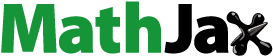
Abstract
A significant number of ionic rare earth tailings slopes were generated during the process of rare earth mining and the factor of safety of these slopes continued to decrease under the action of rainfall. This study focussed on a specific tailings slope located in Dayu County, Ganzhou City. Through the utilization of MatDEM numerical simulation software, the variations in infiltration distribution and destabilization evolution of the tailings slope between different seasons were comparatively examined. The results indicated that during the rainy season (May to June), increased rainfall led to increased rate of water infiltration, consequently reducing the stability of the slope and exacerbating landslide damage. Furthermore, the study provided analyses of safety distances under different seasons of rainfall, taking into account the geographical location of the tailings slope in Dayu County, Ganzhou City, and the results of the analyses provided theoretical foundation into ensuring the safety of buildings and residents downstream of the slope.
1. Introduction
China has a significant amount of rare earth resources (Wübbeke Citation2013), which accounts for approximately 23% of the world’s total reserves. Ionic rare earth resources are primarily found in southern mountainous areas such as Ganzhou City, Jiangxi Province and Longyan City, Fujian Province (Central People’s Government of the People’s Republic of China Citation2018). In pursuit of higher profit returns, some private companies have accumulated a large amount of tailings sand during the mining process, further increasing the potential risk of local tailings landslides (Nguyen et al. Citation2019; Ongpaporn et al. Citation2022). These companies use technical processes such as heap leaching and pond leaching, resulting in a relatively low output ratio of rare earths, with one tonne of rare earths generating more than 2,000 tonnes of tailing sand (Central People’s Government of the People’s Republic of China Citation2012). Because the heavy metals of tailing sand are excessive, tailing sand can only be stockpiled centrally (Yin et al. Citation2016). In recent years, with the government’s emphasis on protecting arable land, the pile of tailing sand is getting higher and higher (Central People’s Government of the People’s Republic of China Citation2017). In the case of continuous rainfall, the slopes are prone to sudden environmental pollution and landslides (Ran et al. Citation2018; Senthilkumar et al. Citation2018). Dayu County has several tailings, including one located in Zuo Ba town, as shown in below. Downstream of the tailings, there are residential houses, farmland, roads, equipment, and factories. During the rainy season, there is a significant risk of tailings landslides that could endanger the safety of the residents.
There are many factors that affect the stability of slopes, such as the type of rainfall, strength reduction factor, cracks and duration of rainfall (Komolvilas et al. Citation2021; Yuan et al. Citation2021; Kafle et al. Citation2022; Yamusa et al. Citation2022). Wu et al. (Citation2022) analyzed the relationship between the degree of saturation and rainfall intensity based on different types of rainfall intensity and discovered that the higher the rainfall intensity, the faster the change in the degree of saturation. Luo et al. (Citation2021) found a negative correlation between rainfall duration and safety factor by changing the duration of artificial rainfall. This relationship may be attributed to the depth of infiltration, where greater depth corresponds to lower safety factor for the slope (Nguyen et al. Citation2017). Cracksas a key factor influencing water infiltration (Chen et al. Citation2018), have a strong adsorption effect on water (Wang et al. Citation2022; Zhang et al. Citation2023), and water stored in cracks reduces the shear strength of slopes (Liu et al. Citation2005; Miao et al. Citation2022). Moreover, landslide susceptibility mapping plays a vital role in analyzing landslide stability. For instance, Miao et al. (Citation2023) proposed a high-precision boosting-C5.0 machine learning model, which can serve as a foundation for geological hazard risk management and control.
2. Methods and materials
In this paper, the analysis related to tailings landslides was mainly done through numerical simulation, as shown in . Before simulating landslides, the macromechanical parameters of discrete units were set according to the actual physical properties of tailing sand, and the infiltration process under different rainfall conditions was calculated using MatDEM based on Darcy’s law (Whitaker Citation1986). During the process, saturation increased with increasing water content and exceeded the saturation threshold for water content, which was around 30% (Gu et al. Citation2022). As saturation continued to increase, the connections between discrete units in the cracks began to break, forming unstable areas along the cracks. This ultimately led to the occurrence of tailings landslides.
2.1. Mechanical analysis of discrete unit connection
In MatDEM, discrete units are connected to each other by springs and generate the normal force () and shear force (
) (Liu Citation2021). Based on the Mohr-Coulomb criterion, the maximum shear force (
) that the springs can withstand can be determined, and a damage criterion for discrete units can be established. When the shear force (
) exceeds the maximum shear force (
), or normal displacement (
) is less than 0 or greater than or equal to the fracture displacement (
), the tangential or normal springs will break, resulting in the disconnection of discrete units, as represented by the following equations.
(1)
(1)
(2)
(2)
(3)
(3)
where
is the shear resistance between discrete units, and
is the friction coefficient between discrete units.
is the normal stiffness,
is the tangential stiffness, and
is the tangential displacement.
2.2. Materials of the discrete units
In stacking models, the stacking and cementation of discrete units change their mechanical parameters, and the values of compressive strength, tensile strength, and modulus of elasticity of the materials are also affected by the phenomenon of "size effect" (Zhou et al. Citation2016). In MatDEM, the macroscopic mechanical parameters of discrete units are usually smaller than the actual values, e.g. Young’s modulus() is usually 20% to 40% of the actual values, and compressive strengths (
) and tensile strengths (
) are 10% to 20% of the actual values (Liu et al. Citation2017).
In this simulation, the Young’s modulus of discrete units was 36% of the actual values, and the compressive and tensile strengths were 18% of the actual values. Since MatDEM is currently unable to adjust the Poisson’s ratio and the coefficient of internal friction of the material, the Poisson’s ratio and the coefficient of internal friction
of discrete units were kept the same as the actual values. The relevant mechanical parameters are shown in below.
Table 1. Mechanical parameters of real materials and discrete units.
In addition, there are conversion equations between the macroscopic mechanical parameters and the micromechanical parameters, which can be used to obtain the five micromechanical parameters of discrete units, as shown in the following :
Table 2. The microscopic mechanical parameters of discrete units.
The five conversion formulas:
(4)
(4)
(5)
(5)
(6)
(6)
(7)
(7)
(8)
(8)
(9)
(9)
where
is the diameter of discrete units and
is the scaling factor.
2.3. Moisture diffusion model
Based on Darcy’s law, the water infiltration equation for unsaturated soil is shown below (Ross Citation1990).
(10)
(10)
where
is the water content (%),
is the soil water flux density (cm s−1),
is the unsaturated hydraulic conductivity (cm s−1),
is the water pressure head (cm),
is the horizontal distance (cm) and
is the time of infiltration (s).
In this numerical simulation, MatDEM implemented the infiltration process of water moving from the upper boundary to the lower boundary by selecting the path with the shortest distance for water infiltration. The equation governing the motion of water during vertical infiltration is as follows (Wang et al. Citation2018).
(11)
(11)
where
is the boundary,
is the initial moisture content (%),
is the water diffusivity,
is the vertical distance (cm) and
is the shape parameter of the soil pore distribution. In addition, according to the two most common B–C and V–G models (Kosugi Citation1994), the specific expressions for the nonlinear term
and the hydraulic conductivity of unsaturated soil
are obtained.
B–C model:
(12)
(12)
V–G model:
(13)
(13)
where
is the residual moisture content (%),
is the saturation moisture content (%), and
is the saturated hydraulic conductivity (cm s−1).
(B–C model),
(V–G model),
is the parameter of the shape of the characteristic soil moisture curve.
The slope was divided into two parts, the regular and irregular areas, based on the boundary conditions of the numerical simulation. The area to the left of the dividing line represents the regular area, where water infiltrates vertically. The corresponding equation is stated below.
(14)
(14)
The area to the right of the zoning line represented the irregular area, where water infiltrated into the slope’s interior. The corresponding equation is stated below.
(15)
(15)
2.4. Rainfall analysis
Rainfall is one of the main causes of landslides disasters (Kuradusenge et al. Citation2020; Ahmed Citation2021), and rainfall is directly related to seasonal changes (Li et al. Citation2016). Warm and humid air currents from the Pacific Ocean pass through several provinces in southern China, resulting in a 2-month-long period of rain known as the rainy season. In addition, the time of occurrence of the rainy varies from region to region. Based on meteorological data from Weather Atlas (Weather Atlas Citation2002–2023), the average rainfall data for Ganzhou city were compiled. As depicted in , the rainy season in Ganzhou occurred between May and June each year. Among these, the average rainfall during the non-rainy season was 73.4 mm, while the average rainfall during the rainy season reached 210 mm, which was approximately 2.86 times higher than the average rainfall during the non-rainy months. Prolonged rainfall led to the accumulation of rainwater on the slope surface and increased the saturation of tailing sand, thereby reducing the slope’s stability (Gasmo et al. Citation2000).
2.5. Slope stability analysis
Rainfall causes an increase in the self-weight of tailing sand, which disrupts the original mechanical equilibrium. Without considering the equilibrium of forces between the slices, the slope is divided into equally spaced slices according to Swedish slice method (Enoki et al. Citation1990), which can be used to find the integral ratio of the shear stress to the shear strength on each slice can be found., i.e. the safety factor (). The formula for calculating the stabilisation coefficient (
) is as follows.
(16)
(16)
are the cohesion and friction angle of the ith slice, respectively, and N is the number of divided slices.
and
are the bottom length, bottom angle, and weight of the ith slice, respectively. Under continuous rainfall, the self-weight of the slices increases as the saturation of tailing sand increases, but the cohesion and angle of internal friction of tailing sand decreases (Zhang and Ding Citation2012). Thus the factor of safety decreases with increasing rainfall (Raj and Sengupta Citation2014), and a larger increase in rainfall leads to a faster decrease in the safety factor, resulting in greater slope instability.
2.6. Numerical model and monitoring sites
The upper limit of the number of units in Madam is approximately 6 million (Liu et al. Citation2017–2021), Moreover, a higher number of units or smaller particle sizes of the units impose greater performance requirements on the computer. The particle size of tailing sand exhibits a relatively wide gradation, with 80% of the particles ranging from 0.05 to 0.5 mm. However, such a wide gradation can hinder simulation efficiency, necessitating a reduction in the range of tailing sand particle size. The particle size of discrete units in the model ranges from 0.028 m to 0.033 m, as shown in . In addition to the grain size, the slope angle is also a worthwhile aspect to consider in establishing the slope model. In general, the greater the slope angle, the more likely tailings landslides will occur. Slopes generally range from 30° to 60°, and in order to analyze the maximum damage caused by the tailings landslides to people and buildings, a slope with an angle of 60° was chosen for modelling. Regarding stratification, the slope was divided into tailing sand and bedrock layers. Tailing sand has a loose structure and the density of 1.48–2.56 g/cm3, and layer 2 is used to replace tailing sand in the numerical simulation. Since the radius of discrete units in layer 2 was different from that of tailing sand, the hydraulic parameter values of layer 2 were different from the actual hydraulic parameter values of tailing sand. The hydraulic parameter values of layer 2 were =0.1,
=0.9,
=5.5,
=0.71 and
=0.6. The bedrock is mainly granite, which has higher compressive and tensile strength, and the density of 2.60–2.82 g/cm3, and layer 1 was used to replace the bedrock in the numerical simulation. Layer 1 had very little effect on the slope, and it was set up mainly to have a supporting effect on the particles of the model and to prevent discrete units from flying out of the interior of the model. Therefore, the values of hydraulic parameters in layer 1 were the same as those in layer 2. In addition, in order to analyse the process of water infiltration, seven detection zones were set up, as shown in . Four vertical monitoring zones with X values of 0.2–0.3 m and Z values of 8.2–7.8, 7.2–6.8, 6.2–5.8, and 5.2–4.8 m, respectively, were used to study the vertical infiltration of water. Three horizontal monitoring zone with Z values of 3.9–4.1 m and X values of 1.5–1.7, 2.7–2.9, and 3.9–4.1 m, respectively, were used to study the horizontal infiltration of water.
3. Results
Numerical simulations of tailings landslides under rainfall were conducted in this study, and the process took approximately 36 s. Twelve MatDEM files and eight data tables were generated for analysis, containing key information such as degree of saturation, displacement, velocity, and impact force. The data were extracted, classified, filtered, and analyzed to derive the evolution patterns of the displacement field, moisture field, and impact force.
3.1. Moisture field data analysis
shows that Monitoring Point 1 reached a saturation of 78% at 15.9 s and remained relatively stable thereafter. Monitoring Point 2 began to show an increase in saturation at 5.8 s, while Monitoring Point 3 started to exhibit an increase at 17.9 s. Monitoring Point 4 experienced minimal changes in saturation. In , Monitoring Point 1 approached a saturation level of approximately 78% at 36 s. The saturation of monitoring point 2 started to increase at 7.7 s, and the degree of saturation increased to about 32% at 36 s. During different rainy seasons, there was a significant disparity in the saturation levels of Monitoring Point 2 at 36 s, with a difference in saturation of approximately 42%. The saturation of monitoring point 3 and point 4 remained essentially unchanged, indicating that there was no water infiltration into monitoring point 3 during the non-rainy season. This indicates that the depth of water infiltration was shallower than 2.5 m.
Figure 6. The variation of the degree of saturation at vertical monitoring points. (a)The rainy season; (b) the non-rainy season.

In addition to the monitoring points of the degree of saturation in the vertical direction, three horizontal monitoring points were also set up, namely monitoring point 5, monitoring point 6, and monitoring point 7. illustrates the changes in saturation levels for the horizontal monitoring points during the rainy season. At 36 s, the saturation of monitoring point 7 was 79%. The saturation of monitoring point 5 and monitoring point 6 increased from 0 s and exceeded 30% at 28.8 and 23.1 s, respectively. Due to the faster vertical infiltration of water compared to the horizontal infiltration in the cracks, the saturation of monitoring point 5 and point 6 was greater than that at monitoring point 7 before 11.4 s. However, the saturation of monitoring point 5 and point 6 was less than that of monitoring point 7 after 11.4 s, this was because the locations of monitoring point 5 and point 6 are closer to the interior of the slope than monitoring point 7. depicts the variation of saturation levels for the horizontal monitoring points during the non-rainy season. The saturation changed curves for monitoring point 5, point 6, and point 7 intersected at 20.1 s, which was delayed by 8.7 s, compared to the rainy season. The saturation of monitoring point 7 exceeded 30% at 25.4 s, which is delayed by 12.2 s, compared to the rainy season. The delay indicated that the rate of water infiltration under the non-rainy season was smaller than the rate of water infiltration under the rainy season.
3.2. Analysis of water infiltration
Rainfall is a key condition for triggering landslides (Argyriou et al. Citation2022). With continuous rainfall, water infiltrated from discrete units with high saturation into discrete units with low saturation. illustrates the temporal distribution of saturation. During the rainy season, it could be observed that water infiltrated more rapidly, reaching a depth of approximately 2 m. During the non-rainy season, the amount of water infiltration was less compared to the rainy season and the water infiltrated at a relatively slower rate to a depth of about 1.5 m. also reveals that the presence of cracks enhanced the rate of water infiltration.
3.3. Connection evolution law
The connections between discrete units in this simulation are shown in below. The green area indicates that discrete units are connected to each other, and the blue area indicates that discrete units are disconnected from each other. As water started to seep into the interior of the slope, the connections of discrete units gradually broke down in the cracks, and tailings landslides started to occur. When the saturation of discrete units in cracks to 30%, the connection began to break. Thus, the change of connection could correspond to the increase of saturation of discrete units in the cracks. In the rainy season, as shown in , the connection of crack 1 was almost completely disconnected at 9 s, the connection of crack 2 was completely disconnected at 27 s, the connection of crack 3 was partially disconnected at 36 s, and the slope underwent an overall landslide. In the non-rainy season, as shown in , the connection of crack 1 started to disconnect at 9 s and was almost completely disconnected at 18 s. The connections of crack 2 and crack 3 were partially disconnected at 36 s, and only partial landslide occurred on the slope.
3.4. Safety distance analysis
In order to analyze the safety distance more accurately, the intervals of 7–9 m, 9–11 m, 11–13 m, 13–15 m, 15–17 m, 17–19 m, and 19–21 m in the x axis were set as the spacing areas. From the start of tailings landslides until their completion, the area and impact force of discrete units in the monitoring areas were calculated at intervals of 0.1 s. These calculations are presented in and . Based on the data from , the maximum area of discrete units within each spacing area during various rainy seasons was determined, and the average height of the maximum area was calculated as the depth of inundation according to the area formula. The related research (Pollock and Wartman Citation2020) suggested that when the inundation depth ranged from 0.9 to 5.9 m, the potential harm caused by tailings landslides to individuals increases. Therefore, a critical value of 0.9 m was selected to determine the depth of inundation.
Figure 10. Area of discrete units in the spacing areas: (a) The rainy season; (b) the nonrainy season.

Figure 11. Impact force of discrete units in the spacing areas: (a)The rainy season; (b) the nonrainy season.

In addition, the maximum force of impact of discrete units in the spacing areas of different rainy seasons could be obtained from , which was useful for analysing the safety distances of buildings. Since the vast majority of buildings are nowadays reinforced concrete structures, the object of analysis in this paper was the reinforced concrete structural type of buildings. Previous research (Kang and Kim Citation2016) indicated that impact pressures exceeding 100 kPa resulted in extensive damage to buildings, while impact pressures ranging from 35 to 100 kPa led to moderate damage. Impact pressures below 35 kPa were associated with slight damage to structures. The results of the specific analysis are shown in .
Table 3. The safety distance analysis for landslides.
4. Discussion
In this paper, the processes of tailings landslides during different rainy seasons were analyzed using MatDEM. Compared with previous experiments and numerical simulations (Montrasio et al. Citation2016), the relationship between tailings landslides and rainfall amounts was investigated, and this relationship was verified through numerical simulations. Furthermore, the hazard of tailings landslides was further analyzed based on previous studies. While the study of tailings landslides was still in the preliminary stage, this study still provided a theoretical basis for local slope management.
Unfortunately, further studies on slopes with different gradients had not been carried out in this paper, resulting in limited data availability from modeling. In addition, the model only considered average monthly rainfall and did not consider the daily fluctuation of rainfall.
For the prevention of tailings landslides, an important direction would be to develop new techniques or materials for slope reinforcement (Ngo et al. Citation2019, Citation2023).
5. Conclusion
In this study, water infiltration and tailings landslides under different rainy seasons were analyzed using numerical simulations. Discrete units were constructed with specific elastic modulus and strength properties through a combination of conversion formulas for discrete units and numerical simulation experiments, enabling automated modeling of discrete units. Furthermore, additional analyses were conducted to determine the safety distances downstream of the tailings slope based on the numerical modeling results. Regarding the depth of landslide inundation, the inundation depth was less than 0.9 m within the 13–19 m during the rainy season, while in the non-rainy season, the inundation depth was less than 0.9 m across all spacing areas. In terms of building damage, extensive damage was observed in all spacing zones during the wet season. During the non-rainy season, buildings within the range of 7–11 m suffered extensive damage, those within the range of 11-15 m suffered moderate damage, and those within the range of 15–19 m suffered slight damage. The numerical simulations demonstrated differences in water infiltration and tailings landslides between the rainy season and the non-rainy season, with higher water infiltration rates and more severe damage observed during the rainy season. Therefore, special attention should be given to ensure the safety of tailings slopes during the rainy season.
Acknowledgments
The authors thank the anonymous reviewers for their helpful suggestions.
Data availability statement
Data is available on request from the authors.
Disclosure statement
No potential conflict of interest was reported by the author(s).
Additional information
Funding
References
- Ahmed B. 2021. The root causes of landslide vulnerability in Bangladesh. Landslides. 18(5):1707–1720. doi: 10.1007/s10346-020-01606-0.
- Argyriou AV, Polykretis C, Teeuw RM, Papadopoulos N. 2022. Geoinformatic analysis of rainfall-triggered landslides in Crete (Greece) based on spatial detection and hazard mapping. Sustainability. 14(7):3956. doi: 10.3390/su14073956.
- Chen X, Ma T, Li C, Liu H, Ding B, Peng W. 2018. The catastrophic 13 November 2015 rock-debris slide in Lidong, South-Western Zhejiang (China): a landslide triggered by a combination of antecedent rainfall and triggering rainfall. Geomat Nat Hazards Risk. 9(1):608–623. doi: 10.1080/19475705.2018.1455750.
- Central People’s Government of the People’s Republic of China. 2012. China’s Rare Earth Situation and Policy. Beijing: Xinhua News Agency [accessed 2023 Sept 10]. https://www.gov.cn/zhengce/2012-06/20/content_2618561.htm.
- Central People’s Government of the People’s Republic of China. 2017. Central Committee of the Communist Party of China State Council on strengthening Arable land protection and improvement of the balance of occupation and replenishment. Beijing: Xinhua News Agency [accessed 2023 Sept 10]. http://www.gov.cn/zhengce/2017-01/23/content_5162649.htm.
- Central People’s Government of the People’s Republic of China. 2018. More than ten new large and medium-sized rare earth deposits were discovered in Gannan. Beijing: Xinhua News Agency [accessed 2023 Sept 10]. http://www.gov.cn/xinwen/2018-01/14/content_5256541.htm.
- Liu C. 2017–2021. MatDEM. Nanjing: Nanjing University [accessed 2023 Sept 10]. http://matdem.com.
- Enoki M, Yagi N, Yatabe RJS. 1990. Generalized slice method for slope stability analysis. Soil Found. 30(2):1–13. doi: 10.3208/sandf1972.30.2_1.
- Gasmo J, Rahardjo H, Leong E. 2000. Infiltration effects on stability of a residual soil slope. Comput Geotech. 26(2):145–165. doi: 10.1016/S0266-352X(99)00035-X.
- Gu X, Nie W, Li Q, Geng J, Zhou T, Yuan C. 2022. Discrete element simulation of the road slope considering rainfall infiltration. Water. 14(22):3663. doi: 10.3390/w14223663.
- Kafle L, Xu W-J, Zeng S-Y, Nagel T. 2022. A numerical investigation of slope stability influenced by the combined effects of reservoir water level fluctuations and precipitation: a case study of the Bianjiazhai landslide in China. Eng Geol. 297:106508. doi: 10.1016/j.enggeo.2021.106508.
- Kang H-s, Kim Y-t 2016. The physical vulnerability of different types of building structure to debris flow events. Nat Hazards. 80(3):1475–1493. doi: 10.1007/s11069-015-2032-z.
- Komolvilas V, Tanapalungkorn W, Latcharote P, Likitlersuang S. 2021. Failure analysis on a heavy rainfall-induced landslide in Huay Khab Mountain in Northern Thailand. J Mt Sci. 18(10):2580–2596. doi: 10.1007/s11629-021-6720-8.
- Kosugi K. 1994. Three‐parameter lognormal distribution model for soil water retention. Water Resour Res. 30(4):891–901. doi: 10.1029/93WR02931.
- Kuradusenge M, Kumaran S, Zennaro M. 2020. Rainfall-induced landslide prediction using machine learning models: the case of Ngororero District, Rwanda. Int J Environ Res Public Health. 17(11):4147. doi: 10.3390/ijerph17114147.
- Li X, Zhou W, Chen YD. 2016. Detecting the origins of moisture over southeast China: seasonal variation and heavy rainfall. Adv Atmos Sci. 33(3):319–329. doi: 10.1007/s00376-015-4197-5.
- Liu B, Li S, Zhang L, Wang J. 2005. Experimental and discrete element numerical analysis of side slope instability induced by fissure water underlying impervious bed. Sci China Ser E: Technol Sci. 48(S1):65–80. doi: 10.1360/04zze20.
- Liu C. 2021. Matrix discrete element analysis of geological and geotechnical engineering. Berlin: Springer. doi: 10.1007/978-981-33-4524-9.
- Liu C, Xu Q, Shi B, Deng S, Zhu H. 2017. Mechanical properties and energy conversion of 3D close-packed lattice model for brittle rocks. Comput Geosci. 103:12–20. doi: 10.1016/j.cageo.2017.03.003.
- Luo X, Yuan J, Gu T. 2021. Study on the stability of slopes on both sides of highway in rock area under rainfall. Arab J Geosci. 14(6):1–6. doi: 10.1007/s12517-021-06857-2.
- Miao F, Zhao F, Wu Y, Li L, Török Á. 2023. Landslide susceptibility mapping in Three Gorges Reservoir area based on GIS and boosting decision tree model. Stoch Environ Res Risk Assess. 37:2283–2303. doi: 10.1007/s00477-023-02394-4.
- Miao F, Zhao F, Wu Y, Li L, Xue Y, Meng J. 2022. A novel seepage device and ring-shear test on slip zone soils of landslide in the three Gorges reservoir area. Eng Geol. 307:106779. doi: 10.1016/j.enggeo.2022.106779.
- Montrasio L, Schilirò L, Terrone A. 2016. Physical and numerical modelling of shallow landslides. Landslides. 13(5):873–883. doi: 10.1007/s10346-015-0642-x.
- Ngo TP, Likitlersuang S, Takahashi A. 2019. Performance of a geosynthetic cementitious composite mat for stabilising sandy slopes. Geosynth Int. 26(3):309–319. doi: 10.1680/jgein.19.00020.
- Ngo TP, Takahashi A, Likitlersuang S. 2023. Centrifuge modelling of a soil slope reinforced by geosynthetic cementitious composite mats. Geotech Geol Eng. 41(2):881–896. doi: 10.1007/s10706-022-02311-6.
- Nguyen TS, Likitlersuang S, Jotisankasa A. 2019. Influence of the spatial variability of the root cohesion on a slope-scale stability model: a case study of residual soil slope in Thailand. Bull Eng Geol Environ. 78(5):3337–3351. doi: 10.1007/s10064-018-1380-9.
- Nguyen TS, Likitlersuang S, Ohtsu H, Kitaoka T. 2017. Influence of the spatial variability of shear strength parameters on rainfall induced landslides: a case study of sandstone slope in Japan. Arab J Geosci. 10(16):369. doi: 10.1007/s12517-017-3158-y.
- Ongpaporn P, Jotisankasa A, Likitlersuang S. 2022. Geotechnical investigation and stability analysis of bio-engineered slope at Surat Thani Province in Southern Thailand. Bull Eng Geol Environ. 81(3):84. doi: 10.1007/s10064-022-02591-5.
- Pollock W, Wartman J. 2020. Human vulnerability to landslides. Geohealth. 4(10):e2020GH000287. doi: 10.1029/2020GH000287.
- Raj M, Sengupta A. 2014. Rain-triggered slope failure of the railway embankment at Malda, India. Acta Geotech. 9(5):789–798. doi: 10.1007/s11440-014-0345-9.
- Ran Q, Hong Y, Li W, Gao J. 2018. A modelling study of rainfall-induced shallow landslide mechanisms under different rainfall characteristics. J Hydrol. 563:790–801. doi: 10.1016/j.jhydrol.2018.06.040.
- Ross PJ. 1990. Efficient numerical methods for infiltration using Richards’ equation. Water Resour Res. 26(2):279–290. doi: 10.1029/WR026i002p00279.
- Senthilkumar V, Chandrasekaran S, Maji V. 2018. Rainfall-induced landslides: case study of the Marappalam landslide, Nilgiris District, Tamil Nadu, India. Int J Geomech. 18(9):05018006. doi: 10.1061/(ASCE)GM.1943-5622.0001218.
- Wang J, Su L, Qin X. 2018. Hermite radial basis collocation method for unsaturated soil water movement equation. Math Prob Eng. 2018:1–19. doi: 10.1155/2018/8298915.
- Wang X, Geng J, Zhang D, Xiao W, Chen Y, Zhang H. 2022. Influence of sub-supercritical CO2 on pore structure and fractal characteristics of anthracite: an experimental study. Energy. 261:125115. doi: 10.1016/j.energy.2022.125115.
- Weather Atlas. 2002–2023. [accessed 2023 Sept 10]. https://www.weather-atlas.com/zh/china/ganzhou-climate#rainfall.
- Whitaker S. 1986. Flow in porous media I: a theoretical derivation of Darcy’s law. Transp Porous Med. 1(1):3–25. doi: 10.1007/BF01036523.
- Wu Y, Yin Y, Ding Y, Zhang B, Sun X. 2022. Rainfall infiltration and water-retention characteristics of rockfill material. Int J Geomech. 22(3):04021303. doi: 10.1061/(ASCE)GM.1943-5622.0002296.
- Wübbeke J. 2013. Rare earth elements in China: policies and narratives of reinventing an industry. Resour Policy. 38(3):384–394. doi: 10.1016/j.resourpol.2013.05.005.
- Yamusa IB, Ismail MS, Tella A. 2022. Highway proneness appraisal to landslides along Taiping to Ipoh segment Malaysia, using MCDM and GIS techniques. Sustainability. 14(15):9096. doi: 10.3390/su14159096.
- Yin H, Tan N, Liu C, Wang J, Liang X, Qu M, Feng X, Qiu G, Tan W, Liu F. 2016. The associations of heavy metals with crystalline iron oxides in the polluted soils around the mining areas in Guangdong Province, China. Chemosphere. 161:181–189. doi: 10.1016/j.chemosphere.2016.07.018.
- Yuan B, Li Z, Su Z, Luo Q, Chen M, Zhao Z. 2021. Sensitivity of multistage fill slope based on finite element model. Adv Civ Eng. 2021:1–13. doi: 10.1155/2021/6622936.
- Zhang C, Wang E, Li B, Kong X, Xu J, Peng S, Chen Y. 2023. Laboratory experiments of CO2-enhanced coalbed methane recovery considering CO2 sequestration in a coal seam. Energy. 262:125473. doi: 10.1016/j.energy.2022.125473.
- Zhang X, Ding S. 2012. Effects of drying and wetting on nonlinear decay of soil shear strength in slope disintegration erosion area. Trans Chinese Soc Agric Eng. 28(5):241–245. doi: 10.3969/J.ISSN.1002-6819.2012.05.040.
- Zhou Y, Wang L, Ding J, Wu H. 2016. Particle flow code analysis of multi-scale jointed rock mass based upon equivalent rock mass technique. Rock Soil Mech. 37(7):2085–2095. doi: 10.16285/j.rsm.2016.07.033.