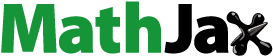
Abstract
Landslides rank as the third most common natural disaster globally, and the Indian Himalaya Region is no exception, experiencing severe impacts during the rainy season. This study focuses on creating a comparative landslide susceptibility map for the West Sikkim district in India using probabilistic and heuristic approaches. The frequency ratio (FR) and information value (IV) methods are employed for the probabilistic approach, while the analytic hierarchy process (AHP) is used for the heuristic approach. Eleven factors are considered in the analysis. The resulting landslide susceptibility (LS) map demonstrates accuracies of 77% for FR, 74% for IV, and 57% for AHP methods. Preliminary qualitative risk assessment is conducted, incorporating building and population density, as population and buildings are the most vulnerable elements in the society. The LS map with the highest accuracy (from FR) serves as the landslide potential factor, combined with building and population density as the risk damage potential factors for risk zonation. The resulting risk zonation map classifies the study area into high-risk (3%), medium-risk (14%), and low-risk (83%) zones. This study primarily addresses the 3% high-risk area where landslides pose a significant threat to population and infrastructure, aiming to inform policy implementation and mitigation measures.
1. Introduction
A mountainous terrain may pose a serious threat to communities living in it owing to landslide disasters that are generated both by unsustainable anthropogenic activities such as mining, road cutting or urbanization, and by natural factors such as earthquakes and excessive rainfall (Martha Citation2011; Dai et al. Citation2022). The Himalayan region receives a lot of rainfall and accounts for more than 70% of all fatal landslides in India (Froude and PetleyCitation2018). The majority of Himalayan region including Indian Himalayan region is classified as a major risk zone due to the incidence of a variety of natural disasters, including landslides. The Sikkim Himalaya in this context experiences similar fate. Landslides are defined as the movement of debris, rock mass, or earth down a slope as a result of gravity (Cruden Citation1991; Yalcin et al. Citation2011). A landslide can inflict significant economic impact, especially in densely populated areas where dislodged rocks hinder highways and sub-roads. As a result of global and micro climate change, population growth, and ever-expanding transportation networks, the mortality toll has risen substantially in recent years (Althuwaynee and Pradhan Citation2017). Landslide risk has increased in recent decades as a result of population growth, which has resulted in the extension of residential areas; the construction of new settlements on steeper slopes and/or in regions with inadequate foundation (bedrock, soil) in terms of structure and stability (Guzzetti et al. Citation1999; Yalcin et al. Citation2011; Zhong et al. Citation2020). The expansion of infrastructure as a result of population increase in the mountainous terrains of the Himalayas is the greatest source of concern for the incidence of landslides (Jaiswal et al. Citation2011). Landslides are often discrete events that are not particularly enormous in scale, they can occur with a high frequency in a given region and do significant damage to both human and physical infrastructure (Van Westen et al. Citation2006). This is due to a higher susceptibility of surface soil to instability, as well as a higher vulnerability of the exposed population (Nadim et al. Citation2006; Hoyois et al. Citation2007). As a result of urbanization, more hill slopes are excavated ‘indiscriminately’, leaving them exposed (Jaiswal Citation2011). Excavated slopes along highways can cause landslides, affecting not only the alignments, automobiles, and people, but also the economy (Van Westen et al. Citation2006). Landslides are considered to be the third most catastrophic type of natural disaster in the world (CitationZillman1999; Castellanos Citation2008). In the Indian context too, landslides are ranked third in terms of devastation caused by natural disasters, trailing only floods and hurricanes (Atlas Magazine Citation2020). According to Kirschbaum et al. (2010), developing countries account for roughly 80% of all landslide fatalities reported worldwide in the recent decade, with India accounting for nearly 8% of all landslide fatalities (Ghosh Citation2011). To comprehend a landslide disaster and mitigate its influence on society, it is vital to make a realistic assessment of the projected disaster losses. The evaluation entails quantifying both direct and indirect risks quantitatively, which requires a large number of input factors to measure each component of hazard, vulnerability, and element at risk (Fell et al. Citation2008; Jaiswal Citation2011). Most research focus on landslide identification, dynamic modelling, and susceptibility analysis, while few focus on landslide hazard and risk quantification.As a result, defining Landslide susceptibility (LS) is the first step in assessing landslide risk (Kouli et al. Citation2010; Rozos et al. Citation2011; Kayastha et al. Citation2013; Foumelis et al. Citation2018).Landslide risk assessment, which includes landslide hazard analysis, vulnerability analysis of disaster-bearing bodies, and risk zonation, has steadily become one of the most important subjects in the field of landslide study (Sui et al. Citation2020).The LS term is frequently used to refer to the location of probable landslides in a certain area, determined by a set of topographical criteria. Future landslides could be induced by the same conditioning parameters that triggered current and previous landslides, according to various research conducted in the past (Carrara et al. Citation1999). Because qualitative approaches rely on expert opinion, they are very subjective, with the weight of the elements that lead to vulnerability being assessed based on expert knowledge and experience (Clerici et al. Citation2010; Chalkias et al. Citation2014; Tsangaratos et al. Citation2017). Semi-quantitative techniques are qualitative methodologies that work in weighting and rating procedures (Ayalew et al. Citation2005; Achour et al. Citation2017).As of now, only a little amount of research has been done to determine the vulnerability and risk of buildings and infrastructures that are vulnerable to landslide disasters (Singh et al. Citation2021).
Considering data availability, codal provisions, and the geographical conditions of the Indian subcontinent, this work aims to establish a preliminary qualitative risk assessmentwith respect to population and building densityfor hilly terrains of the study area. For the purposes of this definition, risk is a measure of the possibility and severity of a devastating impact on one’s health, property, or environment (AGS (Australian Geomechanics Society Sub-Committee on Landslide Risk Management) Citation2000). Varnes (Citation1984) defined landslide risk as the likelihood of lives being lost, people being injured, property being destroyed, and economic activity being hampered as a result of a specific landslide event occurring in a specific area and reference time.Landslide risk assessment requires several parameters, making quantification challenging (Van Westen et al. Citation2006; Li et al. Citation2014). Many research has been done on risk zonation, however the methods used to quantify landslide risk differ according on the scale, mechanism, and data availability. Also, due to the considerable variation in terrain, geology, climate, etc., no common framework for assessing landslide risk exists (Singh et al. Citation2017). The spatial examination of susceptibility to landslide hazards and the assessment of exposure to this particular hazard make up landslide risk assessment (Mishra and Sarkar Citation2020; Nor Diana et al. Citation2021). Remote sensing and GIS have recently given a new dimension to environmental hazard and risk mapping. This technique can manipulate, acquire, store, retrieve, and model large Spatio-temporal data (Panchal et al. Citation2015), and can be used to augment other susceptibility mapping methods and models (Akbari et al. Citation2014). The literature reveals that different strategies were used depending on the objectives, scale, and location of the problem. All of these models have flaws, however, there is no broad consensus on whether method or model is best for assessing landslide risk (Mishra and Sarkar Citation2020). Apart from illicit hill cutting and mining, increased landslide activity in the Himalayan region has been linked to the construction of road networks (Singh et al. Citation2014; Froude and PetleyCitation2018; Hosenuzzaman et al. Citation2022).According to the research conducted by Dikshit et al. (Citation2020), about 15% landslides were occurred in the Indian Himalayan Region from 2004-2017 with respect to the global context. The Himalayan landslides are basically triggered by seimic activity, rainfall and growing anthropogenic activities. Lots of studies in the landslide study in the Himalayan region deals in the susceptibility mapping but there are very few or no studies regarding the early warning and risk assesement especially in the eastern Himayalan region (Dikshit et al. Citation2020). There has also been a lot of studies in climate change and global warming but the exact link is yet to be established in relation to the landslides especially in the context of Indian Himalaya (Dikshit et al. Citation2020). Some of the past landslides in the Indian Himalaya and their loss are shown in the .
Table 1. Past landslides in the Indian Himalaya and their losses.
The main goal of this study is to quantify landslide hazard probability and preliminary qualitative risk zonation in the study area with limited available data. Despite these restrictions, an attempt has been made in this study for risk zonation in West Sikkim district of India. The first section discusses the use of statistical and knowledge-driventechniques to evaluate landslide hazard using data from a landslide inventory. To quantify risk, detailed information on the vulnerability and number of elements at risk is required, as well as the chance of a specific hazard scenario occurring in a given area over a given return period (Vranken et al. Citation2015). Lack of temporal data on landslide incidence is one reason why landslide risk assessment and mapping remain challenging (Pellicani et al. Citation2014). Current study tries to analyze the qualitative risk zonation using the landslide potential factors and the risk damage potential.
1.1. Study area
The study area falls in the north eastern part of India in the Lesser and Greater Himalaya of the Eastern Himalaya covering West Sikkim district of Sikkim (). Thestudy area West Sikkim which covers an area of 1162 sq. km is bounded by 27.614° N − 27.112° N (latitude) and 88.017° E − 88.353°E (longitude), it ranges56km from North to South 35 km East to West. The elevation in the study area ranges from 269 m to 7047 m above mean sea level and slope ranges from 0 to 86°.
Forested ecosystems account for more than 50% of totalstudy area. Sikkim, which makes up 0.20% of India’s total land area, is regarded one of the ecological hotspots to the east of the Himalayas (Govt. of Sikkim, 2022).The lush green vegetation in the area is because of the prolonged and intense rainfall that occurs in both the winter and summer seasons. The mean daily temperature ranges from 4° C (January) to 17° C (July). Kangchenjunga range, which holds the world’s third-highest mountain peak covers northern part of the West Sikkim district (Sharma et al. Citation2023).Because the structural slope runs from north to south, all of the state’s major drainage flow in a southerly direction. Rangit river is the major river in the study area (Hazra and Krishna Citation2019). The interaction between denudational and depositional processes responsible for generating the land surfaces of the Sikkim-Himalayan area can be understood in general terms. Landslides and other mass movements are common in the state. This is because of the fragile lithology, rainfall, ancient debris flow, palaeo-landslides, and complex sloping conditions which render the situation sensitive to slope instability (Rawat Citation2015). During the monsoon season, the study region receives 300-900 mm of rainfall each month, which causes the most landslides. The relative humidity still remains very high during the monsoon seasons, providing adequate moisture for the rock and soil, particularly with clay-rich soils and rocks that allow swelling, causing unpredictable landslides in places. Increasing landslide/soil erosion problems in various locations under different lithology/physiographic zones, relief, angles of slope, and altitudes may pose a threat to rare and endangered species. Sometimes thick piles of debris block the river’s flow paths and when these breaches of natural blocks create flash floods in the downstream regions (Rawat Citation2015). Many small and large landslides studied in West Sikkim district during the present study and attempts were made to assess the likelihood of susceptibility of these slides. Further these susceptibility factors coupled with risk damage potential are used for the preparation of risk zonation map. Some of the significant landslides surveyed during the field visits in the study area are shown in the figure ().
1.2. Geology of study area
The tectonic heterogeneity, polyphase metamorphism, and unfossiliferous nature of the litho units have led to scholarly debate, appraisal, and re-evaluation (Basu Citation2013). The geological formations of the state are arranged in reverse tectonic order from the south to the north of the state (Auden Citation1935). The study area falls in the Lesser and Greater Himalayan division. Low to medium grade Precambrium metapelites and the Baxa carbonate-quartzite association (Daling Group) dominate the landscape of Sikkim Himalaya, which is also covered by high grade gneiss (Darjeeling Group) and deformed granite gneiss (Lingtse Granite Gneiss) (Bose Citation1891, Acharyya Citation1989).Gondwana-equivalent Rishi Group (Namchi formation, Rangit Pebble Slate) and Tethyan rocks are among the Palaeozoic Mesozoic rocks (Everest Pelite, Everest limestone, Lachi, and ChhoLahmo Formations) (Ray Citation1976).The rocks can be seen highly deformed due to the different stages of orogeny (SSDMA Citation2022). Microfractures, fissures, joints, foliation surfaces, faults (shear), and several generations of folds are all examples of deformation. Each of these structures, which range in age from Proterozoic to Mesozoic, has its own set of tectono-sedimentary, magmatic, metamorphic, and structural features. The principal lithotypes of the Sikkim Darjeeling Himalaya, which have complicated fold-thrust tectonostratigraphic sequences that generate nappes, windows, and klippen, among other structures. The main central thrust (MCT) is the major geological fault and the most critical tectonic zone passes through the study area. The Tista Lineament, the biggest lineament in the district passes almost diagonally through east to west of the district (). The planar and the non-planar discontinuities are the chief source of instability in the area.
2. Materials and methods
The first objective of the current work is to prepare a landslide susceptibility map (LSM) based on the comparative study of different methods for the West Sikkim district of the Sikkim Himalaya. As we have already known by now that there is no fixed guideline or rule for preparing a landslide susceptibility map of any study area, the selection of causative factors for landslides in statistical and data-driven methods generally depends on the nature of the study area and the availability of the data (Ayalew and Yamagishi Citation2005; Magliulo et al. Citation2008; Yalcin Citation2008). The different methods used here are frequency ratio method (FR), information value method (IV) and analytic hierarchy process (AHP). The first two methods are statistical driven probabilistic methods while AHP is the knowledge driven heuristic approach. Probabilistic methods are used to calculate the weights of various conditioning factors and the weights are applied to generate the landslide susceptibility map. On the other hand, the heuristic method used the weights based on the expert judgement.The comparative method helps us to choose the best fit method. As there is no universal guideline for the selection of the landslide conditioning factors, therefore depending on the terrain of the study area and the data availability, eleven factors are considered for the preparation of the landslide susceptibility map. These factors are slope aspect, slope angle, slope curvature, drainage density, elevation, lithology, land use and land cover (LULC), normalized difference vegetation index (NDVI), geomorphology, lineament density, and soil type. The principal objective of the study is preparing a landslide risk zonation map for the study area, which can be used for planning for the policy makers and the administrators. Because landslides wreak havoc on settlement areas, landslide risk assessment must be factored into urban planning strategies.The best fit susceptibility map was used to calculate the interrelationship between each landslide causative factor and landslide distribution in order to assess landslides. The detailed methodology adopted in the study is given below (). The data sets and the software used for the study are shown in and .
Table 2. Data sets used for the present study.
Table 3. Software used for the present study.
2.1. Landslide mapping
Identification of landslide incidents was made possible through the use of satellite image (Resourcesat, LISS 4) visual interpretation in conjunction with field investigations and previous records from local administration. The satellite image has a spatial resolution of 5 m after correction. The processes of detection, identification, description, and evaluation of the significance of an item and pattern imaged, are referred to as interpretation. The basic elements that are considered while doing the visual interpretation are shape, size, pattern, tone, texture, shadows, location, association, etc. The locations of these landslides, as well as their coordinates and borders, were marked in the map in the form of polygons.In this study, a total of 685 landslides were mapped and these were further divided in the 70:30 ratio using the random sampling in the GIS tool, as most of the researchers use this proportion for their study (Martha et al. Citation2013). 70% landslides were taken as the training data set, and the remaining 30% landslides were used for the testing of the model. The landslide in the study area has a maximum area of 0.33 square kilometres and a minimum area of 0.000123 square kilometres, with a total area of about 3 square kilometres. The mapped landslides were also validated in during the field survey and in Google Earth image.
2.2. Frequency ratio method
For landslide susceptibility analysis, the frequency ratio model is a bivariate statistical analysis that examines landslide events and associated factors (Lee and Pradhan Citation2006). The frequency ratio model is based on the relationship between landslide distribution and each of the factors that affect landslides (Mandal et al. Citation2018). The frequency ratio value is calculated by dividing the percentage of landslide pixels by the percentage of entire area pixels in each factor’s class (Pradhan and Youssef Citation2010). A value greater than 1 indicates a higher correlation, while a value less than 1 indicates a lower correlation with respect to the occurrence of landslides. EquationEquation (1)(1)
(1) was used to calculate the frequency ratio (Arabameri et al. Citation2019).
(1)
(1)
Where A is the number of landslide pixels in each factor class, B is the total number of landslide pixels in the study area, C is the total number of pixels in each factor class, D is the total number of pixels in the study area, E is the percentage of landslide events in each factor class of the variables, and F is the relative percentage of the area of the total area for each subclass.
The FR values of the conditioning factors are consolidated together in a GIS environment to produce a landslide susceptibility index (Equationequation 2(2)
(2) ).
(2)
(2)
Here, i = weights of landslide conditioning factors.
All thematic maps are reclassified according to the FR values for each factor class and then integrated into GIS to produce the landslide susceptibility index map (Sharma and Mahajan Citation2018).
2.3. Information value model (IVM)
The risk of landslide hazards of any area can be calculated based on the contribution and the relationship between causative factors (Sarkar et al. Citation2013). The information value model is a statistical method for spatial prediction of an event based on the relationship of the parameter and the event. This is a bivariate statistical approach that is based on the frequency of the landslide event on Landslide Inducing Factor spatial distribution over the study region (Cao et al. Citation2016; Banerjee et al. Citation2018). It is an indirect statistical approach and could be used to assess the spatial relationship between the probability of the occurrence of landslides and the classes of the conditioning factor (Du et al. Citation2019). In this model, the probability of occurrence of landslides is influenced by factor information value (Luo et al. Citation2019). The summation of the landslide pixel ratios per factor class (Si/Ni) to the total landslide pixel per factor (S/N) gives statistics for the landslide susceptibility index (Sharma Citation2018). In this process, the spatial prediction of landslides is based on the relationship between the landslide causative factors and landslides. The performance of this model depends on the variables of landslide conditioning being crossed with the landslide distribution (Singh and Kumar Citation2018). To evaluate the weights of the predictors, it is appropriate to measure the ratio of the density of landslide occurrence in each class of a causative factor to the density of landslides in total area (Chen et al. Citation2014). The Equationequation (3)(3)
(3) for the calculation of weight is given below:
(3)
(3)
where, Wt = Weight of a factor class; ln = natural logarithm; Npix(Si) = Number of pixel of landslide within class i; Npix(Ni) = Number of pixel of class i; ΣNpix(Si) = Number of pixel of landslide within the whole study area; ΣNpix(Ni) = Number of pixel of the whole study area.
The natural logarithm is used to give negative weights when the density of the landslide is lower than normal, and positive weights when it is higher than normal. Positive weight (Wt) indicates the direct correlation between predictor factor and the occurrence of landslides and also the presence of predictors in landslides. The weight value for each class of causative factors was calculated using the aforementioned equation. To prepare a map of landslide susceptibility, the landslide susceptibility index (LSI) was calculated for each pixel by summing the weighted values of each factor class, as shown below.
(4)
(4)
Here, i = weights of landslide conditioning factors.
2.4. Analytic hierarchy process
The analytical hierarchy process (AHP) is a decision-making tool that assists in the resolution of complex problems using simple criteria (Panchal and Shrivastava Citation2021). The problem is divided into hierarchical criteria in AHP. These criteria are weighed against one another. The elements’ weights are assigned based on the expert’s assessment, and the weight values range from 1 to 9 (Saaty Citation1980; Saaty and Vargas Citation2001, Saaty Citation2005; Sharma and Mahajan Citation2018). In this study already published work is taken as the expert assessment (Mirdda et al. Citation2020). Weights are assigned to landslide factors and different groups within each factor in the landslide analysis. A rating of 9 indicates exceptional success from one factor to the next, whereas a rating of 1 indicates the same value for both. Typically, the decision-maker’s preference defines the distribution of values for similar trigger variables (Mirdda et al. Citation2020). In this study, ratings based on expert assessment and field observation are both subjective and objective. The Saaty scale determines the priority of each class (). The rankings are calculated using the eigen vector method, and the consistency of the solution is checked using the consistency ratio (CitationSaaty1987). The equation (EquationEquation 5(5)
(5) & EquationEquation 6
(6)
(6) ) below can be used to check the consistency of the weights for relative importance assigned during the pairwise comparison.
(5)
(5)
where λ max = largest eigenvalue of the matrix, n = order of the matrix
(6)
(6)
Table 4. Scale for pairwise comparison (Saaty Citation1995).
where RI = random index (average of consistency index
The random index (RI) varies with the matrices order. Inconsistency is accepted up to 10% (CR 0.1). If the CR is greater than 10%, the factor rating and subjective value judgement must be revised. The revision may involve reassessing factors and their classes. shows Saaty’s random index value. From Equationequation 7(7)
(7) , landslide susceptibility index (LSI) is measured.
(7)
(7)
where fw = weight of factor, cw = weight of classes within each factor.
Table 5. Random index values based on matrix size (Saaty Citation1980).
2.5. Validation of the model
Receiver Operating Characteristic (ROC) Curve: The ROC curve shows how the probability of a true positive rate varies when compared to the probability of a false positive rate. This is a type of cross-validation used to ensure the work’s authenticity and validity. The two distinct data sets are used to test the model’s performance in the sample (Martha Citation2011). The training data (70% sample) were used for the success rate curve and the testing data (30% sample) is used for the prediction rate curve. The success rate curve obtained by plotting the cumulative landslide area against the cumulative total study area. The prediction rate curve is the relationship between the cumulative percentage region of non-modelled landslides and the cumulative percentage region of the study area. These curves provide coherence in landslide susceptibility evaluation (Sarkar et al. Citation2013).
2.6. Landslide conditioning factors
2.6.1. Slope aspect
The direction of the maximum slope with respect to magnetic north is indicated by the slope aspect. The aspect of the slope has an impact on erosion, evapotranspiration, desertification, solar heating, and soil water retention (Khan et al. Citation2019). Most researchers use this slope dimension to map landslide vulnerability. The slope map is extracted from ALOS PALSAR DEM of 12.5 m resolution which is further resampled to 5 m resolution to maintain the uniformity with the other layers. The slope aspect is divided into ten classes ().
2.6.2. Slope gradient
The rate of change in elevation with respect to a horizontal surface is known as slope gradient. Slopes fail when downward shear stresses exceed the shear strength of soil or rock mass. Thus, slope stability is directly affected by slope angle (Regmi et al. Citation2014). The probability of the slope failure increases with increasing slope gradient (Panchal and Shrivastava Citation2021). The slope map is extracted from ALOS PALSAR DEM of 12.5 m resolution which is further resampled to 5 m resolution. The slope gradient map is divided into 5 class ().
2.6.3. Curvature
Slope curvature is a widely used conditioning factor for the landslide susceptibility mapping (Pourghasemi et al. Citation2013). Curvature is another DEM-based derivative that may impact slope stress and groundwater distribution. The slope curvature regulates water inflow and outflow and plays an important role in slope failures (Ramesh and Anbazhagan Citation2015). Curvature is prepared from DEM and is divided into three classes, viz. concave, flat and convex ().
2.6.4. Drainage density
The drainage density is defined as the total stream length of all orders in stream eroded topography (Demir et al. Citation2013). The inclusion of drainage density is required because it causes mass wasting and slope failure. In the study area, the drainage density is divided into five classes ().
2.6.5. Lithology
Because lithological units have varying landslide susceptibility values, they are vital to be considered. The resistance of rocks to weathering and erosion is dependent on their lithology, it is an important factor when assessing landslide susceptibility (Singh and KumarCitation2018). The map is used at a scale of 1:25000. There are six classes of lithology based on published work and field survey (Rawat Citation2015; GSI Citation2016; Biswakarma et al. Citation2020). These are Permafrost Area, Central Crystalline, Everest Pelite, Tourmaline Granite, Lingtse Gneiss and Daling Group ().
2.6.6. Land use and land cover (LULC)
LULC is one of important landslide conditioning factors used by almost all the researchers in landslide susceptibility mapping. Landslides are favoured by changes in land-use patterns, such as agricultural expansion and construction, as well as deforestation, which disrupts and changes the environment (Jaiswal et al. Citation2010). Resourcesat-2A LISS-4 multispectral (2019) bands 2, 3, and 4 were used along with ground-truth testing to plan land use/land cover classification. For land use/land cover class in the study area, supervised classification techniques were used to make interpretations. 50 training samples for each class were used for the supervised classification. A hybrid classification method (both supervised and unsupervised) was further used to address the cumulative errors. To categorise the land use/land cover class, a Google Earth map and an NRSC-prepared LULC map were used as a reference. Seven distinct land use/land cover classes were identified in the study area. Snow/Glaciers, Barren Land/Rock, Waterbodies, Fallow Land, Built-up, Agriculture/Plantation, and Forest Cover are the mapped classes ().
2.6.7. Normalized difference vegetation index (NDVI)
The NDVI is calculated using the Equationequation 8(8)
(8) .
(8)
(8)
where, NIR is the near infrared band and R is the red band of the electromagnetic spectrum (Fiorucci et al. Citation2019). The value of NDVI ranges between −1 to +1, where −1 indicates portion with no vegetation and +1 with the dense vegetation. ERDAS IMAGINE® software was used for this stage, which generated a matrix of real (floating point) numbers in the range between −0.73 and + 1.0 for the image (). The vegetation index uses variations in plant, soil, and water reflective properties that reflect different wavelengths of visible and non-visible light to carry out the calculation. This study utilized this variation in reflective properties to effectively differentiate between green plants and non-plant objects (Ni et al. Citation2019).
2.6.8. Elevation
Land elevation is one of the parameters which affect landslide risk and the researchers use it frequently for landslide susceptibility mapping (YilmazCitation2010; Park Citation2011; Yalcin et al. Citation2011; Du et al. Citation2017; Kose and Turk Citation2019). The elevation is related to other variables, such as rainfall, types of soil, types of vegetation, and coverage of vegetation, which affect stability on the slope. In this study, the elevation is divided into ten classes, with 269 m as the lowest elevation and 7047 m as the highest elevation (). Elevation is prepared from ALOS PALSAR DEM and is divided into ten classes.
2.6.9. Lineament density
Lineament features in hilly terrain indicate joints, fractures, faults, and other structural features of weakness and thus directly relate to the occurrence of landslides. Geological structures such as fractures, faults, and cleavages of rock have a significant role to play in building up pore pressure (Ramakrishnan et al. Citation2013).There are a number of active landslides in the fault zones and major lineaments in the area. The lineament map was prepared using the Bhuvan portal of National Remote Sensing Centre, India (https://bhuvanapp1.nrsc.gov.in/thematic/thematic/index.php) and Resourcesat-2A LISS 4 satellite data (). For the present study, ten classes are prepared in lineament density.
2.6.10. Geomorphology
To create a geomorphic map, visual analysis of Resourcesat-2A LISS 4 (2019) data was conducted at a scale of 1:25,000. The reference map for preparing the geomorphology map was adopted from published work (Anusuya 2017). The area of study is divided into ten geomorphological units such as, Very High Mountain; Narrow Valley; Ridge; Perpetual Snow/Glacier; Escarpment; Low Mountain; High Mountain; Rocky Cliff; Less High Mountain and Mid Mountain ().
2.6.11. Soil texture
Both topography and rock types govern the soil texture. The soils, with minor erosion in the fluvial valleys, are moderately thick, well-drained, fine loamy soils with the loamy surface. The soils on the cliffside are very soft, extremely drained, while the loamy surface soils on the tops and ridges are relatively shallow, overly drained, coarsely loamy (Rawat Citation2015).Five different classes of soil are demarcated in the study area, which is Fine loamy; Coarse loamy; Loamy skeletal; Fine silty and Snow-covered (). The soil data has been prepared from the Soil Resource map obtained from NBSS & LUP, India (2000).
2.7. Preliminary qualitative risk assessment w.r.t population and building
For as long as there have been slopes and landslides, there has also been a need for some kind of risk assessment and management. The more conventional adaptations of risk assessment and management concepts, carried out in a qualitative manner, have been put into effect for landslide risk zoning for the purpose of urban planning and highway slope management for several decades (Hungr et al. Citation2005). The capacity of resources to sustain damage as a result of the landslide potential has been taken into consideration as the landslide risk, and the mapping of these risk potentials has been assigned the title of landslide risk map (Banshtu et al. Citation2020). In order to perform an initial assessment of the landslide hazard zonation in the study region, the landslide susceptibility map is essential. In order to determine the landslide hazard zone, it is necessary to identify the places that are susceptible to landslides and to perform a calculation that estimates the likelihood of a landslide occurring within a specific time frame. Due to the fact that it is usually difficult to demarcate this time span, the landslide hazard is frequently represented by landslide susceptibility (BrabbCitation1984; Banshtu et al. Citation2020). Landslide risk is calculated with the following Equationequation 9(9)
(9) as per Hungr et al. Citation2005:
Where hazard is the spatial and temporal probability, vulnerability is the degree of loss and exposure is the elements at risk like population, buildings, infrastructures, etc. However, for the current study the different version of the landslide risk is used as per Banshtu et al. Citation2020, which is given as follows. This is done as per the available data to assess the preliminary qualitative risk w.r.t. to population and building
Where landslide potential is represented by the landslide susceptibility map prepared using eleven conditioning factors mentioned previously, whereas the risk damage potential is represented by population density map and the building density map. The current study is more of a qualitative study rather than the quantitative study; therefore, the quantitative estimation of risk is not mentioned.
2.8. Population density
The population is the most exposed and affected element in any kind of disaster. Therefore, to assess the population density and the preparation of population density map is essential for the disaster risk study. The population data was collected using the census data from the Government of Sikkim (Citation2022). The population of the West Sikkim district according to the 2011 census is 1,36,435. The data was also cross validated with the population density data of “The Gridded Population of World Version 4” (NASA). The data collected were further plotted in the GIS platform to produce the population density map ().
2.9. Building density
The building data was prepared through the indexing method using normalized difference building index (NDBI). The data was further validated using the building data collected from the Bhukosh, GSI (Govt. of India). The satellite data which was used to analyse the NDBI is the Sentinel 2 data which has the spectral resolution of 10 m further enhanced to 5 m after orthorectification and resampling. NDBI uses the NIR and SWIR bands of the electromagnetic spectrum to differentiate the built-up structure from the other structures.
(9)
(9)
The ratio generally rectifies the terrain illumination differences and the atmospheric effects to get the desired values. The values of NDBI generally ranges from −1 to 1. The negative values generally indicate the non-built class whereas the values close to 1 are confirmative of built class. Keeping this relation in mind, the result obtained from NDBI were classified into 5 classes using the natural breaks in the GIS software. The highest values close to 1 were further extracted as the building class ().
3. Results
3.1. Frequency ratio
The weights of the sub-factors of the landslide conditioning factors based on the frequency ratio method are given in the . The south facing slopes are gaining the maximum FR weights in the study area. South-east, south and south-west facing slopes have obtained the weights 1.76, 1.61 and 1.15 respectively. South-east slope covers 10% among the subclass which is around 18% landslide area among the ten sub-class. On the contrast north facing slope are having the lowest FR weights, where north, north-west and north-east have FR weights of 0.24, 0.33 and 0.45 respectively. Slope gradient above 40° have obtained the higher weights. The highest value is obtained for sub-class 45°-86° followed by 40-45° sub-class and the values are 1.92 and 1.17 respectively. The sub-class 45°-86° covers an area of 14% but landslide area in the class is 28%, which shows that this class has maximum landslide probability. The lowest value for slope gradient is obtained for 15-25° sub-classes. For the curvature the highest weight of 1.01 is obtained for convex curvature, followed by concave curvature (FR = 1). The flat curvature has the lowest weight. In the drainage density the highest weight is obtained for the sub-class 0-1 sq. km followed by 1-2 sq. km and 4-8 sq. km. The weights are 1.8, 0.8 and 0.68 respectively. The lowest weight is obtained for sub-class 2-3 sq. km. 3500-4000 m elevation is showing the highest FR weight of 3.77 followed by 3000-3500 m sub-class with a weight of 3.74. The lowest weight for elevation is given by class above 4500 m. For the LULC class the highest weight is given by built-up sub-class with a weight of 5.17 followed by fallow land with a weight of 1.42. The lowest weight in this class is given by sub-class barren rock/land. NDVI class is showing highest weight for sub-class 0.2−0.4 with a weight value of 3.47. The lowest weight of 0.06 is obtained for sub-class −0.73-0. For the lithology, Everest pelite is showing the maximum value of 3.48 followed by Daling group with 1.08. Permafrost area has the lowest value. In the geomorphology section High Mountain class has the maximum value of 5.27 followed by Narrow valley and Rocky cliff with values 2.01 and 1.77 respectively. The lowest value is obtained for Perpetual snow/glacier and Very High Mountain. Coarse loamy soil type has the maximum value of 1.57 followed by Loamy skeletal with value of 0.997. Snow covered sub-class has the minimum value. For the lineament density class, the highest value is obtained for 0–150 subclass and the lowest value is obtained for 1400–1600 sub-class. The graphical representation in the shows the weights obtained by different conditioning factors and their classes.
Figure 7. Graphical representation of weights (FR) from different conditioning factors A) aspect, B) slope, C) curvature, D) drainage density, E) lithology, F) LULC, G) NDVI, H) elevation, I) lineament density, J) geomorphology, K) soil type.

Table 6. Weights obtained from the probabilistic methods (FR & IV).
3.2. Information value method
The weights of the different classes of the landslide conditioning factors based on the information value method are given in the . The weights obtained from the information value method are following the similar trend as the frequency ratio method. The southing facing slopes have higher values as compared to the other classes in the slope aspect. The highest value is obtained for the south-east, south and south-west slope with values 0.57, 0.47 and 0.14 respectively. The lowest values are obtained for northerly facing slopes. The slope gradient with 40° have shown the positive value for the information value weight. This shows the positive correlation towards the occurrence of landslide. The highest valueclasses are 45–86° and 40–45° with values 0.66 and 0.16 respectively. The lowest value for the slope gradient is obtained for the 20–25° class with value of −0.43. Convex curvature is more vulnerable to landslides with highest information value weight of 0.015, followed by concave curvature with weight of 0.0011 and the lowest value is obtained for flat curvature. For the drainage density, the highest value is obtained for the class 0–1 sq. km and the lowest value is obtained for 2–3 sq. km sub-class. In the elevation factor the highest value is obtained for the sub-class 3500–4000 m followed by 3000–3500 m sub-class. The lowest value in this category is given by the sub-class 4000–4500 m. Built-up, Fallow land and Forest cover are showing the positive correlation IV weight of 1.64, 0.35 and 0.31 respectively. Waterbody class in this factor has attained a minimum value of −4.9, which indicates the minimal probability of landslide in the class. The vegetation index is showing the maximum value for the sub-class 0.2−0.4 followed by 0−0.2 with values 1.24 and 0.118 respectively. The sub-classes −0.73-0 and 0.6−1 for NDVI have shown the minimum and maximum values respectively for IV method. Lithology is an important factor for consideration while doing the landslide susceptibility analysis. Everest Pelite and Daling Group have shown more positive correlation the towards the occurrence of landslide having IV weights 1.25 and 0.081 respectively. Lingtse Gneiss is this factor class have the lowest value with weight of −0.25. High Mountain sub-class in the geomorphology factor have the highest value followed by Narrow Valley, Rocky Cliffs and Escarpment having weights 0.70, 0.57 and 0.24 respectively. The lowest value for the geomorphology is obtained for the classes Low Mountain and Mid Mountain respectively. According to IV weight the highest correlation for the occurrence of landslide in soil type class is obtained for the Coarse loamy soil with weight of 0.45. The minimum weight in this category is obtained for Fine loamy soil with a weight of −0.82. In the lineament density parameter, the only sub-class with positive correlation for the occurrence of landslides is 0-150. All other sub-classes in the lineament density are showing negative correlation with a minimum value of −1.59 for the sub-class 1400-1600. The graphical representation in the shows the weights obtained by different conditioning factors and their classes.
3.3. Analytic hierarchy process (AHP)
The weights of the classes of the landslide conditioning factors based on the AHP method are given in the . The weights of overall conditioning factors using AHP study is shown in the . The weights obtained in this method are mainly based on heuristic approach, there results obtained by this method may vary with probabilistic method. In the slope aspect the highest value is obtained for the north-west and the west sub-class followed by the southerly sub-class. The lowest value of 0.018 is obtained for the flat aspect. The highest weight in the slope gradient is obtained for the sub-class 45–86°, followed by 40–45° sub-class with AHP weight of 0.31 and 0.2 respectively. The class has the lowest weight with 0.015 weightage. In the curvature the highest weightage is obtained for convex curvature, followed by the flat curvature and concave curvature having the weightage 0.63, 0.3 and 0.06 respectively. For the drainage density the highest value is obtained for 4–8 sq. km followed by 3-4 sq. km having the weightage 0.57 and 0.22 respectively. The lowest weight for drainage density is obtained for the sub-class 0–1 sq. km with a weight of 0.03. For the elevation the highest weight is obtained for 5500–7047 m class, followed by 4500–5500 m with weights 0.28 and 0.2 respectively. The lowest weight of 0.017 is obtained for sub-class 269–1000 m. The LULC is important parameter and the highest value is obtained for built-up class followed by fallow land with weight value of 0.31 and 0.2 respectively. The lowest value in the LULC class is obtained for the Snow/Glaciers class having the weight 0.028. The highest weight in the vegetation index is obtained for 0.6−1 class, followed by 0.4−0.6 with the weightages 0.445 and 0.279 respectively. The lowest value for vegetation index is given by sub-class −0.73-0 with value of 0.035. For the lithology the highest value is obtained for the Daling Group followed by LingtseGniess and the lowest value is obtained for the Permafrost area. The weights of Daling group, LingtseGniess and Permafrost area 0.39, 0.26 and 0.024 respectively. In the geomorphology section the maximum value is obtained for the High Mountain, Narrow Valley and Rocky cliff with values 0.198, 0.155 and 0.144 respectively. The lowest value in this section is given by weights 0.017 for Perpetual Snow/Glacier sub-class. The coarse loamy soil type has the maximum impact for the landslide occurrence followed by loamy skeletal soil type. The snow-covered area in the soil type class has the lowest value. The values of coarse loamy, loamy skeletal and snow-covered area are 0.557, 0.2 and 0.03 respectively. The highest weight for the lineament density is obtained for the 1800-2004 sub-class having the weight value of 0.29. The lowest value in this section is given by 0-150 sub-class with a value of 0.015. The graphs show the different weights obtained from the AHP method ().
Figure 9. Graphical representation of weights (AHP) from different conditioning factors A) aspect, B) slope, C) curvature, D) drainage density, E) lithology, F) LULC, G) NDVI, H) elevation, I) lineament density, J) geomorphology, K) soil type.

Table 7. Weights obtained for different class using AHP method.
Table 8. Weights obtained for conditioning factors using AHP method.
These normalised weights are further used to prepare the landslide susceptibility map. The landslide susceptibility index based on the weighted value of all the three methods (FR, IV and AHP) were determined. The weight of the calculated values has been depicted in and for FR, IV and AHP respectively. There is always a question mark while preparing the landslide susceptibility map, therefore it is recommended to go for comparative methods and choose the best method. As already discussed above, in this paper probabilistic and the heuristic methods are applied to make a comparative study for the production of landslide susceptibility mapping.
3.4. Validation of the model
Validation is required to determine the comparative assessment of the techniques used for landslide susceptibility zonation. Receiver operating characteristic(ROC) curve is used for the current study to determine the validity of the methods used. The receiver operating characteristic (ROC) curve is a useful tool for evaluating the performance of any classification scheme, and it was used to validate all the methods used in this study, including IV, FR, and AHP. As the cut-off probability changes, the ROC curve plots the probability of true positive identified landslides versus false positive identified landslides (Martha Citation2011). ROC is also showing a relation between the sensitivity and the specificity. The ROC curve for all the methods is shown in the . The value of the ROC is 77%, 74% and 57% for FR, IV and AHP methods respectively. These values are well beyond the acceptable limit and hence shows that the methods applied for the current study are having good accuracy for all the methods. The result of the susceptibility map was validated using the success rate curve (SRC) and the prediction rate curve (PRC) for the methods. The 70% training samples were used to prepare the SRC and the remaing 30% testing samples were used to prepare the PRC. All the methods inferred that SRC showing good match with PRC (). This suggests that the techniques and factors used in the study are capable of accurately predicting the spatial occurence of landslides.
3.5. Preliminary qualitative risk assessment w.r.t population and building
For the landslide risk the best of the three susceptibility models used in the study were considered. In the current study, the highest accuracy was obtained for the frequency ratio model, hence susceptibility map prepared from FR model was used as landslide potential (LP) factor. For the risk damage potential (RDP) the two important exposing factors were chosen as already discussed earlier. The factors are population and building which are most affected by the landslide disaster. The population density per sq. km in the current study varies from 10 to 3293 (). The areas with the higher density are more risk prone which is obviously understood. Similarly, the building density from the current analysisranges up to 190 building per sq. km irrespective of the sizes of the building (). Here in this study, we have preferred the numbers of building rather than their size. The mapped building was also validated with the building data of GSI. 40 building data were validated from the GSI and were obtained a 100% accuracy for these data (). The final risk map prepared was classified into three zones as the high-risk zone, medium risk zone and the low-risk zone. From the present study it is obtained that 83% area falls under the low-risk zone, 14% falls under the medium risk zone and the 3% falls under the high-risk zone.
4. Discussion
Preparation of landslide susceptibility map in the mountainous terrain is always a challenging task, as the selection of landslide conditioning factors is another challenge due to the thick forest cover leading to moist soil, slope hiding under natural shade, inaccessibility of high elevation and many other factors in the mountainous terrain (Sharma Citation2018). Considering all these factors, high-resolution remote sensing data (LISS-4) and ancillary data for the application of statistical models (FR and IV) and knowledge driven methods have been used for the generation of LSM for the present study area. Main causative factors were selected and used for the assessment of landslide susceptibility based on landslide polygons prepared and high-resolution remote sensing image interpretation. The main purpose of this study is to compare the probabilistic and the heuristic methods for the preparation of landslide susceptibility mapping.
A relation between the slope aspect and the slope failures were established using the IV and FR model, which infers that southerly facing slopes are more prone to landslides. Previous research done in the Himalayan terrain w.r.t slope aspect also reveals the same interpretation (Sarkar et al. Citation2013, CitationMartha 2011). This indicates the probability of occurrence of landslides in these slopes is maximum. Because the Himalayan south-facing slopes receive sunlight throughout the day, there are more anthropogenic activities (Selvakumar et al. Citation2009; Rai et al. Citation2014; Bhardwaj et al. Citation2021). As anthropogenic activities increase, the slope becomes more vulnerable. The south west monsoon winds that travel west along the southern slopes of the Himalaya bring more rainfall to the southern slopes, which is also one of the major reasons for these failures (Fu et al. Citation2018). However, in case of AHP the maximum weights are obtained for north-west facing slopes followed by the south facing slopes, showing coherence with the probabilistic methods. The probable reason for north facing slopes contributing higher value is that the right bank of the main stream is dominant towards north (Bera et al. Citation2019).
In terms of slope gradient, it is always challenging to establish a perfect relationship between the slope angle and the slope failures. Previous research in Darjeeling Himalayas, however, shows that debris slides in the lower slope and rockslides typically occurred along steeper slopes (Kanungo and SarkarCitation2003; Ghosh et al. Citation2009). Nevertheless, in the present study, failures were not categorized, but the overall failures were considered, which shows that slopes with the gradient >40° are very vulnerable as per the ratings obtained from all the three models used here. This correlation supports the hypothesis that steeper slopes have a higher downhill component of force, causing gravitational sheer stress on gradient components and slope failure (Dai et al. 2001; Chapin et al. Citation2011). It is also found that the slope gradient influences the shear force, so with the increase of the slope gradient, the probability of the slope failures increases (Lee and Min Citation2001). Apart from this the component responsible for slope failure are also the cohesion of the slope material, angle of repose and the moisture condition (Selby 1993).
The curvature of the slope controls the inflow and outflow of water. Studies also reveal that curvature of a slope influences the moisture content dependent on saturation and erosion (Singh and Kumar Citation2018). Ridge sites had a positive curvature of the slope, which represented an upwardly convex surface. Places on the shallow and valley floor had a negative curvature, the surface is concave upwards (Fan et al. Citation2020). In the present study, it is observed that the convex slope are more susceptible to landslides followed by concave surface and flat surface are least susceptible and the same can be compared with the research done by other researchers (Gnyawali et al. Citation2020). Convex hill slopes, where seepage forces are directed upward from the steep, lower section of the slope, have the highest failure potentials in saturated homogeneous materials (Reid and Iverson Citation1992).
In general, the area with the high drainage density is more susceptible to slope failures (Singh and Kumar Citation2018), but in the present study, the negative correlation is seen for drainage density for the probabilistic method.Wang et al. Citation2003 also found in his research that low drainage density is most likely for landslide. He also mentioned that surface runoff is reduced by the high infiltration capacity, which erodes the ground surface and forms a gully. However, for the heuristic method, the positive corelation is seen showing higher weightage for the higher density value. The area with the lowest drainage is showing the highest weighted value. This means that local terrain influences are more important than drainage density alone. The other reason for this negative correlation is that the lowest class consists of the highest pixel values, i.e. it covers the maximum area, so probability increases. That is why it is always important to consider the multiple factors for these mappings.
It is generally found that the landslides in the Daling Group of rocks of Sikkim Himalaya are comparatively high (Sarkar et al. Citation2013). This group mainly contains interbedded chlorite-sericite schist/phyllite, feldspathic greywacke, biotite phyllite/mica schist, biotite quartzite, mica schist, variegated cherty phyllite, chert etc.However,in all the methods more weights were obtained for Everest Pelite. Everest Pelite Formation consists of PhyIlite, quartzite, biotite gneiss (Rawat Citation2015). The phyllitic, quartzite and gneissic lithological units in Everest Pelite has a high landslide density because it weathers quickly and produces less cohesive soil (Sarkar et al. Citation2013).All these rocks types have weak planes and get oversaturated with water during the continuous rainfall. As a result, these rocks have the highest probability of slope failures.
The human interference has accelerated the landslide incidence in the study area as supported by the results obtained from all the methods. In the Land use and land cover category, the Built-Up area shows the highest weighted value rating, followed by Fallow land and Forest cover. The other classes show a negative correlation. The highest rating in the Built-Up area is because of the unplanned and unscientific construction in vulnerable slopes (Jesiya and Gopinath Citation2019). The higher value obtained for the fallowlandas obtained in the current study can be attributed to the inherent properties of the LULC class (Anbalagan et al. Citation2015). On the other hand, the positive correlation on the forest cover is that according to predictive models, the area under forest cover is better correlated with landslides, which highlights the role of high precipitation in the Himalayan region (Sharma Citation2018).
NDVI suggests the characteristics of vegetation production, and it mainly changes the hydrological and soil shear strength characteristics of slope soil (Hong et al. Citation2017). The Normalized Difference Vegetation Index (NDVI) is one of the most important indicators used to determine vegetation cover over a wide range of time periods and in specific areas by employing remote sensing technology (Aburas et al. Citation2015). NDVI is the indexing that gives the idea about the vegetation cover in an area with the values ranging from −1 to +1, where +1 has the highest vegetation cover. The negative value generally indicates the cloud or snow cover. Above 0.3, the vegetation cover increases, and between 0.1to 0.3 generally represents the barren land, and zero value is for water bodies (Gandhi et al. Citation2015). The probabilistic analysis shows the positive corelation of NDVI with the occurrence of slope failures. So, in this scenario, the weighted value obtained shows a positive correlation. However, the heuristic approach is showing the negative correlation.
The elevation in the study area varies from 269 m to above 7000 m, but the more susceptible class, according to probabilistic method, are the classes where the elevation is ranging from 3500-4000 m and 4000-4500 m. The elevation class, mainly catchment areas with high surface runoff, tends to be more landslide-prone (Du et al. Citation2019). The spatial relation between landslides and elevation classes suggests that slope failures in the study region occurred mainly in locations with an elevation 3500-4500 m, often catchment areas with high surface runoff, which tend to be more landslide-prone. This is the reason why these two classes showing high weighted value, as the area with the elevation ranging from 3500-4500 m contains mostly catchment areas. Studies also reveal that in the hilly terrains, moderate elevations are more prone and susceptible to slope failures (Kose and Turk Citation2019). For the AHP method the higher values are shown for the higher elevation. Nakileza and Nedala (Citation2020), in their research found similar results as of AHP method.
Lineaments of any types such as joints, foliation in rocks decrease resistance, material cohesion, and make surface water to percussion and it is the explanation for the high probability of failure in the area of structural discontinuity (Sarkar et al. Citation2013). A lineament has a minimal impact zone on the rock power relative to a thrust that has a broader region of impact (Martha et al. Citation2013). In the present study, lineament features include all the linear features, be it a joint or thrust. Both the geomorphic and the structural features taken together for the lineament density. This is the reason why the present study is showing the negative correlation with increasing lineament density for the probabilistic method. However, for the heuristic method the increasing lineament density is showing positive corelation with the occurrence of landslides.
Geomorphologically, the folded ridge and the highly dissected mountain regions have the highest potential for landslide occurrence. The hilly and mountainous regions are significantly more susceptible to landslides than plain and plateau regions (Roy et al. Citation2019). The same has been observed in the current study for all the methods used, with the highest weighted value in High Mountain followed by Narrow Valley, Rocky Cliff, and Escarpment. The reason for showing the high value in the Narrow Valley is that Narrow Valley contains steep sides, which makes the area susceptible to landslides.
The soil texture is strongly associated with the probability of a landslide incidence (Basu and Pal Citation2018). All soils fail under the effect of shear, where shear is a mixture of coherence and internal stress (Shooshpasha and Shirvani Citation2015). Cohesion decreases with an increase in sand content and increases with the clay content. Therefore, any mass of debris or siliceous sandy soil with poor cohesion is likely to fail when saturated on steep slopes (Bell Citation2013). Research also suggests that coarse-loamy, gravel-loamy, and loamy-skeletal soil texture classes indicate a higher risk of landslide occurrence compared to other soil texture classes (Roy et al. Citation2019). For the present study, the Coarse-loamy soil type shows the highest weighted value indicating the highly susceptible class in terms of landslide occurrence. Coarse loamy soil also contains a high percentage of sand as compared to clay content. Therefore, in the study area, steep slopes of over 40° occur frequent landslides consisting of soils with predominant sand fractions.
In the study the different comparisons have been made for the preparation of the susceptibility map keeping in the mind that both the statistical method (probabilistic) and the knowledge driven method (heuristic) are taken into consideration. The maps produced by using different methods for preparing landslide susceptibility map are shown in the . The high accuracy in the probabilistic method is indicated of the unbiased in the analysis, however there can be some biasness in giving the ranking for heuristic methods. A comparatively less value in the ROC for AHP method might be the result of the biasness in the rating process. Therefore it is suggested to compare our results with the statistical method. Comparative study is very crucial when we have to prepare landslide risk zonation map, because the susceptibility map produced will be the landslide potential factor for the landslide risk zonation map. For the current study as the highest accuracy was obtained for the FR method, therefore the landslide susceptibility map obtained form it is considered for risk zonation. On the other hand, many researchers have used building and population as the risk damage potential for calculation of risk zonation map. Though the quantification is not done in the current study for the risk but it gives a very good qualitative risk zonation of the study area. Susceptibility map should not be the ultimate goal of the study. There can always be risk element associated with the susceptibility study. It is also very clear from the susceptibility map and the risk map that when the risk damage potential is associated, the outcome is different and the same is very obvious in the risk zonation map ().
5. Conclusions
The present study basically dealt with landslide susceptibility map using various methods, including the frequency ratio (FR) method and information value (IV) method for a probabilistic approach, and the analytic hierarchy process (AHP) for a heuristic approach. All methods performed well within acceptable accuracy limits during validation. The ultimate objective was to create a landslide preliminary qualitative risk zonation for the study area. The FR method, which yielded the best fit landslide susceptibility model (LSM), was used for the risk map. The accuracy, as measured by the receiver operating characteristic curve, was 77% for FR, 74% for IV, and 57% for AHP. The susceptibility maps were categorized into three classes—high, medium, and low susceptibility—using natural breaks. All three models indicated that land use/land cover (LULC), lithology, and geomorphology factors had the highest influence on landslide susceptibility. Specifically, the built-up class had the highest weightage among LULC, Everest Pelite among lithology, and high mountain class among geomorphology. Such studies also aid in prioritizing causative factors. After susceptibility mapping, the preparation of the risk zonation was crucial. Consistent with previous research, population and buildings were considered as indicators of risk and damage potential, given their exposure and vulnerability. The building map, created using the normalized difference built-up index (NDBI) and validated against GSI data, showed good accuracy. The resulting risk zonation map was divided into three zones: high-risk, medium-risk, and low-risk. The findings revealed that 3% of the study area fell under the high-risk zone, 14% under the medium-risk zone, and the remaining 83% under the low-risk zone (). This type of research is highly valuable, particularly for planners, policymakers, and stakeholders involved in the area’s planning and development.
Compliance with ethical standards
Disclosure of potential conflicts of interest
Ethical approval
This article does not contain any studies with human participants or animals performed by any of the authors.
Contributions
P.B. and V.J.- Writing Original Draft, Conceptualization, Formal analysis, Investigation, Methodology, Software, Visualization, Review & Editing; I.R.C -Review & Editing, H.G.A.- Funding allocation, Review & Editing H.A., A.A.A., and M.M. - Review & Editing. All authors have read and agreed to the published version of the manuscript
Acknowledgements
PB is thankful to Guru Gobind Singh Indraprastha University, India for financial support through Indraprastha Research Fellowship vide F.No. GGSIPU/DRC/2019/1683. All the authors are thankful to the Dean, USEM, Guru Gobind Singh Indraprastha University, India for providing the facility in carrying out the research work.The authors are thankful to the editors and potential reviewers.
Disclosure statement
No potential conflict of interest was reported by the author(s).
Data availability statement
The data that support the findings of this study are available on request from the corresponding author.
Additional information
Funding
References
- Aburas MM, Abdullah SH, Ramli MF, Ash’aari ZH. 2015. Measuring land cover change in Seremban, Malaysia using NDVI index. Proc Environ Sci. 30:238–243. https://cyberleninka.org/article/n/586987/viewer. doi: 10.1016/j.proenv.2015.10.043.
- Acharyya SK. 1989. The Daling Group, its nomenclature, tectono-stratigraphy and structural grain: with notes on their possible equivalents. VisesaPrakasana-BharatiyaBhuvaijñanikaSarveksana. 22:5–13.
- Achour Y, Boumezbeur A, Hadji R, Chouabbi A, Cavaleiro V, Bendaoud EA. 2017. Landslide susceptibility mapping using analytic hierarchy process and information value methods along a highway road section in Constantine, Algeria. Arab J Geosci. 10(8):1–16. doi: 10.1007/s12517-017-2980-6.
- AGS (Australian Geomechanics Society Sub-Committee on Landslide Risk Management). 2000. Landslide risk management concepts and guidelines. Australian Geomech J. 35:49–92. https://eagcg.org/common/pdf/LRM2000-Concepts.pdf.
- Akbari A, Yahaya FBM, Azamirad M, Fanodi M. 2014. Landslide susceptibility mapping using logistic regression analysis and GIS tools. Electr J Geotech Engin. 19:1687–1696.
- Althuwaynee OF, Pradhan B. 2017. Semi-quantitative landslide risk assessment using GIS-based exposure analysis in Kuala Lumpur City. Geomatics, Nat Hazards and Risk. 8(2):706–732. doi: 10.1080/19475705.2016.1255670.
- Anbalagan R, Kumar R, Lakshmanan K, Parida S, Neethu S. 2015. Landslide hazard zonation mapping using frequency ratio and fuzzy logic approach, a case study of Lachung Valley, Sikkim. Geoenviron Disasters. 2(1):1–17. doi: 10.1186/s40677-014-0009-y.
- Anusuya D. 2015. Quaternary geomorphological landforms on the Southeast facing slopes of Kanchenjunga around Dzongri in West Sikkim [PhD thesis]. Visva Bharti University. http://hdl.handle.net/10603/234251.
- Arabameri A, Pradhan B, Rezaei K, Lee CW. 2019. “Assessment of landslide susceptibility using statistical-and artificial intelligence-based FR–RF integrated model and multiresolution DEMs. Remote Sens. 11(9):999. doi: 10.3390/rs11090999.
- Atlas Magazine. 2020. [accessed 2022 Dec 29]. https://www.atlas-mag.net/en/article/natural-disasters-risk-in-india.
- Auden JB. 1935. Traverses in the Himalaya. Records of Geol Survey of India. 69:123–167.
- Ayalew L, Yamagishi H. 2005. The application of GIS-based logistic regression for landslide susceptibility mapping in the Kakuda-Yahiko Mountains, Central Japan. Geomorphology. 65(1–2):15–31. doi: 10.1016/j.geomorph.2004.06.010.
- Ayalew L, Yamagishi H, Marui H, Kanno T. 2005. Landslides in Sado Island of Japan: part II. GIS-based susceptibility mapping with comparisons of results from two methods and verifications. Engin Geol. 81(4):432–445. doi: 10.1016/j.enggeo.2005.08.004.
- Banerjee P, Ghose MK, Pradhan R. 2018. Analytic hierarchy process and information value method-based landslide susceptibility mapping and vehicle vulnerability assessment along a highway in Sikkim Himalaya. Arab J Geosci. 11(7):1–18. doi: 10.1007/s12517-018-3488-4.
- Banshtu RS, Versain LD, Pandey DD. 2020. Risk assessment using quantitative approach: Central Himalaya, Kullu, Himachal Pradesh, India. Arab J Geosci. 13(5):219. doi: 10.1007/s12517-020-5143-0.
- Basu SK. 2013. “Geology of Sikkim State.” http://14.139.206.50:8080/jspui/bitstream/1/4375/1/Geology%20of%20Sikkim%20State.pdf.
- Basu T, Pal S. 2018. Identification of landslide susceptibility zones in Gish River basin, West Bengal, India. Georisk: Assessment and Manage Risk for Engineered Syst Geohazards. 12(1):14–28. doi: 10.1080/17499518.2017.1343482.
- Bell FG. 2013. Engineering properties of soils and rocks. Butterworth-Heinemann, Elsevier.
- Bera A, Mukhopadhyay BP, Das D. 2019. Landslide hazard zonation mapping using multi-criteria analysis with the help of GIS techniques: a case study from Eastern Himalayas, Namchi, South Sikkim. Nat Hazards. 96(2):935–959. doi: 10.1007/s11069-019-03580-w.
- Bhardwaj DR, Tahiry H, Sharma P, Pala NA, Kumar D, Kumar A, Bharti B. 2021. Influence of aspect and elevational gradient on vegetation pattern, tree characteristics and ecosystem carbon density in northwestern Himalayas. Land. 10(11):1109. doi: 10.3390/land10111109.
- Biswakarma P, Joshi V, Kumar K. 2020. Study of Slope Failures in and around Yuksom, the First Capital of Sikkim, India- A case study. Environ & We an Int J Sci Technol. 15(1):39–48. http://www.sedindia.in/ewijst/issues/vol15/ewijst1501042019023.pdf.
- Bose PN. 1891. Notes on the geology and mineral resources of Sikkim. Rec Geol Surv India. 24(4):217–230.
- Brabb EE. 1984. Innovative approaches to landslide hazard and risk mapping. In Proceedings of the 4th International Symposium on Landslides.” Canadian Geotechnical Society, Ed.; Canadian Geotechnical Society: Toronto, ON, Canada, 1, p. 307–324.
- Cao C, Wang Q, Chen J, Ruan Y, Zheng L, Song S, Niu C. 2016. Landslide susceptibility mapping in vertical distribution law of precipitation area: case of the Xulong Hydropower Station Reservoir, Southwestern China. Water. 8(7):270. doi: 10.3390/w8070270.
- Carrara A, Guzzetti F, Cardinali M, Reichenbach P. 1999. Use of GIS technology in the prediction and monitoring of landslide hazard. Natural Hazards. 20(2/3):117–135. doi: 10.1023/A:1008097111310.
- Castellanos AEA. 2008. Multi-scale landslide risk assessment in Cuba [PhD]. thesis number 154, University of Utrecht. ISBN 978-90-6164-268-8.
- Chalkias C, Kalogirou S, Ferentinou M. 2014. Landslide susceptibility, Peloponnese Peninsula in South Greece. J Maps. 10(2):211–222. doi: 10.1080/17445647.2014.884022.
- Chapin FS, Matson PA, Vitousek PM. 2011. The ecosystem concept. In: Principles of terrestrial ecosystem ecology. New York, NY: Springer; p. 3–22. doi: 10.1007/978-1-4419-9504-9_1.
- Chen W, Li W, Hou E, Zhao Z, Deng N, Bai H, Wang D. 2014. Landslide susceptibility mapping based on GIS and information value model for the Chencang District of Baoji, China. Arab J Geosci. 7(11):4499–4511. doi: 10.1007/s12517-014-1369-z.
- Clerici A, Perego S, Tellini C, Vescovi P. 2010. Landslide failure and runout susceptibility in the upper T. Ceno valley (Northern Apennines, Italy). Nat Hazards. 52(1):1–29. doi: 10.1007/s11069-009-9349-4.
- Cruden DM. 1991. A simple definition of a landslide. Bull Int Assoc Engin Geol-Bulletin de l'Association Internationale de Géologie de l'Ingénieur. 43(1):27–29. doi: 10.1007/BF02590167.
- Dai K, Deng J, Xu Q, Li Z, Shi X, Hancock C, Wen N, Zhang L, Zhuo G. 2022. Interpretation and sensitivity analysis of the InSAR line of sight displacements in landslide measurements. GIScience& Remote Sens. 59(1):1226–1242. doi: 10.1080/15481603.2022.2100054.
- Demir G, Aytekin M, Akgün A, İkizler SB, Tatar O. 2013. A comparison of landslide susceptibility mapping of the eastern part of the North Anatolian Fault Zone (Turkey) by likelihood-frequency ratio and analytic hierarchy process methods. Nat Hazards. 65(3):1481–1506. doi: 10.1007/s11069-012-0418-8.
- Dikshit A, Sarkar R, Pradhan B, Segoni S, Alamri AM. 2020. Rainfall induced landslide studies in Indian Himalayan region: a critical review. Appl Sci. 10(7):2466. doi: 10.3390/app10072466.
- Du G, Zhang Y, Iqbal J, Yang Z, Yao X. 2017. Landslide susceptibility mapping using an integrated model of information value method and logistic regression in the Bailongjiang watershed, Gansu Province, China. J Mt Sci. 14(2):249–268. doi: 10.1007/s11629-016-4126-9.
- Du G, Zhang Y, Yang Z, Guo C, Yao X, Sun D. 2019. Landslide susceptibility mapping in the region of eastern Himalayan syntaxis, Tibetan Plateau, China: a comparison between analytical hierarchy process information value and logistic regression-information value methods. Bull Eng Geol Environ. 78(6):4201–4215. doi: 10.1007/s10064-018-1393-4.
- Fan B, Tao W, Qin G, Hopkins I, Zhang Y, Wang Q, Lin H, Guo L. 2020. Soil micro-climate variation in relation to slope aspect, position, and curvature in a forested catchment. Agricultural and Forest Meteorol. 290:107999. doi: 10.1016/j.agrformet.2020.107999.
- Fell R, Corominas J, Bonnard C, Cascini L, Leroi E, Savage WZ. 2008. Guidelines for landslide susceptibility, hazard and risk zoning for land use planning. Engin Geol. 102(3–4):85–98. doi: 10.1016/j.enggeo.2008.03.022.
- Fiorucci F, Ardizzone F, Mondini AC, Viero A, Guzzetti F. 2019. Visual interpretation of stereoscopic NDVI satellite images to map rainfall-induced landslides. Landslides. 16(1):165–174. doi: 10.1007/s10346-018-1069-y.
- Foumelis M, Lekkas E, Parcharidis I. 2018. Landslide susceptibility mapping by GIS-based qualitative weighting procedure in Corinth area. Geosociety. 36(2):904. doi: 10.12681/bgsg.16840.
- Froude MJ, Petley DN. 2018. Global fatal landslide occurrence from 2004 to 2016. Nat Hazards Earth Syst Sci. 18(8):2161–2181. doi: 10.5194/nhess-18-2161-2018.
- Fu Y, Pan X, Xian T, Liu G, Zhong L, Liu Q, Li R, Wang Y, Ma M. 2018. Precipitation characteristics over the steep slope of the Himalayas in rainy season observed by TRMM PR and VIRS. Clim Dyn. 51(5–6):1971–1989. doi: 10.1007/s00382-017-3992-3.
- Gandhi GM, Parthiban B, Thummalu N, Christy A. 2015. Ndvi: vegetation change detection using remote sensing and GIS–A case study of Vellore District. Proc Computer Sci. 57:1199–1210. doi: 10.1016/j.procs.2015.07.415.
- Ghosh S, van Westen CJ, Carranza EJM, Ghoshal TB, Sarkar NK, Surendranath M. 2009. A quantitative approach for improving the BIS (Indian) method of medium-scale landslide susceptibility. J Geol Soc India. 74(5):625–638. doi: 10.1007/s12594-009-0167-9.
- Ghosh S. 2011. “Knowledge guided empirical prediction of landslide hazard.” Enschede, Netherlands: ITC. https://webapps.itc.utwente.nl/librarywww/papers_2011/phd/ghosh.pdf.
- Gnyawali KR, Zhang Y, Wang G, Miao L, Pradhan AMS, Adhikari BR, Xiao L. 2020. Mapping the susceptibility of rainfall and earthquake triggered landslides along China–Nepal highways. Bull Eng Geol Environ. 79(2):587–601. doi: 10.1007/s10064-019-01583-2.
- Government of Sikkim. 2022. [accessed 2022 December 29]. https://sikkim.gov.in/departments/forest-environment-and-wildlife-department/biodiversity.
- GSI. 2014. Final report on study of lingtse granite gneiss in Sikkim Himalaya for elucidating the role of proterozoic granitoids in Himalayan orogeny. Geological Survey of India.
- GSI. 2016. Final report on the specialised thematic mapping in the area between Dentam and Yuksom in parts of West and South Districts, Sikkim. Geological Survey of India.
- Guzzetti F, Carrara A, Cardinali M, Reichenbach P. 1999. Landslide hazard evaluation: a review of current techniques and their application in a multi-scale study, Central Italy. Geomorphology. 31(1–4):181–216. doi: 10.1016/S0169-555X(99)00078-1.
- Hazra P, Krishna AP. 2019. Spatio-temporal and surface elevation change assessment of glaciers of Sikkim Himalaya (India) across different size classes using geospatial techniques. Environ Earth Sci. 78(14):1–20. doi: 10.1007/s12665-019-8390-1.
- Hong H, Ilia I, Tsangaratos P, Chen W, Xu C. 2017. A hybrid fuzzy weight of evidence method in landslide susceptibility analysis on the Wuyuan area, China. Geomorphology. 290:1–16. doi: 10.1016/j.geomorph.2017.04.002.
- Hosenuzzaman M, Kibria MG, Sarkar R, Abedin MA. 2022. Landslide, agricultural vulnerability, and community initiatives: a case study in South-East part of Bangladesh. In: Impact of climate change, land use and land cover, and socio-economic dynamics on landslides. Singapore: Springer; p. 123–145.
- Hoyois P, Scheuren JM, Below R, Guha-Sapir D. 2007. Annual disaster statistical review: numbers and trends 2006. Centre for Research on the Epidemiology of Disasters (CRED) School of Public Health, Catholic University of Louvain Brussels, Belgium.
- Hungr O, Fell R, Couture R, Eberhardt E. 2005. Landslide risk management. 1st ed. London: CRC Press. doi: 10.1201/9781439833711.
- Jaiswal P, van Westen CJ, Jetten V. 2010. Quantitative landslide hazard assessment along a transportation corridor in southern India. Engin Geol. 116(3–4):236–250. doi: 10.1016/j.enggeo.2010.09.005.
- Jaiswal P, van Westen CJ, Jetten V. 2011. Quantitative assessment of landslide hazard along transportation lines using historical records. Landslides. 8(3):279–291. doi: 10.1007/s10346-011-0252-1.
- Jaiswal P. 2011. Landslide risk quantification along transportation corridors based on historical information. Enschede, Netherlands: ITC.
- Jesiya NP, Gopinath G. 2019. A Customized Fuzzy AHP-GIS based DRASTIC-L model for intrinsic groundwater vulnerability assessment of urban and peri urban phreatic aquifer clusters. Groundwater for Sustainable Devel. 8:654–666. doi: 10.1016/j.gsd.2019.03.005.
- Kanungo DP, Sarkar S. 2003. Landslides and terrain parameters in Darjeeling Himalaya. Himalayan Geol. 24(2):55–62.
- Kayastha P, Dhital MR, De Smedt F. 2013. Application of the analytical hierarchy process (AHP) for landslide susceptibility mapping: a case study from the Tinauwatershed, West Nepal. Computers and Geosci. 52:398–408. doi: 10.1016/j.cageo.2012.11.003.
- Khan H, Shafique M, Khan MA, Bacha MA, Shah SU, Calligaris C. 2019. Landslide susceptibility assessment using Frequency Ratio, a case study of northern Pakistan. Egyptian J Remote Sens and Space Sci. 22(1):11–24. doi: 10.1016/j.ejrs.2018.03.004.
- Kose DD, Turk T. 2019. GIS-based fully automatic landslide susceptibility analysis by weight-of-evidence and frequency ratio methods. Phys Geograph. 40(5):481–501. doi: 10.1080/02723646.2018.1559583.
- Kouli M, Loupasakis C, Soupios P, Vallianatos F. 2010. Landslide hazard zonation in high-risk areas of Rethymno Prefecture, Crete Island, Greece. Nat Hazards. 52(3):599–621. doi: 10.1007/s11069-009-9403-2.
- Lee J, Li X, Xu ZW, Dai CF. 2001. Assessment of landslide susceptibility on the natural terrain of Lantau Island, Hong Kong. Environ Geol. 40(3):381–391. doi: 10.1007/s002540000163.
- Lee S, Min K. 2001. Statistical analysis of landslide susceptibility at Yongin, Korea. Environ Geol. 40(9):1095–1113. doi: 10.1007/s002540100310.
- Lee S, Pradhan B. 2006. Probabilistic landslide hazards and risk mapping on Penang Island, Malaysia. J Earth Syst Sci. 115(6):661–672. doi: 10.1007/s12040-006-0004-0.
- Li Y, Chen G, Han Z, Zheng L, Zhang F. 2014. A hybrid automatic thresholding approach using panchromatic imagery for rapid mapping of landslides. GISci Remote Sens. 51(6):710–730. doi: 10.1080/15481603.2014.972867.
- Luo X, Lin F, Zhu S, Yu M, Zhang Z, Meng L, Peng J. 2019. Mine landslide susceptibility assessment using IVM, ANN and SVM models considering the contribution of affecting factors. PLoS One. 14(4):e0215134. doi: 10.1371/journal.pone.0215134.
- Magliulo P, Di Lisio A, Russo F, Zelano A. 2008. Geomorphology and landslide susceptibility assessment using GIS and bivariate statistics: a case study in southern Italy. Nat Hazards. 47(3):411–435. doi: 10.1007/s11069-008-9230-x.
- Mandal SP, Chakrabarty A, Maity P. 2018. Comparative evaluation of information value and frequency ratio in landslide susceptibility analysis along national highways of Sikkim Himalaya. Spat Inf Res. 26(2):127–141. doi: 10.1007/s41324-017-0160-0.
- Martha TR, van Westen CJ, Kerle N, Jetten V, Kumar VK. 2013. Landslide hazard and risk assessment using semi-automatically created landslide inventories. Geomorphology. 184:139–150. doi: 10.1016/j.geomorph.2012.12.001.
- Martha TR. 2011. Detection of landslides by object-oriented image analysis. Enschede, Netherlands: ITC. https://webapps.itc.utwente.nl/librarywww/papers_2011/phd/martha.pdf.
- Mirdda HA, Bera S, Siddiqui MA, Singh B. 2020. Analysis of bi-variate statistical and multi-criteria decision-making models in landslide susceptibility mapping in lower Mandakini Valley, India. GeoJournal. 85(3):681–701. doi: 10.1007/s10708-019-09991-3.
- Mishra M, Sarkar T. 2020. A multistage hybrid model for landslide risk mapping: tested in and around Mussoorie in Uttarakhand state of India. Environ Earth Sci. 79(19):1–24. doi: 10.1007/s12665-020-09180-3.
- Nadim F, Kjekstad O, Peduzzi P, Herold C, Jaedicke C. 2006. Global landslide and avalanche hotspots. Landslides. 3(2):159–173. doi: 10.1007/s10346-006-0036-1.
- Nakileza BR, Nedala S. 2020. Topographic influence on landslides characteristics and implication for risk management in upper Manafwa catchment, Mt Elgon Uganda. Geoenviron Disasters. 7(1):1–13. doi: 10.1186/s40677-020-00160-0.
- Ni Z, Yang Z, Li W, Zhao Y, He Z. 2019. Decreasing trend of geohazards induced by the 2008 Wenchuan earthquake inferred from time series NDVI data. Remote Sens. 11(19):2192. doi: 10.3390/rs11192192.
- Nor Diana MI, Muhamad N, Taha MR, Osman A, Alam MM. 2021. Social vulnerability assessment for landslide hazards in Malaysia: a systematic review study. Land. 10(3):315. doi: 10.3390/land10030315.
- Panchal S, Sangwan S, Usman M. 2015. A review of techniques of landslide susceptibility mapping using GIS. Int J Engin Sci Res Technol. 4(2):142–145.
- Panchal S, Shrivastava AK. 2021. A comparative study of frequency ratio, Shannon’s entropy and analytic hierarchy process (AHP) models for landslide susceptibility assessment. IJGI. 10(9):603. doi: 10.3390/ijgi10090603.
- Park NW. 2011. Application of Dempster-Shafer theory of evidence to GIS-based landslide susceptibility analysis. Environ Earth Sci. 62(2):367–376. doi: 10.1007/s12665-010-0531-5.
- Pellicani R, van Westen CJ, Spilotro G. 2014. Assessing landslide exposure in areas with limited landslide information. Landslides. 11(3):463–480. doi: 10.1007/s10346-013-0386-4.
- Pourghasemi HR, Pradhan B, Gokceoglu C, Mohammadi M, Moradi HR. 2013. Application of weights-of-evidence and certainty factor models and their comparison in landslide susceptibility mapping at Haraz watershed, Iran. Arab J Geosci. 6(7):2351–2365. doi: 10.1007/s12517-012-0532-7.
- Pradhan B, Youssef AM. 2010. Manifestation of remote sensing data and GIS on landslide hazard analysis using spatial-based statistical models. Arab J Geosci. 3(3):319–326. doi: 10.1007/s12517-009-0089-2.
- Rai PK, Mohan K, Kumra VK. 2014. Landslide hazard and its mapping using remote sensing and GIS. J Sci Res. 58:1–13.
- Ramakrishnan D, Singh TN, Verma AK, Gulati A, Tiwari KC. 2013. Soft computing and GIS for landslide susceptibility assessment in Tawaghat area, Kumaon Himalaya, India. Nat Hazards. 65(1):315–330. doi: 10.1007/s11069-012-0365-4.
- Ramesh V, Anbazhagan S. 2015. Landslide susceptibility mapping along Kolli hills Ghat road section (India) using frequency ratio, relative effect and fuzzy logic models. Environ Earth Sci. 73(12):8009–8021. doi: 10.1007/s12665-014-3954-6.
- Rawat MS. 2015. Geo-environmental studies in A part of East Sikkim with special reference to landslide. Dept. of Geology, HNB Garhwal University, India [PhD Thesis].
- Ray KK. 1976. Some problems of stratigraphy and tectonics of the Darjeeling and Sikkim Himalayas. Geol Surv Ind Misc Pub. 24:279–394.
- Regmi AD, Yoshida K, Pourghasemi HR, Dhital MR, Pradhan B. 2014. Landslide susceptibility mapping along Bhalubang—Shiwapur area of mid-Western Nepal using frequency ratio and conditional probability models. J Mt Sci. 11(5):1266–1285. doi: 10.1007/s11629-013-2847-6.
- Reid ME, Iverson RM. 1992. Gravity‐driven groundwater flow and slope failure potential: 2. Effects of slope morphology, material properties, and hydraulic heterogeneity. Water Resources Res. 28(3):939–950. doi: 10.1029/91WR02695.
- Roy J, Saha S, Arabameri A, Blaschk T, Bui DT. 2019. A novel ensemble approach for Landslide Susceptibility Mapping (LSM) in Darjeeling and Kalimpong Districts, West Bengal, India. Remote Sens. 11(23):2886. doi: 10.3390/rs11232866.
- Rozos D, Bathrellos GD, Skillodimou HD. 2011. Comparison of the implementation of rock engineering system and analytic hierarchy process methods, upon landslide susceptibility mapping, using GIS: a case study from the Eastern Achaia County of Peloponnesus, GREECE. Environ Earth Sci. 63(1):49–63. doi: 10.1007/s12665-010-0687-z.
- Saaty RW. 1987. The analytic hierarchy process—what it is and how it is used. Mathematical Modelling. 9(3–5):161–176. doi: 10.1016/0270-0255(87)90473-8.
- Saaty TL, Vargas LG. 2001. Models, methods, concepts and application of analytical hierarchy process. Boston: Kluwer; p. 333.
- Saaty TL. 1980. The analytic hierarchy process. New York: McGrawHill.
- Saaty TL. 1995. Transport planning with multiple criteria: the analytic hierarchy process applications and progress review. ATR. 29(1):81–126. doi: 10.1002/atr.5670290109.
- Saaty TL. 2005. Theory and application of the analytic network process. Pittsburg: RWS.
- Sarkar S, Roy AK, Martha TR. 2013. Landslide susceptibility assessment using information value method in parts of the Darjeeling Himalayas. J Geol Soc India. 82(4):351–362. doi: 10.1007/s12594-013-0162-z.
- Selvakumar G, Joshi P, Mishra PK, Bisht JK, Gupta HS. 2009. Mountain aspect influences the genetic clustering of psychrotolerant phosphate solubilizing Pseudomonads in the Uttarakhand Himalayas. Curr Microbiol. 59(4):432–438. doi: 10.1007/s00284-009-9456-1.
- Sharma A, Gupta M, Sharma N. 2023. Glacier facies characterisation in transboundary West Sikkim Himalaya from TerraSAR-X; GLCM based classification approach. J Spatial Sci. 1–17. doi: 10.1080/14498596.2022.2164085.
- Sharma S, Mahajan AK. 2018. Comparative evaluation of GIS-based landslide susceptibility mapping using statistical and heuristic approach for Dharamshala region of Kangra Valley, India. Geoenviron Disasters. 5(1):4. doi: 10.1186/s40677-018-0097-1.
- Sharma S, Mahajan AK. 2018. A comparative assessment of information value, frequency ratio and analytical hierarchy process models for landslide susceptibility mapping of a Himalayan watershed, India. Bull Eng Geol Environ. 78(4):2431–2448. doi: 10.1007/s10064-018-1259-9.
- Shooshpasha I, Shirvani RA. 2015. Effect of cement stabilization on geotechnical properties of sandy soils. Geomech Eng. 8(1):17–31. doi: 10.12989/gae.2015.8.1.017.
- Singh A, Pal S, Kanungo DP, Pareek N. 2017. An overview of recent developments in landslide vulnerability assessment- presentation of a new conceptual framework. In: Mikoš M, Arbanas Ž, Yin Y, Sassa K, editors. Advancing culture of living with landslides. Advances in landslide science. New York: Springer; vol. 2; p. 795–802. doi: 10.1007/978-3-319-53498-5_91.
- Singh A, Pal S, Kanungo DP. 2021. An integrated approach for landslide susceptibility–vulnerability–risk assessment of building infrastructures in hilly regions of India. Environ Dev Sustain. 23(4):5058–5095. doi: 10.1007/s10668-020-00804-z.
- Singh K, Kumar V. 2017. Landslide hazard mapping along national highway-154A in Himachal Pradesh, India using information value and frequency ratio. Arab J Geosci. 10(24):1–18. doi: 10.1007/s12517-017-3315-3.
- Singh K, Kumar V. 2018. Hazard assessment of landslide disaster using information value method and analytical hierarchy process in highly tectonic Chamba region in bosom of Himalaya. J Mt Sci. 15(4):808–824. doi: 10.1007/s11629-017-4634-2.
- Singh R, Umrao RK, Singh TN. 2014. Stability evaluation of road-cut slopes in the Lesser Himalaya of Uttarakhand, India: conventional and numerical approaches. Bull Eng Geol Environ. 73(3):845–857. doi: 10.1007/s10064-013-0532-1.
- SSDMA. 2022. [accessed 2022 Apr 25]. http://www.ssdma.nic.in/Uploads/PdfFiles/multi_hazard_risk_and_vulnerability_assessment_of_sikkim.pdf.
- Sui H, Hu R, Gao W, Gao W, Luo G. 2020. Risk assessment of individual landslide based on the risk acceptable model: a case study of the Shiyantan landslide in Mayang County, China. Human and Ecol Risk Assessment: An Int J. 26(9):2500–2519. doi: 10.1080/10807039.2019.1710461.
- Tsangaratos P, Ilia I, Hong H, Chen W, Xu C. 2017. Applying information theory and GIS-based quantitative methods to produce landslide susceptibility maps in Nancheng County, China. Landslides. 14(3):1091–1111. doi: 10.1007/s10346-016-0769-4.
- Van Westen CJ, Van Asch TW, Soeters R. 2006. Landslide hazard and risk zonation—Why is it still so difficult? Bull Eng Geol Environ. 65(2):167–184. doi: 10.1007/s10064-005-0023-0.
- Varnes DJ. 1984. “Landslide hazard zonation: a review of principles and practice” (No. 3). Natural Hazards, Publisher: United Nations
- Vranken L, Vantilt G, Van Den Eeckhaut M, Vandekerckhove L, Poesen J. 2015. Landslide risk assessment in a densely populated hilly area. Landslides. 12(4):787–798. doi: 10.1007/s10346-014-0506-9.
- Wang WN, Chigira M, Furuya T. 2003. Geological and geomorphological precursors of the Chiu-fen-erh-shan landslide triggered by the Chi-chi earthquake in central Taiwan. Engineering Geol. 69(1–2):1–13. doi: 10.1016/S0013-7952(02)00244-2.
- Yalcin A, Reis S, Aydinoglu AC, Yomralioglu T. 2011. A GIS-based comparative study of frequency ratio, analytical hierarchy process, bivariate statistics and logistics regression methods for landslide susceptibility mapping in Trabzon, NE Turkey. Catena. 85(3):274–287. doi: 10.1016/j.catena.2011.01.014.
- Yalcin A. 2008. GIS-based landslide susceptibility mapping using analytical hierarchy process and bivariate statistics in Ardesen (Turkey): comparisons of results and confirmations. Catena. 72(1):1–12. doi: 10.1016/j.catena.2007.01.003.
- Yilmaz I. 2010. Comparison of landslide susceptibility mapping methodologies for Koyulhisar, Turkey: conditional probability, logistic regression, artificial neural networks, and support vector machine. Environ Earth Sci. 61(4):821–836. doi: 10.1007/s12665-009-0394-9.
- Zhong C, Liu Y, Gao P, Chen W, Li H, Hou Y, Nuremanguli T, Ma H. 2020. Landslide mapping with remote sensing: Challenges and opportunities. Int J Remote Sens. 41(4):1555–1581. doi: 10.1080/01431161.2019.1672904.
- Zillman J. 1999. The physical impact of disaster. In: Natural Disaster Manage. Leicester: Tudor Rose Holdings Ltd. Leicestr; 320.