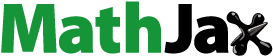
Abstract
The feature extraction of blasting and natural earthquake waves can effectively discriminate between the two, and is conducive to mastering the time–frequency distribution and failure mechanism. However, feature extraction and discrimination between the two are often subject to external interference. A complete ensemble empirical mode decomposition and multiscale permutation entropy and Hilbert–Huang transform method is proposed to extract features and discriminate for blasting and natural earthquake waves efficiently and accurately. Firstly, the simulation signals verify feasibility and applicability. Secondly, six scenarios for blasting and natural earthquakes are collected and compared. Thirdly, feature extraction and discrimination are performed. Results show that the proposed method can extract and discriminate features between the two effectively. Furthermore, the energy of the former is concentrated within 200 Hz, while the latter is within 3 Hz, and different scenarios or magnitudes have different characteristics. Moreover, the blasting earthquake has features such as short duration, peaks corresponding to the blasting initiation time and fast energy attenuation. The natural earthquake has features such as long duration, multiple peaks, and slow energy attenuation, resulting in more significant harm. This method can extract features and discriminate for blasting and natural earthquake waves from the perspective of time–frequency and energy effectively.
1. Introduction
Blasting earthquakes is the vibration of ground particles caused by the release of explosive energy, which breaks the media around the blast hole and transmits part of the energy to the ground in the form of waves. Natural earthquakes are relatively large areas of underground or surface structure vibration caused by large-scale collapse or fracture of the earth’s faults through the release of energy from the earth’s crust. Blasting and natural earthquake waves propagate in the medium in the form of elastic waves, causing vibrations on the surface during energy release, thereby posing a threat to the safety of infrastructure and people. The two earthquake waves have similarities and differences. Blasting and natural earthquake waves have certain similarities in waveform characteristics, and they also have significant differences in frequency, duration, energy distribution, influence coverage, carried energy and vibration intensity. Accurately distinguish between blasting and natural earthquakes are done by various researchers worldwide (Xia et al. Citation2021; Weng et al. Citation2022; Huang et al. Citation2023; Kan et al. Citation2023).
Feature extraction and discrimination of blasting and natural earthquake waves are significant. The propagation, waveform characteristics, energy characteristics, and attenuation laws of blasting earthquake waves in the medium can be identified by monitoring, extracting and analysing the information in blasting vibration signals and studying the spectrum and energy distribution characteristics. The quality and effect of blasting construction and the safety and stability of buildings under blasting vibration must also be evaluated. Similarly, the study of natural earthquake waves can invert the structure of the earth’s media and seismic source parameters, and it is of great significance for seismic exploration, earthquake mitigation, and structural seismic (Zhou et al. Citation2020; Man et al. Citation2021; Zhao et al. Citation2021; Li et al. Citation2022).
Both blasting and natural earthquake waves have nonlinear and non-stationary characteristics. How to effectively extract and discriminate the main characteristics of earthquake waves is one of the important issues of current research. At present, the primary methods for processing nonlinear and non-stationary complex signals include fast Fourier transform (FFT), wavelet transform (WT), wavelet packet transform (WPT), Hilbert–Huang Transform (HHT), and machine learning (ML) (Jia et al. Citation2022; Yang et al. Citation2022), which are widely used in earthquake wave feature extraction, discrimination, classification.
In terms of time-frequency analysis methods, The time-frequency analysis and particle motion are employed for separating blasting and earthquakes (Singh et al. Citation2017). Yılmaz et al. relied on two-dimensional time-frequency analysis methods to discriminate quarry blasts from earthquakes (Yılmaz et al. Citation2012). Korrat et al. adopted spectral analysis to calculate source parameters, including scalar moments and corner frequencies in order to discriminate between earthquakes and quarry blasts (Korrat et al. Citation2022). Liu et al. analyzed the time-frequency characteristics on blasting vibration effect by wavelet transform and wavelet package decomposition (Liu et al. Citation2021). Hao and Yao applied the wavelet domain inversion method to determine the source parameters of small and moderate earthquakes, especially the strong aftershocks after a large, disastrous earthquake (Hao J and Yao Citation2011). Heidari and Majidi employed a discrete wavelet theory based on the Mallat method to analyze the acceleration of earthquake records (Heidari and Majidi Citation2021). Hao et al. analyzed the time and frequency of the monitored blasting seismic wave by HHT transformation to obtain the energy distributions of blasting vibration characteristics and seismic wave (Hao et al. Citation2020). Huang et al. proposed an improved HHT method in the spectral representation of earthquake accelerograms to obtain the completely orthogonal IMFs (Huang et al. Citation2018). In terms of machine learning or deep learning methods, an artificial neural network (ANN) with multiwindow spectral data are applied to automate discriminating earthquakes from quarry and mining blasts, and 97% of all recordings were accurately classified (Miao et al. Citation2020). The ML algorithms can be employed to detect the existence of artificial seismic sources in seismicity catalogs as well as to predict the earthquake’s intensity and location, and achieve good accuracy (Abdalzaher et al. Citation2021; Abdalzaher, Soliman, et al. Citation2022; Abdalzaher et al. Citation2023). An efficient ML model for decontaminating the seismicity database was built and achieved 100% discrimination between the earthquakes and quarry blasts relying on the extreme gradient boosting (XGB) model (Abdalzaher, Moustafa, et al. Citation2022). A peak ground acceleration model using ML along with geographic information system, with an accuracy of 96%, was developed (Hamdy et al. Citation2022). The ML model was employed to estimate high-frequency seismic body-wave attenuation, and was useful in assessment of seismic hazards and the damage pattern of earthquakes (Moustafa et al. Citation2023). Yıldırım et al. (Yıldırım et al. Citation2011) used feedforward neural networks (FFNNs), adaptive neural fuzzy inference systems (ANFIS), and probabilistic neural networks (PNNs) to discriminate between earthquakes and quarry blasts. Saad et al. used the scalogram and the capsule neural network to distinguish between earthquakes and quarry blasts (Saad et al. Citation2022).
However, in the above earthquake, waves feature extraction and discrimination methods, although FFT can describe the entire frequency distribution, it cannot simultaneously reflect the overall or local characteristics of the signal in terms of time, frequency, and harsh application conditions (Singh Citation2015). Moreover, there are problems such as frequency component confusion for non-stationary signals (Huang et al. Citation2019). WT/WPT is a Fourier transform with adjustable windows, which cannot avoid the limitations of the Fourier transform. In addition, the selection of wavelet bases can have an impact on the results, and the finite length of wavelet leads to signal energy leakage, causing difficulty in analyzing the time-frequency and energy distribution characteristics (Wang et al. Citation2019; Zhang et al. Citation2022). HHT is composed of an empirical mode decomposition (EMD) and Hilbert transform. As the most important step in HHT, EMD can adaptively decompose the original signal into a series of intrinsic mode functions (IMF) according to the characteristics of the signal itself. However, the decomposition process often contains severe modal aliasing and endpoint divergence effects, which, to some extent, influence the accuracy of signal analysis. Although methods such as ensemble EMD (EEMD) derived from EMD can avoid endpoint effects and modal aliasing in EMD by adding uniformly distributed Gaussian white noise, the completeness of the decomposition results is insufficient, and there is an impact of white noise on the decomposition results (Jin et al. Citation2023). Although machine learning or deep learning can be used for seismic phase recognition, waveform classification, earthquake intensity prediction, etc., there are still problems, such as sample acquisition, high cost, manual intervention, unstable prediction, and sensitive missing data (Álvarez-Vigil et al. Citation2012; Zhang et al. Citation2019; Li et al. Citation2022).
In the current time-frequency analysis methods, the complete ensemble empirical mode decomposition (CEEMD) based on EEMD adds positive and negative paired adaptive white noise signals during the decomposition process. Thus, it can suppress modal aliasing and errors caused by adding noise, and it can improve the completeness of signal decomposition. Moreover, there is no need to rely on the choice of the size of the windows like FFT, nor does it need to determine the wavelet basis function like WT. In addition, the signal decomposition efficiency is high and the local adaptability is good, which can more realistically depict the distribution patterns of time- frequency and energy on various scales.
The earthquake waves decomposed by CEEMD can obtain multiple IMF components, including effective components and noise components. The selection of actual effective IMF components is still a significant issue in current signal processing. Multiscale permutation entropy (MPE), as a method of detecting signal randomness and dynamic mutation, was introduced to detect signal complexity and randomness to identify noise signals and false components (Bandt and Pompe Citation2002). For the random background noise of blasting and natural earthquake monitoring, the complexity and randomness of each IMFs can be estimated by MPE after the adaptive decomposition of CEEMD, and the high-frequency noise or false components can be determined, thereby providing the basis for subsequent data processing and feature extraction.
At the same time, unlike traditional time-frequency analysis methods, HHT is not constrained by the Heisenberg uncertainty principle, and the obtained instantaneous frequency, instantaneous energy, Hilbert spectrum, and marginal spectrum make the analysis results clearer and more intuitive. Therefore, HHT is very suitable for studying the nonlinear and non-stationary earthquake waves. In the study of discrimination between blasting and natural earthquake waves, HHT was presented to extract signal time-domain, frequency-domain, and time-frequency spectrum characteristics effectively.
In this paper, a CEEMD–MPE–HHT method for feature extraction of blasting and natural earthquake waves were proposed to address the current problems of feature extraction and discrimination in the field of earthquake wave research, such as insufficient analysis, poor denoising, jamming environments and artificial intervention and sample requirements in machine learning. Firstly, CEEMD was adopted to decompose earthquake waves to obtain IMF adaptive components with different frequencies. Then, signal randomness was detected by calculating the MPE of the IMFs, and the noise or false components are recognised. Next, HHT was performed on the reconstructed signal to obtain the Hilbert marginal spectrum and Hilbert instantaneous energy spectrum with local detailed time–frequency characteristics. The time–frequency and energy characteristics of the two earthquake waves were also extracted and discriminated. Finally, the earthquake characteristics of the on-site monitoring data for three different scenarios of blasting and natural earthquakes were extracted and discriminated. The feature extraction method proposed in this paper can clearly reflect the specific distribution of earthquake wave energy with time and frequency, and it can extract features from the perspective of time–frequency and energy effectively. It helps to provide decision-making methods for waveform classification, seismic phase identification and safety evaluation of blasting and natural earthquake waves.
Our contribution is: (1) In terms of feature extraction of blasting and natural earthquake waves, a CEEMD-MPE-HHT method is proposed from the perspective of time-frequency and energy. (2) The method proposed in this article can extract time-frequency features more accurately than conventional methods. (3) The proposed method can analyse and compare the safety state and waveform law of blasting and natural earthquake waves according to time-frequency domain and energy distribution.
This paper proposes a CEEMD-MPE-HHT feature extraction method based on the characteristics of blasting and natural earthquake waves to discriminate between the two. In Section 2, the implementation process and method of the CEEMD-MPE-HHT method was proposed. The simulation signal was constructed, and processed using the proposed method and conventional methods, then compared by the SNR/RMSE indicators in Section 3. In Section 4, three different scenarios for analysis and comparison of blasting and natural earthquake waves were selected, including the monitoring equipment and schemes for each scenario. The earthquake waves in various scenarios were compared, extracted and discriminated by the method proposed in this article and conventional methods in Section 5. The feature extraction method of CEEMD-MPE-HHT proposed in this article can extract the earthquake characteristics of blasting and natural earthquake waves and discriminate them effectively.
2. Feature extraction method
In this study, the feature extraction method for blasting and natural earthquake waves mainly includes three parts: CEEMD, MPE, and HHT. The implementation flow is shown in .
2.1. CEEMD
CEEMD is a noise-assisted improvement method based on EEMD. It adds pairs of adaptive white noise with opposite symbols at each stage of signal decomposition to improve the quality of decomposition. It also eliminates the influence of white noise on the decomposition results and is not limited by ensemble times. The reconstructed signal always remains approximately equal to the original signal. The method cannot only suppress EMD mode aliasing but also significantly improve the problems of insufficient completeness and reconstruction errors in EEMD.
Step 1: In the original signal add a pair of positive and negative white noise sequences
and
to obtain new sequences
and
as follows:
(1)
(1)
(2)
(2)
Step 2: Use the cubic spline interpolation method to determine the upper and lower envelope sequences of Then, obtain the mean sequence
of the upper and lower envelopes. Finally, subtract
from
to obtain the residual component as follows:
(3)
(3)
Step 3: Repeat Step 1 for the newly obtained time series until two conditions of the EMD decomposition method are satisfied: (a) the number of local extreme points and zero crossing points is equal or their difference is 1; (b) at any time, the average envelope of the local maximum and local minimum is 0. Then, generate the first
component, namely,
Step 4: Subtract from
to obtain a new sequence
as follows:
(4)
(4)
Step 5: Repeat Steps 1–4 to obtain each component of namely, IMF and a residual component Res (t), as follows:
(5)
(5)
Step 6: Repeat the above steps for to obtain each component of
namely, IMF and a residual component Res (t). The final IMF components and residual components decomposed by CEEMD are obtained by averaging the respective components and residual components of
and
2.2. MPE
The MPE is a method for detecting the randomness and dynamic mutation of the signal. The complexity and randomness of each component can be seen and decomposed by using MPE, and the noisy signals and spurious signals can be distinguished to achieve signal noise reduction. MPE performs multi-scale coarse-graining of the time series and calculates its permutation entropy. The implementation principle is as follows:
Step 1: Coarse-grained processing on sequence is performed to obtain the processed coarse-grained sequence:
(6)
(6)
where
is the scale factor, and
is the time series under different scale factors.
Step 2: Time series is reconstructed to obtain:
(7)
(7)
where
is the embedding dimension, and
is the delay time.
Step 3: The permutation entropy of the time series under scale factor is calculated:
(8)
(8)
where
is the probability of the time occurrence of the symbol sequence.
Step 4: The permutation entropy calculated above is normalized:
(9)
(9)
2.3. HHT
HHT is a new method for dealing with nonlinear and unstable signals. This method generates sequences with distinct characteristics by decomposing signals at various scales, which can accurately reflect the distribution law of signals on different spaces or time scales. HHT is composed of two parts: EMD and Hilbert transform. The signal is decomposed into different IMFs through EMD to provide actual physical meaning for the instantaneous frequency. Meanwhile, Hilbert transform is performed on different IMFs to obtain the Hilbert spectrum and comprehensively describe the time, frequency, and energy spectrum of the signal. The Hilbert transform is described as (Li et al. Citation2016).
3. Simulation analysis
In order to verify the feasibility of CEEMD-MPE feature extraction proposed in this paper, a simulation signal is constructed, which is composed of a stationary sine function with a frequency of f = 100Hz and a Gaussian white noise with a RMSE = 0.2. The sampling frequency is 4KHz, the sampling duration is t = 2s, and the number of sampling points is N = 8000. The synthesized signal, sinusoidal signal, and noise signal are obtained, as shown in .
Figure 2. Simulation signal for feature extraction methods. (a) Simulation signal. (b) Sinusoidal signal. (c) Noise signal.

First of all, for the above simulation signal, three methods, EMD, EEMD, and CEEMD, are adopted for signal processing to obtain the first six IMF components and the corresponding Hilbert marginal spectrum of each element, as shown in .
Figure 3. EMD decomposition and Hilbert marginal spectrum results of simulation signal. (a) EMD decomposition. (b) Hilbert marginal spectrum.

Figure 4. EEMD decomposition and Hilbert marginal spectrum results of simulation signal. (a) EEMD decomposition. (b) Hilbert marginal spectrum.

Figure 5. CEEMD decomposition and Hilbert marginal spectrum results of simulation signal. (a) CEEMD decomposition. (b) Hilbert marginal spectrum.

Next, the simulation signal is decomposed by CEEMD to obtain 13 IMFs and a trend term R. The MPE of each IMF is calculated as shown in . After multiple trials and references, it is determined that when the MPE is greater than 0.6, the corresponding component can be considered abnormal with greater randomness and complexity, which needs to be eliminated. When the abnormal components are removed, the remaining components are reconstructed to obtain a new signal. Then CEEMD is performed to obtain 7 IMFs, 1 trend item, and the corresponding Hilbert marginal spectrum, as shown in . The results were obtained by comparing the signals before and after CEEMD-MPE are shown in .
Figure 6. CEEMD-MPE decomposition and Hilbert marginal spectrum results of simulation signal. (a) CEEMD-MPE decomposition. (b) Hilbert marginal spectrum.

Table 1. MPE of each IMF.
Furthermore, in order to verify the processing effect of the CEEMD-MPE method for the simulation signal, the WT and WPT methods were compared, and the results were obtained according to the SNR (signal to interference plus noise ratio) and RMSE (root mean squared error) indicators as shown in . It can be seen that the proposed method has the highest SNR and the lowest RMSE, which can extract the actual signal from the simulation signal more efficiently.
Table 2. Comparison of simulation signal processing effects.
The following conclusions can be drawn by :
There exists significant modal aliasing in the first six components of EMD, the first four components of EEMD and the first four components of CEEMD. The Hilbert marginal spectrum analysis of each component shows that the frequency of the first three components of EMD, EEMD and CEEMD exceeds 250 Hz, far exceeding the 100 Hz frequency of the actual signal. This finding indicates that this component contains high-frequency noise or false components.
The modal aliasing phenomenon still exists in the simple implementation of CEEMD. MPE was adopted to detect the randomness and complexity of each component. When the abnormal components were removed, the remaining components were reconstructed and decomposed to obtain, as shown in . The figure shows that the modal aliasing phenomenon of each IMF is suppressed. The corresponding Hilbert marginal spectrum shows that the frequency of each the component is within the 100 Hz of the simulation signal, and the signal energy is mainly concentrated in the first five components of IMF1–IMF5.
The comparison of the original signal and the processed signal by the CEEMD–MPE () demonstrates that the feature extraction method proposed in this work can reduce the burrs and irregularities in the signal while retaining the original signal feature information effectively and reflect the signal characteristics better.
In summary, the CEEMD–MPE method proposed in this work can eliminate the modal aliasing phenomenon of the original signal, detect abnormal components, remove high-frequency noise, retain actual information and improve the analysis accuracy compared with the conventional methods effectively. It can provide an effective approach for the extraction and discrimination of the characteristics of earthquake waves.
4. Case source
For blasting and natural earthquake waves, three different scenarios of blasting earthquake and three different magnitudes of natural earthquake were selected as case sources in this article.
4.1. Blasting excavation case
4.1.1. Tunnel blasting
The Huangdao groundwater-sealed tunnel rock mass blasting engineering is located in Huangdao District, Qingdao City, Shandong Province (). This project is the first large-scale groundwater-sealed tunnel oil depot project in China. The designed storage capacity is 3 million m3, is divided into two individual projects: underground and above-ground. The underground project has a total of three groups of tunnels, consisting of nine main tunnels, five water curtain tunnels, six process shafts, construction tunnels, ventilation tunnels, etc. The section of the main tunnel is a straight wall and circular arch, with a span of 20 m, a height of 30 m and a total length of 5.6 km. The rock mass type is mainly grade II.
The layout of the site blasting vibration monitoring measuring points is shown in . At the blasting area rear, the 1# and 3# measuring points are arranged on the rock wall at the foot of the side wall; the 2# measuring point is arranged on the side wall bolt; the 4# measuring point is arranged on the top of the main tunnel; and the 5# measuring point is arranged in the adjacent tunnel. The self-developed blasting recorder BJY-III is utilized for monitoring. The monitoring system shown in is established, the sampling frequency is f = 5 KHz, and each measuring point collects the particle vibration velocity in three directions. The on-site blasting vibration monitoring is shown in .
4.1.2. Expressway roadbed blasting
The expressway roadbed blasting case was conducted on the concrete foundation at the tunnel entrance of the Eight Acre Land Tunnel between Fu-Yin expressway and Han-Shi high-speed railway in Shiya, Hubei, China. The Eight Acre Land Tunnel is located on Shuang Gao Road and is an important passage connecting Shiyan East Railway Station and the main urban area of Shiyan. The total length of the route is 3.18 km, and it is located in a mountainous area with a complex environment and is a fast track. The schematic diagram of tunnel excavation blasting monitoring is shown in and the blasting source of the tunnel is 300 m away from the entrance. Blasting vibration monitoring on the concrete foundation is shown in .
4.1.3. Earthwork excavation blasting
The earthwork excavation blasting case is at the new site of Dongfeng Vehicle Factory in Shiyan City, Hubei Province, China. The new site of Dongfeng Vehicle Factory is located at Fazhan Avenue, the main road of Shiyan City, and the total excavation volume is 6.32 million m3. Many mountain slopes and civil buildings are found nearby, and the blasting area and vibration monitoring are shown in , respectively. The distance between the blasting source and the monitoring point is about 50 m.
In sum, three blasting earthquake scenarios and monitoring equipment parameters are summarized in . The time series of radial, tangential, and vertical blasting vibration velocities in three scenarios monitored by a blasting recorder are shown in (in which, scenario 1 takes the measurement point 4 # at the top arch of the main tunnel, as an example). The data collection duration is 1.6s, 3.5s, and 4.8s, respectively. From the original three-axis velocity time series in , it can be preliminarily seen that: (1) The entire blasting time series are relatively short (within a few seconds), and the waveform exhibits sudden, non-stationary, nonlinear characteristics, and there are multiple peaks with precise intervals between them. (2) There are differences in the characteristics of blasting vibration in different scenarios. The waveform of tunnel blasting is greatly affected by the initiation of detonators, with concentrated amplitude. The difference between the initiation of detonators in earthwork and roadbed blasting are insignificant, and the amplitude is relatively dispersed. At the same time, the amplitude of tunnel blasting is relatively large and the vibration duration is short, while the amplitude of earthwork and roadbed blasting is relatively small and the vibration duration is relatively long.
Table 3. Three scenarios and monitoring equipment parameters.
4.2. Natural earthquake case
Original data of natural earthquake cases with different magnitudes were obtained from the Pacific Earthquake Engineering Research Centre (https://ngawest2.berkeley.edu/) Ground Motion Database. In order to compare the vibration characteristics of blasting with natural earthquake waves in different scenarios and increase the natural earthquake magnitude range, the 1972 earthquake with a magnitude of Ms = 6.6, the 2009 earthquake with a magnitude of Ms = 5.4, and the 2010 earthquake with a magnitude of Ms = 4.0 was taken from the PEER database as examples for research. The information of three types of natural earthquake scenarios is shown in .
Table 4. Three natural earthquakes scenarios information.
The horizontal-1, horizontal-2, and vertical, original acceleration time series of the seismic network with magnitudes of Ms = 6.6, M = 5.4, and Ms = 4.0 were obtained as shown in . It can be preliminarily seen that: (1) the effective time series of the entire earthquake vibration is relatively long, with 70s, 45s, and 35s, respectively. The overall waveform presents non-stationary and nonlinear characteristics, and there are multiple peaks with gradually decreasing amplitudes. (2) There are significant differences in the vibration characteristics of natural earthquakes under different magnitudes. The larger the importance of the earthquake in the scenario, the longer the vibration duration, the slower the amplitude attenuation, and the longer the energy release, which poses greater harm to the building. At a magnitude of Ms = 4.0, the amplitude is the smallest and the attenuation is the fastest, with less damage.
In sum, through the analysis and comparison of three different scenarios of blasting and natural earthquake waves, it can be preliminarily concluded that there are significant differences in waveform characteristics, vibration duration, vibration amplitude, attenuation speed between the two. Furthermore, for three types of scenarios or magnitudes under blasting and natural earthquake waves, there are significant differences in vibration characteristics among different scenarios, which can further help discriminate between additional blasting and natural earthquake waves.
5. Results and discussion
5.1. Comparative analysis of data processing
Taking the radial components of blasting earthquake with tunnel blasting (scenario 1 in blasting earthquake) and the horizontal-1 component of a natural earthquake with magnitude Ms = 6.6 (scenario 1 in natural earthquake) as examples, four feature extraction methods were adopted, namely Wavelet, Wavelet Package, EMD-MPE, and CEEMD-MPE, to obtain the comparison result shown in (orange dashed box). The correlation coefficients between the blasting and natural earthquake waves shown in under four feature extraction methods were calculated by calculating the correlation coefficients with the original data, The following preliminary conclusions can be drawn:
Figure 13. Comparison of feature extraction methods for original data of blasting and natural earthquake waves.

Table 5. Comparative analysis between this method and conventional methods.
The correlation coefficient between natural earthquakes before and after processing with the four methods is higher than that of blasting earthquakes, indicating that due to different vibration monitoring methods, the noise of blasting earthquake monitoring data is relatively higher, while the noise of earthquake monitoring data is somewhat lower. The monitoring data by the current blasting recorder in blasting earthquake needs further processing due to the influence of the construction environment and monitoring equipment.
Furthermore, among the four feature extraction methods, the Wavelet Package method has the smallest correlation coefficient and several corresponding peaks are also filtered out, resulting in the erroneous removal of effective data. The correlation coefficient of the Wavelet method is relatively high, but the denoising effect is poor, the burrs are still noticeable, and the noise removal is incomplete. The correlation coefficient of the EMD-MPE method lies between the Wavelet and Wavelet Package methods, and the peaks are removed, resulting in the peaks not being obvious. This further demonstrates the presence of modal aliasing in EMD during signal decomposition, which makes it difficult to accurately distinguish between effective and noise components, resulting in distortion in the final data processing. Meanwhile, by comparing , it can be seen that the EMD-MPE method performs better results in processing blasting earthquake waves than natural earthquake waves.
The CEEMD-MPE method proposed in this article has the highest correlation coefficient in the processing of blasting and natural earthquake waves. Through waveform comparison, it can be seen that the processed results not only remove noise but also retain the features of the original data, providing a foundation for subsequent feature extraction and discrimination of earthquake waves.
In addition, compared to the existing methods, the CEEMD-MPE method can better suppress modal aliasing and signal noise in blasting and natural earthquake waves, and extract effective components. Meanwhile, when the time-frequency analysis of blasting and natural earthquake waves is conducted, the former must process the original data, while the latter can be performed time-frequency analysis and feature extraction directly.
5.2. Hilbert marginal spectrum
In order to analyse the energy concentration of earthquake waves at unit frequency, the Hilbert marginal spectra of velocity and acceleration time series of blasting and natural earthquake waves were calculated, respectively. At the same time, to further analyse the energy ratio of earthquake wave in each frequency band, the vibration frequency of blasting earthquake wave is divided into six frequency bands: 0–50, 50–100, 100–200, 200–300, 300–400 and 400–500 Hz. The square of the Hilbert spectrum of the velocity series in the three scenarios of blasting earthquake are integrated in each frequency band interval (taking the radial component as an example) to obtain the energy proportion in different frequency bands of each scenario shown in . Similarly, the vibration frequency of natural earthquake wave is divided into six frequency bands: 0–1, 1–2, 2–3, 3–4, 4–5, and >5 Hz. The square of the Hilbert spectrum of the acceleration series under three magnitudes of natural earthquake wave are integrated in each frequency band interval (taking the horizontal-1 component as an example) to obtain the energy proportion of each frequency band of each scenario shown in , and the energy proportions in different frequency bands in several scenarios of blasting and natural earthquake are as shown in .
Table 6. Energy proportion in different frequency bands of each blasting scenario and natural earthquake.
From and , it can be seen that:
In , according to the energy proportion in different frequency bands for three types of blasting scenarios and three magnitudes, as the frequency bands increase, the energy of the two types of earthquake waves shows a downward trend in the corresponding frequency bands. However, the energy of blasting earthquakes decreases faster than that of natural earthquakes, indicating that the former has faster energy attenuation, while the latter has slower attenuation, resulting in greater harm. The blasting earthquake energy is mainly concentrated at 0-200 Hz, while the natural earthquake energy is concentrated at 0-3 Hz, and the degree of energy release varies among different blasting earthquake scenarios and natural earthquake magnitudes.
In terms of the energy distribution of blasting earthquake waves, the energy proportion of earthwork blasting, roadbed blasting, and tunnel blasting in the 0-50Hz frequency band is 99.5%, 97.9%, and 77.3%, respectively. The energy proportion within the 0-100Hz frequency band is 100%, 100%, and 87.5%, respectively. The energy proportion in the 0-200Hz frequency band is 100%, 100%, and 96.3%, respectively (). In the three scenarios of blasting earthquakes, the energy of open blasting is mainly concentrated within 50Hz, and the energy of tunnel blasting is mainly concentrated within 200Hz. Moreover, the dominant vibration frequency of hard rock mass is relatively high, while that of soft rock soil is relatively low.
In terms of energy distribution of natural earthquake waves, the energy proportion of natural earthquake with magnitudes Ms = 4.0, Ms = 4.5, and Ms = 6.6 in the 0-1Hz frequency band are 29.4%, 54.6%, and 93.2%, respectively; The energy proportion in the 0-2Hz frequency band is 50.7%, 92.6%, and 98.2%, respectively; The energy proportion in the 0-3 Hz frequency band is 64.9%, 96.7%, and 99.1%, respectively; The energy proportion in the 0-4 Hz frequency band is 78.0%, 98.3%, and 99.7%, respectively; The energy proportion in the 0-4 Hz frequency band is 91.1%, 100%, and 100%, respectively (). This indicates that the higher the magnitude of a natural earthquake, the more energy is released in the corresponding frequency band. Moreover, the energy with magnitude Ms = 4.0 is mainly concentrated within 0 ∼ 4Hz, while the energy with magnitude Ms = 5.6 and Ms = 6.6 is mainly concentrated within 0 ∼ 1Hz. The larger the magnitude, the closer the dominant vibration frequency of the natural earthquake is to the natural frequency of buildings, and the greater the harm.
Therefore, according to the energy proportion analysis of different frequency bands of blasting and natural earthquake, it is possible to discriminate between blasting and natural earthquakes based on the energy change, energy proportion, energy attenuation and other characteristics of the corresponding frequency bands, and further distinguish between blasting and earthquakes in different scenarios and magnitudes.
5.3. Hilbert instantaneous energy
In order to reflect the energy accumulation of blasting and natural earthquake waves in the entire frequency domain at each moment, the Hilbert instantaneous energy spectrum of velocity time series (taking the radial components as an example) and acceleration time series (taking the horizontal-1 as an example) for each scenario of both were calculated, respectively and the results are shown in and . The following conclusions can be drawn:
Energy duration. The energy duration of blasting is relatively short, usually within 3 seconds, while the energy duration of natural seismic earthquake is fairly long, typically lasting for tens of seconds or more.
Peaks characteristics. Blasting and natural earthquakes usually have multiple peaks. The number of blasting earthquake peaks is relatively small but significant, with peak intervals ranging from tens to hundreds of milliseconds, which represents the actual initiation time of the detonator segment and can reflect millisecond blasting. However, there are many natural earthquake peaks with a decreasing trend and large intervals.
Energy attenuation. The overall attenuation of blasting earthquake energy is rapid, and the energy increases rapidly with the initiation of the detonator, while that of natural earthquake energy is slow and the energy shows a decreasing trend.
Furthermore, in three scenarios of blasting earthquake, the energy peaks of tunnel blasting are more pronounced than that of open-pit blasting, which can better identify the initiation time of detonator. However, the open blasting has many more minor and useless energy peaks due to the influence of environmental influence. This indicates that the blasting characteristics are more significant in hard rock mass, while in soft rock and soil, such as open air, the blasting characteristics will be affected by environmental factors. In the three magnitudes of natural earthquake, the magnitude Ms = 4 has the lowest energy peak and the fastest attenuation, followed by magnitude Ms = 5, while the magnitude Ms = 6 has the highest energy peak and the slowest attenuation. This further indicates that the larger the magnitude of natural earthquake, the slower the attenuation, the longer the energy release time, and the greater the destructive force.
In sum, for the blasting earthquake, as each peak of the Hilbert instantaneous energy spectrum represents the energy release caused by detonator initiation, the millisecond blasting can be identified and the blasting effect can be evaluated. For the natural earthquake, there are multiple obvious peaks throughout the entire time series, showing an overall attenuation trend, and the attenuation trend is slow. The Hilbert instantaneous energy spectrum of blasting earthquake waves reflects the initiation time of each detonator section in millisecond blasting. In contrast, natural earthquake waves reflect the persistence, randomness, and complexity of crustal energy release.
6. Conclusion
In terms of the non-stationary, non-linear and transient characteristics of blasting and natural earthquake waves, as well as the situation that the current feature extraction methods for both have issues such as inaccuracy and interference, the CEEMD–MPE–HHT time–frequency analysis process, which is seldom explored in blasting and natural earthquake waves, is proposed to conduct feature extraction and discrimination. Three representative scenarios of both were selected for feature extraction and discrimination. The following conclusions can be drawn:
The proposed CEEMD–MPE–HHT combines the adaptability of signal decomposition and the detectability of signal anomalies. In comparison with conventional Wavelet, EMD related or other methods, the proposed can better suppress modal aliasing and signal noise in the processing of blasting and natural earthquake waves through simulated and measured data, and extract and discriminate the characteristics of the two earthquake waves from the time–frequency and energy perspective accurately and effectively.
The blasting earthquake energy is mainly concentrated at 0-200Hz, while the natural earthquake energy is concentrated at 0-3Hz, and the degree of energy release varies among different blasting earthquake scenarios and natural earthquake magnitudes. The former has faster energy attenuation, while the latter has slower attenuation, resulting in more significant harm. Furthermore, the energy release from earthwork blasting, roadbed blasting, and tunnel blasting varies from fast to slow, while the energy release of natural earthquakes with magnitudes of Ms = 6.6, Ms = 5.6, and Ms = 4.0 varies from slow to fast. The larger the magnitude of a natural earthquake, the closer the dominant vibration frequency is to the natural frequency of buildings, and the longer the vibration duration, the greater the harm ().
According to the Hilbert instantaneous energy spectrum, blasting earthquake waves have the characteristics of short energy duration, significant peaks, fast energy attenuation, and can identify millisecond blasting. Natural earthquake waves have the features of long-energy duration, multiple and decreasing peaks, large peak intervals, and slow and decreasing energy attenuation. Meanwhile, in terms of blasting earthquake, the peak energy of tunnel blasting is more pronounced than that of open-air blasting, while in terms of natural earthquakes, the larger the magnitude of natural earthquakes, the slower the attenuation, the longer the energy release time, and the greater the destructive force ().
The time-frequency feature extraction method proposed in this article can extract and discriminate the vibration characteristics of blasting and natural earthquake waves accurately and effectively. In terms of blasting, it can help to identify the attributes of millisecond blasting, analyze vibration effects, assist in safety evaluation, and optimize blasting design and construction. In terms of natural earthquakes, it’s conductive to waveform recognition, parameter inversion, earthquake disaster reduction, structural seismic resistance, etc.
It should be pointed out that the limitations of this article mainly lie in: (a) Due to experimental conditions, the blasting and natural earthquake scenarios selected in this article are not enough to cover all scenarios. In the next step of work, we will continue to enrich scenarios and conduct further study proposed in this article. (b) As a result of the complexity of the seismic medium structure and source parameters, the features of blasting and natural earthquake were extracted and discriminated only from the perspective of time-frequency energy, and the in-depth research of earthquake waves will be the focus of our subsequent work.
Table 7. Comparison of features between blasting and natural earthquakes.
Data availability statement
The data that support the findings of this study are available from the corresponding author upon reasonable requests.
Disclosure statement
No potential conflict of interest was reported by the authors.
Additional information
Funding
References
- Abdalzaher MS, Moustafa SSR, Abd-Elnaby M, Elwekeil M. 2021. Comparative performance assessments of machine-learning methods for artificial seismic sources discrimination. IEEE Access. 9:65524–65535. doi:10.1109/ACCESS.2021.3076119.
- Abdalzaher MS, Moustafa SSR, Hafiez HEA, Ahmed WF. 2022. An optimized learning model augment analyst decisions for seismic source discrimination. IEEE Trans Geosci Remote Sens. 60:1–12. doi:10.1109/TGRS.2022.3208097.
- Abdalzaher MS, Soliman MS, El-Hady SM. 2023. Seismic intensity estimation for earthquake early warning using optimized machine learning model. IEEE Trans Geosci Remote Sens. 61:1–11. doi:10.1109/TGRS.2023.3296520.
- Abdalzaher MS, Soliman MS, El-Hady SM, Benslimane A, Elwekeil M. 2022. A deep learning model for earthquake parameters observation in IoT system-based earthquake early warning. IEEE Internet Things J. 9(11):8412–8424. doi:10.1109/JIOT.2021.3114420.
- Álvarez-Vigil AE, González-Nicieza C, López Gayarre F, Álvarez-Fernández MI. 2012. Predicting blasting propagation velocity and vibration frequency using artificial neural networks. Int J Rock Mech Min Sci. 55:108–116. doi:10.1016/j.ijrmms.2012.05.002.
- Bandt C, Pompe B. 2002. Permutation entropy: a natural complexity measure for time series. Phys Rev Lett. 88(17):174102. doi:10.1103/PhysRevLett.88.174102.
- Hamdy O, Gaber H, Abdalzaher MS, Elhadidy M. 2022. Identifying exposure of urban area to certain seismic hazard using machine learning and GIS: a case study of greater Cairo. Sustainability. 14(17):10722. doi:10.3390/su141710722.
- Hao J, Yao Z. 2011. Determination of regional earthquake source parameters in wavelet domain. Sci China Earth Sci. 55(2):296–305. doi:10.1007/s11430-011-4341-8.
- Hao X, Xu Y, Liu H. 2020. Study on propagation characteristics of seismic wave in rock tunnel excavation blasting. IOP Conf Ser: Earth Environ Sci. 455(1):012166. doi:10.1088/1755-1315/455/1/012166.
- Heidari A, Majidi N. 2021. Earthquake acceleration analysis using wavelet method. Earthq Eng Eng Vib. 20(1):113–126. doi:10.1007/s11803-021-2009-8.
- Huang D, Cui S, Li X. 2019. Wavelet packet analysis of blasting vibration signal of mountain tunnel. Soil Dyn Earthquake Eng. 117:72–80. doi:10.1016/j.soildyn.2018.11.025.
- Huang J-Z, Yuan S-J, Li D-S, Li H-N. 2023. A kernel canonical correlation analysis approach for removing environmental and operational variations for structural damage identification. J Sound Vib. 548:117516. doi:10.1016/j.jsv.2022.117516.
- Huang T-L, Lou M-L, Chen H-P, Wang N-B. 2018. An orthogonal Hilbert-Huang transform and its application in the spectral representation of earthquake accelerograms. Soil Dyn Earthquake Eng. 104:378–389. doi:10.1016/j.soildyn.2017.11.005.
- Jia L, Chen H, Xing K. 2022. Rapid classification of local seismic events using machine learning. J Seismol. 26(5):897–912. doi:10.1007/s10950-022-10109-5.
- Jin S-Y, Su Y, Guo C-J, Fan Y-X, Tao Z-Y. 2023. Offshore ship recognition based on center frequency projection of improved EMD and KNN algorithm. Mech Syst Sig Process. 189:110076. doi:10.1016/j.ymssp.2022.110076.
- Kan J, Dou L, Li X, Li J, Bai J, Cao J, Liu M. 2023. Effect of initiation pattern on rock damage and blasting seismic under multi-hole blasting. Geomatics Nat Hazards Risk. 14(1) doi:10.1080/19475705.2023.2192334.
- Korrat IM, Lethy A, ElGabry MN, Hussein HM, Othman AS. 2022. Discrimination between small earthquakes and quarry blasts in Egypt using spectral source characteristics. Pure Appl Geophys. 179(2):599–618. doi:10.1007/s00024-022-02953-w.
- Li Q, Li Y, He Q. 2022. Mine-microseismic-signal recognition based on LMD–PNN method. Applied Sciences. 12(11):5509. doi:10.3390/app12115509.
- Li X, Li Z, Wang E, Feng J, Kong X, Chen L, Li B, Li N. 2016. Analysis of natural mineral earthquake and blast based on Hilbert–Huang transform (HHT). J Appl Geophys. 128:79–86. doi:10.1016/j.jappgeo.2016.03.024.
- Li XY, Guan YH, Law SS, Zhao W. 2022. Monitoring abnormal vibration and structural health conditions of an in-service structure from its SHM data. J Sound Vib. 537:117185. doi:10.1016/j.jsv.2022.117185.
- Liu X, Zeng Y, Li H, Xia X, Li J, Li Z. 2021. Research on blasting vibration effect and time-frequency characteristics of vibration signals in a road corridor at xianning nuclear power station. J Vibroeng. 23(4):823–846. doi:10.21595/jve.2021.21848.
- Man K, Liu X, Song Z. 2021. Rock blasting vibration velocity and excavation damaged zone for the high-level radioactive waste geological disposal. Geomatics Nat Hazards Risk. 12(1):2590–2606. doi:10.1080/19475705.2021.1970032.
- Miao F, Carpenter NS, Wang ZM, Holcomb AS, Woolery EW. 2020. High-accuracy discrimination of blasts and earthquakes using neural networks with multiwindow spectral data. Seismol Res Lett. 91(3):1646–1659. doi:10.1785/0220190084.
- Moustafa SSR, Mohamed G-EA, Elhadidy MS, Abdalzaher MS. 2023. Machine learning regression implementation for high-frequency seismic wave attenuation estimation in the Aswan Reservoir area, Egypt. Environ Earth Sci. 82(12). doi:10.1007/s12665-023-10947-7.
- Saad OM, Soliman MS, Chen Y, Amin AA, Abdelhafiez HE. 2022. Discriminating earthquakes from quarry blasts using capsule neural network. IEEE Geosci Remote Sensing Lett. 19:1–5. doi:10.1109/LGRS.2022.3207238.
- Singh AP. 2015. Seismic hazard evaluation in Anjar city area of western India: microtremor array measurement. Soil Dyn Earthquake Eng. 71:143–150. doi:10.1016/j.soildyn.2015.01.009.
- Singh AP, Kumar MR, Kumar S, Sateesh A, Mahesh P, Shukla A, Talukdar R. 2017. Seismicity and subterranean sounds in the northwest Deccan volcanic province of India. Bull Seismol Soc Am. 107(3):1129–1135. doi:10.1785/0120160143.
- Wang T, Han Q, Chu F, Feng Z. 2019. Vibration based condition monitoring and fault diagnosis of wind turbine planetary gearbox: a review. Mech Syst Sig Process. 126:662–685. doi:10.1016/j.ymssp.2019.02.051.
- Weng S, Chen Z, Yan Y, Xiao C, Li R, Li J. 2022. Sensitivity-based constitutive parameter identification of nonlinear structures with unknown input earthquake excitation. J Sound Vib. 537:117188. doi:10.1016/j.jsv.2022.117188.
- Xia J, Li Y, Cheng Y, Li J, Tian S. 2021. Research on compressive sensing of strong earthquake signals for earthquake early warning. Geomatics Nat Hazards Risk. 12(1):694–715. doi:10.1080/19475705.2021.1889689.
- Yang N, Lei Y, Li J, Hao H, Huang J-s 2022. A substructural and wavelet multiresolution approach for identifying time-varying physical parameters by partial measurements. J Sound Vib. 523:116737. doi:10.1016/j.jsv.2021.116737.
- Yıldırım E, Gülbağ A, Horasan G, Doğan E. 2011. Discrimination of quarry blasts and earthquakes in the vicinity of Istanbul using soft computing techniques. Comput Geosci. 37(9):1209–1217. doi:10.1016/j.cageo.2010.09.005.
- Yılmaz Ş, Bayrak Y, Çınar H. 2012. Discrimination of earthquakes and quarry blasts in the eastern Black Sea region of Turkey. J Seismol. 17(2):721–734. doi:10.1007/s10950-012-9348-x.
- Zhang C, Mousavi AA, Masri SF, Gholipour G, Yan K, Li X. 2022. Vibration feature extraction using signal processing techniques for structural health monitoring: a review. Mech Syst Sig Process. 177:109175. doi:10.1016/j.ymssp.2022.109175.
- Zhang J, Jiang R, Li B, Xu N. 2019. An automatic recognition method of microseismic signals based on EEMD-SVD and ELM. Comput Geosci. 133:104318. doi:10.1016/j.cageo.2019.104318.
- Zhao Y, Xu H, Yang T, Wang S, Sun D. 2021. A hybrid recognition model of microseismic signals for underground mining based on CNN and LSTM networks. Geomatics Nat Hazards Risk. 12(1):2803–2834. doi:10.1080/19475705.2021.1968043.
- Zhou H, Che A, Wang L, Wang L. 2020. Investigation and mechanism analysis of disasters under Hokkaido Eastern Iburi earthquake. Geomatics Nat Hazards Risk. 12(1):1–28. doi:10.1080/19475705.2020.1856201.