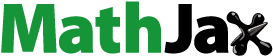
Abstract
The Sichuan Luding earthquake that struck on September 5, 2022 is one of the strongest earthquakes in China in recent years. The analysis of precipitable water vapor (PWV) retrieved from the ground-based global navigation satellite system (GNSS), surface pressure (SP), surface latent heat flux (SLHF), and land surface temperature (LST) from the reanalysis dataset was carried out in the epicenter and the nearby areas. The results show that PWV decreases distinctly and reaches the trough at the outburst with significant minimums of 43.21 mm and 37.84 mm over the nearest SCSM and SCTQ station from the epicenter. SLHF also has the same trend, and SP increased. Additionally, the LST analysis from two-temporal series was conducted to reveal that the Luding event accompanies by a low-temperature anomaly. Based on the background field established from the same period of the last ten years, LST at the epicenter on the day of occurrence was 5.68 °C lower than in previous years. Furthermore, the strongest low-temperature anomalies were observed from September 4 to 6, with the anomaly index of −1.95, −1.71, and −1.60, respectively. It is plain that the parameters from the land and atmosphere perform the anomalies at the minimum during the Luding earthquake.
1. Introduction
As one of the common natural disasters, earthquakes are catastrophic events with geologic origins that can cause considerable damage to people’s production and health, resulting from plate movement under tectonic stress. This rupture mechanism and tectonic pattern are controlled by the movement of the Earth’s crust and internal physical circumstances (Elliott et al. Citation2016), characterized by strong destructive forces, broad influences, and interlocking effects. Usually, all sorts of anomalies with respect to geophysics and geochemistry are good proxies for abnormal seismic information. Therefore, exploring the changes and anomalies in various parameters before, during, and after an earthquake allows us to provide valuable references for early warnings and monitoring associated with post-earthquake secondary disasters in this earthquake-prone region in the future.
Water vapor is a vital component of atmospheric material, with the energy absorbed in being vaporized is again released as heat during condensation, so it is identified as a greenhouse gas (Held and Soden Citation2000), playing a vital role in atmospheric circulation and climate change. Since the introduction of GPS meteorology in the twentieth century (Bevis et al. Citation1992), water vapor acquisition techniques have advanced to higher accuracy and spatio-temporal resolution (Businger et al. Citation1996; Rocken et al. Citation1997; Ware et al. Citation2000). Compared with the traditional meteorological observation means, global navigation satellite system (GNSS) technology has significant advantages such as lower operating costs, all-weather availability, and higher temporal resolution in high precision. The zenith tropospheric delay (ZTD) can be estimated from GNSS signals, from which precipitable water vapor (PWV) can be calculated. Used for describing water vapor, PWV is defined as the total of the liquid water condensed by all of the vapor in the air column and is closely related to precipitation. Dey et al. (Citation2004) discovered the anomaly in water vapor for the first time after the Gujarat earthquake. It is found that abnormal changes in water vapor after the earthquake, with precipitation after reaching a peak (Wang et al. Citation2019). Moreover, Guo et al. (Citation2023) have discovered the first declines and significant increases in water vapor content over the GNSS stations nearest the epicenters after three earthquakes occurred in Qinghai-Tibet Plateau. As another essential meteorological parameter, relative humidity (RH) has also been observed to be abnormal in a large number of seismic events (Ross and Elliott Citation1996; Pulinets and Dunajecka Citation2007; Singh et al. Citation2010a and 2010b; Jing et al. Citation2013; Salh et al. Citation2022). Embodying the energy exchanges between land and atmosphere in the phase-change form of water, surface latent heat flux (SLHF) has the potential to represent the land-air coupling. Dey and Singh (Citation2003) found abnormal SLHF before the Gujarat earthquake on January 26, 2001. Not only that, but many studies have revealed that SLHF precursor anomalies were detected prior to the massive earthquake events (Cervone et al. Citation2005; Qin et al. Citation2009; Zhang et al. Citation2013). In addition, PWV, RH, and SLHF correspond pretty well to reflect the phase transition process of water.
Land surface temperature (LST) is one of the most commonly used parameters to examine the identification of seismic temperature anomalies, and many researchers have conducted a series of satellite-based surface temperature anomaly discrimination prior to earthquakes (Wang and Zhu Citation1984; Pergola et al. Citation2010). On the one hand, LST has been observed for the thermal anomaly (Tronin Citation2000; Tramutoli 2001; Ouzounov and Freund Citation2004) and can be treated as the precursor signal for detecting earthquakes because of the apparent temperature change before the mainshock (Gornyi et al. Citation1988; Qiang et al. Citation1992; Jiao et al. Citation2018). In many studies on the identification of seismic temperature anomalies, six main thermal parameters are widely used, which mainly are represented by thermal infrared remote (TIR), outgoing longwave radiation (OLR), surface latent heat flux (SLHF), microwave brightness temperature (MBT), surface temperature (ST), air temperature (AT) (Wu et al.Citation 2012; Xiong and Shen Citation2017; Jing et al. Citation2018; Qi et al.Citation 2021). For instance, LST has been observed with a 4 ∼ 8 °C rise in the southern epicenter seven days before the Kashmir earthquake in 2005 (Panda et al. Citation2007). The high sea surface temperature anomaly appeared around the epicenter 6 days before the 2021 Haiti Mw 7.2 earthquake (Zhang et al. Citation2022). On the other hand, some low-temperature anomalies are also associated with earthquakes. The surface air temperature computed from AIRS data showed a significant decline over the epicenter of the Haiti earthquake of January 12, 2010 (Singh et al. Citation2010b). The decrease in temperature of the Banglazhang spring, China, following two earthquakes was recorded, which was credited to a decrease in permeability (Yan et al. Citation2020). A review of Nepal magnitude 7.9 earthquake on April 25, 2015 showed that LST cooled slowly before the event and reached the minimum during the outbreak (Chen et al. Citation2020).
On September 5, 2022, a strong earthquake with a magnitude of Ms 6.8 hit Luding County, Garze Tibetan Autonomous Prefecture of Sichuan Province, China, and the hypocenter was located at 29.59°N and 102.08°E with the depth concentrating at about 16 km. The data set is provided by China Earthquake Networks Center, National Earthquake Data Center (http://data.earthquake.cn). This Luding earthquake struck in the south of the Xuemengkan to Moxi section of the Xianshui River Faulture Zone, which is one of the three major active fracture zones in Sichuan, along with the Longmen Mountain Fault Zone and the Anning River Fault Zone. This section is located at the intersection of faults and has a complex tectonic background (Burchfiel et al. Citation1995). Since 1700, there have been 17 earthquakes of M 7.0 or greater and 29 earthquakes of M 6.5 or above in these three main fault zones. However, the Xianshui River Fault Zone has been hit by 9 earthquakes with a magnitude of 7.0 or above, suggesting an average of such strong earthquakes once every 36 years. Co-seismic slips of Luding event have ruptured to the surface. It has also been found that anomalous signals were identified within two months prior to the Luding earthquake (Jing et al. Citation2023). Thus, this article analyzes the changes and anomalies of multiple physical parameters from land and atmosphere (PWV, SP, SLHF, LST) during the co-seismic period. The findings of this study can provide a practical reference for earthquake prediction and monitoring, and post-earthquake secondary disaster analysis in this area.
2. Study area, data, and method
2.1. Study area
Sichuan Province is located in western China, adjacent to the Tibetan Plateau in the west, with the terrain of high-west and low-east. The extrusion and collision of the crust have made it unstable and formed fracture zones with the complex tectonic background. Consequently, Sichuan Province has become a strong-earthquake-prone province in China, such as the Wenchuan earthquake in 2008, the Ya’an earthquake in 2013, and the Jiuzhaigou earthquake in 2017. These earthquakes could cause casualties, economic losses, secondary disasters, and environmental problems.
2.2. Data
This study introduced the land and atmospheric sources of data such as PWV, total column water vapor (TCWV), surface pressure (SP), SLHF, LST, and air temperature (AT) for analysis. Among them, PWV is retrieved from GNSS observation data, and other parameters (including meteorological data used for PWV inversion) are obtained from ERA5 reanalysis data. shows the geographic distribution and GNSS stations of the studying area.
Figure 1. Overview of the study area, Sichuan Province, China. The red star indicates the epicenter location. The blue triangle represents the GNSS stations. The irregular lines are fracture zones, the red one refers explicitly to the Xianshui River Fault Zone, where the Luding earthquake occurred, and the orange one is the Anning River Fault Zone.

SP, SLHF, TCWV, LST, and AT are available from the ERA5 dataset, an atmospheric reanalysis of the fifth generation of global climate datasets conducted by the European Centre for Medium-Range Weather Forecasts (ECMWF). With the continuous development of data assimilation algorithms, many scholars assimilate them and numerical weather prediction closely, using data assimilation technology to introduce data into the numerical model. The quality control of the various types of observations (such as ground observations, satellites, radar, sounding, buoys, aircraft, ships, etc.) and their assimilation into the global model resulted in the integration of a comprehensive dataset that can be considered, to some extent, as an approximation of the actual state of the atmosphere and as an optimal reflection. ERA5 reanalysis data provide accurate hourly estimates of a range of meteorological variables for the atmosphere and surface with a high spatial and temporal resolution of 30 km and 1 h (Hersbach et al. Citation2020). Here, we choose the data from August to September, 2022. Additionally, nighttime observations of LST were used because solar radiation, shadows from high-altitude terrain, and heat production from human activities during the day can strongly interfere with surface feature’s temperature information.
PWV is derived from GNSS observation data from the Crustal Movement Observation Network of China (CMONOC) (Gan et al. Citation2012; Liang et al. Citation2015). The GNSS stations we worked with are SCSM, SCTQ, SCJL, SCYX, SCXD, and SCXJ. is the detailed information of GNSS stations.
Table 1. GNSS station information.
2.3. Method
2.3.1. The retrieval of PWV from GNSS
The GNSS signal can generate the tropospheric delay as it passes through the atmosphere, consisting of hydrostatic and wet delays. Furthermore, we also capture PWV indirectly by using the rapidly changing wet delay. The time resolution of GNSS raw observations is 30 s. RTKLIB software was used to obtain the ZTD with the precise point positioning (PPP) static method (Takasu and Yasuda Citation2009). The forward mode was adopted for the filter type. The elevation mask angle was set to 10°. Moreover, the ionosphere-free combination was used for ionospheric correction. In the retrieval, an apriori constraint information was implied that the value from the Saastamoinen model (Saastamoinen Citation1972) was applied for the tropospheric delay correction and still estimated the ZTD in the PPP process. Satellite precise products (orbit and clock error) were applied. The corresponding meteorological data were provided by the ERA5 reanalysis dataset with the 1-h time resolution, as the meteorological file of CMONOC was missing in the selected period. Thus, the PWV derived from GNSS has the same time resolution of 1 h as ERA5. Upon acquiring the estimated ZTD, the zenith hydrostatic delay (ZHD) was calculated using the classical Saastamoinen model. The difference between the above two can get zenith wet delay (ZWD), which is the delayed part where the water vapor changes. The implementation from ZWD to PWV also requires a key parameter, which is the water vapor conversion coefficient Ԥ, calculated from the atmospheric weighted average temperature Tm and some atmospheric constants. Moreover, Tm was obtained from the atmospheric weighted average temperature model for different latitudes proposed by Yao et al. (Citation2014), Tm = 105.1529 + 0.6117 × Ts for 15 ∼ 30°N, Tm = 2.8034 + 0.9533 × Ts for 30 ∼ 45°N.
(1)
(1)
(2)
(2)
(3)
(3)
(4)
(4)
where Ps presents the pressure of ground GNSS station; f (
) is a function of the latitude φ and geodetic height H. Ԥ means the water vapor conversion coefficient, calculated by Askne and Nordius (Citation1987):
(5)
(5)
where
is the density of water;
stands for water gas parameter;
and
mean the physical constants for the atmosphere with values equal to
and
Tm is based on the latitude of different GNSS stations using the different formulas mentioned above.
2.3.2. The RST algorithm
Robust Satellite Technique (RST) is a statistical-based thermal anomaly discrimination technique that highlights anomalies in comparatively stable conditions over the spatial and temporal domains. It has the advantage of being widely applicable by accommodating multiple sensor data, diverse geographical locations, different observation conditions, etc. The algorithm was first proposed by Tramutoli (Citation2007) and was mainly used for forest fire monitoring. Besides, it has been developed for other natural environmental problems such as flood warnings, volcanic eruption, and earthquake anomaly monitoring, as RST excels in identifying abnormal heat signals. The anomaly index obtained by the RST algorithm is commonly called ALICE (Absolutely Local Index of Change of the Environment).
(6)
(6)
where
is the value of LST for the current time;
indicates the average LST of multiple years;
is the standard deviation of LST of multiple years.
3. Results
3.1. Precipitable water vapor
Concerning water recycling, PWV is one of the primary conditions for precipitation. The ZTDs from six GNSS stations in and around the Luding epicenter were estimated, and we further conducted PWV retrieval according to the method described in the last section. shows the PWV changes over six GNSS stations from August 16 to September 30. It is evident that the mainshock eruption triggered a rapid decrease in PWV. Before the earthquake, PWVs at six stations began to decline on August 28 and reached the trough until September 5, when the earthquake broke out, and no precipitation formed. After the earthquake, PWV gradually rose and returned to normal. Stations SCSM and SCTQ are relatively closest to the epicenter, which are 47 km and 79 km far away, so the PWV changes most dramatically and distinctly due to the tremendous clout. The value was 43.21 mm for the SCSM station and 37.84 mm for the SCTQ station at the event outbreak, decreased by 33.49 and 36.05 mm, respectively. On the other hand, the fluctuant reductions at stations SCJL, SCYX, SCXD, and SCXJ were small, most likely due to the relatively long distance from the epicenter and the higher altitude with short ait column. In addition, the variation of PWV in these GNSS stations also implies the variation of PWV over the epicenter. This is the most significant dropping trend and the most apparent change accompanying the earthquake occurrence. Prior to this co-seismic period, relatively intensive precipitation began on August 28 with 8.9 mm of rainfall, with light to moderate precipitation levels until August 31. This is due to the fact that PWV, which developed at the time of the gestation of the earthquake, created precipitation conditions that subsequently decreased. As a matter of fact, PWV is one of the conditions for precipitation. High PWV levels are bound to trigger precipitation events, and a prerequisite for precipitation is an increase in PWV or saturation of high values. In other words, precipitation is accompanied by high levels of PWV. However, the change in GNSS-PWV found in Luding outburst was characterized by decreasing and minimum. Therefore, this change was not caused by the precipitation. So, the effect of precipitation was ruled out. Thus, the abnormal decline change of PWV was displayed with the Luding earthquake occurring.
Figure 2. Water vapor changes during the event. (a) GNSS-PWV retrieval results and precipitation on six GNSS stations from August 16 to September 30, 2022. The red vertical line represents the moment of the earthquake outbreak. (b) Difference between total column water vapor of each year for 2015 to 2022 and multi-year average from August 1 to September 30. The gray vertical line represents the moment of the Luding earthquake outbreak in 2022.

Based on the location distribution of individual GNSS stations in and the variation of GNSS-PWV in , it can actually be visualized that the PWVs of the SCTQ station, which is the nearest station to the epicenter, and the SCSM, SCYX, and SCXD stations, which are located at the southeastern end of the Xianshui River rupture zone, have a trough at the moment of the outburst, which further reflects the change of the seismic response along the rupture zone. The stations SCXJ and SCJL, which are neither in close proximity to the epicenter nor along the Xianshui River Fault Zone, did not have the lowest values at the moment of the earthquake outburst, but only on September 10 and September 12, respectively, and then rose with the same trend as the other four stations.
shows the difference between each year’s total column water vapor from 2015 to 2022 and a multi-year average of the epicenter. It is clearly illustrated that the variation of total column water vapor in the epicenter from ERA5 is consistent with the PWV results retrieved from the six GNSS stations. Over the epicenter, the water vapor content was at the lowest trough of 18.61 mm on the outbreak day, particularly 10.52 mm lower than the average of the past seven years, coupling with a dramatic drop from the peak on August 28 and subsequently a return to normal levels after September 13 (Bold red line in ). This difference is the second largest over the same period in the last eight years, with the most enormous amount of water vapor below the average for the same period occurring on August 16, 2019. Based on meteorological data for August and September in 2019, we find that this extraordinarily low value is attributed to the fact that August 16 had the highest air temperatures of the two months, with a maximum temperature of 33 °C. High temperatures and high solar radiation stimulate the scorching, making the air dry, with little water vapor content. From the same period from 2015 to 2022, it is also apparent that the total water vapor on September 5 during the co-seismic in 2022 was remarkably lower than that of others. Furthermore, total column water vapor had the highest value on August 28, before the earthquake, and was 8.39 mm higher than the other multi-year averages. Both studies from the epicenter and six nearby GNSS stations found that PWV began to drop sharply on August 28 and was at the trough when the Luding event occurred. The abnormal phenomenon in water vapor existed from August 28 to September 13. Therefore, the Luding earthquake leads to a significant decrease in water vapor. In all likelihood, the underground and surface temperatures do not rise, and no thermal anomalies are present, so the surface evaporation and moisture movement are not stimulated. Next, we will examine the changes in land surface temperature as well in Section 3.4.
3.2. Surface pressure
Surface pressure can indicate the movement of air in a vertical plane. The density of water vapor is smaller than the density of air. When the air contains more water vapor, the air becomes less dense, and the atmospheric pressure decreases with it; conversely, when the air has less water vapor, the density of air increases, and the pressure rises. From , it can be seen that surface pressure has risen since September 5, 2022 and the change was the most pronounced from 2015 to 2022, being about 5.8 pa above the multi-year average. This is precisely because of the sharp decrease in water vapor, which causes air density to increase and air currents to sink, lagging behind and causing surface air pressure to rise.
3.3. Surface latent heat flux
SLHF is the parameter that reflects the energy transferring of water between the surface and atmosphere, which mainly embodies different forms in the phase transition of water, such as evaporation, liquefaction, and condensation. It has been suggested that the enhancement and accumulation of stress contributed to the energy exchange between the surface and the atmosphere in the coastal area before the earthquake, resulting in a significant increase in SLHF (Ghosh et al. Citation2021). It is repeatedly confirmed that earthquakes occurring inland are accompanied by anomalies in SLHF, expanding the application of it in seismic research (Cervone et al. Citation2006; Natarajan and Philipoff Citation2018). illustrates the spatial evolution of the SLHF gain and loss change with the background field removed. The background field was established using data for the same period from 2013 to 2021. Positive values imply an increase in the exchange of water heat from the surface system to the atmosphere, i.e. an increase in evaporation compared to previous years; negative values indicate a decrease in this water heat exchange, i.e. a decrease in evaporation. The phenomenon of decreasing latent heat flux from previous years, indicating a decrease in latent heat exchange from the surface to the atmosphere, i.e. a decrease in evaporation, gradually appeared at the epicenter and nearby areas from August 28 onward, and it continued until September 7 onward. The weakening at the epicenter was significant. Moreover, combining with , it can be seen that the latent heat at the location of the epicenter is lower than the background field data from August 28 to September 9 of the co-seismic period by 76.8 W/m2, 80.7 W/m2, 64.1 W/m2, and 76.0 W/m2, respectively. Starting after September 9, the SLHF of 2022 shows an increasing trend compared to the previous years, with an increase in the exchange of water heat from the surface to the atmosphere. Notably, the SLHF increased in the eastern plains of Sichuan Province with substantial area on September 5, with most of them increasing by about 150 W/m2. Furthermore, a predominantly negative correlation exists between surface latent heat flux and precipitation on the plateau. The precipitation of light rain and showers represented high moisture level over the epicenter from September 18 to 25, while together with low surface latent heat flux, is satisfying this pattern. While there was almost 0 precipitation in the days before and after the earthquake eruption (September 4 to 7), high surface latent heat fluxes were not seen, but also very low levels. Thus, the first trough of the SLHF presents the anomaly accompanied by an earthquake, and the second trough shows the negative correlation during the precipitation occurrence. In light of the above analysis, SLHF in the epicenter responded to the significantly decreasing in water vapor over the epicenter.
Figure 4. Surface latent heat flux difference variation from August to September of 2013–2022. (a) Spatial evolution of SLHF changes in 2022 with background fields removed. The red irregular line is the Xianshui River Fault Zone, and the black star denotes the location of the epicenter. (b) Comparison of SLHF of epicenter in 2022 with average values from 2013 to 2021.

3.4. Land surface temperature and air temperature
Earthquakes are usually accompanied by temperature anomalies, which can manifest as thermal or low-temperature anomalies. In the process of seismic development, underground rock fracture, fault friction, hot gas upwelling, and tectonic stress accumulation will generate a large amount of energy so that the surface temperature will keep going up. At the same time, the temperature significantly affects rock tensile strength, which is tied to the loss of water and minerals as well as thermal stresses (Lü et al. Citation2017). Thus, studies on the temperature anomaly characteristics of these events are essential for earthquake prediction.
In this paper, two temporal scales of LST are taken: one is the changes during the present earthquake period, and another is the changes supported by the background field data established from the same period of the past ten years, i.e. the results of anomalies screening applying robust satellite technique (RST) method. The RST algorithm employs the LST multi-year average as the background field to obtain the anomaly index ALICE. Establishing the background field is conducive to removing interference backgrounds such as climate change, seasonal perturbations, and geographic differences for effectively extracting anomalies, especially in the plateau region. Therefore, it can better extract the surface temperature anomalies of the current earthquake and highlight the anomalies in the spatial and temporal domains. The results from the two separate temporal studies demonstrate that the Luding earthquake event is a low-temperature anomaly. shows the LST variation in Sichuan province before and after the earthquake, illustrating that the current LST gradually cooled over a wide area during the period with the onset of the earthquake. The epicenter and nearby areas began to cool down extensively on August 28 before the quake, with a minimum of 2.4 °C on September 5, and then gradually warmed up again to previous levels. As a result, the event occurred with a temperature reduction of around 10 °C before and after.
Based on the RST algorithm using decadal background data of the same period from 2013 to 2021, we found a significantly low-temperature anomaly in the epicenter area of Luding from September 4 to 6. depicts the LST anomaly based on the decadal background field. shows the variation of the difference between the current period of the earthquake and the 10-year mean value of the same period, and gives the variation of the anomaly index ALICE by RST. As shown in , LST at the epicenter area was lower than in previous years from September 4 to 6, with the difference values of −6.20 °C, −5.68 °C, and −4.30 °C. In late August, the increased amplitude of LST was less than 2 °C in the epicenter, with a slight change. However, there was a significant temperature increase in the plain area of eastern Sichuan Province, even with a maximum increase of 9.19 °C. Then, this phenomenon gradually subsided with the incoming earthquake. It is shown in that a large area of intense low-temperature anomalies existed in the epicenter and its western region from September 4 to 6, excluding the low-temperature factor due to high altitude. Especially on September 4, the anomaly index in the epicenter was −1.95 (negative value indicates low-temperature anomaly), while the western part was in the range of −2.5 to −2, and it could even reach an extremum of −2.7. The extent of the low-temperature anomaly along with this earthquake is evident. When the earthquake broke out on September 5, the anomaly index in the epicenter was −1.71, the area of the low-temperature anomaly was decreasing in the western mountainous region, and the most substantial anomaly index was −2.58. It also indicated that the intensity of the low-temperature anomaly began to weaken progressively, and the space of the anomaly tended to reduce. ALICEs of the epicenter from August 26 to 28 were 0.88, 1.20, and 0.92, respectively, with a small degree of anomaly. In summary, employing LST data of the same period with the earthquake from ten years, this study uncovered the appearance of low-temperature anomalies associated with the Luding earthquake from September 4 to 6.
Figure 6. Land surface temperature changes in Sichuan bases on previous multi-year. (a) Anomaly changes in LST deviation based on the background field data of August 26 to September 8 from 2013 to 2021. (b) Anomaly changes in the LST anomaly index (ALICE) based on the background field data of August 26 to September 8 from 2013 to 2021.

In parallel, the air temperature over the epicenter is shown in , which also significantly decreased during the earthquake.
With the analysis of the multi-parameter from the land and atmosphere, we can conclude that there are anomalies in LST, SLHF, PWV, and SP. Specifically, air and land surface temperatures are consistent with changes in SLHF. Both started to fall on August 28 and remained minimal during the co-seismic until September 9. The change in PWV is a sudden and abrupt drop at the onset of the main shock, and SP is precisely the opposite. The low temperature in LST of the Luding earthquake caused a decrease in SLHF, indicating a decline in ground-air energy transfer and a decrease in water vapor by evapotranspiration, and subsequently, a downturn in the sharp change in PWV on the day of the outbreak. The coupling of the lithosphere and atmosphere during the period of the Luding earthquake is well studied in this paper.
4. Discussion
In conjunction with the parameters of atmospheric precipitable water vapor, surface pressure, surface latent heat flux, land surface temperature, and air temperature that we analyzed, excluding the background field data from previous years, we revealed that the Luding earthquake showed low-value anomalies in the temperature, water vapor, latent heat from August 28 to September 5 before the occurrence and accompanied by the minimum on the Luding earthquake outbreak day.
The land surface and air temperatures are both in low-temperature anomalies, and the cooling started on August 28. The LST anomaly index (ALICE) of the epicenter reached −1.95, −1.71, and −1.60 on September 4 to 6, respectively, with a substantial degree of low temperature. According to the results of the rock test, it is found that compression leads to a temperature increase, and tension leads to a temperature decrease. Moreover, the Luding earthquake is in a zone of weakness at the high-value edge of the shear strain of a large strike-slip fault zone and a tensile zone of the strain perpendicular to the fault direction (Yuan et al. Citation2023). Wang et al. (Citation2023) found that the source region of Luding earthquake was located exactly at the transition of the surface strain rate from extrusion strain to tensile strain. Hence, the tensile effect was responsible for the presence of pores in narrow cracks in the underground and surface cooling, consequently leading to insufficient surface heat energy that suppressed surface evaporation. Because the surface latent heat is mainly generated by phase changes in water (evaporation of surface moisture and plant transpiration), less water vapor was made available to the atmospheric air column. According to physical properties, the air is denser than water vapor. It plays a leading role when PWV content is reduced, so the airflow is sunk, resulting in high surface pressure. On the other hand, high pressure was also developed as a direct result of the cooling of the land surface. The high pressure did not immediately form on September 5, but lagged for 1 day, gradually increasing and peaked on September 9. Additionally, PWV serves as one of the conditions for precipitation. The presence of light and moderate rainfall at the epicenter location one week before the Luding earthquake but the complete absence of rainfall on the eruption day is consistent with the minimum value characteristic of PWV. Meanwhile, a study showed through low-velocity anomalies that fluids reaching the seismic zone may reduce the coefficient of friction and the effective positive stress on the section, thus lowering the friction and triggering the strike-slip motion of the fracture zone and the occurrence of the Luding earthquake (Song et al. Citation2023). The reduction of friction also inhibited the generation of thermal anomalies. In accordance with the PWV variations from the retrieval of six ground-based GNSS stations, stations SCTQ, which is close by, and SCSM, SCYX, and SCXD, which are along the southeastern end of the Xianshui River Fault Zone, were the ones that reached the minimums at the time of the outburst. However, stations SCXJ and SCJL, which are neither close nor along the fault zone, were experiencing the trough with a lag. The effects of earthquake-induced PWV fluctuations were transmitted along the rupture zone to the southeast of Xianshui River and Anning River Fault Zone, and subsequently propagated to both sides. Possibly, it is indicated that seismic wave transmission and fracture are more inclined along the southeast direction of the Xianshui River Fault Zone and Anning River Fault Zone. The Luding earthquake brought the fault zones closer to failure, denoting enhanced earthquake hazard (Li et al. Citation2022). Hu et al. (Citation2018) defined the process of thermal coupling between the surface and the atmosphere as atmospheric temperature feedback, so this feedback mechanism will also have the effect of cold-low coupling between the ground and the air, as in the case of the Luding earthquake we presented above.
5. Conclusions
We worked with multiple data sources, such as GNSS and ERA5 reanalysis information, to retrieve PWV and acquire land surface temperature anomalies at the stations near the epicenter. The results show that the occurrence of the Luding earthquake caused a sharp drop in atmospheric PWV, which returned to normal after the event. Surface latent heat flux, a parameter representing the heat flux caused by the water phase change, began to plummet and reached a minimum at the time of the event, which was also aligned with the water vapor changes. The individual changes of these two parameters also coincide with each other to manifest the reduction of atmospheric water vapor. There was a lagged increase in surface air pressure, signifying the sinking of air currents due to a decrease in water vapor and an increase in air density. Furthermore, the low-temperature anomalies of the earthquake were found based on two-temporal scales. Comparing the pre- and post-event periods, we revealed that the temperature commenced decreasing on August 28 and achieved the trough on September 5, with a drop of 10 °C. Further, a large-area low-temperature anomaly was detected at the epicenter and to the west based on the LST background field of the ten-year same period, with the anomaly indices ranging from −2.7 to −2, and then the anomaly gradually disappeared. Regarding epicenter, LST was lower than on September 4 to 6 in previous years, with differences of −6.20 °C, −5.68 °C, and −4.30 °C, corresponding to the anomaly indices of −1.95, −1.71, and −1.60, respectively. The low LST anomaly associated with the Luding earthquake reduced the effect of heat exchange between the terrestrial environment and air. Hence, the evaporation and movement collision of moisture was not promoted, the water vapor content decreased, the SLHF was minimized on September 5, and the surface pressure rose. These changes are not only found in the current earthquake year, but also are anomalous compared to the same period for the past few years.
Many significant earthquakes along the Xianshuihe fault zone, such as Luhuo County Ms 6.8 earthquake on August 30, 1967; Luhuo County Ms 7.6 earthquake on February 6, 1973; Daofu County Ms 6.9 earthquake on January 24, 1981; Kangding County Ms 6.3 earthquake on November 22, 2014, and Luding Ms 6.8 earthquake on September 5, 2022 have the aftershocks. Even these have triggered secondary disasters (An et al. Citation2023). Consequently, it is significant to strengthen earthquake forecasting and earthquake-proof construction in these areas. Furthermore, tracking changes in surface and meteorological parameters during the earthquake event permits monitoring and predicting the occurrence of post-earthquake secondary disasters.
Author contributions
A.G. and Y.X. proposed the idea, designed computer programs, and wrote the initial draft. A.G., N.J., Y.W., and Z.G. performed the PWV results retrieved from GNSS and reanalysis data. A.G. and S.L. performed the temperature anomaly data presentation and had a further analysis on other parameters. Y.X., N.J., and T.X. revised the manuscript and acquired financial support for this publication. Y.X. revised the equations and format of the manuscript, specifically critical review, and revision.
Acknowledgments
The authors thank for the data support from China Earthquake Networks Center, National Earthquake Data Center (http://data.earthquake.cn).
Disclosure statement
No potential conflict of interest was reported by the authors.
Data availability statement
The data that support the findings of this study are available from the corresponding author upon reasonable request.
Additional information
Funding
References
- An Y,Wang D,Ma Q,Xu Y,Li Y,Zhang Y,Liu Z,Huang C,Su J,Li J, et al. 2023. Preliminary report of the September 5, 2022 MS 6.8 Luding earthquake, Sichuan, China. Earthq Res Adv. 3(1):100184.doi: 10.1016/j.eqrea.2022.100184.
- Askne J, Nordius H. 1987. Estimation of tropospheric delay for microwaves from surface weather data. Radio Sci. 22(3):379–386. doi:10.1029/RS022i003p00379.
- Bevis M, Businger S, Herring TA, Rocken C, Anthes RA, Ware RH. 1992. GPS meteorology: remote sensing of atmospheric water vapor using the global positioning system. J Geophys Res. 97(D14):15787–15801. doi:10.1029/92JD01517.
- Burchfiel BC, Zhiliang C, Yupinc L, Royden LH. 1995. Tectonics of the Longmen Shan and Adjacent Regions, Central China. Int Geol Rev. 37(8):661–735. doi:10.1080/00206819509465424.
- Businger S, Chiswell SR, Bevis M, Duan J, Anthes RA, Rocken C, Ware RH, Exner M, VanHove T, Solheim FS. 1996. The Promise of GPS in atmospheric monitoring. Bull Amer Meteor Soc. 77(1):5–18. doi:10.1175/1520-0477(1996)077<0005:TPOGIA>2.0.CO;2.
- Cervone G, Maekawa S, Singh RP, Hayakawa M, Kafatos M, Shvets A. 2006. Surface latent heat flux and nighttime LF anomalies prior to the Mw = 8.3 Tokachi-Oki earthquake. Nat Hazards Earth Syst Sci. 6(1):109–114. doi:10.5194/nhess-6-109-2006.
- Cervone G, Singh RP, Kafatos M, Yu C. 2005. Wavelet maxima curves of surface latent heat flux anomalies associated with Indian earthquakes. Nat Hazards Earth Syst Sci. 5(1):87–99. doi:10.5194/nhess-5-87-2005.
- Chen S, Liu P, Feng T, Wang D, Jiao Z, Chen L, Xu Z, Zhang G. 2020. Exploring changes in land surface temperature possibly associated with earthquake: case of the April 2015 Nepal Mw 7.9 earthquake. Entropy. 22(4):377. doi:10.3390/e22040377.
- Dey S, Sarkar S, Singh RP. 2004. Anomalous changes in column water vapor after Gujarat earthquake. Adv Space Res. 33(3):274–278. doi:10.1016/S0273-1177(03)00475-7.
- Dey S, Singh RP. 2003. Surface latent heat flux as an earthquake precursor. Nat Hazards Earth Syst Sci. 3(6):749–755. doi:10.5194/nhess-3-749-2003.
- Elliott JR, Walters RJ, Wright TJ. 2016. The role of space-based observation in understanding and responding to active tectonics and earthquakes. Nat Commun. 7(1):13844. doi:10.1038/ncomms13844.
- Gan W, Li Q, Zhang R, Shi H. 2012. Construction and application of tectonic and environmental observation network of mainland China. J Eng Stud. 4(04):324–331.
- Ghosh S, Chowdhury S, Kundu S, Sasmal S, Politis DZ, Potirakis SM, Hayakawa M, Chakraborty S, Chakrabarti SK. 2021. Unusual surface latent heat flux variations and their critical dynamics revealed before strong earthquakes. Entropy. 24(1):23. doi:10.3390/e24010023.
- Gornyi VI, Sal’Man AG, Tronin AA, Shilin BV. 1988. Outgoing infrared radiation of the earth as an indicator of seismic activity. Akademiia Nauk SSSR Doklady. 301:67–69.
- Guo A, Xu Y, Jiang N, Wu Y, Gao Z, Li S, Xu T, Bastos L. 2023. Analyzing correlations between GNSS retrieved precipitable water vapor and land surface temperature after earthquakes occurrence. Sci Total Environ. 872:162225. doi:10.1016/j.scitotenv.2023.162225.
- Held IM, Soden BJ. 2000. Water vapor feedback and global warming. Annu Rev Energy Environ. 25(1):441–475. doi:10.1146/annurev.energy.25.1.441.
- Hersbach H, Bell B, Berrisford P, Hirahara S, Horányi A, Muñoz-Sabater J, Nicolas J, Peubey C, Radu R, Schepers D, et al. 2020. The ERA5 global reanalysis. Quart J Royal Meteoro Soc. 146(730):1999–2049. doi:10.1002/qj.3803.
- Hu X, Cai M, Yang S, Sejas SA. 2018. Air temperature feedback and its contribution to global warming. Sci China Earth Sci. 61(10):1491–1509. doi:10.1007/s11430-017-9226-6.
- Jiao Z-H, Zhao J, Shan X. 2018. Pre-seismic anomalies from optical satellite observations: a review. Nat Hazards Earth Syst Sci. 18(4):1013–1036. doi:10.5194/nhess-18-1013-2018.
- Jing F, Jiang M, Zhang L, Singh RP. 2023. Detection and identification of pre-seismic thermal anomalies in cloudy conditions associated with the 2022 Luding (China) Mw 6.6 earthquake. IEEE Trans Geosci Remote Sensing. 61:1–12. doi:10.1109/TGRS.2023.3304975.
- Jing F, Shen XH, Kang CL, Xiong P. 2013. Variations of multi-parameter observations in atmosphere related to earthquake. Nat Hazards Earth Syst Sci. 13(1):27–33. doi:10.5194/nhess-13-27-2013.
- Jing F, Singh RP, Sun K, Shen X. 2018. Passive microwave response associated with two main earthquakes in Tibetan Plateau, China. Adv Space Res. 62(7):1675–1689. doi:10.1016/j.asr.2018.06.030.
- Li Y, Zhao D, Shan X, Gao Z, Huang X, Gong W. 2022. Co-seismic Slip Model of the 2022 Mw 6.7 Luding (Tibet) Earthquake: pre- and Post-Earthquake Interactions With Surrounding Major Faults. Geophys Res Lett. 49(24):e2022GL102043. doi:10.1029/2022GL102043.
- Liang H, Cao Y, Wan X, Xu Z, Wang H, Hu H. 2015. Meteorological applications of precipitable water vapor measurements retrieved by the national GNSS network of China. Geod Geodyn. 6(2):135–142. doi:10.1016/j.geog.2015.03.001.
- Lü C, Sun Q, Zhang W, Geng J, Qi Y, Lu L. 2017. The effect of high temperature on tensile strength of sandstone. Appl Therm Eng. 111:573–579. doi:10.1016/j.applthermaleng.2016.09.151.
- Natarajan V, Philipoff P. 2018. Observation of surface and atmospheric parameters using “NOAA 18” satellite: a study on earthquakes of Sumatra and Nicobar Is regions for the year 2014 (M ≥ 6.0). Nat Hazards. 92(2):1097–1112. doi:10.1007/s11069-018-3242-y.
- Ouzounov D, Freund F. 2004. Mid-infrared emission prior to strong earthquakes analyzed by remote sensing data. Adv Space Res. 33(3):268–273. doi:10.1016/S0273-1177(03)00486-1.
- Panda SK, Choudhury S, Saraf AK, Das JD. 2007. MODIS land surface temperature data detects thermal anomaly preceding 8 October 2005 Kashmir earthquake. Int J Remote Sens. 28(20):4587–4596. doi:10.1080/01431160701244906.
- Pergola N, Aliano C, Coviello I, Filizzola C, Genzano N, Lacava T, Lisi M, Mazzeo G, Tramutoli V. 2010. Using RST approach and EOS-MODIS radiances for monitoring seismically active regions: a study on the 6 April 2009 Abruzzo earthquake. Nat Hazards Earth Syst Sci. 10(2):239–249. doi:10.5194/nhess-10-239-2010.
- Pulinets SA, Dunajecka MA. 2007. Specific variations of air temperature and relative humidity around the time of Michoacan earthquake M8.1 Sept. 19, 1985 as a possible indicator of interaction between tectonic plates. Tectonophysics. 431(1-4):221–230. doi:10.1016/j.tecto.2006.05.044.
- Qi Y,Miao Z,Wu L,Ding Y. 2021.Seismic Microwave Brightness Temperature Anomaly Detection Using Multitemporal Passive Microwave Satellite Images: Ideas and Limits. IEEE J Sel Top Appl Earth Obs Remote Sens. 14:6792–6806. doi:10.1109/JSTARS.2021.3093819.
- Qiang Z, Kong L, Wang Y, Li Q, Lin C, Xu X. 1992. Earth gas emission, infrared thermo-anomaly and seismicity. Chin Sci Bull. (24):2259–2262.
- Qin K, Guo G, Wu L. 2009. Surface latent heat flux anomalies preceding inland earthquakes in China. Earthq Sci. 22(5):555–562. doi:10.1007/s11589-009-0555-7.
- Rocken C, Van Hove T, Ware R. 1997. Near real-time GPS sensing of atmospheric water vapor. Geophys Res Lett. 24(24):3221–3224. doi:10.1029/97GL03312.
- Ross RJ, Elliott WP. 1996. Tropospheric Water Vapor Climatology and Trends over North America: 1973–93. J Climate. 9(12):3561–3574. doi:10.1175/1520-0442(1996)009<3561:TWVCAT>2.0.CO;2.
- Saastamoinen J. 1972. Atmospheric correction for the troposphere and stratosphere in radio ranging satellites. In: Henriksen SW, Mancini A, Chovitz BH, editors. The Use of artificial satellites for geodesy. Washington, DC: American Geophysical Union. . pp. 247– 251.
- Salh H, Muhammad A, Ghafar MM, Külahcı F. 2022. Potential utilization of air temperature, total electron content, and air relative humidity as possible earthquake precursors: a case study of Mexico M7.4 earthquake. J Atmos Sol Terr Phys. 237:105927. doi:10.1016/j.jastp.2022.105927.
- Singh RP, Mehdi W, Gautam R, Senthil Kumar J, Zlotnicki J, Kafatos M. 2010a. Precursory signals using satellite and ground data associated with the Wenchuan Earthquake of 12 May 2008. Int J Remote Sens. 31(13):3341–3354. doi:10.1080/01431161.2010.487503.
- Singh RP, Waseem M, Manish S, Waseem Mehdi. 2010b. Complementary nature of surface and atmospheric parameters associated with Haiti earthquake of 12 January 2010. Nat. Hazards Earth Syst. Sci. 10(6):1299–1305. doi:10.5194/nhess-10-1299-2010.
- Song X, Lei J, Zou K. 2023. The 2022 Luding, Sichuan, China, M 6.8 earthquake: a fluid-related earthquake? J Asian Earth Sci. doi:10.1016/j.jseaes.2023.105543.
- Takasu T, Yasuda A. 2009. Development of the low-cost RTK-GPS receiver with an open source program package RTKLIB. International symposium on GPS/GNSS. Jeju: International Convention Center.
- Tramutoli V. 2007. Robust Satellite Techniques (RST) for natural and environmental hazards monitoring and mitigation: Theory and applications. Proceedings of the 2007 International workshop on the analysis of multi-temporal remote sensing images; Leuven, Belgium. pp. 139–144. doi:10.1109/MULTITEMP.2007.4293057.
- Tramutoli V, Bello GD, Pergola N, Piscitelli S. 2001. Robust satellite techniques for remote sensing of seismically active areas. Ann Geophys. 44(2):295–312.
- Tronin AA. 2000. Thermal IR satellite sensor data application for earthquake research in China. Int J Remote Sens. 21(16):3169–3177. doi:10.1080/01431160050145054.
- Wang L, Zhu C. 1984. Anomalous variation of ground temperature before the Tangsan and Haicheng earthquake. J Seismol Res. 97(06):649–656.
- Wang X, Li S, Song S. 2023. Coseismic Slip distribution and coulomb stress variation of 2022 Luding MW 6.6 earthquake based on InSAR constraint. J Geodesy Geodynam. 43(3):226–231,238.
- Wang Y, Ren D, Lou Z, Hao Z. 2019. Preliminary study on abnormal changes of PWV after earthquake. J Geodesy Geodynam. 39(09):881–883 + 909.
- Ware RH, Fulker DW, Stein SA, Anderson DN, Avery SK, Clark RD, Droegemeier KK, Kuettner JP, Minster JB, Sorooshian S. 2000. SuomiNet: a real–time national GPS network for atmospheric research and education. Bull Amer Meteor Soc. 81(4):677–694. doi:10.1175/1520-0477(2000)081<0677:SARNGN>2.3.CO;2.
- Wu L-x,Qin K,Liu S-j. 2012.GEOSS-based thermal parameters analysis for earthquake anomaly recognition. Proc IEEE. 100(10):2891–2907.doi:10.1109/JPROC.2012.2184789.
- Xiong P, Shen X. 2017. Outgoing longwave radiation anomalies analysis associated with different types of seismic activity. Adv Space Res. 59(5):1408–1415. doi:10.1016/j.asr.2016.12.011.
- Yan X, Shi Z, Zhou P, Zhang H, Wang G. 2020. Modeling Earthquake-Induced Spring Discharge and Temperature Changes in a Fault Zone Hydrothermal System. JGR Solid Earth. 125(7):e2020JB019344. doi:10.1029/2020JB019344.
- Yao Y, Zhang B, Xu C, Chen J. 2014. Correlation analysis of T_m-T_s and construction of linear relationship model of global latitude correlation. Chin Sci Bull. 59(19):2340–2347. doi:10.1007/s11434-014-0275-9.
- Yuan Z, Zhao J, Huang Y, Yu H, Niu A, Ma H, Ma L. 2023. Study on deformation characteristics and dynamic cause of the Luding MS6.8 earthquake. Front Earth Sci. 11. doi:10.3389/feart.2023.1232205.
- Zhang L, Jiang M, Jing F. 2022. Sea temperature variation associated with the 2021 Haiti Mw 7.2 earthquake and possible mechanism. Geomatics Nat Hazards Risk. 13(1):2840–2863. doi:10.1080/19475705.2022.2137439.
- Zhang W, Zhao J, Wang W, Ren H, Chen L, Yan G. 2013. A preliminary evaluation of surface latent heat flux as an earthquake precursor. Nat Hazards Earth Syst Sci. 13(10):2639–2647. doi:10.5194/nhess-13-2639-2013.