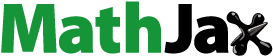
Abstract
Natural hazards always have significant impacts on the regional ecological environment, causing huge damages to biodiversity, soil erosion, and impoverishment. This study aimed to analyze the variation of ecological environment quality in Nepal from 2014 to 2018, with a specific focus on the impact and recovery of the 2015 Ms8.1 Nepal earthquake. We utilized Google Earth Engine (GEE) and remote sensing based ecological index (RSEI) to evaluate the ecological environment quality. Moreover, Moran’s I and Geodetector model were employed to explore spatial clustering and driving forces of post-earthquake recovery. The results indicate that after the 2015 Nepal earthquake, there was a decrease of 0.0225 in the mean RSEI in Nepal. The monitoring of the severe-affected areas showed that the regional environmental quality gradually improved within three years after the earthquake. After a span of three years, the ecological environment managed to recover to its pre-earthquake level. In terms of spatial distribution, there is a notable correlation in Nepal, with the ecological environment exhibiting higher quality in the southern regions and lower quality in the northern regions. By examining the driving forces behind the changes in ecological environment quality during the post-earthquake recovery period, it has been determined that altitude, precipitation, and their interaction serve as influential factors. These findings provide valuable insights into the state of ecological environment recovery after the 2015 Nepal earthquake. Furthermore, this study establishes a robust theoretical foundation for local decision-makers to develop ecological protection measures and enhance local disaster risk management.
1. Introduction
To this day, a wide range of natural hazards continues to occur frequently, including storms, floods, tsunamis, earthquakes, mudslides, and more (Liu, Liu, Lin, et al. Citation2023; Liu, Zhang, et al. Citation2023). These events often lead to ecological deterioration, building damage, economic losses, and psychological issues for the affected population (Kane et al. Citation2018; Liu et al. Citation2021). The process of post-disaster recovery is both complex and lengthy (Gao, Chen, et al. Citation2020). Among these natural hazards, earthquakes stand out as one of the most destructive geological phenomena, inflicting significant and enduring negative effects (Liu et al. Citation2019, Citation2020; Li et al. Citation2020). The effective recovery after such natural hazards holds paramount importance for local human survival and sustainable social development. Despite its crucial significance, the post-disaster recovery stage is often overlooked or neglected within the various stages of disaster risk management (Ghaffarian et al. Citation2021). This, in turn, hampers the provision of crucial information to decision-makers and planners regarding the extent of damage and the recovery process after a natural hazard.
With the advent of remote sensing technology, the availability and analysis of large-scale, real-time ground information have greatly improved, leading to its widespread utilization in disaster risk management. For instance, Lin et al. (Citation2004) conducted an evaluation of vegetation recovery in landslide-prone areas resulting from the 921 earthquake in central Taiwan, employing SPOT satellite images. Contreras et al. (Citation2016) utilized Italy as a case study, effectively integrating remote sensing, geographic information system (GIS), and ground observations to assess the progress of post-earthquake recovery. Additionally, Cooner, Shao, and Campbell (Citation2016) employed remote sensing technology in conjunction with machine learning algorithms to detect damage to urban areas after the 2010 Haiti earthquake. These studies showcase the significant role that remote sensing plays significance in monitoring and analyzing the impacts of earthquakes and guiding post-disaster recovery efforts.
In ecological environment assessment studies, the development of remote sensing technology has also made satellite imagery a valuable tool for collecting data in the post-disaster phase (Lin et al. Citation2004; Ghaffarian, Kerle, et al. Citation2018; Ghaffarian, Rezaie Farhadabad, et al. Citation2020; Ghaffarian et al. Citation2021). So far, various remote sensing evaluation indexes have been developed to characterise or quantify ecological quality status (Yang et al. Citation2021). Such as enhanced vegetation index (EVI) (Wang et al. Citation2008), land surface temperature (LST) (Liao and Jiang Citation2020), normalized difference build-up index (NDBI) (Luo and Li Citation2014), and hyperspectral flower index (HFI) (Chen et al. Citation2009). Some researchers employ the above or other single indexes for evaluation, but this often cannot truly reflect the complex ecological environment of the region. Xu (Citation2013) proposed the RSEI integrating greenness, humidity, dryness, and temperature, which can quickly and objectively reflect the regional ecological status. Compared with the evaluation of a single index, RSEI can more accurately quantify ecological quality (Xiong et al. Citation2021) and has been widely used (Hu et al. Citation2020; Yan et al. Citation2021; Cui, Zhu, Mi, et al. Citation2022; Yang, Meng, Fu, Zhang, et al. Citation2022; Yang and Li Citation2023). Meanwhile, the emergence of the Google Earth Engine (GEE) platform has brought a lot of convenience to remote sensing image processing (Geng et al. Citation2022; Yang, Tian, et al. Citation2022). It has a geographic database that stores massive images (Zhao et al. Citation2021; Cui, Zhu, Liang, et al. Citation2022; Yang, Meng, Fu, Wang, et al. Citation2022; Campos et al. Citation2023), including Landsat, MODIS, meteorological and socioeconomic data sets, etc. The GEE platform overcomes the low efficiency of traditional remote sensing image processing by writing simple programs online, directly accessing the database, and quickly processing and analyzing geospatial data (Kumar and Mutanga Citation2018; Amani et al. Citation2020; Waleed et al. Citation2023). For example, based on the GEE platform and the RSEI, Zhang, She, et al. (Citation2022) conducted a 20-year long-term analysis of the Chang-Zhu-Tan metropolitan circle. The results found that the ecological environment level in this area is mainly good and excellent, and it is on the rise. Huang et al. (Citation2021) also used the GEE cloud computing platform to build the RSEI to assess and monitor the changes in the quality of the ecological environment in the Lhasa metropolitan area. In addition to discovering ecological changes, the results also confirmed that GEE is an efficient platform that can be used for large-scale environmental monitoring.
On the basis of RSEI, it can reflect the changing trend of regional ecological quality, and in addition, the driving forces of image RSEI can be explored. Geodetector is a model that combines GIS spatial overlay technology with set theory (Wang and Xu Citation2017; Cui, Zhu, Liang, et al. Citation2022), which can reveal the driving factors behind their changes, and can also quantitatively describe the interaction between driving factors (Gao, Kasimu, et al. Citation2020; An et al. Citation2022; Wang et al. Citation2023; Yang and Su Citation2023), so it is widely used in the ecological quality assessment field. For instance, Cui, Zhu, Liang, et al. (Citation2022) delved into the driving forces behind land use/land cover changes within the Shandong section of the Yellow River Basin over the period from 2000 to 2020. Zhu et al. (Citation2020) utilized Geodetector to analyze the influential effects of both natural and human factors on NDVI alterations in the middle reaches of the Heihe River Basin. Likewise, Huo and Sun (Citation2021) employed Geodetector as a foundation to explore the dynamic spatiotemporal variations in vegetation and the factors influencing these changes, selecting the northwest Yunnan Plateau as their case study area. The implementation of Geodetector in these studies showcased their efficacy in unraveling the complexities of geographical phenomena and provided valuable insights for understanding environmental dynamics and decision-making processes.
Situated at the foothills of the Himalayas, Nepal stands among the countries frequently struck by natural hazards (Aryal Citation2014). On April 25, 2015 (11:56 am local time), the epicenter was located in the Gorkha District, and a Ms8.1 earthquake occurred (Okamura et al. Citation2015). Nepal’s complex terrain exacerbates the impact of earthquakes and their secondary disasters on the regional ecological environment. The preservation of a healthy ecological environment is of utmost significance to humanity. Consequently, meticulous monitoring of ecological changes and post-earthquake recovery becomes imperative. However, there is still a lack of understanding regarding the changes in the quality of the ecological environment after the earthquake.
In this study, we first employed the GEE platform as the foundation for utilizing remote sensing data to develop the RSEI, facilitating a swift and accurate assessment of the ecological quality status before and after the 2015 Ms8.1 Nepal earthquake. Moreover, conducting raster analysis on the severe-affected areas enhances our comprehension of post-earthquake recovery dynamics. After that, Moran’s I was employed to delve into the spatial autocorrelation analysis of the ecological environment in Nepal. Finally, the Geodetector model was introduced, considering both natural and social factors, to investigate the driving forces behind changes in ecological quality. Additionally, this study possessed the potential to make significant contributions to the advancement of more comprehensive and effective methods for ecological quality assessment and disaster risk management in Nepal. By combining remote sensing technology and GIScience, this study significantly strengthened the ongoing efforts towards enhancing resilience and promoting sustainable development in the aftermath of seismic events.
2. Study area and data
2.1. Study area
Nepal, located in South Asia (26°22′N-30°27′N, 80°04′E-88°12′E) (Pangali Sharma et al. Citation2019), is a landlocked country bordered by the Tibet Autonomous Region of China in the north and India in the east, south, and west (shown in ). The country has a total area of 147,181 square kilometers, spanning 800 kilometers from east to west and 200 kilometers from north to south. With altitudes ranging from 45 meters in the south to 8,729 meters in the north, Nepal’s terrain varies widely, with the south characterized by low-lying areas and the north marked by high peaks. Nepal is divided into five climatic zones that roughly correspond to altitude. These include the tropical and subtropical zones below 1,200 m, the temperate zone between 1,200 and 2,400 m, the cold zone between 2,400 and 3,600 m, the subarctic zone between 3,600 and 4,400 m, and the Arctic zone above 4,400 m. And, Nepal experiences five seasons: summer, monsoon, autumn, winter, and spring. Annual precipitation varies from 200 mm in parts of the northern Himalayas to 5,500 mm in the south, with a national average of 1,800 mm. Meanwhile, average temperatures in the south can reach as high as 30 °C, whereas in the north they can drop as low as −10 °C. In summary, Nepal is a diverse country with varying topography and climate. Its climatic zones and seasons are largely determined by altitude, and the country experiences a wide range of temperatures and precipitation levels across its different regions.
On April 25, 2015, a devastating Ms8.1 earthquake struck Nepal, known as the Gurkha Earthquake (Liu, Fang, et al. Citation2021). Strong aftershocks with Ms7.0, Ms7.1, and Ms7.5 occurred at 34 min, 24 h, and 17 days, respectively (presented in ). The 2015 Nepal earthquake resulted from the low-angle subduction of the Indian plate towards the Eurasian plate along the Main Himalayan Thrust (MHT) fault zone. The Himalayan tectonic belt is mainly composed of three giant thrust fault systems: the Main Central Thrust (MCT) Zone, the Main Boundary Thrust (MBT) Zone and the Main Front Thrust (MFT) Zone from north to south, and the fault zone has a high frequency of seismic activity. Among them, there have been additional earthquakes in Nepal, such as the 1505 Ms8.2 Lo Mustang earthquake, the 1833 Ms8.0 Kathmandu earthquake, and the 1934 Ms8.1 Bihar earthquake. Of note, the 2015 Nepal earthquake stands as the largest recorded earthquake in Nepal since 1934 (Okamura et al. Citation2015) and left an indelible impact on the affected regions. More than 8,790 people died in the earthquake and 22,000 were injured (Earthquake Citation2015). The total economic loss is around $10 billion, or about half of Nepal’s gross domestic product, the report said (Goda et al. Citation2015). The earthquake’s epicenter was located in Gurkha District, approximately 80 kilometers northwest of the capital city, Kathmandu. The earthquake had a focal depth of 15 kilometers and reached a high seismic intensity level of Ⅹ in the severe-affected areas. The rupture of the earthquake extended from west to east (Goda et al. Citation2015), resulting in powerful tremors that were felt in Kathmandu and the surrounding cities. The Central Development Region and Western Development Region of Nepal, which were former administrative divisions, were among the severe-affected areas by the earthquake.
Figure 2. Seismic intensity map of 2015 Nepal earthquake
(Sun and Yan, Citation2015).

2.2. Data collection
Multi-source data was employed in this study, including remote sensing data, digital elevation models (DEM), precipitation data, population density data, and nighttime lights data. Remote sensing data played a crucial role in this study, and the MODIS dataset was selected due to its high temporal resolution, making it well-suited for large-scale monitoring purposes (Xu et al. Citation2019; Lu et al. Citation2022). Specifically, the MODIS dataset’s MOD09A1, MOD11A2, and MOD13A1 subsets were selected for preprocessing on the GEE platform, with July to September being used to generate four indicators and the RSEI. Within this timeframe, T0 represents the pre-earthquake period in 2014, T1 represents the year 2015 just after the earthquake, and T2 to T4 represents the subsequent post-disaster recovery stage from 2016 to 2018. Apart from remote sensing data, this study incorporated DEM and precipitation data, which were also processed using the GEE platform. The elevation data was utilized to calculate slope and aspect information. As a result, six factors—elevation, slope, aspect, precipitation, nighttime lights, and population density—were employed to explore the driving forces behind the recovery of the RSEI. presents comprehensive details regarding the study data, while illustrates the spatial distribution of the selected impact factors.
Table 1. Details of datasets.
3. Methodology
The flowchart of this study is shown in . This study used MODIS datasets as the basis for calculating four component indexes—greenness, dryness, humidity, and temperature—using the GEE cloud computing platform. Principal component analysis (PCA) was then conducted to generate the RSEI to monitor the spatiotemporal changes of the ecological environment quality before and after the 2015 Nepal earthquake. In order to explore the recovery of the ecological environment quality after the earthquake, a grid analysis was carried out for the RSEI in the severe-affected areas. Additionally, we analyzed the spatial distribution of RSEI from T0 to T4 with the help of Moran’s I index. Finally, the driving factors behind the changes in RESI during the recovery period were explored based on the Geodetector model.
3.1. Methods of RSEI
RSEI is a quantitative evaluation method of the ecological environment that integrates greenness, humidity, dryness, and temperature and is completely based on remote sensing information (Xu et al. Citation2019). To represent the greenness index, we chose NDVI, which is closely related to plant biomass, leaf area index, and vegetation coverage (Goward et al. Citation2002) and is the most widely used vegetation index (de Araujo Barbosa et al. Citation2015; Mishra et al. Citation2015). The humidity index in this study is represented by the third component WET in the tasseled cap transformation, which reflects the water content of water, soil, and vegetation (Crist Citation1985). In urban areas, normalized difference built-up and soil index (NDBSI) constructed by bare soil index and building index is used to represent dryness since building land can also have an impact on the dryness of the surface. Lastly, LST is the most common index of urban heat and is employed as the temperature index in this study (Nichol Citation2005). The RSEI can be expressed as a function of these four indicators as follows:
(1)
(1)
We calculate the four indicators mentioned above using MODIS data and GEE platform. NDVI is calculated from the MOD13A1 dataset, while LST is calculated from the MOD11A2 dataset. WET and NDBSI are calculated by MOD09A1. The calculation formula of WET (Lobser and Cohen Citation2007) and NDBSI (Yuan et al. Citation2021) is as follows:
(2)
(2)
(3)
(3)
Where to
denote bands 1 to 7 corresponding to the red, NIR1, blue, green, NIR2, short-wavelength infrared 1 (SWIR1), and SWIR2 bands of the MODIS image, respectively.
To avoid any weight imbalances among the four indicators mentioned above, which have different dimensions, normalization was necessary before conducting the PCA. This ensured that the range of all indexes was unified between 0 and 1 (Jing et al. Citation2020). After normalization, a PCA was performed, and the first principal component (PC1) was extracted to calculate the RSEI. For comparative analysis, the RSEI is normalized again as follows (Xiong et al. Citation2021). After normalization, RSEI is evenly divided into five levels at intervals of 0.2, including Poor: 0-0.2; Fair: 0.2-0.4; Moderate: 0.4-0.6; Good: 0.6-0.8 and Excellent: 0.8-1.
(4)
(4)
(5)
(5)
(6)
(6)
Where is the image normalization result;
is the numerical pixel value of the image;
and
are the maximum and minimum values of the image pixel, respectively;
is the first principal component of the four indicators;
indicates the initial ecological index value;
and
are the maximum and minimum
value, respectively.
3.2. Spatial correlation analysis
3.2.1. Global Moran’s I
Global Moran’s I is a widely used statistic in global correlation analysis. It is primarily utilized to describe the average degree of correlation between all spatial units and their surrounding areas within the entire study area (Zhang, Zhao, et al. Citation2022), and the formula is as follows:
(7)
(7)
Where is the number of spatial elements,
presents the variable of the study object,
donates the binary weight matrix of the general cross-production statistic,
means the average of the property values of the study object.
The value range of is
When
means that all regional attribute values have a positive correlation in space; when
indicates that all regional attribute values are randomly distributed without spatial correlation; when
presents that all regional attribute values have negative spatial correlation (Luo et al. Citation2022).
3.2.2. Local Moran’s I
Local Indicators of Spatial Association (LISA) can further determine the degree of correlation between the RSEI of each spatial unit and the RSEI of its neighbors (Anselin Citation1995). Meanwhile, it can indicate the location and degree of its spatial aggregation or dispersion. The formula of the Local Moran’s I is as follows:
(8)
(8)
Where
, and
involved in the calculation formula all have the same parameters as formula (Equation7
(7)
(7) ).
3.3. Geodetector model
The Geodetector is a statistical method that is used to detect spatial heterogeneity and reveal its driving factors by detecting spatial differentiation (Wang et al. Citation2010; Wu et al. Citation2015). This model has been extensively employed in various fields, such as geography and ecology. Here, we focus on the detection of the factors influencing ecological environment changes and their interactions using the Geodetector model.
Factor detector
This module is capable of quantitatively detecting the spatial heterogeneity of the dependent variable and the explanatory power of the influencing factor
on the spatially heterogeneous characteristics of RSEI changes (Zhang, Jiang, et al. Citation2022);
can be expressed mathematically as follows (Wang et al. Citation2021; Cai et al. Citation2023; Liu, Liu, Qiu, et al. Citation2023):
Interaction detector
The module can identify the interactive effect of two driving factors on RSEI (Zhu et al. Citation2020). Assuming that
and
are the explanatory powers of the influence factors
and
respectively,
is the explanatory power of the two factors when they interact with each other, and five patterns of influence exist (as presented in ).
Table 2. Types of interaction between two covariates.
4. Results
4.1. Ecological quality assessment before and after the 2015 Nepal earthquake
4.1.1. Principal component analysis of the ecological environment index
Based on the aforementioned formula principles, four indicators that were used to construct RSEI in remote sensing image were extracted, and their corresponding mean values are shown in . There are differences in the trend of changes in the four indicators from T0 to T4. Among them, the changes in NDVI indicate that vegetation continued to decline after the earthquake, until the NDVI decreased by 8.18% at T2, and vegetation began to recover and grow after T2. The average WET representing humidity increased by 8.13% at T1 after the earthquake and showed a downward trend during the recovery period. NDBSI represents dryness, and there is no obvious pattern of dryness fluctuation from T0 to T4. The average LST increased slightly at T1 after the earthquake, with an increase rate of 0.7761%, but gradually decreased during the recovery period. Comparing the changes in the four indicators before the earthquake (T0) and in the third year after recovery (T4), it was found that the mean NDVI increased by 7.4599%, indicating a positive change in vegetation. On the other hand, the mean WET decreased by 10.7575%, implying a decrease in humidity. Furthermore, NDBSI and LST, which are negatively associated with the ecological environment, decreased by 2.5150% and 6.6774%, respectively.
Table 3. Statistics of four indicators from T0 to T1.
shows the results of the principal component analysis. The principal component 1 (PC1) of each year exhibits a consistent sign pattern among the variables NDVI, WET, NDBSI, and LST. As shown in , the loadings of NDVI and WET in PC1 exhibit positive values, indicating their positive contribution to the ecology. Conversely, the loadings of LST and NDBSI have negative values, suggesting their negative impact on the ecology, as expected (Xu and Deng Citation2022). In contrast, PC2, PC3, and PC4 do not demonstrate a regular pattern among the symbols of the four indicators. Moreover, PC1 has a contribution rate exceeding 70% for each year, implying that it captures the majority of the information. Consequently, utilizing PC1 to construct the RSEI is deemed more appropriate for this study.
Table 4. PCA results of RSEI from T0 to T1.
4.1.2. The spatiotemporal variation characteristics of RSEI
shows the spatial distribution of RSEI grades from T0 to T4. The RSEI grades are indicators of ecological environment quality, with green indicating high quality, dark yellow representing medium quality, and light colors indicating poor quality. As can be seen from the distribution of RSEI in Nepal is high in the south, low in the north region, and transitional in the middle region. Notably, in T1 (shown in ), there is a noticeable decrease in green colors and an increase in light colors near the epicenter. This indicates that the quality of the ecological environment in this area is disturbed by the earthquake. To enhance the visual representation of the changes in the ecological environment before and after the earthquake, we calculated the annual mean RSEI and performed area statistics based on different grades of RSEI. These findings are presented in . The average RSEI values from T0 to T1 were 0.6932, 0.6707, 0.6480, 0.6862, and 0.7232, respectively. The results demonstrate that the overall ecological environment in Nepal showed a pattern of decreasing from T0 to T2 and then increasing from T2 to T4 over time. The decline of the RSEI value from T0 to T1 indicates that the ecological environment in Nepal has been affected by the earthquake and deteriorated. The intensity of the earthquake was high, and after the earthquake, there were many aftershocks and secondary disasters, which increased the ecological vulnerability of the area involved. In addition, the heavy rain at T2 in Nepal caused large-scale floods and debris flow disasters, which caused the average RSEI to continue to decline. It was only in the second year after the earthquake that a rebound in the ecological environment was observed. Through the calculation of the area occupied by each level of RSEI, it was observed that from T0 to T4, the Excellent grade consistently accounted for over 40% of the total area, maintaining the highest proportion each year. Conversely, the Poor grade consistently had the lowest area, never exceeding 5% of the total area. However, after the occurrence of the earthquake in T1, there was a decrease in the proportion of areas in the Excellent grade from 48% to 40%, accompanied by an increase in the proportion of areas in the Poor grade. One year after the earthquake, there was a simultaneous increase in the proportions of areas in the Poor and Fair grades, exceeding a total of 30%. At T3 and T4, the proportion of areas in the Excellent grade increased to 46% and 51%, respectively, while the proportion of areas with moderate or lower ecological environment levels decreased. Comparing the numerical values of the overall regional statistical data, it becomes evident that the ecological quality during the post-earthquake recovery period consistently improves, eventually reaching the level observed before the earthquake. These findings offer valuable insights into the ecological environment quality in Nepal from T0 to T4, as well as the changes observed during the post-earthquake recovery phase.
4.1.3. Analysis of RSEI transfer
The transfer matrix provides a quantitative representation of the relationship between different grades of the RSEI in different periods, offering a comprehensive assessment of the impact of earthquakes on Nepal’s ecological environment and the changes during the post-earthquake recovery phase. and present the statistical results of RSEI level transfers from T0 to T4 in Nepal. The findings indicate that there was mutual conversion between different RSEI levels, with overall frequent conversions observed. From the data displayed, it is evident that the area where the ecological environment changed from Excellent to Good was 19,556.046 km2 from T0 to T1, representing the highest value for this type of transformation. Moreover, the amount of transformation area for this type decreased gradually in subsequent years. Additionally, the results reveal a decreasing trend in the area transitioning to the Poor ecological grade from T0 to T4, reaching a minimum transfer amount of 0 km2. This decrease can be attributed to the significant damage caused by the earthquake to areas with initially Excellent ecological conditions. During the recovery period, the transfer of areas with Excellent ecological environments gradually decreased, and the overall ecological environment started to stabilize.
Table 5. Statistics of RSEI level transfer types and area in Nepal from T0 to T4.
4.2. Characteristics of ecological environment recovery after the 2015 Nepal earthquake
To conduct a detailed analysis of the changes and recovery of the ecological environment after the Nepal earthquake, we performed the grid analysis specifically focusing on the RSEI in the severe-affected areas. and present the results of post-earthquake damage and recovery at the administrative district level in Nepal. The degree of damage was derived from the analysis of RSEI changes at T0 and T1, and it is classified using the natural break point method. In , larger values indicate a smaller degree of damage, while smaller values indicate a greater degree of damage. On the other hand, the degree of recovery is generated based on the RSEI values at T0 and T2, T3, and T4, respectively. Values closer to 1 indicate a higher post-earthquake recovery level, as they closely approach the RSEI value at T0. Conversely, smaller values in indicate a lower recovery level, signifying that the ecological environment has not yet fully recovered from the impact of the earthquake.
It is evident that the ecological environment of Gorkha District, where the earthquake’s epicenter was located, experienced severe damage. Additionally, districts surrounding Gorkha were also affected to varying degrees, with most of the damage falling into grades IV and V. As we move outward from the epicenter, the degree of damage tends to decrease. In general, the southwest and northeast of the development region suffered relatively minor damage, while the central region near the epicenter faced more serious damage. illustrates variations in the level of recovery across different districts. Notably, Kaski District and Manang District experienced the same degree of damage, yet Kaski District demonstrated a higher recovery level during the same recovery period. This observation highlights that Kaski District has a faster recovery speed compared to Manang District. In fact, the degree and speed of recovery not only reflect the changes in the ecological environment of a region but also indirectly indicate the region’s ability to withstand hazards. This highlights the importance of decision-makers considering the specific conditions of each region when formulating ecological and environmental protection measures. When looking at the two development regions as a whole, it becomes apparent that areas with less damage return a higher degree of recovery. Referring to , it is evident that after three years of recovery, both the Central and West development regions have achieved a relatively considerable recovery.
5. Discussion
5.1. Spatial autocorrelation analysis
5.1.1. Global spatial autocorrelation
We established a 2 km × 2km grid within the study area to investigate the potential spatial correlation of the RSEI in Nepal. Subsequently, Moran’s I index and LISA were utilized to analyze the spatial autocorrelation of RSEI. The results of the analysis, including Moran’s I index and scatter diagram, are presented in and . Over the period from T0 to T4, the p-value of 0.0010 confirms the scientific validity of the spatial autocorrelation analysis. The Global Moran’s I values for T0 to T4 are 0.8742, 0.8865, 0.8824, 0.8971, and 0.9217, respectively, indicating a clustering phenomenon in the spatial distribution of RSEI during these periods. Notably, the Global Moran’s I value exhibits an increasing trend, with the spatial correlation of RSEI in Nepal being the strongest at T4. As shown in , the annual scatter points are mainly clustered in the first and third quadrants, indicating a positive spatial correlation in Nepal’s RSEI from T0 to T4.
Table 6. Global Moran’s I of RSEI in Nepal from T0 to T4.
5.1.2. Local spatial autocorrelation
In order to visually depict the spatial distribution pattern of the RSEI in Nepal and further analyze the spatial correlation between various geographical units, a LISA cluster diagram was created using a scatter plot, as illustrated in . The High-High clustering areas from T0 to T4 are primarily concentrated in low-altitude regions in southern Nepal. On the other hand, the Low-Low clustering areas are mainly distributed in high-altitude regions in northern Nepal. The areas with insignificant distribution are mainly found in central Nepal.
5.2. Analysis of driving mechanism of ecological environment quality
Understanding the driving forces behind the recovery process is crucial for developing effective strategies to mitigate the negative impacts of future hazards. This contributes significantly to developing a more comprehensive comprehension of the intricate relationship between natural and social factors in the ecological environment’s recovery after a major hazard. Ultimately, this can be harnessed to develop more efficient disaster risk management strategies in Nepal.
5.2.1. Single-factor detection analysis
The ecological environment is characterized by complexity and spatial heterogeneity, and its distribution and changes are influenced by a multitude of factors. To investigate the driving forces behind the post-earthquake ecological environment recovery, we employed six key factors encompassing both natural and socioeconomic aspects. These factors, including elevation, slope, aspect, precipitation, population density, and nighttime lights, were selected to examine their impact on RSEI changes, using the Geodetector model. These factors were classified using the natural breakpoint method. presents the results of the single-factor detection analysis. From , it is evident that only four factors consistently passed the significance test, and the explanatory power of natural factors is greater than that of social factors. These factors, namely population density, elevation, slope, and precipitation, were identified as having significant driving forces on the change of RSEI after the 2015 Nepal earthquake. Among these four factors, elevation exhibited the most substantial contribution rate, indicating that post-earthquake RSEI in Nepal is primarily influenced by changes in elevation. The corresponding q-values for elevation at T2, T3, and T4 were 0.5742, 0.6886, and 0.7835, respectively. Additionally, the precipitation factor also exerted a notable impact, with q-values of 0.1566, 0.2721, and 0.2734 at T2, T3, and T4, respectively. The trend suggests that the influence of elevation and precipitation on the quality of the post-earthquake ecological environment has been increasing over time. The slope factor, as the third largest driving factor, has q-values of 0.0881, 0.0778, and 0.0729 at T2, T3, and T4, respectively, with a contribution rate of less than 10%. Lastly, the impact of population density on the changes in RSEI during the recovery period is very low, with no more than 5%.
Table 7. Explanatory power of the factors to the RSEI detection for T2 to T4.
5.2.2. Interaction detection analysis
Changes in the ecological environment result from the combined influence of various factors, including natural elements and human activities. The interaction among these driving factors plays a crucial role in shaping RSEI in Nepal. Therefore, it is of utmost importance to understand the interactive influence and the mode of action of these factors behind RSEI changes. Interaction detection helps to reveal individual interactions between two factors.
As depicted in , the results illustrate that the interaction effect of any two factors is more significant than that of a single factor. The types of factor interaction are mainly double synergy and nonlinear synergy. These outcomes highlight that the changes in RSEI after the earthquake are not solely attributed to the individual effects of population density, elevation, slope, and precipitation, but also to the interactions between these factors. Particularly noteworthy is the interaction between elevation and precipitation, which has the most substantial impact on RSEI, accounting for 63.41% to 78.63% of the observed changes. Moreover, the interaction between elevation and slope also exerts a significant influence on RSEI, contributing to over 57% of the changes. However, the single factor of the slope has a comparatively minimal effect on RSEI variations. In summary, the interaction between elevation and precipitation has the most substantial impact, followed by the interactions involving elevation and slope, and elevation and population density.
According to the results of the Geodetector analysis, it is suggested that the difference in recovery rates between Kaski District and Manang District can be attributed to variations in natural conditions, specifically the elevation and precipitation. These findings underscore the crucial significance of taking into account the interactions among multiple factors when studying the changes in RSEI during the recovery process. In light of these insights, the local government should enhance the environmental protection policy system and strive for a harmonious balance between ecological governance and social development. Implementing appropriate and moderate measures to develop ecological civilization will lead to the achievement of a win-win scenario, wherein the regional ecological and economic environments are both improved.
5.3. Strengths and limitations
This study employed the RSEI as an ecological environment quality assessment indicator and utilized remote sensing and GIScience methods for post-earthquake recovery monitoring. In contrast to previous studies (Jiao et al. Citation2014; Yang and Qi Citation2017), we utilized RSEI instead of a single remote sensing index to detect changes in the ecological environment. RSEI is a fast evaluation method entirely based on remote sensing, and the PCA used in its construction can avoid human subjectivity. Thus, the application of RSEI in this study to monitor the changes and recovery of the ecological environment in Nepal after hazards is scientifically and logically sound. However, the application of RSEI holds limitations in terms of regional environmental monitoring. Optical remote sensing images are often affected by non-biological factors that can negatively impact the inversion of RSEI and ecological monitoring performance. For large study areas, it is always a challenging task that obtaining the complete high-resolution Landsat image data without cloud or snow. Therefore, we used MODIS data products with lower resolution, which applied methods such as quality control and perspective field angle to improve data quality to some extent. Additionally, previous studies revealed that RSEI is sensitive to different image acquisition times, so RSEI was combined with the Continuous Change Detection and Classification (CCDC) algorithm and a time-series RSEI was plotted (Sun et al. Citation2022). If more accurate quantification of changes in the regional ecological environment is needed, future research should introduce high-resolution images and algorithms to improve the accuracy of RSEI inversion.
To ensure the reliability of earthquake recovery monitoring, this study focuses on the three years after the earthquake, taking into account the severe flooding in Nepal in 2019, which marks the fourth year post-earthquake. Moreover, this study conducted a more comprehensive analysis and statistical assessment of the RSEI based on the transfer matrix. We further performed grid operations and displayed administrative regions to gain valuable insights into the post-earthquake damage and recovery degree in Nepal. Finally, Moran’s I index was introduced to explore the strong spatial correlation as evidence for non-random patterns in Nepal’s RSEI, and the driving forces behind the RSEI changes after the Nepal earthquake were investigated using the Geodetector model. While we acknowledged data limitations in the selection of influencing factors, future studies should pay attention to the diversity of factor selection to more comprehensively reflect the driving forces of post-disaster recovery. This will provide a solid theoretical basis for relevant government departments and decision-makers to effectively build and develop the ecological environment. In conclusion, we focused on the three-year recovery period, in-depth analysis, and spatial autocorrelation exploration, as well as the application of the Geodetector model, which contributes valuable insights to post-earthquake recovery monitoring and offers crucial guidance for ecological environment development in Nepal.
6. Conclusion
In this study, we analyzed the changes in the quality of the ecological environment in Nepal from 2014 to 2018, with a particular focus on investigating the impact and extent of post-recovery after the 2015 Nepal earthquake. Initially, we employed the RSEI generated through the integration of GEE to assess the overall changes in Nepal’s ecological environment. Subsequently, we utilized a transfer matrix to examine the shifts observed among different RSEI grades. Moreover, we conducted a detailed analysis of the extent of damage and subsequent recovery in the severely affected areas following the earthquake. Finally, we employed spatial autocorrelation analysis and utilized GIScience to explore the driving forces responsible for the observed changes in Nepal’s RSEI. The findings of this study can be concluded as follows:
The analysis of mean RSEI values reveals a noticeable delay in the recovery of the regional ecological environment after the earthquake, with signs of an improvement in the ecological environment becoming evident from the second year of recovery. Notably, the mean RSEI value of Nepal surpassed the pre-earthquake mean value in the third year of recovery, indicating a positive trend in ecological recovery. Furthermore, during the study period, frequent conversions between different ecological grades were observed. However, the conversion from high to low ecological grades exhibited a declining trend, while the area occupied by high ecological grades displayed an increasing trend. This suggests an overall improvement in the ecological conditions over time, with fewer regions experiencing degradation and a growing portion of the area demonstrating higher ecological quality.
From the district-level perspective, the districts within the Central Development Region and Western Development Region of Nepal experienced varying degrees of damage. However, the analysis of the recovery degree shows that the ecological environment of each district consistently improved throughout the recovery period. By the third year of recovery, most areas in the severe-affected areas had better recovered their ecological conditions to or even surpassed the levels observed before the earthquake. This positive trend in the overall recovery process indicates significant progress and recovery in the severe-affected areas.
Based on the analysis results using GIScience, a robust positive correlation was observed in the spatial distribution of RSEI in Nepal. The high-high agglomeration primarily occurred at high altitudes, while the low-low agglomeration was mainly concentrated at lower elevations in Nepal. This suggests that areas with similar ecological conditions tend to cluster together based on altitude. Additionally, this study delved further into the driving forces behind the RSEI changes by conducting single-factor and interaction tests. The results indicated that elevation, precipitation, and their interaction were identified as the primary factors influencing RSEI. These findings emphasize the significance of both elevation and precipitation in shaping the ecological environment’s recovery, and the interaction between these two factors plays a crucial role in influencing RSEI changes in Nepal.
To further enhance the achievements of ecological recovery and disaster risk management after the 2015 Nepal earthquake, relevant departments should optimize ecological protection measures on an existing basis. To begin with, implement land recovery and vegetation recovery initiatives to stabilize the soil, mitigate soil erosion, and facilitate ecosystem rehabilitation. Furthermore, it is suggested to establish and reinforce natural reserves and sanctuaries to safeguard the affected ecosystems and vulnerable species post-earthquake. Lastly, it is imperative to enhance public awareness and understanding of disaster risks, environmental preservation, and sustainable development through informative campaigns, educational programs, and training activities aimed at fostering environmental consciousness.
This study conducted a comprehensive monitoring of the ecological environment quality both before and after the 2015 Nepal earthquake, thereby generating valuable insights into the changes that transpired during the subsequent recovery phase. The obtained findings provide a profound understanding of the recovery process, thereby establishing a scientific foundation for relevant governmental departments to enhance their efforts in safeguarding and advancing ecological civilization and disaster risk management in the future. Furthermore, the outcomes of this study can serve as a theoretical framework for localizing Sustainable Development Goal (SDG) 11, which specifically targets sustainable cities and communities. By promoting the realization of SDG 11, this study contributes to the broader agenda of achieving sustainable development, consequently fostering a more resilient and ecologically balanced future for Nepal.
Acknowledgments
We sincerely thank the anonymous reviewers and associated editors for their valuable and constructive comments and suggestions that helped to improve this paper.
Disclosure statement
The authors report there are no competing interests to declare.
Data availability statement
The data that support the findings of this study are available upon reasonable request.
Additional information
Funding
References
- Amani M, Ghorbanian A, Ahmadi SA, Kakooei M, Moghimi A, Mirmazloumi SM, Moghaddam SHA, Mahdavi S, Ghahremanloo M, Parsian S, et al. 2020. Google earth engine cloud computing platform for remote sensing big data applications: a comprehensive review. IEEE J Sel Top Appl Earth Observations Remote Sens. 13:5326–5350. doi:10.1109/JSTARS.2020.3021052.
- An M, Xie P, He W, Wang B, Huang J, Khanal R. 2022. Spatiotemporal change of ecologic environment quality and human interaction factors in three gorges ecologic economic corridor, based on RSEI. Ecol Indicators. 141:109090. doi:10.1016/j.ecolind.2022.109090.
- Anselin L. 1995. Local indicators of spatial association—LISA. Geogr Anal. 27(2):93–115. doi:10.1111/j.1538-4632.1995.tb00338.x.
- Aryal KR. 2014. Disaster vulnerability in Nepal. Int J Disaster Risk Reduc. 9:137–146. doi:10.1016/j.ijdrr.2014.05.009.
- Cai Z, Zhang Z, Zhao F, Guo X, Zhao J, Xu Y, Liu X. 2023. Assessment of eco-environmental quality changes and spatial heterogeneity in the Yellow River Delta based on the remote sensing ecological index and geo-detector model. Ecol Informatics. 77:102203. doi:10.1016/j.ecoinf.2023.102203.
- Campos JC, Garcia N, Alírio J, Arenas-Castro S, Teodoro AC, Sillero N. 2023. Ecological Niche Models using MaxEnt in Google Earth Engine: evaluation, guidelines and recommendations. Ecol Informatics. 76:102147. doi:10.1016/j.ecoinf.2023.102147.
- Chen J, Shen M, Zhu X, Tang Y. 2009. Indicator of flower status derived from in situ hyperspectral measurement in an alpine meadow on the Tibetan Plateau. Ecol Indicators. 9(4):818–823. doi:10.1016/j.ecolind.2008.09.009.
- Contreras D, Blaschke T, Tiede D, Jilge M. 2016. Monitoring recovery after earthquakes through the integration of remote sensing, GIS, and ground observations: the case of L’Aquila (Italy). Cartogr Geogr Informat Sci. 43(2):115–133. doi:10.1080/15230406.2015.1029520.
- Cooner AJ, Shao Y, Campbell JB. 2016. Detection of urban damage using remote sensing and machine learning algorithms: revisiting the 2010 Haiti earthquake. Remote Sens. 8(10):868. doi:10.3390/rs8100868.
- Crist EP. 1985. A TM tasseled cap equivalent transformation for reflectance factor data. Remote Sens Environ. 17(3):301–306. doi:10.1016/0034-4257(85)90102-6.
- Cui J, Zhu M, Liang Y, Qin G, Li J, Liu Y. 2022. Land use/land cover change and their driving factors in the Yellow River Basin of Shandong Province based on google earth Engine from 2000 to 2020. IJGI. 11(3):163. doi:10.3390/ijgi11030163.
- Cui J, Zhu M, Mi H, Liu Y. 2022. Evaluation of eco-environmental quality and analysis on spatio-temporal variation in Jinan, China. Pol J Environ Stud. 31(2):1061–1072. doi:10.15244/pjoes/140297.
- de Araujo Barbosa CC, Atkinson PM, Dearing JA. 2015. Remote sensing of ecosystem services: a systematic review. Ecol Indicators. 52:430–443. doi:10.1016/j.ecolind.2015.01.007.
- Earthquake N. 2015. Post disaster needs assessment (vol. A). Kathmandu: Government of Nepal, Nepal Planning Commission.
- Gao S, Chen Y, Liang L, Gong A. 2020. Post-earthquake night-time light piecewise (PNLP) pattern based on NPP/VIIRS night-time light data: a case study of the 2015 Nepal earthquake. Remote Sens. 12(12):2009. doi:10.3390/rs12122009.
- Gao P, Kasimu A, Zhao Y, Lin B, Chai J, Ruzi T, Zhao H. 2020. Evaluation of the temporal and spatial changes of ecological quality in the Hami oasis based on RSEI. Sustainability. 12(18):7716. doi:10.3390/su12187716.
- Geng J, Yu K, Xie Z, Zhao G, Ai J, Yang L, Yang H, Liu J. 2022. Analysis of spatiotemporal variation and drivers of ecological quality in Fuzhou based on RSEI. Remote Sens. 14(19):4900. doi:10.3390/rs14194900.
- Ghaffarian S, Kerle N, Filatova T. 2018. Remote sensing-based proxies for urban disaster risk management and resilience: a review. Remote Sens. 10(11):1760. doi:10.3390/rs10111760.
- Ghaffarian S, Rezaie Farhadabad A, Kerle N. 2020. Post-disaster recovery monitoring with google earth engine. Appl Sci. 10(13):4574. doi:10.3390/app10134574.
- Ghaffarian S, Roy D, Filatova T, Kerle N. 2021. Agent-based modelling of post-disaster recovery with remote sensing data. Int J Disaster Risk Reduc. 60:102285. doi:10.1016/j.ijdrr.2021.102285.
- Goda K, Kiyota T, Pokhrel RM, Chiaro G, Katagiri T, Sharma K, Wilkinson S. 2015. The 2015 Gorkha Nepal earthquake: insights from earthquake damage survey. Front Built Environ. 1:8. doi:10.3389/fbuil.2015.00008.
- Goward SN, Xue Y, Czajkowski KP. 2002. Evaluating land surface moisture conditions from the remotely sensed temperature/vegetation index measurements: an exploration with the simplified simple biosphere model. Remote Sens Environ. 79(2-3):225–242. doi:10.1016/S0034-4257(01)00275-9.
- Hu X, Wen-Ke B, Xin D. 2020. Evaluation of regional eco-environmental quality before and after earthquake in Jiuzhaigou County, Sichuan Province. Chin J Ecol. 39(3):969.
- Huang H, Chen W, Zhang Y, Qiao L, Du Y. 2021. Analysis of ecological quality in Lhasa Metropolitan Area during 1990–2017 based on remote sensing and Google Earth Engine platform. J Geogr Sci. 31(2):265–280. doi:10.1007/s11442-021-1846-8.
- Huo H, Sun C. 2021. Spatiotemporal variation and influencing factors of vegetation dynamics based on Geodetector: a case study of the northwestern Yunnan Plateau, China. Ecol Indicators. 130:108005. doi:10.1016/j.ecolind.2021.108005.
- Jiao Q, Zhang B, Liu L, Li Z, Yue Y, Hu Y. 2014. Assessment of spatio-temporal variations in vegetation recovery after the Wenchuan earthquake using Landsat data. Nat Hazards. 70(2):1309–1326. doi:10.1007/s11069-013-0875-8.
- Jing Y, Zhang F, He Y, Kung H-t, Johnson VC, Arikena M. 2020. Assessment of spatial and temporal variation of ecological environment quality in Ebinur Lake Wetland National Nature Reserve, Xinjiang, China. Ecol Indicators. 110:105874. doi:10.1016/j.ecolind.2019.105874.
- Kane JC, Luitel NP, Jordans MJD, Kohrt BA, Weissbecker I, Tol WA. 2018. Mental health and psychosocial problems in the aftermath of the Nepal earthquakes: findings from a representative cluster sample survey. Epidemiol Psychiatr Sci. 27(3):301–310. doi:10.1017/S2045796016001104.
- Kumar L, Mutanga O. 2018. Google Earth Engine applications since inception: usage, trends, and potential. Remote Sens. 10(10):1509. doi:10.3390/rs10101509.
- Li X, Li Z, Yang J, Li H, Liu Y, Fu B, Yang F. 2020. Seismic vulnerability comparison between rural Weinan and other rural areas in Western China. Int J Disaster Risk Reduc. 48:101576. doi:10.1016/j.ijdrr.2020.101576.
- Liao W, Jiang W. 2020. Evaluation of the spatiotemporal variations in the eco-environmental quality in China based on the remote sensing ecological index. Remote Sens. 12(15):2462. doi:10.3390/rs12152462.
- Lin C-Y, Lo H-M, Chou W-C, Lin W-T. 2004. Vegetation recovery assessment at the Jou-Jou Mountain landslide area caused by the 921 Earthquake in Central Taiwan. Ecol Model. 176(1-2):75–81. doi:10.1016/j.ecolmodel.2003.12.037.
- Liu C, Fang D, Zhao L. 2021. Reflection on earthquake damage of buildings in 2015 Nepal earthquake and seismic measures for post-earthquake reconstruction. Structures. 30:647–658. doi:10.1016/j.istruc.2020.12.089.
- Liu Y, Liu W, Lin Y, Zhang X, Zhou J, Wei B, Nie G, Gross L. 2023. Urban waterlogging resilience assessment and postdisaster recovery monitoring using NPP-VIIRS nighttime light data: a case study of the ‘July 20, 2021’heavy rainstorm in Zhengzhou City, China. Int J Disaster Risk Reduc. 90:103649. doi:10.1016/j.ijdrr.2023.103649.
- Liu Y, Liu W, Qiu P, Zhou J, Pang L. 2023. Spatiotemporal evolution and correlation analysis of carbon emissions in the nine provinces along the Yellow River since the 21st century using nighttime light data. Land. 12(7):1469. doi:10.3390/land12071469.
- Liu Y, Li Z, Wei B, Li X, Fu B. 2019. Seismic vulnerability assessment at urban scale using data mining and GIScience technology: application to Urumqi (China). Geomatics Nat Hazards Risk. 10(1):958–985. doi:10.1080/19475705.2018.1524400.
- Liu Y, So E, Li Z, Su G, Gross L, Li X, Qi W, Yang F, Fu B, Yalikun A, et al. 2020. Scenario-based seismic vulnerability and hazard analyses to help direct disaster risk reduction in rural Weinan, China. Int J Disaster Risk Reduc. 48:101577. doi:10.1016/j.ijdrr.2020.101577.
- Liu Y, Zhang X, Liu W, Lin Y, Su F, Cui J, Wei B, Cheng H, Gross L. 2023. Seismic vulnerability and risk assessment at the urban scale using support vector machine and GIScience technology: a case study of the Lixia District in Jinan City, China. Geomatics Nat Hazards Risk. 14(1):2173663. doi:10.1080/19475705.2023.2173663.
- Liu Y, Zhang W, Chen X, Yu M, Sun Y, Meng F, Fan X. 2021. Landslide detection of high-resolution satellite images using asymmetric dual-channel network. Paper presented at the 2021 IEEE International Geoscience and Remote Sensing Symposium IGARSS.
- Lobser SE, Cohen WB. 2007. MODIS tasselled cap: land cover characteristics expressed through transformed MODIS data. Int J Remote Sens. 28(22):5079–5101. doi:10.1080/01431160701253303.
- Lu Z, Leigang S, Junjing L, Quanhong X, Jianfeng L, Xiaoqian M. 2022. MODIS-based comprehensive assessment and spatial-temporal change monitoring of ecological quality in Beijing-Tianjin-Hebei region. Remote Sens Nat Resour. 34(2):203–214.
- Luo X, Li W. 2014. Scale effect analysis of the relationships between urban heat island and impact factors: case study in Chongqing. J Appl Remote Sens. 8(1):084995. doi:10.1117/1.JRS.8.084995.
- Luo M, Zhang S, Huang L, Liu Z, Yang L, Li R, Lin X. 2022. Temporal and spatial changes of ecological environment quality based on RSEI: a case study in Ulan Mulun River Basin, China. Sustainability. 14(20):13232. doi:10.3390/su142013232.
- Mishra NB, Crews KA, Neeti N, Meyer T, Young KR. 2015. MODIS derived vegetation greenness trends in African Savanna: deconstructing and localizing the role of changing moisture availability, fire regime and anthropogenic impact. Remote Sens Environ. 169:192–204. doi:10.1016/j.rse.2015.08.008.
- Nichol J. 2005. Remote sensing of urban heat islands by day and night. Photogramm Eng Remote Sens. 71(5):613–621. doi:10.14358/PERS.71.5.613.
- Okamura M, Bhandary NP, Mori S, Marasini N, Hazarika H. 2015. Report on a reconnaissance survey of damage in Kathmandu caused by the 2015 Gorkha Nepal earthquake. Soils Found. 55(5):1015–1029. doi:10.1016/j.sandf.2015.09.005.
- Pangali Sharma TP, Zhang J, Koju UA, Zhang S, Bai Y, Suwal MK. 2019. Review of flood disaster studies in Nepal: a remote sensing perspective. Int J Disaster Risk Reduc. 34:18–27. doi:10.1016/j.ijdrr.2018.11.022.
- Sun B,Yan P. 2015. Damage characteristics and seismic capacity of buildings during Nepal M s 8.1 earthquake. Earthq Eng Eng Vib. 14(3):571–578. doi:10.1007/s11803-015-0046-x.
- Sun C, Li J, Liu Y, Cao L, Zheng J, Yang Z, Ye J, Li Y. 2022. Ecological quality assessment and monitoring using a time-series remote sensing-based ecological index (ts-RSEI). GIScience Remote Sens. 59(1):1793–1816. doi:10.1080/15481603.2022.2138010.
- Waleed M, Sajjad M, Shazil MS, Tariq M, Alam MT. 2023. Machine learning-based spatial-temporal assessment and change transition analysis of wetlands: an application of Google Earth Engine in Sylhet, Bangladesh (1985–2022). Ecol Informatics. 75:102075. doi:10.1016/j.ecoinf.2023.102075.
- Wang Z, Dong C, Dai L, Wang R, Liang Q, He L, Wei D. 2023. Spatiotemporal evolution and attribution analysis of grassland NPP in the Yellow River source region, China. Ecol Informatics. 76:102135. doi:10.1016/j.ecoinf.2023.102135.
- Wang J, Li X, Christakos G, Liao Y, Zhang T, Gu X, Zheng X. 2010. Geographical detectors‐based health risk assessment and its application in the neural tube defects study of the Heshun Region, China. Int J Geogr Informat Sci. 24(1):107–127. doi:10.1080/13658810802443457.
- Wang JF, Xu CD. 2017. Geodetector: principle and prospective. Acta Geogr Sin. 72(1):116–134.
- Wang Y, Zhang Z, Chen X. 2021. Quantifying influences of natural and anthropogenic factors on vegetation changes based on geodetector: a case study in the Poyang Lake Basin, China. Remote Sens. 13(24):5081. doi:10.3390/rs13245081.
- Wang XD, Zhong XH, Liu SZ, Liu JG, Wang ZY, Li MH. 2008. Regional assessment of environmental vulnerability in the Tibetan Plateau: development and application of a new method. J Arid Environ. 72(10):1929–1939. doi:10.1016/j.jaridenv.2008.06.005.
- Wu J, Li J, Peng J, Li W, Xu G, Dong C. 2015. Applying land use regression model to estimate spatial variation of PM 2.5 in Beijing, China. Environ Sci Pollut Res Int. 22(9):7045–7061. doi:10.1007/s11356-014-3893-5.
- Xiong Y, Xu W, Lu N, Huang S, Wu C, Wang L, Dai F, Kou W. 2021. Assessment of spatial–temporal changes of ecological environment quality based on RSEI and GEE: a case study in Erhai Lake Basin, Yunnan province, China. Ecol Indicators. 125:107518. doi:10.1016/j.ecolind.2021.107518.
- Xu HQ. 2013. A remote sensing urban ecological index and its application. Acta Ecol Sin. 33(24):7853–7862.
- Xu H, Deng W. 2022. Rationality analysis of MRSEI and its difference with RSEI. Remote Sens Technol Appl. 37:1–7.
- Xu H, Wang Y, Guan H, Shi T, Hu X. 2019. Detecting ecological changes with a remote sensing based ecological index (RSEI) produced time series and change vector analysis. Remote Sens. 11(20):2345. doi:10.3390/rs11202345.
- Yan Y, Zhuang Q, Zan C, Ren J, Yang L, Wen Y, Zeng S, Zhang Q, Kong L. 2021. Using the Google Earth Engine to rapidly monitor impacts of geohazards on ecological quality in highly susceptible areas. Ecol Indicators. 132:108258. doi:10.1016/j.ecolind.2021.108258.
- Yang Y, Li H. 2023. Spatiotemporal dynamic decoupling states of eco-environmental quality and land-use carbon emissions: a case study of Qingdao City, China. Ecol Informatics. 75:101992. doi:10.1016/j.ecoinf.2023.101992.
- Yang X, Meng F, Fu P, Wang Y, Liu Y. 2022. Time-frequency optimization of RSEI: a case study of Yangtze River Basin. Ecol Indicators. 141:109080. doi:10.1016/j.ecolind.2022.109080.
- Yang X, Meng F, Fu P, Zhang J, Liu Y. 2022. Instability of remote sensing ecological index and its optimisation for time frequency and scale. Ecol Informatics. 72:101870. doi:10.1016/j.ecoinf.2022.101870.
- Yang X, Meng F, Fu P, Zhang Y, Liu Y. 2021. Spatiotemporal change and driving factors of the eco-environment quality in the Yangtze River Basin from 2001 to 2019. Ecol Indicators. 131:108214. doi:10.1016/j.ecolind.2021.108214.
- Yang W, Qi W. 2017. Spatial-temporal dynamic monitoring of vegetation recovery after the Wenchuan earthquake. IEEE J Sel Top Appl Earth Observations Remote Sens. 10(3):868–876. doi:10.1109/JSTARS.2016.2616511.
- Yang S, Su H. 2023. Evaluation of urban ecological environment quality based on Google Earth Engine: A Case study in Xi’an, China. Pol J Environ Stud. 32(1):927–942. doi:10.15244/pjoes/152448.
- Yang Z, Tian J, Su W, Wu J, Liu J, Liu W, Guo R. 2022. Analysis of ecological environmental quality change in the Yellow River Basin using the remote-sensing-based ecological index. Sustainability. 14(17):10726. doi:10.3390/su141710726.
- Yuan B, Fu L, Zou Y, Zhang S, Chen X, Li F, Deng Z, Xie Y. 2021. Spatiotemporal change detection of ecological quality and the associated affecting factors in Dongting Lake Basin, based on RSEI. J Cleaner Prod. 302:126995. doi:10.1016/j.jclepro.2021.126995.
- Zhang Y, Jiang X, Lei Y, Gao S. 2022. The contributions of natural and anthropogenic factors to NDVI variations on the Loess Plateau in China during 2000–2020. Ecol Indicators. 143:109342. doi:10.1016/j.ecolind.2022.109342.
- Zhang Y, She J, Long X, Zhang M. 2022. Spatio-temporal evolution and driving factors of eco-environmental quality based on RSEI in Chang-Zhu-Tan metropolitan circle, central China. Ecol Indicators. 144:109436. doi:10.1016/j.ecolind.2022.109436.
- Zhang C-y, Zhao L, Zhang H, Chen M-n, Fang R-y, Yao Y, Zhang Q-p, Wang Q. 2022. Spatial-temporal characteristics of carbon emissions from land use change in Yellow River Delta region, China. Ecol Indicators. 136:108623. doi:10.1016/j.ecolind.2022.108623.
- Zhao Q, Yu L, Li X, Peng D, Zhang Y, Gong P. 2021. Progress and trends in the application of Google Earth and Google Earth Engine. Remote Sens. 13(18):3778. doi:10.3390/rs13183778.
- Zhu L, Meng J, Zhu L. 2020. Applying Geodetector to disentangle the contributions of natural and anthropogenic factors to NDVI variations in the middle reaches of the Heihe River Basin. Ecol Indicators. 117:106545. doi:10.1016/j.ecolind.2020.106545.