’ may have some effect. displays the area covered for the ‘soil erosion’ zones classified as ‘very low,’ ‘low,’ ‘moderate,’ ‘high,’ and ‘very high’ while taking SSP126, SSP245, SSP370, and SSP585 into account.
Figure 10. Soil erosion in the projected period with considering SSP 126 (a), SSP 245 (b), SSP 370 (c) and SSP 585 (d).

Table 3. Areal coverage of projected soil erosion with considering different SSPs.
4. Discussion
Future ‘soil erosion’ may be increasingly severe due to CC, rising population pressure, unsuitable land use, and the loss of natural resources. As the population and land use change, the mapping and quantitative assessment of soil has increased in importance for sustainable use and the growth of conservation activities. The main causes of ‘soil erosion’ include inadequate land use, improper hill slope reduction, agricultural growth, urbanization, and a decline in plant cover. However, in certain environments, the physical process may be a major cause of erosion. As a result, human activities as well as physical processes, are linked to erosion and its accompanying sedimentation.
The global differences in ‘soil erosion’ caused by CC, the potential for ‘land use’ and ‘soil management’ strategies to mitigate these impacts, and the ambiguities surrounding these policies are all poorly understood despite several published research (Eekhout and de Vente Citation2022). Changes in severe precipitation have the most effects on SE as a consequence of CC. Extreme rainfall is expected to rise because a hotter atmosphere can hold more moisture, leading to a more active hydrological cycle (Parr and Wang Citation2014). Globally, data over a long period of time show an increase in heavy rainfall, and projections from climate models show that this trend will continue in the next decades (Fischer et al. Citation2013). Excessive rainfall results in ‘soil erosion’ due to drainage as well as separating soil particles due to the impact of raindrops (Römkens et al. Citation2002).
It is commonly known that altering how land is used has a vital influence on ‘soil erosion,’ which is connected to CC (Borrelli et al. Citation2017). Predicting how ‘land use’ will change under ‘future climate conditions’ is not a simple undertaking, however, given the evident large range of elements that operate at local to global dimensions (Riebsame et al. Citation1994). Due to this complexity, only a few studies have looked at how ‘land use change’ and increased plant growth relate to how ‘soil erosion’ is affected by climate change (Eekhout and de Vente Citation2022). The projected increase in ‘soil erosion’ as a consequence of CC is commonly used as an argument for soil conservation (Altieri et al. Citation2015). By providing important biological services like carbon and nitrogen sequestration in addition to reducing ‘soil erosion,’ soil conservation techniques may aid in the mitigation of CC and the preservation of biodiversity. According to this viewpoint, soil erosion has a specific effect on the local climate. Therefore, this kind of issue might be seen as a major environmental concern that could have a negative impact on society.
In mountainous locations, CC, unsustainable LU practices, and steep terrain all contribute to soil erosion (Tarolli and Straffelini Citation2020). In order to estimate the hydrological, ecological, and soil carbon dynamics reactions to global warming, it is essential to evaluate how climate change affects soil erosion. Therefore, assessing the effect of CC on erosion is essential for comprehending the consequences for the ecosystem and food security. Only the quantity, intensity, and erosivity of rainfall are impacted by climate change (Nearing et al. Citation2004). In the present context for land use planning and management, CC is, therefore, a crucial role. This conservation planning requires spatially dispersed soil erosion data. Users and politicians must be informed of this information in order to ensure the sustainability of farming.
During calculating the ‘average annual soil erosion’ during the base period, the ‘USLE’ and ‘RUSLE’ have been taken into consideration in this study. The result was then verified by taking into account primary measured erosion data. This research reveals that ‘RUSLE’ is more quantitatively efficient than ‘USLE’ in comparison. When it comes to modeling erosion in various circumstances, ‘RUSLE’ is more adaptable and effective than ‘USLE’ (Wischmeier and Smith Citation1965). Empirical and process-based design is combined in ‘RUSLE, ’ which has an advantage over ‘USLE’ in terms of dataset utilization. Because RUSLE components are divided into sub-factors, you have additional alternatives when it comes to assessing soil loss. It also makes it possible to estimate deposits via sediment transfer. RUSLE applies a concept known as ‘rainfall erosivity’ in order to determine how much of an impact rainfall has on erosion. Since rainfall erosivity is a combined evaluation of the amount and ‘kinetic energy of rainfall’, the ‘sub-hourly rainfall’ occurrences provide the most accurate results when used to make the determination. According to ‘RUSLE’, ‘soil erosion’ is exactly proportional to ‘rainfall erosivity’ as long as other characteristics such as ‘topography, soil type, land cover, and management’ stay unchanged.
In this viewpoint, ‘RUSLE’ has been used to quantify the expected ‘soil erosion’. To simulate the probable effects of ‘climate’ and ‘LULC’ change on SE, the expected rainfall and its related R factor and ‘LULC’ were utilized. Monsoon rains make SE worse. Numerous major environmental problems are brought on by ‘soil erosion’. Sediment is a result of SE and is unusual in that it may serve as both a pollutant and a carrier for further contaminants that may be deposited onto soil particles. A practical and effective strategy for lowering contaminant transmission via sediment formation is stabilization of the sediment source by restricting ‘soil erosion’ through the use of appropriate management techniques (Toy et al. Citation2002).
5. Conclusion
One of the most important factors affecting land gradation in an area dominated by ‘sub-tropical monsoon’ is the issue of ‘soil erosion’. The majority of the subtropical zone is already free from the issue of severe climatic events worldwide. On the other hand, human actions have the ability to significantly alter the environment. Future estimations of the potential impact of ‘climate and LULC change’ on ‘soil erosion’ are thus necessary. ‘Average annual soil erosion’ has been calculated for the base period while taking ‘USLE’ and ‘RUSLE’ into account. In this case, ‘RUSLE’ offers more computing efficiency than ‘USLE.’ In this viewpoint, ‘soil erosion’ has been forecasted using the RUSLE, while ‘rainfall’ and ‘LULC’ simulations have been taken into account. In comparison to the base era, there is a growing trend for higher ‘soil erosion’ zones. The higher projected ‘soil erosion’ has been seen, going by higher SSPs. So the impact of climate and LULC change on future soil erosion has been established in this research. Due to the enhanced erosive potential of the upcoming severe rainfall, the soil loss rate is thus anticipated to rise under both scenarios. Future estimations of the potential impact of CC and ‘LULC’ change on ‘soil erosion’ are thus necessary. The primary goal of future investigators will be to use this methodology across many socio-political aspects while taking the environment’s geography into consideration.
Disclosure statement
No potential conflict of interest was reported by the authors.
Data availability statement
The data will be made available upon request by the first author.
References
- Alewell C, Borrelli P, Meusburger K, Panagos P. 2019. Using the USLE: chances, challenges and limitations of soil erosion modelling. Int Soil Water Conserv Res. 7(3):203–225. doi:10.1016/j.iswcr.2019.05.004.
- Allan RP, Barlow M, Byrne MP, Cherchi A, Douville H, Fowler HJ, Gan TY, Pendergrass AG, Rosenfeld D, Swann ALS, et al. 2020. Advances in understanding large‐scale responses of the water cycle to climate change. Ann N Y Acad Sci. 1472(1):49–75. doi:10.1111/nyas.14337.
- Altieri MA, Nicholls CI, Henao A, Lana MA. 2015. Agroecology and the design of climate change-resilient farming systems. Agron Sustain Dev. 35(3):869–890. doi:10.1007/s13593-015-0285-2.
- Anderson RL, Rowntree KM, Le Roux JJ. 2021. An interrogation of research on the influence of rainfall on gully erosion. CATENA. 206:105482. doi:10.1016/j.catena.2021.105482.
- Aryal B, Gurung R, Camargo AF, Fongaro G, Treichel H, Mainali B, Angove MJ, Ngo HH, Guo W, Puadel SR. 2022. Nitrous oxide emission in altered nitrogen cycle and implications for climate change. Environ Pollut. 314:120272. doi:10.1016/j.envpol.2022.120272.
- Bennett JC, Grose MR, Corney SP, White CJ, Holz GK, Katzfey JJ, Post DA, Bindoff NL. 2014. Performance of an empirical bias‐correction of a high‐resolution climate dataset. Intl Journal of Climatology. 34(7):2189–2204. doi:10.1002/joc.3830.
- Bhattacharya RK, Das Chatterjee N. 2021. Geomorphic threshold and sand mining: a geo-environmental study in Kangsabati River. In River sand mining modelling and sustainable practice: the Kangsabati River, India. Springer Nature Switzerland AG; p. 21–50.
- Borrelli P, Robinson DA, Fleischer LR, Lugato E, Ballabio C, Alewell C, Meusburger K, Modugno S, Schütt B, Ferro V, et al. 2017. An assessment of the global impact of 21st century land use change on soil erosion. Nat Commun. 8(1):2013. doi:10.1038/s41467-017-02142-7.
- Borrelli P, Robinson DA, Panagos P, Lugato E, Yang JE, Alewell C, Wuepper D, Montanarella L, Ballabio C. 2020. Land use and climate change impacts on global soil erosion by water (2015-2070). Proc Natl Acad Sci U S A. 117(36):21994–22001. doi:10.1073/pnas.2001403117.
- Casanueva A, Herrera S, Iturbide M, Lange S, Jury M, Dosio A, Maraun D, Gutiérrez JM. 2020. Testing bias adjustment methods for regional climate change applications under observational uncertainty and resolution mismatch. Atmos Sci Lett. 21(7):e978. doi:10.1002/asl.978.
- Chakraborty SK, Chakraborty SK. 2021. Physiography of rivers: relevant hypothesis and theories. In Riverine Ecology Volume 1: eco-Functionality of the Physical Environment of Rivers, Springer Nature Switzerland AG, 235–374.
- Dignac M-F, Derrien D, Barré P, Barot S, Cécillon L, Chenu C, Chevallier T, Freschet GT, Garnier P, Guenet B, et al. 2017. Increasing soil carbon storage: mechanisms, effects of agricultural practices and proxies. A review. Agron Sustain Dev. 37(2):14. doi:10.1007/s13593-017-0421-2.
- Doulabian S, Toosi AS, Calbimonte GH, Tousi EG, Alaghmand S. 2021. Projected climate change impacts on soil erosion over Iran. J Hydrol. 598:126432. doi:10.1016/j.jhydrol.2021.126432.
- Eekhout JP, De Vente J. 2020. How soil erosion model conceptualization affects soil loss projections under climate change. Prog Phys Geogr: Earth Environ. 44(2):212–232. doi:10.1177/0309133319871937.
- Eekhout JP, Hunink JE, Terink W, de Vente J. 2018. Why increased extreme precipitation under climate change negatively affects water security. Hydrol Earth Syst Sci. 22(11):5935–5946. doi:10.5194/hess-22-5935-2018.
- Eekhout JP, de Vente J. 2019. The implications of bias correction methods and climate model ensembles on soil erosion projections under climate change. Earth Surf Processes Landf. 44(5):1137–1147. doi:10.1002/esp.4563.
- Eekhout JPC, de Vente J. 2022. Global impact of climate change on soil erosion and potential for adaptation through soil conservation. Earth Sci Rev. 226:103921. doi:10.1016/j.earscirev.2022.103921.
- Etehadi Abari M, Majnounian B, Malekian A, Jourgholami M. 2017. Effects of forest harvesting on runoff and sediment characteristics in the Hyrcanian forests, northern Iran. Eur J Forest Res. 136(2):375–386. doi:10.1007/s10342-017-1038-3.
- Falco M, Carril AF, Li LZ, Cabrelli C, Menéndez CG. 2020. The potential added value of Regional Climate Models in South America using a multiresolution approach. Clim Dyn. 54(3-4):1553–1569. doi:10.1007/s00382-019-05073-9.
- Fischer EM, Beyerle U, Knutti R. 2013. Robust spatially aggregated projections of climate extremes. Nature Clim Change. 3(12):1033–1038. doi:10.1038/nclimate2051.
- Giorgi F. 2019. Thirty years of regional climate modeling: where are we and where are we going next? JGR Atmospheres. 124(11):5696–5723. doi:10.1029/2018JD030094.
- Gudmundsson L, Bremnes JB, Haugen JE, Engen-Skaugen T. 2012. Downscaling RCM precipitation to the station scale using statistical transformations–a comparison of methods. Hydrol Earth Syst Sci. 16(9):3383–3390. doi:10.5194/hess-16-3383-2012.
- Hazell P, Poulton C, Wiggins S, Dorward A. 2010. The future of small farms: trajectories and policy priorities. World Development. 38(10):1349–1361. doi:10.1016/j.worlddev.2009.06.012.
- Hou X, Chang B, Yu X. 2004. Land use change in Hexi corridor based on CA-Markov methods. Nongye Gongcheng Xuebao (Trans Chinese Soc Agric Eng). 20(5):286–291.
- Kalognomou E-A, Lennard C, Shongwe M, Pinto I, Favre A, Kent M, Hewitson B, Dosio A, Nikulin G, Panitz H-J, et al. 2013. A diagnostic evaluation of precipitation in CORDEX models over southern Africa. J Climate. 26(23):9477–9506. doi:10.1175/JCLI-D-12-00703.1.
- Keating BA, Herrero M, Carberry PS, Gardner J, Cole MB. 2014. Food wedges: framing the global food demand and supply challenge towards 2050. Global Food Secur. 3(3-4):125–132. doi:10.1016/j.gfs.2014.08.004.
- Kinnell P. 2017. A comparison of the abilities of the USLE-M, RUSLE2 and WEPP to model event erosion from bare fallow areas. Sci Total Environ. 596-597:32–42. doi:10.1016/j.scitotenv.2017.04.046.
- Lafon T, Dadson S, Buys G, Prudhomme C. 2013. Bias correction of daily precipitation simulated by a regional climate model: a comparison of methods. Int J Climatol. 33(6):1367–1381. doi:10.1002/joc.3518.
- Lal R. 2004. Soil carbon sequestration to mitigate climate change. Geoderma. 123(1-2):1–22. doi:10.1016/j.geoderma.2004.01.032.
- Li Z, Fang H. 2016. Impacts of climate change on water erosion: a review. Earth Sci Rev. 163:94–117. doi:10.1016/j.earscirev.2016.10.004.
- Lin H, Cheng X, Bruijnzeel LA, Duan X, Zhang J, Chen L, Zheng H, Lu S, Dong Y, Huang J, et al. 2023. Land degradation and climate change lessened soil erodibility across a wide area of the southern Tibetan Plateau over the past 35–40 years. Land Degrad Dev. 34(9):2636–2651. doi:10.1002/ldr.4636.
- Mandal B, Bej D, Baghmar N. 2021. Environmental impact and management of sand mining: a case study of Kangsabati River Watershed, West Bengal using remote sensing and GIS technique. Int J Technol Res Manag. 8(8):1–9.
- Nearing M, Lane LJ, Lopes VL. 2017. Modeling soil erosion. In Soil erosion research methods. Routledge; p. 127–158.
- Nearing M, Pruski F, O'Neal M. 2004. Expected climate change impacts on soil erosion rates: a review. J Soil Water Conserv. 59(1):43–50.
- Nearing MA, Yin S, Borrelli P, Polyakov VO. 2017. Rainfall erosivity: An historical review. Catena. 157:357–362. doi:10.1016/j.catena.2017.06.004.
- O'Neal MR, Nearing MA, Vining RC, Southworth J, Pfeifer RA. 2005. Climate change impacts on soil erosion in Midwest United States with changes in crop management. Catena. 61(2-3):165–184. doi:10.1016/j.catena.2005.03.003.
- Ouyang W, Wu Y, Hao Z, Zhang Q, Bu Q, Gao X. 2018. Combined impacts of land use and soil property changes on soil erosion in a mollisol area under long-term agricultural development. Sci Total Environ. 613-614:798–809. doi:10.1016/j.scitotenv.2017.09.173.
- Parr D, Wang G. 2014. Hydrological changes in the US Northeast using the Connecticut River Basin as a case study: part 1. Modeling and analysis of the past. Global Planet Change. 122:208–222. doi:10.1016/j.gloplacha.2014.08.009.
- Plangoen P, Babel M, Clemente R, Shrestha S, Tripathi N. 2013. Simulating the impact of future land use and climate change on soil erosion and deposition in the Mae Nam Nan Sub-Catchment, Thailand. Sustainability. 5(8):3244–3274. doi:10.3390/su5083244.
- Pongratz J, Reick C, Raddatz T, Claussen M. 2008. A reconstruction of global agricultural areas and land cover for the last millennium. Global Biogeochem Cycles. 22(3):n/a–n/a. doi:10.1029/2007GB003153.
- Rajbanshi J, Bhattacharya S. 2022. Modelling the impact of climate change on soil erosion and sediment yield: a case study in a sub-tropical catchment, India. Model Earth Syst Environ. 8(1):689–711. doi:10.1007/s40808-021-01117-4.
- Riebsame WE, Meyer WB, Turner B. 1994. Modeling land use and cover as part of global environmental change. Clim Change. 28(1-2):45–64. doi:10.1007/BF01094100.
- Römkens MJ, Helming K, Prasad S. 2002. Soil erosion under different rainfall intensities, surface roughness, and soil water regimes. Catena. 46(2-3):103–123. doi:10.1016/S0341-8162(01)00161-8.
- Sang L, Zhang C, Yang J, Zhu D, Yun W. 2011. Simulation of land use spatial pattern of towns and villages based on CA–Markov model. Math Comput Modell. 54(3-4):938–943. doi:10.1016/j.mcm.2010.11.019.
- Segura C, Sun G, McNulty S, Zhang Y. 2014. Potential impacts of climate change on soil erosion vulnerability across the conterminous United States. J Soil Water Conserv. 69(2):171–181. doi:10.2489/jswc.69.2.171.
- Smith P, House JI, Bustamante M, Sobocká J, Harper R, Pan G, West PC, Clark JM, Adhya T, Rumpel C, et al. 2016. Global change pressures on soils from land use and management. Glob Chang Biol. 22(3):1008–1028. doi:10.1111/gcb.13068.
- Stefanidis S, Alexandridis V, Mallinis G. 2022. A cloud-based mapping approach for assessing spatiotemporal changes in erosion dynamics due to biotic and abiotic disturbances in a Mediterranean Peri-Urban forest. Catena. 218:106564. doi:10.1016/j.catena.2022.106564.
- Switanek MB, Troch PA, Castro CL, Leuprecht A, Chang H-I, Mukherjee R, Demaria E. 2017. Scaled distribution mapping: a bias correction method that preserves raw climate model projected changes. Hydrol Earth Syst Sci. 21(6):2649–2666. doi:10.5194/hess-21-2649-2017.
- Tamene L, Adimassu Z, Ellison J, Yaekob T, Woldearegay K, Mekonnen K, Thorne P, Le QB. 2017. Mapping soil erosion hotspots and assessing the potential impacts of land management practices in the highlands of Ethiopia. Geomorphology. 292:153–163. doi:10.1016/j.geomorph.2017.04.038.
- Tanyaş H, Kolat Ç, Süzen ML. 2015. A new approach to estimate cover-management factor of RUSLE and validation of RUSLE model in the watershed of Kartalkaya Dam. J Hydrol. 528:584–598. doi:10.1016/j.jhydrol.2015.06.048.
- Tarolli P, Straffelini E. 2020. Agriculture in hilly and mountainous landscapes: threats, monitoring and sustainable management. Geography Sustain. 1(1):70–76. doi:10.1016/j.geosus.2020.03.003.
- Teng H, Liang Z, Chen S, Liu Y, Viscarra Rossel RA, Chappell A, Yu W, Shi Z. 2018. Current and future assessments of soil erosion by water on the Tibetan Plateau based on RUSLE and CMIP5 climate models. Sci Total Environ. 635:673–686. doi:10.1016/j.scitotenv.2018.04.146.
- Thomas J, Joseph S, Thrivikramji KP. 2018. Assessment of soil erosion in a monsoon-dominated mountain river basin in India using RUSLE-SDR and AHP. Hydrol Sci J. 63(4):542–560. doi:10.1080/02626667.2018.1429614.
- Tiwari H, Rai S, Kumar D, Sharma N. 2016. Rainfall erosivity factor for India using modified Fourier index. J Appl Water Eng Res. 4(2):83–91. doi:10.1080/23249676.2015.1064038.
- Toy TJ, Foster GR, Renard KG. 2002. Soil erosion: processes, prediction, measurement, and control. New York: John Wiley & Sons.
- Walsh KJ, McBride JL, Klotzbach PJ, Balachandran S, Camargo SJ, Holland G, Knutson TR, Kossin JP, Lee T, Sobel A, et al. 2016. Tropical cyclones and climate change. Wiley Interdiscip Rev Clim Change. 7(1):65–89. doi:10.1002/wcc.371.
- Wang F, Tian D. 2022. On deep learning-based bias correction and downscaling of multiple climate models simulations. Clim Dyn. 59(11-12):3451–3468. doi:10.1007/s00382-022-06277-2.
- Wickramasuriya RC, Bregt AK, Van Delden H, Hagen-Zanker A. 2009. The dynamics of shifting cultivation captured in an extended Constrained Cellular Automata land use model. Ecol Modell. 220(18):2302–2309. doi:10.1016/j.ecolmodel.2009.05.021.
- Wischmeier WH, Smith DD. 1965. Predicting rainfall-erosion losses from cropland east of the rocky mountains: guide for selection of practices for soil and water conservation. [place unknown]: Agricultural Research Service, US Department of Agriculture.
- Xiong M, Sun R, Chen L. 2019. Global analysis of support practices in USLE-based soil erosion modeling. Prog Phys Geogr: Earth Environ. 43(3):391–409. doi:10.1177/0309133319832016.
- Zhao T, Bennett JC, Wang QJ, Schepen A, Wood AW, Robertson DE, Ramos M-H. 2017. How suitable is quantile mapping for postprocessing GCM precipitation forecasts? J Climate. 30(9):3185–3196. doi:10.1175/JCLI-D-16-0652.1.