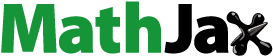
Abstract
Landslides are a widespread occurrence in various regions across the globe, and Ethiopia serves as a typical example. The objective of this research was to develop and evaluate a landslide susceptibility map (LSM) using two different approaches: the frequency ratio (FR) and analytical hierarchy process (AHP). The suitability and effectiveness of both approaches were assessed for the specific study area. In the initial phase, a total of 175 past landslides, covering an area of 13.09 km2, were identified and mapped for further analysis. Eight causative factors, including elevation, slope steepness, slope aspect, lithology, distance from fault and stream, precipitation, and land use/land cover, were selected and mapped accordingly. Subsequently, a landslide susceptibility index (LSI) was calculated based on the relative influence of these factors on the recorded past landslides. The resulting LSMs were then categorized into five zones representing varying degrees of susceptibility: very low, low, moderate, high, and very high. For the LSM derived from the FR approach, the very high and high susceptibility zones accounted for 16.65 km2 (7.58%) and 79.96 km2 (36.4%) of the total area, respectively. Conversely, for the LSM derived from the AHP approach, these values were 21.9 km2 (9.99%) and 68.9 km2 (31.37%). Generally, both the FR and AHP approaches yielded comparable results. The LSMs were validated using the area under the curve (AUC) method. The FR model exhibited higher accuracy and prediction rates, with values of 0.807 and 80.7%, respectively, compared to the AHP model with values of 0.756 and 75.6%, respectively. These generated LSMs offer valuable insights for identifying and addressing the requirements of landslide hazard mitigation and adaptation.
1. Introduction
Landslides are destructive geological hazards that primarily occur in hilly and mountainous regions around the world. These events involve the rapid downward movement of earth materials triggered by external factors (such as rainfall and earthquakes) (Soeters and Van Westen Citation1996; Hutchinson 2001) and gravity (Malamud et al. Citation2004). The occurrence of landslides is influenced by factors, such as slope-forming materials, topography, groundwater, and land use/land cover. In Ethiopia, certain areas like Dessie town, Adishuhu, and Tarmaber have experienced repeated slope instability and significant damage to human lives and infrastructure.
For example, between 1993 and 1998, landslides and associated problems in Ethiopia caused the loss of around 300 lives, damaged over 100 km of roads, destroyed more than 200 houses, and devastated over 500 hectares of fertile land (Ayalew Citation1999). In the Tarmaber area of the central Ethiopian highlands, a landslide event led to the displacement of more than 3000 people, the demolition of 1250 houses, the destruction of four churches, four mills, and a school, and the devastation of over 1500 hectares of farmland (Woldearegay Citation2013).
The limited studies and records available in Ethiopia indicate that landslides have had severe impacts on the lives and properties of communities. The Adwa Volcanic Mountain Plugs (AVMP) and its surroundings are also susceptible to landslides and related phenomena, leading to potential damage to infrastructure, such as houses, farmland, forests, and roads, although these impacts have not been well-documented.
To prevent or minimize the losses caused by landslides, landslide susceptibility prediction and zoning methods are crucial (Tang et al. Citation2023). Updated landslide susceptibility maps can provide valuable information for decision-making, land use planning, and implementing development activities. In this context, a landslide susceptibility zonation map (LSM) was created for the AVMP and its surrounding areas using the Frequency Ratio (FR) and Analytical Hierarchy Process (AHP) methods. This study aimed to compare and explore the potential applications of these two models specific to the Volcanic Mountain Plugs and their implications for land use planning. The FR and AHP methods were supplemented with extensive fieldwork, including geological surveys and the collection of data on past landslide events.
The detection and mapping of landslide-susceptible areas are crucial inputs for policymakers and strategy makers involved in planning and implementing various developmental activities in these areas. By utilizing such information, strategies can be formulated to minimize landslide-related damages and losses.
2. Study area
2.1. Location
The study area is defined by the geographic coordinates of 488,000–505,000 mE longitude and 1,566,000 to 1,579,000 mN latitude, within UTM Zone 37. It spans ∼219.66 square kilometers (). The elevation in the area varies from 2000 to 2850 meters above sea level (m.a.s.l.), and it exhibits a dendritic drainage pattern. The average annual precipitation in the area is ∼792.72 mm, as reported by ENMSA (Citation2002).
Figure 1. Study area location. (a) A section of the African continent highlighting the eastern part (Ethiopia), (b) map of Ethiopia indicating the regional states, such as Tigray, and (c) study area and its surroundings depicted on a map (with digital elevation model as the background). Source for digital elevation model: www.earthexplorer.usgs.gov.

2.2. Geological setting
The study area is characterized by different rock units , each with its own unique features (such as degree of weathering, strength, composition, etc.). These are major factors influencing the potential failure. Each lithologic unit is described in light of the aforementioned premises.
2.2.1. Phyllite
The Phyllite rock unit is mainly found in the northeast, northwest, and south strips of the study area. It is fine-grained and exhibits well-developed foliation features. These rocks have a variegated color, ranging from light grey to dark grey and green. They are highly weathered, soft, and show different types of fine lineation in low strain zones. Quartz veins are also commonly found along shear zones and other discontinuities within these rock units.
2.2.2. Meta-agglomerate
The Meta-agglomerate rock unit extends towards the northwest and south of the study area. It is characterized by angular volcanic clasts of varying sizes, ranging from blocks to ash size fragments. The predominant color of this rock unit is greenish, but violet/purple pigmented varieties are also common. The pigmentation is a result of the migration of fluid related to shear deformation. It is moderately weathered in most places.
2.2.3. Glacial tillites
The study area also contains glacial tillites, which are generally composed of a grey to black sand-silt-clay matrix. These tillites are interbedded with thin layers of laterite sandstone. They are mainly found overlaying the phyllite and meta-agglomerate units and are in turn overlaid by lateritic sandstone. On road cuts, a thickness ranging from 1.5 to 2 m of glacial tillites can be observed. It is inherently soft to weak in strength, highly weathered, erodible, and in many places without vegetation (bare land). Past landslides are frequently observed in this unit.
2.2.4. Laterized basement and granitic intrusive rocks
Laterization affects the exposed basement and granitic intrusive rocks in the study area. These rocks, which are found on the gentle slopes and rolling landforms, exhibit a reddish-brown color due to a high content of iron oxides. The laterized rock unit covers a small area with a thickness of ∼13–16 m. It is mainly exposed on hill sides due to erosion and mass wasting. Within the lateritic coverage area, heavy iron-rich pebbles and cobbles are distributed. This rock unit is highly compacted; less weathered, and exhibits some irregular fractures.
2.2.5. Aphanitic basalt
The Aphanitic basalt is a black rock that can also appear brown due to weathering. It is a highly fractured and weathered mafic rock with a fine-grained (aphanitic) texture. Irregular fractures and columnar joints are present in this rock unit, which contribute to weathering and potential landslides.
2.2.6. Trachytic rock unit
The Trachytic rock unit is characterized by closely spaced sub-vertical and columnar joints. It is fine-grained and light-colored. These rocks are typically massive and hard, and they outcrop in the elevated peaks of up to 2400 m above mean sea level. Mostly it is hard or strong, fresh to slightly weathered, and resistant to erosion processes ().
3. Methods
3.1. Data source and preparation
3.1.1. Landslide inventory mapping
To ensure a comprehensive understanding of the study area, the collection of past landslide inventory data was deemed essential, based on the assumption that the past serves as a valuable indicator for future events. The data collection process involved a combination of methods. Firstly, field observations conducted on-site involved the use of handheld GARMIN GPS devices to directly record landslide occurrences. Additionally, valuable information was obtained through personal interviews with local residents. However, it is important to note that certain landslides were concealed or located in remote and inaccessible areas, such as gorges, steep cliffs, and densely vegetated regions. To identify these concealed landslides, Google Earth Image (GEI) from Google Earth 2018 and aerial photograph interpretations at a scale of 50,000 were utilized. The collected field data and remote sensing data were compiled, organized, and digitized. To maximize clarity for digitizing past landslides, the study area was buffered using Google Earth Engine, employing the highest possible resolution within which blurred images could be enhanced. The digitized files were then exported in KML file format from Google Earth and subsequently imported and converted into shapefiles using ArcGIS 10.4.1 software. illustrates the common landslide types in the area.
3.1.2. Selection of causal factors for landslide susceptibility mapping
The process of selecting causal factors for landslide susceptibility mapping involved a combination of collected landslide characteristics in the area and a review of relevant literature on similar settings. After careful analysis, eight influential factors were identified: elevation, slope steepness, slope aspect, lithology, distance to faults, precipitation, distance to streams, and land use/cover. The first three factors (elevation, slope steepness, and slope aspect) were extracted from a digital elevation model (DEM) with a resolution of 45 × 45 m using ArcGIS 10.4.1. Expert opinions were utilized to reclassify these factors into categorical classes.
3.1.2.1. Slope steepness
The slope steepness was transformed into a thematic map layer and categorized into six classes based on expert opinion and the topography of the area. The classes were defined as 0–6, 6.1–15, 15.1–20, 20.1–30, 30.1–55, and >55° ().
3.1.2.2. Slope aspect
The slope aspect map was created using a DEM obtained from www.earthexplorer.usgs.gov. It was classified into nine classes based on all possible geographical directions, following the methodology described by Hong et al. (2016a). These classes include north, northeast, east, southeast, south, southwest, west, and northwest (as shown in ).
3.1.2.3. Elevation
The elevation map underwent a reclassification process using the expert opinion method, informed by literature reviews conducted by Hong et al. (Citation2016a, Citation2016b) and Pradhan and Kim (Citation2016). It was divided into six classes with intervals of 200 m, ranging from 1700 to >2700 m (as depicted in ).
3.1.2.4. Distance to streams
The drainage map of the area was categorized into eight classes with a 100 m interval, ranging from 0 to 100 to >700 m ().
3.1.2.5. Distance to faults
The distance to fault map was reclassified into nine classes using the equal interval method, ranging from 0 to 200 to >1600 m ().
3.1.2.6. Geological map
The geological map of the study area was created using interpretations from the 2018 Google Earth Image (GEI), field traverses, and the 1:50,000 scale geological map of the Adwa-Adigrat area by Berhane et al. (Citation2020). Distance to faults and streams maps were prepared based on field observations and the geological map (Anis et al. Citation2019; Berhane and Tadesse Citation2021; Tesfa and Woldearegay Citation2021).
3.1.2.7. Land use/land cover
The land use/land cover map was created using the 2018 GEI data, supplemented by field surveys.
3.1.2.8. Precipitation
Rainfall data covering a 15-year period (1992–2017) were gathered from four meteorological stations located nearby. The stations and their corresponding coordinates are as follows: (1) Adwa: 524,730 mE, 1,577,023 mN; (2) Rama: 476,364 mE, 1,592,083 mN; (3) Yeha: 516,756 mE, 1,551,735 mN; and (4) Fresmay: 497,961 mE, 1,571,783 mN (UTM, Zone 37 N).
To analyze the data, the mean annual precipitation was calculated for each of the stations. To create a comprehensive precipitation map, interpolation techniques were applied.
The resulting map was then divided into six distinct classes using the equal interval method. These classes were defined based on precipitation levels, ranging from <700 to >1100 mm. The categorization of the map can be observed in .
3.1.3. Exclusion of roads from LSM analysis
During the field assessment, the impact of roads on landslide initiation was thoroughly examined. It was observed that the road routes were primarily situated on flat land at the base of the majority of mountains. After careful consideration, it was determined that the influence of the roads on landslide occurrence was negligible. As a result, the roads were excluded from the LSM analysis.
3.2. Landslide susceptibility mapping
3.2.1. FR method
The FR value for each class of the causative factors was calculated using EquationEquation (1)(1)
(1) (Lee and Pradhan Citation2006). The area was calculated using EquationEquation (2)
(2)
(2) (Berhane et al. Citation2020).
(1)
(1)
(2)
(2)
Once all FR values were calculated, they were assigned to the respective causative factor classes in a GIS environment. The landslide susceptibility index (LSI) raster was obtained by summing the FR values of the eight causative factors using EquationEquation (3)(3)
(3) (Lee and Talib Citation2005).
(3)
(3)
The LSI map generated was then classified into five zones (very low, low, moderate, high, and very high) based on the landslide inventory, review of literature, and expert opinion. The areal coverage of each zone was calculated in the ArcGIS 10.4 environment using the field calculator command. The results were compared between the landslide inventory and causative factor classes with the generated LSM.
3.2.2. AHP method
Problems related to decision-making require the consideration of all potential factors. Properly assigning priority to these potential influencing factors can lead to better decisions (Devara et al. Citation2021). The Analytic Hierarchy Process (AHP) is a multi-criteria decision-making approach that allows users to establish a scale of preferences from a set of alternatives (Yalcin et al. Citation2011). The AHP has been widely applied in suitability analysis and landslide susceptibility mapping, as seen in studies by Ayalew et al. (Citation2005) and Pourghasemi et al. (Citation2018).
For the AHP analysis in this study, the eight causative factors selected for the FR method were used. These factors, along with their respective classes, were arranged in a hierarchical order to evaluate landslides using the AHP. To determine the relative importance of each criterion (factor), a pairwise comparison matrix (PCM) was created. The elements in the PCM were compared pairwise to establish their relative importance, based on the 9-point scale introduced by Saaty (Citation1980, Citation1994), which includes values like 1/9, 1/8, 1/7, 1/6, 1/5, 1/4, 1/3, 1/2, 1, 2, 3, 4, 5, 6, 7, 8, and 9.
To compute the Eigen vector of a reciprocal matrix, the following steps were performed:
The values in each column of the reciprocal matrix were summed.
Each element in the matrix was divided by its column total, resulting in the normalized PCM, where the sum of each column is 1.
The average of the elements in each row of the normalized matrix was computed by dividing the sum of normalized scores for each row by the number of criteria.
The consistency ratio (CR) was calculated using the following steps:
The Eigen vector or relative weights and the largest Eigen value (λmax) were calculated for each matrix of order ‘n’. The principal λmax is obtained from the summation of products between each element of the Eigen vector and the sum of columns of the reciprocal matrix.
The consistency index (CI) for each matrix of the causative n factors was computed using EquationEquation (4)
(4)
(4) proposed by Saaty (Citation1977).
(4)
(4)
Calculation of Consistency Ratio (CR)
To determine the consistency of the pairwise comparisons in the AHP analysis, the consistency ratio (CR) was calculated using EquationEquation (5)(5)
(5) as proposed by Saaty (Citation1977):
(5)
(5)
Here, RI represents the Random Consistency Index, which is obtained through numerous simulation runs and varies based on the order of the matrix.
After obtaining the weighted values as mentioned above, the values of the respective classes of the causative factors were manually assigned to a new column in each attribute table of the causative factors within a Geographic Information System (GIS) environment. This manual assignment was done to facilitate the aggregation process.
Similar to the FR method, a weighted sum of all the causative factors was performed to generate the final Landslide Susceptibility Index (LSI) map. This map provides an aggregate representation of the combined influence of all the causative factors on landslide susceptibility.
Subsequently, the LSI map was divided into five landslide susceptibility zones based on the respective area coverage. This zoning process allows for the identification of areas with varying degrees of landslide susceptibility, providing valuable information for land management and decision-making.
3.2.2.1. Limitations of AHP method
It is important to note that the AHP method has certain limitations. One limitation is its inability to account for the uncertainty resulting from changes in the rankings of causative factors. The ranking of causative factors in the AHP method is subjective, and this subjectivity can influence the final LSM generated through the AHP analysis.
3.3. Validation of LSM using area under curve (AUC) method
To assess the reliability of the derived LSM, the Area Under Curve (AUC) method, specified in EquationEquation (6)(6)
(6) by Bouckaert (Citation2006), was employed.
(6)
(6)
In this method, a success curve is created to determine the percentage of known landslides (inventory) that correspond to each defined level of the Landslide Susceptibility Index (LSI) value. The ideal success scenario is represented by the highest AUC value of 1 (or 100%).
To generate the success rate curve, the LSI values of all pixels are sorted in descending order, from highest to lowest. These ordered pixels are then divided into 100 classes with 1% intervals. The accumulated percentage of landslides for each class is identified and plotted. The x-axis of the plot represents the LSI ranks (0–100), with higher ranks indicating lower LSI values, starting from the higher portion (VHS) to the lower portion (VLS) of LSI values.
The AUC value is calculated based on the cumulative landslide occurrence (CLO) and LSI of each zone. The AUC represents the total area under the success rate curve and can be used to qualitatively assess the success rate of the susceptibility map. A larger area under the curve indicates a higher success rate.
To facilitate quantitative comparisons, the AUC values are rescaled to have a total area of 1. This rescaling ensures that the AUC represents a perfect success rate.
4. Results
4.1. Landslide inventory
The past inventory data of landslides serves as a crucial foundation for conducting landslide susceptibility mapping. In this study, a total of 175 landslides were identified and mapped, covering a combined area of 13.09 km2. These landslides were classified into four distinct categories based on their failure mechanisms: topples (9), complex or compound mass movements (11), debris flows (61), and rock falls (94).
In a separate investigation conducted by Nyssen et al. (Citation2003) in the Atbara-Tekeze headwaters, they identified 34 landslides and grouped into different types of landslides. These types included 11 landslide depletion areas, 10 debris flows, one slump, three debris and rock slides, three landslide belts, and six rock falls.
Analyzing the total 175 landslides, it was found that debris flows accounted for 6.53 km2 (49.92%), rock falls covered 4.89 km2 (37.38%), topple mode of failure occupied 0.12 km2 (0.95%), and complex or compound types encompassed 1.54 km2 (11.76%) of the total area affected by landslides.
4.2. Relationships between landslide causative factors and past landslide inventories
To generate a reliable LSM, it is crucial to comprehend the factors that contribute to landslides at a specific site. In this particular study area, eight causative factors were identified based on their impact on landslide initiation and their correlation with landslide inventories. These factors were determined through a comprehensive review of relevant literature, field verification, and an assessment of the area’s geo-environmental conditions. The primary causative factors considered in this study are slope steepness, slope aspect, elevation, distance to stream, distance to fault, lithology, precipitation, and land use/cover (as depicted in and ).
4.2.1. Slope steepness
The largest proportion of the area (28.5%) falls within the 6.1–15° slope class, while the smallest proportion (3.4%) falls within the 30.1–55° slope class. A higher percentage of landslides (22.86% or 2.99 km2) were observed in the 30.1–55° slope class, whereas the smallest landslides (6.8% or 0.98 km2) were found in the 0–6°slope class. The increased density of landslides in the 30.1–55° slope class can be attributed to agricultural practices conducted on such steep slopes. On the other hand, in areas with slopes >55°, agricultural activities are limited and are predominantly characterized by massive trachyte rock outcrops.
4.2.2. Slope aspect
The majority of the study area is characterized by slopes facing west (13.4% or 29.4 km2) and northwest (14% or 30.9 km2). The smallest area (0.64%) is associated with slopes facing north.
Analyzing the distribution of past landslides in relation to slope aspect, it can be observed that the highest number of landslides occurred on slopes inclined towards the west (15.51%), south (15.30%), southwest (14.83%), northwest (14.31%), and northeast (13.85%) directions. The remaining landslides occurred on slopes facing east (11.35%), north (7.61%), and northeast (7.21%). There were very few or no reported landslides on flat slope aspects. The variations in landslide distribution across slope aspect classes can be attributed to the presence or absence of moisture, discontinuities, and the direction of runoff (erosion), which are influenced by the direction of sunlight.
4.2.3. Elevation
When analyzing the distribution of landslides, it was observed that a higher proportion of landslides (29.61%) were concentrated in the elevation class >2700 m. This was followed by the elevation classes of 2500–2700 m (23.1%), 2300–2500 m (22.21%), and 2100–2300 m (14.59%). The elevation classes of 1700–1900 and 1900–2100 m exhibited a lower distribution of landslides, with respective area coverages of 3.28 and 7.15%.
4.2.4. Distance to stream
Upon analyzing the distribution of landslides, it was observed that the highest concentration of landslides (26.26%) occurred within a distance of <100 m from streams. This could be attributed to factors, such as increased pore water pressure near streams, the presence of moisture, and erosion, which collectively result in reduced shear strength of slope materials. In contrast, areas located at a greater distance from streams (e.g. >700 m) experienced a lower incidence of landslides, with only 5.96% of the affected area being affected by minor landslides.
4.2.5. Distance to fault
Among classes, the areas within the 0–200, 200–400, 400–600, and 600–800 m distance ranges exhibited higher proportions of landslide occurrences, with respective area coverages of 15.53, 15.5, 13.61, and 12.95%. This can be attributed to the presence of greater heave and closely spaced fractures near faults, which facilitate weathering and weaken the strength of slope materials.
In contrast, out of the inventoried landslides, 11.22, 10.77, 8.82, 6.59, and 5.05% of the affected area fell within the distance classes of 800–1000, 1000–1200, 1200–1400, 1400–1600, and >1600 m from the faults, respectively. Lee and Sambath (Citation2006) reported the presence of closely spaced joints and a higher degree of weathering in close proximity to faults, which increases the likelihood of landslides occurring in these areas.
4.2.6. Lithology
Lithology is a crucial factor in landslide studies as it is closely linked to the geo-mechanical properties that influence weathering (Yalcin et al. Citation2011; Hong et al. Citation2016a). In the study area covering a total area of 219.66 km2, the distribution of different lithologies is as follows: trachyte covers an area of 88.97 km2, phyllite covers 78.27 km2, aphanitic basalt covers 28.29 km2, lateritic sandstone covers 14.24 km2, meta-agglomerate covers 5.61 km2, and glacial deposit/tillite covers 4.28 km2 ().
Among these lithologies, 53.81% of the landslides occurred in the trachyte units. This can be attributed to the presence of joints, particularly columnar joints, which allow water to percolate through and weaken the strength of the material. In the case of the other lithologies, 36.33, 4.63, 3.98, 1.04, and 0.22% of the landslides occurred in the phyllite, aphanitic basalt, lateritic sandstone, meta-agglomerate, and tillite units, respectively.
4.2.7. Precipitation
The spatial distribution of rainfall has a significant impact on soil saturation (Hong et al. Citation2016b), pore water pressure, and erosion. Previous researchers, such as Youssef et al. (Citation2015), have reported a strong correlation between mean annual precipitation and landslide occurrences. It is observed that as the precipitation increases, so does the area affected by landslides. This can be attributed to the reduction in shear strength caused by saturation in the upper part of the soil profile and rock units, as well as the build-up of pore water pressures in the lower parts of the slopes.
4.2.8. Land use/cover
The land use/cover classes of the area were categorized into cultivation, shrub/bush, bare land, grassland, water body, and settlement (). In terms of area coverage, cultivated land accounted for 69.21% (152.02 km2), shrub/bush covered 26.06% (57.25 km2), and settlement comprised 1.86% (4.09 km2) of the total area. Grassland, bare land, and water body accounted for 1.25% (2.74 km2), 1.07% (2.34 km2), and 0.56% (1.22 km2), respectively.
It is noteworthy that a higher proportion of landslide areas (47.45%) are found in the shrub/bush land, which can be attributed to the steep slope morphology and the shallow root systems of shrubs/bushes. Following the shrub/bush land, 42.07% (1 km2) of past landslides occurred in agricultural land. The bare land and grassland accounted for 7.73% (1.01 km2) and 1.76% (0.23 km2) of landslide areas, respectively. The bare land area is primarily characterized by unconsolidated soil, which has a high susceptibility to erosion and softening when saturated.
5. Discussion
In the study area, the landslide inventories were classified into four types based on the classifications by Varnes (Citation1978) and Hungr et al. (Citation2014). These types include debris flow (49.92%), rockfall (37.38%), topple (0.95%), and complex (11.76%). The causal factors affecting landslide occurrences were analyzed and their significance is presented in .
Table 1. Summary of calculated FR values for each landslide causative factors.
Table 2. Area occupied by each landslide susceptibility classes.
Table 3. Pairwise comparison matrix (PCM), factor weights and CR of the eight causative factors.
Table 4. Pairwise comparison matrix (PCM), factor weights and CR of the data layers or causative factors.
For instance, according to the FR method, the dominant factor influencing landslides was the distance to the stream, followed by elevation, slope steepness, and land use/cover. Other factors had a limited influence on triggering landslide events. These findings align closely with a similar study conducted by Hong et al. (Citation2016a, Citation2016b) in the Chongren area of China.
In contrast, based on the weight values obtained from the AHP ( and ), the causative factors of slope, distance to fault, lithology, precipitation, and elevation had the highest weight values. On the other hand, factors, such as distance to the stream, land use/cover, and aspect were found to have less importance in inducing landslides. The significance of each sub-class within the factors was discovered to be intricate and not easily discernible. For example, the study revealed that the distance to streams within a range of 300 m was significantly associated with landslide occurrences. Similar observations were made for other factors, such as land use/land cover and slope aspect.
A detailed discussion of the models used and the specific subclasses of causative factors follow below.
5.1. LSM using FR method
The FR values of the causative factors were calculated using EquationEquation (1)(1)
(1) () following the methodology proposed by Akgun et al. (Citation2008). As per previous studies by researchers, such as Nourani et al. (Citation2014) and Yalcin et al. (Citation2011), an FR value >1 indicates a strong relationship or a greater contribution to landslide occurrence, while an FR value <1 suggests the opposite.
Based on the calculated FR values, it was observed that slope classes ranging from >55, 30.1–55, and 20.1–30° had higher FR values of 2.8, 5.43, and 1.56, respectively. These classes exhibited a higher proportion of past landslides, which could be attributed to lower shear strength or higher stress associated with low gradients (Ahmed Citation2015). Similarly, slopes facing southwest, south, west, southeast, and northwest had higher FR values () of 1.21, 1.2, 1.16, 1.13, and 1.02, respectively. These variations can be attributed to the presence of discontinuities and moisture in relation to specific slope face directions, a finding confirmed during field campaigns. Wieczorek and Guzzetti (Citation1999) reported that slope aspect plays a role in landslide occurrence by influencing the orientation and density of tectonic fractures.
The study area exhibited significant elevation contrast and relief. Elevation ranges of >2700, 2500–2700, and 2300–2500 m had higher FR values of 2.95, 5.95, and 1.03, respectively, indicating their greater contribution to landslide occurrences. This can be explained by the presence of more intense erosion and weathering in elevated areas. Similar results and observations have been reported by Wan et al. (Citation2012) in other regions.
The distance to the stream is a controlling factor for slope stability (Yalcin et al. Citation2011). The FR values decrease from a higher value of 7.4 for the closest distance range of 0–100 m to a value of 0.17 for distances >700 m. Therefore, closer proximity to streams corresponds to higher landslide occurrences. This can be explained by the fact that streams are associated with erosion (toe erosion, bank erosion, etc.), and the proximity of slopes to drainage or streams affects the degree of saturation, which directly contributes to slope instability. During field observations, many deep gullies, toe erosion, and bank erosion were observed in areas dominated by soil and highly weathered rocks.
Areas within a distance of <1000 m from fault structures were found to be more susceptible to landslides compared to distant areas (). Faults are tectonic surface features that typically have highly fractured rock masses, which are conducive to landslide occurrences.
The trachyte rock unit showed a strong correlation with landslide occurrence, with an FR value of 1.33 (). This is due to weaknesses developed as a result of vertical or sub-vertical columnar joints in this unit. Phyllite, with an FR value of 1.02, also exhibited a significant relationship with landslide occurrences, attributed to weaknesses caused by weathering and foliations. However, the tillite, aphanitic basalt, meta-agglomerate, and laterite sandstone lithologic units had very little contribution to landslide occurrences, with FR values of 0.11, 0.36, 0.41, and 0.61, respectively. This can be attributed to their small area coverage relative to other classes (1.95, 12.88, 2.56, and 6.48% of the total area).
Bare land had a strong contribution to landslide occurrences with an FR value of 7.25, which can be attributed to the impacts of deforestation and cultivation practices on hill slopes. Shrub/bush and grassland also exhibited a strong association with landslide occurrences, with FR values of 1.81 and 1.41, respectively. This can be explained by the weight of vegetation on sloping areas, which contributes to an increase in destabilizing stress (Gray and Leiser Citation1982). According to Farrokhnia et al. (Citation2011), shrubs can increase infiltration, leading to an increase in pore water pressure and the unit weight of sliding material. On the other hand, cultivated land, settlement areas, and water bodies (with FR values <1) did not significantly contribute to landslide occurrences in the area. These findings were consistent with field observations.
The rate of landslide occurrence was found to be strongly related to mean annual precipitation (Zhang et al. Citation2018). Precipitation ranges of 900–1000, 1000–1100, and >1100 mm exhibited a strong relationship with landslide occurrences, with FR values >1 (). Woldearegay (Citation2013) reported that rainfall is one of the main factors influencing slope stability in the Ethiopian highlands.
Finally, based on the landslide susceptibility index (LSI), which is the sum of the FR values of all factors, the landslide susceptibility map (LSM) was generated. The map was divided into five susceptibility classes: very high, high, moderate, low, and very low (). Similar approaches have been reported by other researchers, such as Avinash and Ashamanjari (Citation2010), Yalcin (Citation2007), and Yalcin et al. (Citation2011).
The very high and high susceptibility zones constituted 16.65 km2 (7.58%) and 79.96 km2 (36.4%) of the total area, respectively. The moderate, low, and very low susceptibility zones covered 92.103 km2 (41.93%), 25.766 km2 (11.73%), and 5.19 km2 (2.35%) of the total area, respectively ().
5.2. LSM using AHP method
All the causative factors and sub-classes have attained their own distinct weights with respect to their degree of influence on landslide occurrence. Slope steepness (with a weight value of 32%) has the highest influence among all factors (). This result is in line with field observations and landslide inventories.
Specifically, slope class categories 30.1–55, >55, and 20.1–30° play an important contribution to landslide occurrences in the area (). Maharjan (Citation2006) reported similar findings to the present results from a study conducted in Turkey.
Areas or slopes closer to faults play an important role in the occurrences of landslides with a weighted value of 32% ().
Cultivated land shares higher percentage for the occurrence of landslide with a weight value of 38% followed by shrub/bush lands (27%) (). Deforestation and improper farming techniques result in the high percentage of landslides.
LSM was generated using the eight different landslide causative factors in a GIS environment. The area was classified into five susceptibility zones (very low, low, moderate, high, and very high) (). The area and percentage distribution of the susceptibility zones in the study area were also determined.
Accordingly, 21.9 km2 (9.99%) and 68.9 km2 (31.37%) of the area are found to be of very high and high susceptibility zones. Moderate, low, and very low susceptible zones represent 90.08 km2 (41.01%), 32.64 km2 (14.86%), and 6.08 km2 (2.77%) of the total area, respectively ().
5.3. Comparison and validation of LSMs
For the LSM derived from AHP, the total area was divided into different susceptibility zones. These zones include very low (2.77% or 6.08 km2), low (14.86% or 32.64 km2), moderate (41.01% or 90.08 km2), high (31.3% or 68.91 km2), and very high (9.99% or 21.94 km2) susceptible zones. On the other hand, the LSM derived from FR resulted in the following distribution: very low (2.35% or 5.19 km2), low (11.73% or 25.76 km2), moderate (41.93% or 92.1 km2), high (36.4% or 79.96 km2), and very high (7.58% or 16.65 km2) susceptible zones. Overall, both methods yielded similar LSM results. However, the AHP-derived LSM had slightly higher proportions of very low, low, and very high susceptible zones, and slightly lower proportions of moderate and high susceptible zones compared to the FR-derived LSM.
The variation observed between the two methods can be attributed to their overall approach. The AHP method involves a certain level of subjectivity due to the incorporation of experts’ opinions. On the other hand, the FR method is more objective in nature. This fundamental difference in approach contributes to the variation in results obtained from the two methods.
To evaluate the performance of the LSMs, the area under the curve (AUC) was calculated based on the graph of cumulative landslide occurrence (%) and landslide susceptibility index (%). The success rate curves showed that the top 10% classes of the area, where the LSI had a higher rank, could explain ∼62 and 60% of all inventoried landslides for FR and AHP, respectively. Similarly, the curves indicated that the top 20% class of the areas, where the LSI had a high rank, explained about 78 and 74% of the failures for AHP and FR, respectively. The AUC values calculated for FR and AHP were 0.807 (80.7%) and 0.756 (75.6%), respectively (). This indicates that the FR method had a better success rate than AHP for this study.
Figure 8. A cumulative frequency diagram illustrating the rank of landslide susceptibility index (x-axis) against the cumulative percentage of landslide occurrence (y-axis).

Overall, the comparison and validation of the LSMs showed that both AHP and FR methods produced similar results, but with slight differences in the distribution of susceptibility zones. The AUC values indicated that the FR method had a higher success rate compared to AHP in predicting landslide susceptibility in the study area.
6. Conclusions and recommendations
The study findings indicate that both the FR and AHP methods are suitable for landslide susceptibility mapping in the area. However, the FR method yielded the best results for landslide susceptibility mapping. This superior performance of the FR method can be attributed to its reliance on objective factors, such as the quality of landslide inventories and the selection of appropriate causative factors for the specific study site, rather than subjective expert opinions as required by the AHP method.
For the FR method, the most influential factors in determining landslide susceptibility were identified as follows:
Distance to streams (<400 m)
Slope steepness (>20°)
Elevation (>2300 m above mean sea level)
Land use/land cover (including bare land, shrub-bush, and grassland)
Rainfall (>900 mm)
Conversely, factors, such as slope aspect, distance to faults, and lithology were found to have the lowest importance in causing landslide events.
On the other hand, for the AHP method, the main factors contributing to landslide occurrences were determined to be:
Slope steepness
Distance to fault
Lithology
Precipitation
Elevation
In terms of accuracy, the FR method achieved an accuracy rate of 80.7%, while the AHP method achieved a slightly lower accuracy rate of 75.6%. These results indicate that the FR method outperforms the AHP method in terms of the area under the curve (AUC). Overall, both methods demonstrated reasonable accuracy and were consistent with existing landslides and field observations.
The paper’s target audience includes land use planners, practicing geologists and engineers, geo-hazard experts, and decision-makers for their routine as well as medium and long-term activities and strategies. The findings of this study suggest prioritizing the use of the FR method for landslide susceptibility mapping due to its better performance and objectivity. Future research could focus on refining the importance of identified factors and exploring additional causative factors to enhance the understanding of landslide occurrences in the area.
Acknowledgements
We would like to express our gratitude to Mekelle University (MU), Ethiopia, for providing the financial support for this study. We would also like to extend our appreciation to the anonymous reviewers and the Editor-in-Chief, Prof. Ramesh Singh, for their valuable suggestions and comments, which greatly contributed to the improvement of the manuscript.
Data availability statement
The data that support the findings of this study are available upon request.
Disclosure statement
No potential conflict of interest was reported by the author(s).
Additional information
Funding
References
- Ahmed B. 2015. Landslide susceptibility mapping using multi-criteria evaluation techniques in Chittagong Metropolitan Area, Bangladesh. Landslides. 12(6):1077–1095. doi: 10.1007/s10346-014-0521-x.
- Akgun A, Dag S, Bulut F. 2008. Landslide susceptibility mapping for a landslide-prone area (Findikli, NE of Turkey) by likelihood-frequency ratio and weighted linear combination models. Environ Geol. 54(6):1127–1143. doi: 10.1007/s00254-007-0882-8.
- Anis Z, Wissem G, Vali V, Smida H, Essghaier GM. 2019. GIS-based landslide susceptibility mapping using bivariate statistical methods in North-western Tunisia. Open Geosci. 11(1):708–726. doi: 10.1515/geo-2019-0056.
- Avinash K, Ashamanjari K. 2010. A GIS and frequency ratio based landslide susceptibility mapping: Aghnashini river catchment, Uttara Kannada, India. Int J Geomatics Geosci. 1:343.
- Ayalew L, Yamagishi H, Marui H, Kanno T. 2005. Landslides in Sado Island of Japan: part II. GIS-based susceptibility mapping with comparisons of results from two methods and verifications. Eng. Geol. 81(4):432–445. doi: 10.1016/j.enggeo.2005.08.004.
- Ayalew L. 1999. The effect of seasonal rainfall on landslides in the highlands of Ethiopia. Bull Eng Geol Env. 58(1):9–19. doi: 10.1007/s100640050065.
- Berhane G, Kebede M, Alfarah N, Hagos E, Grum B, Giday A, Abera T. 2020. Landslide susceptibility zonation mapping using GIS-based frequency ratio model with multi-class spatial data-sets in the Adwa-Adigrat mountain chains, northern Ethiopia. J Afr Earth Sci. 164:103795. doi: 10.1016/j.jafrearsci.2020.103795.
- Berhane G, Tadesse K. 2021. Landslide susceptibility zonation mapping using statistical index and landslide susceptibility analysis methods: a case study from Gindeberet District, Oromia Regional State, Central Ethiopia. J Afr Earth Sci. 180:104240. doi: 10.1016/j.jafrearsci.2021.104240.
- Bouckaert RR. 2006. Efficient AUC learning curve calculation. In: Sattar A, Kang B, editors. AI2006: advances in artificial intelligence, AI2006. Lecture notes in computer sciences. Vol. 4304. Berlin; Heidelberg: Springer. doi: 10.1007/11941439_22.
- Devara M, Tiwari A, Dwivedi R. 2021. Landslide susceptibility mapping using MT-InSAR and AHP enabled GIS-based multi-criteria decision analysis. Geomatics Nat Hazards Risk. 12(1):675–693. doi: 10.1080/19475705.2021.1887939.
- [ENMSA] Ethiopian National Meteorology Service Agency. 2002. Daily rainfall data of Adwa. Tigray: Yaha & Feresmay Weather Station.
- Farrokhnia A, Pirasteh S, Pradhan B, Pourkermani M, Arian M. 2011. A recent scenario of mass wasting and its impact on the transportation in Alborz Mountains, Iran using geo-information technology. Arab J Geosci. 4(7–8):1337–1349. doi: 10.1007/s12517-010-0238-7.
- Gray DH, Leiser AT. 1982. Biotechnical slope protection and erosion control. New York: Van Nostrand Reinhold Company Inc.
- Hong H, Chen W, Xu C, Youssef AM, Pradhan B, Bui DT. 2016a. Rainfall-induced landslide susceptibility assessment at the Chongren area (China) using frequency ratio, certainty factor, and index of entropy. Geocarto Int. 32:1–16. doi: 10.1080/10106049.2015.1130086.
- Hong H, Pourghasemi HR, Pourtaghi ZS. 2016b. Landslide susceptibility assessment in Lianhua County (China): a comparison between a random forest data mining technique and bivariate and multivariate statistical models. Geomorphology. 259:105–118. doi: 10.1016/j.geomorph.2016.02.012.
- Hungr O, Leroueil S, Picarelli L. 2014. The Varnes classification of landslide types, an update. Landslides. 11(2):167–194. doi: 10.1007/s10346-013-0436-y.
- Lee S, Pradhan B. 2006. Probabilistic landslide hazards and risk mapping on Penang Island, Malaysia. J Earth Syst Sci. 115(6):661–672. doi: 10.1007/s12040-006-0004-0.
- Lee S, Sambath T. 2006. Landslide susceptibility mapping in the Damrei Romel area, Cambodia using frequency ratio and logistic regression models. Environ Geol. 50(6):847–855. doi: 10.1007/s00254-006-0256-7.
- Lee S, Talib JA. 2005. Probabilistic landslide susceptibility and factor effect analysis. Environ Geol. 47:982–990. https://doi.org/10.1007/s00254-005-1228-z.
- Maharjan S. 2006. Investigation of triggering factors on landslide occurrence and landslide hazard zonation–a GIS based approach [B.Sc. thesis]. Pokhara: Institute of Forestry.
- Malamud BD, Turcotte DL, Guzzetti F, Reichenbach P. 2004. Landslide inventories and their statistical properties. Earth Surf Processes Landf. 29(6):687–711. doi: 10.1002/esp.1064.
- Nourani V, Pradhan B, Ghaffari H, Sharifi SS. 2014. Landslide susceptibility mapping at Zonouz Plain, Iran using genetic programming and comparison with frequency ratio, logistic regression, and artificial neural network models. Nat Hazards. 71(1):523–547. doi: 10.1007/s11069-013-0932-3.
- Nyssen J, Moeyersons J, Poesen J, Deckers J, Haile M. 2003. The environmental significance of the remobilisation of ancient mass movements in the Atbara–Tekeze headwaters, Northern Ethiopia. Geomorphology. 49(3–4):303–322. doi: 10.1016/S0169-555X(02)00192-7.
- Pourghasemi HR, Yansari ZT, Panagos P, Pradhan B. 2018. Analysis and evaluation of 622 landslide susceptibility: a review on articles published during 2005–2016 (periods of 2005–623 2012 and 2013–2016). Arab J Geosci. 11:193. doi: 10.1007/s12517-018-3531-5.
- Pradhan AMS, Kim YT. 2016. Evaluation of a combined spatial multi-criteria evaluation model and deterministic model for landslide susceptibility mapping. Catena. 140:125–139. doi: 10.1016/j.catena.2016.01.022.
- Saaty L. 1977. A scaling method for priorities in hierarchical structures. J Math Psychol. 15(3):234–281. doi: 10.1016/0022-2496(77)90033-5.
- Saaty TL. 1980. The analytic hierarchy process. New York (NY): McGraw Hill; p. 350.
- Saaty TL. 1994. How to make a decision: the analytic hierarchy process. Interfaces. 24(6):19–43. doi: 10.1287/inte.24.6.19.
- Soeters R, Van Westen C. 1996. Slope instability recognition, analysis and zonation: investigation and mitigation. Landslides. 247:129–177.
- Tadesse T. 1997. Geology of Axum area. Addis Ababa: Ethiopian Institute of Geological Surveys, Memoir No. 9.
- Tang G, Fang Z, Wang Y. 2023. Global landslide susceptibility prediction based on the automated machine learning (AutoML) framework. Geocarto Int. 38(1):2236576. doi: 10.1080/10106049.2023.2236576.
- Tesfa C, Woldearegay K. 2021. Characteristics and susceptibility zonation of landslides in Wabe-Shebelle Gorge, south eastern Ethiopia. J Afr Earth Sci. 182:104275. doi: 10.1016/j.jafrearsci.2021.104275.
- Varnes DJ. 1978. Slope movement types and processes. In: Schuster RL, Krizek RJ, editors. landslides, analysis and control, transportation research board, Special Report No. 176. National Academy of Sciences. P. 11–33.
- Wan S, Lei TC, Chou TY. 2012. A landslide expert system: image classification through integration of data mining approaches for multi-category analysis. Int J Geogr Inf Sci. 26(4):747–770. doi: 10.1080/13658816.2011.613397.
- Wieczorek GF, Guzzetti F. 1999. A review of rainfall thresholds for triggering landslides. Proceedings of the EGS Plinius Conference; Maratea, Italy; p. 407–414.
- Woldearegay K. 2013. Review of the occurrences and influencing factors of landslides in the highlands of Ethiopia: with implications for infrastructural development. Momona Ethiop J Sci. 5(1):3–31. doi: 10.4314/mejs.v5i1.85329.
- Yalcin A, Reis S, Aydinoglu A, Yomralioglu T. 2011. A GIS-based comparative study of frequency ratio, analytical hierarchy process, bivariate statistics and logistics regression methods for landslide susceptibility mapping in Trabzon, NE Turkey. Catena. 85(3):274–287. doi: 10.1016/j.catena.2011.01.014.
- Yalcin A. 2007. The effects of clay on landslides: a case study. Appl Clay Sci. 38(1–2):77–85. doi: 10.1016/j.clay.2007.01.007.
- Youssef AM, Al-Kathery M, Pradhan B. 2015. Landslide susceptibility mapping at Al-Hasher area, Jizan (Saudi Arabia) using GIS-based frequency ratio and index of entropy models. Geosci J. 19(1):113–134. doi: 10.1007/s12303-014-0032-8.
- Zhang S, Zhao L, Delgado-Tellez R, Bao H. 2018. A physics-based probabilistic forecasting model for rainfall-induced shallow landslides at regional scale. Nat Hazards Earth Syst Sci. 18(3):969–982. doi: 10.5194/nhess-18-969-2018.