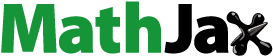
Abstract
In this study, the region along the Mongolia-China-Russia Economic Corridor was selected as the subject. Here, a freeze-thaw erosion model would be built and used. By contrasting the probability of a geological disaster under the effect of an influencing factor with the probability of a geological disaster in the whole research region, the information value model is realized. In order to identify the most prone factors of freeze-thaw erosion in the research region, we replaced the possibility of geological disasters with the possibility of freeze-thaw erosion. The study region under each influencing factor was separated into five grades. Each grade’s information value was computed using the principles of the information quantity model and the freezing and thawing erosion grade division. Since there were positive and negative information values, for the purposes of calculating probabilities, they were normalized to obtain the similar information value for each grade before calculating the overall similar information value of each factor. The similar information value model (SIVM) underlies this procedure determines the weight. We compared the Analytic Hierarchy Process (AHP) and SIVM with the results of 0.070–0.758 and 0.063–0.776, respectively. Finally, the outcomes were confirmed through visual interpretation.
1. Introduction
Considering the research history and status of freezing and melting erosion at home and abroad, it has become an important environmental problem restricting the sustainable development of the global society and economy. An increasing number of scholars worldwide have begun to pay attention to freeze-thaw erosion. The United States and Canada are at the forefront of research in this area (Sun et al. Citation2019). China started later and has recently seen a rise in the number of academics researching freeze-thaw erosion (Frauenfeld et al. Citation2004; Eigenbrod Citation1996; Harden and Mathews Citation2002). However, little research has considered the spatiotemporal distribution and intensity classification evaluation, as well as the formation mechanism and evolution. Climatic conditions drive the freeze-thaw erosion process. However, in recent years, it is not clear how the climate characteristics and changing trends drive conditions that impact soil freeze-thaw erosion.
Many scholars have analyzed the impact of temperature, vegetation, terrain, snow, hydrological conditions, and other local factors on the freeze-thaw cycle of the active layer, which are relatively significant (Vecellio and Frauenfeld Citation2022; Juselius et al. Citation2022; Brandon et al. Citation2020). Based on GIS technology, Li et al. (Citation2011) used slope, slope direction, annual precipitation, annual temperature difference, vegetation covering, and other factors as intensity evaluation indicators in their study of freeze-thaw erosion intensity in the Sanjiangyuan area. Guo et al. (Citation2017) carried out a quantitative evaluation and spatial pattern analysis of the Qinghai-Tibet Plateau. Furthermore, they introduced a freeze-thaw erosion dynamic factor and precipitation to build a freeze-thaw erosion evaluation model. Combining the relevant factors that influence the degree of freeze-thaw erosion, the distribution of freeze-thaw areas across China was preliminary mapped by Wang et al. (Citation2021) after discussing the effects of temperature and precipitation on freeze-thaw action and freeze-thaw erosion in climatic and environmental settings. Liu et al. (Citation2023) studied the relationship between the seasonal freezing and thaw cycle and soil porosity in the middle and high latitudes. The results found that the number of freeze and thaw cycles expanded the soil porosity (R2 = 0.503, p < 0.05, R2 expresses the degree of explanation of the relationship between variables, the percentage degree, and the p value is an indicator of the confidence of the test sample), which eventually led to the decrease of soil strength and affected the degree of erosion.
Li et al. (Citation2018) used the annual temperature range, slope, aspect, vegetation, and annual precipitation as grading evaluation indicators of freeze-thaw erosion in the Yili River Valley, and used the model classification method to evaluate the erosion characteristics. In order to study the temporal and geographical variations in the freezing and thawing processes of the permafrost active layer and seasonal permafrost in the Yellow River source zone, Luo et al. (Citation2014) collected soil temperature and moisture data from four sites. Wang et al. (Citation2017) obtained the required conclusions by studying the annual difference in temperature, precipitation, slope, slope orientation, and vegetation cover during a comprehensive evaluation of freeze-thaw erosion sensitivity on the Tibetan Plateau. For the grading evaluation of freeze-thaw erosion, Li et al. (Citation2015) used six elements: the annual freeze-thaw daily circulation time, slope, aspect, vegetation cover, average daily freeze-thaw phase change water value, and average annual precipitation, and calculated the flow, assignment standard, and weight.
Oztas (Taskin and Ferhan Citation2003) found that soil stability decreased with increased water content during freezing and changed with soil ype. To determine if black soil wet aggregate stability changes during freeze-thaw, Wang et al. (Citation2010) performed an indoor test. According to the test results, soil aggregate stability is impacted by the water content condition. Shi et al. (Citation2012)’s quantitative research and analysis on freeze-thaw erosion focused on key variables such as annual temperature range, vegetation coverage, precipitation, slope, and sun radiation. Li et al. (Citation2015) selected slope, average precipitation during the thawing period, annual temperature range, vegetation coverage, average minimum temperature during the freezing period, and annual precipitation as graded evaluation indexes of freeze-thaw erosion influence factors for assessment. Jing et al. (Citation2003)’s analysis of the form and risk level of freeze-thaw erosion led them to conclude that more research is necessary to understand the influencing variables and causality of this phenomenon. Nevertheless, these influencing factors are not comprehensive enough, and some are even interrelated within the same experiment, which causes overlapping influence effects of the same factor. We need further analysis of the influence factors to find multiple angles based on the existing data and avoid the superposition effect of the same factor as much as possible.
Lu et al. (Citation2019) used hierarchical analysis to determine the weight of various factors and analyzed the distribution of freeze-thaw erosion along the Brahmaputra River Basin. Guo and Jiang (Citation2017) determined the weight of evaluation factors using Analytic Hierarchy Process and analyzed freeze-thaw erosion in 2000 and 2015, and found that the erosion improved during that time. Xu et al. (Citation2011) calculated the information quantity value of each influencing factor for a disaster, used the GIS and AHP coupling model, and finally generated and mapped each factor’s information quantity. The hierarchical analysis method is becoming increasingly widely used, but this semi-quantitative method has certain limitations. It requires further study of its advantages and disadvantages, reduction of its limitations, or combination with other semi-quantitative methods to find more accurate and reasonable weight determination methods.
The comprehensive index evaluation method uses a different evaluation factor for obtaining a comprehensive evaluation index (2022). The larger the comprehensive evaluation index, the stronger the freeze-thaw erosion; otherwise, it is weaker. Lu et al. (Citation2019) obtained the range of freeze-thaw erosion strength by using the comprehensive index evaluation method and showed its distribution in the Brahmaputra River Basin. Shi et al. (Citation2012) analyzed the erosion intensity by combining the main impact elements of freeze-thaw erosion. Lv et al. (Citation2016) adopted a multi-factor comprehensive analysis method, and selected the freeze-thaw erosion sensitivity as part of the evaluation basis for the refined ecological sensitivity of the western Sichuan Plateau. For the purpose of defining the boundary and evaluating the erosion strength of the freeze-thaw erosion zone in Gansu Province, Li et al. (Citation2015) integrated two approaches: the complete evaluation index and relative grading assessment. The comprehensive index evaluation method is widely used and is a reasonable evaluation model in different fields. Such as the fields of economics (Wang et al. Citation2021; Oliveira et al. Citation2021), environmental science (Ali et al. Citation2023), architecture (Wang, Citation2022), and medicine (Manzi et al. Citation2022; Kuchera Citation2022), among other. This study needs to further investigate the rationality of spatiotemporal analysis in freeze-thaw erosion.
Li et al. (Citation2019) analyzed the superiority and limitations in disaster susceptibility assessment by using information value model and logistic regression models and found that the accuracy and success rate of the information model is slightly higher. But the information model cannot consider the contribution weight difference of the disaster influencing factors, and the logical regression model cannot express the correlation between the internal level and the disaster occurrence. To improve the accuracy and precision of disaster-prone assessment, Yang et al. (Citation2020) proposed a weighted information value model. Zhu et al. (Citation2004) used a GIS spatial analysis function and an information model to classify risk. According to the quantity of information, they created a distribution zoning map, where the information amount was calculated by stacking the historical disaster distribution density map and the distribution map of various influencing factors. Fan et al. (Citation2018) used a logistic regression model, information value model, and two-model coupling analysis and generated the geological disaster susceptibility evaluation. The evaluation results of the logistic regression model and information value model are more reasonable and have higher accuracy. Information value model-based hazard evaluation has not been widely applied in the spatial and temporal analysis of freeze-thaw erosion.
AHP is the main focus of the majority of the current research techniques on the variables influencing freeze-thaw erosion, but the hierarchical analysis technique is subjective, which may lead to a significant departure from the real results. How to increase precision and more objectively characterize the degree to which various elements influence freeze-thaw erosion is one of our primary focus. The information value model is also frequently applied to geological catastrophes like mudslides and landslides. Therefore, how to apply the idea of informative modelling to erosion, which is also a natural geological hazard, is another focus of this article. Based on the above, this article improve the information value model in order to integrate our two focus of inquiry and introduce our Similar Information value model. This article will develop a spatio-temporal analysis model of freeze-thaw erosion based on information approach, using the China-Mongolia-Russia Economic Corridor as an example, and assess the system’s viability.
In order to create two result maps and a comparative analysis, this article uses a hierarchical analysis approach to classify the freeze-thaw erosion analysis results of the China-Mongolia-Russia Economic Corridor into five grades: micro, mild, moderate, strong, and severe erosion.
2. Research area
The China-Mongolia-Russia Economic Corridor is one of the six economic corridors under the Belt and Road Initiative (Dong et al. Citation2017), which is the northernmost economic corridor. It is characterized by high latitude, low annual temperature, low winter temperature, large difference between the lowest and hottest average monthly temperature, extensive permafrost area, and serious soil freeze-thaw erosion. These factors cause a fragile ecological environment and leads to unbalanced economic development, and various engineering construction difficulties (Dong et al. Citation2019). The spatial and temporal analysis in freeze-thaw erosion of this region will facilitate timely measures to decelerate the natural environment deterioration in ecologically fragile areas and their surroundings (Wang Citation2019). It will also reduce the engineering construction losses caused by freeze-thaw erosion, and provide a scientific basis for regional farmers’ agricultural production and future agricultural development.
The economic corridor mainly comprises plains and plateaus with largely flat terrain. The western portion extends deep inside the Eurasian continent and the eastern edge approaches the ocean; the climate gradually becomes arid moving from east to west across the region. The annual average temperature lies from −5 to 15 °C, and the annual average precipitation is in the range of 200–800 mm. Vegetation along the route is significantly affected by climatic characteristics and vegetation coverage. The land use types in the research area are shown in .
In the buffer zone of the China-Mongolia-Russia Economic Corridor, Mongolia and Russia are geographically close to each other. Furthermore, Mongolia mainly supports animal husbandry, and its economic structure is complementary to Russia. The unique resource structure has built a firm groundwork for China. China, Mongolia and Russia have created the China-Mongolia-Russia railroad route in order to promote economic development, as shown in .
3. Data source and preprocessing
According to , the experimental data sources in this article. We further processed the source data by the following steps. Firstly, we considered the temperature data collected from 228 meteorological stations as well as the annual temperature range at each station. Secondly, we used the Thornthwaite model to calculate the moisture index for each site from the perspective of temperature and precipitation data collected from weather stations in and around the study area. Next, the annual temperature range and moisture index were encrypted by the Crikin weighted method (Le and Zidek Citation2006) to obtain the region annual temperature range and moisture index images. Subsequently, we calculated the vegetation coverage from the MODIS NDVI data, and global soil data were used to derive the sand content in the research area with the sand soil value content. Then, we unified the geographic reference value of each factor based on the Albers coordinate system using the D_Krasovsky_1940 benchmark. All images were taken from the same study area. Finally, the factor grid data obtained from image preprocessing at the same spatial resolution were resampled to give an image spatial resolution of 1,000 m.
Table 1. Data sources.
3.1. Definition of the freeze-thaw erosion zone range
In general, when studying freeze-thaw erosion zones, it is necessary to first determine the extent of the freeze-thaw erosion zone. Moreover, the determination method refers to the results of previous studies and takes the lower boundary of the ice edge area as the boundary range of the freeze-thaw erosion area. Based on Qiu’s study in 2000 that the lower boundary of the freeze-thaw zone is 100–300 m lower than the lower boundary of the permafrost zone (Qiu et al. Citation2000). Therefore, an average value of 200 m was chosen as the difference between the two. The temperature (T), elevation(H), latitude(X), and longitude(Y) of the meteorological stations were combined to generate the regression equations between T and H, X and Y (Zhang and Liu Citation2005); The freeze-thaw erosion zone was subsequently determined using this regression equation along with the average yearly air temperature (Zhang and Liu Citation2005), and it has since been widely employed (Chen et al. Citation2020; Luo. et al. 2020; Guo et al. Citation2017).
Using the X, Y, and H obtained from the DEM data, the regression calculated the average annual temperature as 0 °C, minus 200 m to obtain the lower bound for freeze-thaw erosion. The calculation formula is as follows:
(1)
(1)
where H is the elevation (m) of the lower freeze-thaw erosion zone boundary, X is the longitude, and Y is the latitude (N).
EquationEquation (1)(1)
(1) was used to extract the basic freeze-thaw erosion zone range, as shown in .
3.2. Preprocessing the influence factors
3.2.1. Daily freeze-thaw cycle days (Daily FT-cycle days)
Freeze-thaw erosion occurs through two processes: freezing and thawing (Jing et al. Citation2003). Soil temperatures are cycled through week after week, its corrosion resistance has been compromised.
The FT-cycle revolves around daily soil temperatures with a maximum greater than 0 °C and a minimum less than 0 °C (Chen et al. Citation2020). The magnitude of the daily soil FT-cycle is equivalent to the difference between the maximum and minimum soil temperatures in the daily FT-cycle (Henry Citation2007). Transformations in soil temperature are observations that take a long time to complete. However, soil temperature has been found to be strongly correlated with air temperature (Shi et al. Citation2012), so we used air temperature instead of soil temperature to obtain the number of daily FT-cycle days, as shown in .
3.2.2. Annual temperature range
The primary driver is temperature (Luo et al. Citation2020), and along with this cycle, it has an impact on how quickly the soil freezes and thaws, which alters the thickness of the permafrost (Yang et al. Citation2022). The thickness of permafrost increases with decreasing temperature; on the other hand, it decreases. Global warming influences how frequently soil cycles of freezing and thawing occur (Henry Citation2008). FT dynamics and hydroclimate are strongly dependent, mainly through influencing the frequency of freeze-thaw cycles and the depth of the permafrost layer in various ways (Wang et al. Citation2021; Hatami and Nazemi Citation2022). Permafrost hinders soil infiltration and alters water and nutrient inputs (Iwata et al. Citation2010). Since temperature affects the depth and duration of freezing, this article selects the annual temperature range as one of the influencing factors.
Based on the temperature observation data value from 228 meteorological stations in and around the test area, the average annual difference in temperature was calculated for the 20 years from 2001 to 2020. A few meteorological stations needed to be converted, and Kriging interpolation was used to obtain an annual temperature range distribution, as shown in .
3.2.3. Elevation
In general, elevation influences ground temperature and contributes to the strength of freeze-thaw erosion.
From the use of the study area boundary cropping DEM data and mapping the elevation distribution in this area, it can be seen that the elevation of the study area varies significantly between 82m and 4203 m, as shown in .
3.2.4. Slope
The amount and location of erosion are intimately related to slope; typically, the steeper the slope, the more severe the erosion.
The slope factor was obtained through the DEM data, and was extracted using the slope method in ArcGIS. The regional slope distribution was drawn, as shown in .
3.2.5. Aspect
Due to solar radiation, different slopes are exposed to different levels of radiation, making the amount of evaporation from the soil very different. This can also lead to differences in the degree of freeze-thaw erosion.
The aspect factor was extracted from the DEM data. We used the Aspect method in ArcGIS to draw the regional aspect distribution, as shown in .
3.2.6. Vegetation coverage
Vegetation cover has a mitigating effect on the extent of freeze-thaw erosion. Because it serves to stabilize the internal properties of the soil (Li et al. Citation2011; Zhang et al. Citation2011), reduces the degree of variability in erosion caused by temperature changes (Chen et al. Citation2020), thereby reducing the probability of freeze-thaw erosion occurring.
The vegetation coverage factor was calculated by downloading the NDVI data for 20 years (2001–2020). We drew the vegetation coverage distribution of the area through the vegetation coverage EquationEq. (2)(2)
(2) (Lee et al. Citation2022), as shown in . The following is the vegetation coverage EquationEq. (2)
(2)
(2) :
(2)
(2)
where R stands for the red band's reflectance and NIR for the near infrared band.
3.2.7. Moisture index
The water content in the soil affects the stability of soil aggregates (Wang et al. Citation2010), so this article use moisture index to represent the factor of soil water content.
First, the data from 228 meteorological stations in the research and surrounding areas were collected. Based on the average monthly temperature and precipitation data in the observation data, the moisture index from 2001 to 2020 was calculated, and then the average moisture index over 20 years was obtained (EquationEqs. (3)(3)
(3) and Equation(4)
(4)
(4) ). In this article, the ratio of annual precipitation to annual potential evaporation value is defined as the soil wetness index of the study area, and the temperature method is chosen to calculate the potential soil evaporation value. The enhanced Thornthwaite technique outperforms the initial formulation (Antonio and William Citation2003). The following is the calculating formula:
(3)
(3)
(4)
(4)
In EquationEq. (3)(3)
(3) :
with a unit of mm/d, is the
value determined by the enhanced TW method; C is used to convert the calculation period from months to days,
(the model assumes 30 days per month and 12 hours of sunshine every day, so N = 360), I is the heat index,
is the effective temperature in degrees Celsius;
is the monthly average temperature;
is the maximum temperature in degrees Celsius;
is the minimum temperature in degrees Celsius.
The moisture index was calculated, and the moisture index distribution of the area was drawn, as shown in .
3.2.8. Soil content
First off, sands in the research area are substantially larger than silts, despite the fact that both have an impact (Bartczak et al. Citation2021). Sands also have larger particles than silts, and larger soil particles have less erosion resistance and are more susceptible to erosion during freezing and thawing cycles. Therefore, this article only chose sands as one of the characteristics to be analyzed.
According to the global soil data, the sand content image in the research area was derived by value, and the sand content distribution of the area was drawn, as shown in .
4. Calculation of freeze-thaw erosion based on analytic hierarchy process
Analytic hierarchy process, AHP for short, was introduced to China in 1982 for widespread use (Yu et al. Citation2023). A simple summary of the characteristics of AHP is that the complex relationship between factors is made hierarchical and organized. Typically, when research is analysed using AHP, a combination of expert opinion and the user’s own objective evaluation is used. Mathematical methods are also used to assign weights to and rank the influences at each level.
It was discovered, with reference to the earlier study by Lu et al. (Citation2021), that the conclusions reached using AHP strongly agreed with those of the water resources department’s bulletins on soil and water conservation. In order to acquire the reference results, this article referred to the study of Lu et al. (Citation2021) and chose seven factors but daily FT-cycle days for study. In previous studies, the AHP has been recognized by many scholars; therefore, because the research area is extensive and there are no feasible field investigations, the research results of the AHP are used as the comparative test.
AHP (Bhushan and Kanwal Citation2004; Saaty Citation2008; Fan et al. Citation2018) is used to obtain the evaluation factor judgment matrix and determine each factor weight (.). The results of the hierarchical analysis method showed that the maximum characteristic root was 7.13 and the RI (Hierarchical Total Ordering Average Random Consistency Index) value was 1.341, so CR (Hierarchical Total Ordering Random Consistency Proportion) value is therefore 0.016 < 0.1, which passes the one-time test (Xu Citation2008), as shown in .
Table 2. Judgment matrix.
Table 3. Evaluation factor weights and computational process.
The factor weights obtained using the AHP are listed in . The erosion intensity calculated using the composite index method was located between 0.0701 and 0.7581, and the erosion intensity classification of the study area was plotted based on the calculation results (as shown in ), as well as the area occupied by different erosion intensities in the study area is calculated (as shown in ).
Figure 5. Classification map of freeze-thaw erosion based on the AHP and comprehensive index method.

Table 4. Evaluation results of freeze-thaw erosion intensity (AHP).
5. Calculation of freeze-thaw erosion based on similar information value model
The primary goal of the spatio-temporal analysis of freeze-thaw erosion based on the similar information value model is to contrast the study area’s estimated and actual levels of freeze-thaw erosion. Because the information value model cannot reflect the degree of influence of the same factor, in this article, the information value model is improved. It calculates the amount of information obtained for the factors, uses the improved values as weights. The weights are weighted with the normalised values of the factors to obtain a map of freeze-thaw erosion results for the study area.
5.1. Original information value model
The information value model method is a common method in disaster prediction to establish a disaster susceptibility evaluation model based on the amount of information provided by disaster factors. A reasonable evaluation model is combined with the information value model method and applied to the evaluation accuracy to some extent. The information value model method is infrequently for spatio-temporal analysis of freeze-thaw erosion, so it is necessary to further explore the reasonable application of this method.
Shannon proposed the information value model, which can accurately reflect the probability of landslide occurrence under different and mutually independent factors, as well as the related conceptual expressions (Zhang et al. Citation2022). This can be calculated using EquationEq. (5)(5)
(5) :
(5)
(5)
The concept of single-factor information quantity is thus introduced, and using to denote the information quantity of the factor alone in the scope of disaster y, we have EquationEq. (6)(6)
(6) :
(6)
(6)
where
denotes a hazard event;
denotes the different contributing factors;
denotes the probability of a hazard occurring under factor
denotes the probability of a landslide occurring in the area used for normalisation;
indicates the amount of information that factor xi alone acts on hazard y
The expression has since been further refined as the information value model has been widely used in practice(Li et al. Citation2017; Lu et al. Citation2022; Mao et al. Citation2022) as in EquationEq. (7)(7)
(7) :
(7)
(7)
where
is the total number of evaluation units in the study area;
is the total number of hazards occurring in the study area;
is the number of units containing evaluation factor
in the study area; and
is the number of hazards distributed within a specific category within factor
5.2. Similar information value model
The chance of a disaster occurring under a specific circumstance and the probability of a disaster occurring in the study region are how the concept of information value model is articulated. Since varying erosion degrees could result in a negative information value, linear normalization is used to determine the weight under various circumstances. From this, we deduced the similar information value model for freeze-thaw erosion, abbreviated as SIVM.
5.2.1. Formula for deriving the SIVM of a single factor
The one-factor SIVM model is calculated from the probability of freeze-thaw erosion occurring under a given factor condition and the probability of freeze-thaw erosion occurring under the whole study area, as in EquationEq. (8)(8)
(8) :
(8)
(8)
where
represents the SIVM under different erosion levels under factor
alone;
is the different freeze-thaw erosion levels under factor
(j = 1, 2, 3, 4, 5);
is the area of the study area;
is the area of freeze-thaw erosion within the study area,
is the area under erosion level
in the study area under factor
is the area under erosion level
under factor
The SIVM for the different degrees of freeze-thaw erosion under factor were subsequently linearly normalised and summed to give the SIVM
for the factor.
5.2.2. Calculation of weights
Usually the larger the value of information under a factor means the greater the likelihood of a disaster occurring under the conditions of that factor. Similarly, the larger the SIVM means the greater the likelihood of freeze-thaw erosion occurring under the conditions of that factor, from which the value of the size of the weight accounted for by that factor can be deduced (Wang, Citation2022), as in EquationEq. (9)(9)
(9) :
(9)
(9)
where
denotes the size of the weights under factor
denotes the corresponding similar information value of different factors.
We obtained the weights of each factor based on the above formula, as shown in .
5.3. Experimental results
The freeze-thaw erosion intensity was calculated to range from 0.0698 to 0.7572 and graded by the natural interruption point grading method into micro erosion, mild erosion, moderate erosion, strong erosion and severe erosion. The erosion intensity classification map of the study area was drawn (as shown in ) and the area under each erosion intensity were calculated based on the five levels of classification. We found the results were found to be within acceptable limits as shown in . Additionally, we selected multiple and randomized groups of points within the region, and the results from the SIVM and the AHP showed a high degree of agreement.
6. Result verification and analysis
6.1. Result verification
We randomly and repeatedly selected 38 location points for visual interpretation and divided them into six groups for validation. The remote sensing images were mostly in the winter and spring. By importing point vector data into the remote sensing image diagram, the freeze-thaw erosion strength was determined according to . By comparing the results with those from the information model method, the strength of the same position and some information model method results were weaker than those obtained by the visual interpretation method. The results of the study show strong plausibility and reliability, and we only show example plots for some of the sampling points (as shown in , and ), where the ID of corresponds to the ID of .
Table 5. Factor weights based on SIVM process.
Table 6. Image interpretation of freeze-thaw erosion intensity.
Table 7. Comparison of freeze-thaw erosion intensity.
6.2. Interpretation of results
Using the evaluation system of freeze-thaw erosion intensity and the freeze-thaw erosion sensitivity factor grading standard reference (Li et al. Citation2015; Guo et al. Citation2019; Wu and Liu Citation2010), some factor assignments were regraded due to the different study areas. As shown in . and and , the relationship between the influence of each factor and the allocation of freeze-thaw erosion zones was further investigated and analyzed.
Table 8. Grading criteria for freeze-thaw erosion sensitivity factors.
As shown in , among the seven influencing factors analyzed in this article, the annual difference in temperature, elevation, slope, vegetation cover index and sand content have a more obvious effect, while the effect of aspect and soil wetness is not significant.
The annual difference in temperature has a clear tendency to influence freeze-thaw erosion, which is reflected in the fact that the area increases and then decreases with increasing annual difference in temperature, and reaches a peak in the 28–30° interval. Meanwhile, the area of micro and mild erosion remains similar to the overall trend. But on the contrary, the area of moderate, strong and severe erosion shows a tendency to decrease and then increase with increasing annual difference in temperature, and reaches a boundary in the 26 to 28° interval to reach the demarcation. Elevation and slope have similar trends, both with the increase of factor value, the percentage of eroded area decreases, but during the period there is a small increase with the increase of slope. Furthermore, the two factors in the process of increasing the value of the erosion intensity level continues to increase, as shown in the micro, mild and moderate with the subsequent increase and decrease, and strong and severe erosion increases. In addition, the opposite trend to the effect of elevation and slope is the vegetation cover index, which shows that the eroded area increases with the increase of it and has a significant inhibitory effect on the degree of erosion. When analyzing the sand content, it is obvious that the eroded area is mainly concentrated in the interval range between sand content below 50% and above 70%, but the micro mild erosion decreases when the sand content is above 50%.
The effect of slope orientation on freeze-thaw erosion is not obvious and has a relatively even distribution among different slope orientation zones. In the analysis of soil wetness index, it was found that erosion was mainly concentrated in the range of soil wetness index from 20 to 60. Soil wetness on the one hand promotes the growth of vegetation and thus expands the vegetation cover, which inhibits the increase of erosion, and on the other hand increases the water content of the rock, which promotes the occurrence of freeze-thaw erosion. Therefore, this balance makes the role of soil wetness index on the degree of erosion insignificant, but also makes the degree of erosion unevenly distributed because the degree of promotion and inhibition are not exactly the same.
From the above analysis, it can be shown that the increase of erosion can be suppressed in the following ways.
Control the mass clearing of slopes, consolidate the soil and protect the slopes, and pay attention to the protection of already cleared slopes.
Use vegetation to inhibit the aggravation of erosion, and increase the area of greening and reduce the occurrence of indiscriminate logging.
Wind protection and sand fixation can also effectively inhibit the aggravation of erosion.
7. Conclusions
SIVM is based on mathematical probabilistic problems and informativeness models as a way to derive, which is an attempt to expand the methodology of freeze-thaw erosion research. We start from the perspective of combining economics and geography, which makes our method more objective to calculate the degree of freeze-thaw erosion strength.
This article mainly uses the AHP with the comprehensive index evaluation method, and the weighted SIVM to conduct spatio-temporal analysis of freeze-thaw erosion in the China-Mongolia-Russian economic corridor.
The spatial distribution and intensity distribution of the weighted similar information value model method are strongly consistent with the comprehensive index evaluation method. The intensity of freeze-thaw erosion in the study area was largest for mild and moderate erosion, which is followed by micro and strong erosion, while severe erosion is the least. The spatial distribution of freeze-thaw erosion in the study area varies significantly, showing a more severe intensity of freeze-thaw erosion from the northeast region connected to Lake Baikal to the southwest region, and gradually weakening intensity from the connection line to the southeast and northwest. All seven factors selected for the experiment significantly affect the intensity of freeze-thaw erosion, and relevant measures are proposed for different factors to help mitigate the hazards caused by freeze-thaw erosion. The results for both methods are similar, but there are also certain differences. However, the differences are small, and the weighted information value model method is scientific and reasonable.
At present, we propose a new method for studying the factors affecting freeze-thaw erosion. The applicability of this method has been verified in the China-Mongolia-Russia Economic Corridor region. In the future, we will conduct experiments on SIVM in other research areas to verify its wide application. Also, we will further optimise SIVM based on the results from different experimental areas.
Data availability statement
The data that support the findings of this study are available from [NOAA National Environmental Information Center, Geospatial Data Cloud, NASA Data Center, World Soil Database]. Restrictions apply to the availability of these data, which were used under license for this study. Data are available [at https://www.ncei.noaa.gov, http://www.gscloud.cn/, https://ladsweb.modaps.eosdis.nasa.gov/, https://iiasa.ac.at/] with the permission of [NOAA National Environmental Information Center, Geospatial Data Cloud, NASA Data Center, World Soil Database].
Disclosure statement
No potential conflict of interest was reported by the authors.
Additional information
Funding
References
- Ali BD, Masoud S, Najmeh SN, Mohsen B, Masomeh Q, Ramin P, Saham M. 2023. Assessment of rural vulnerability to sand and dust storms in Iran. Atmosphere. 14(2):281.
- Antonio RP, William OP. 2003. Adaptation of the Thornthwaite scheme for estimating daily reference evapotranspiration. Agric Water Manage. 66(3):251–257.
- Bartczak A, Kaczmarek H, Badocha M, Krzemiński M, Tyszkowski S. 2021. Measured and predicted freeze-thaw days frequencies in climate change conditions in central Poland. PeerJ. 9:e12153. doi: 10.7717/peerj.12153.
- Bhushan N, Kanwal R. 2004. Strategic decision making: applying the analytic hierarchy process. London: Springer-Verlag.
- Brandon VH, Richard Mp Jonathan SP, William LQ, John WP. 2020. Seasonal ground ice impacts on spring ecohydrological conditions in a western boreal plains peatland. Hydrol Processes. 34(03):765–779.
- Chen LX, Chen ZS, Jia GD, Zhou J, Zhao JC, Zhang ZQ. 2020. Influences of forest cover on soil freeze-thaw dynamics and greenhouse gas emissions through the regulation of snow regimes: a comparison study of the farmland and forest plantation. Sci Total Environ. 726:138403. doi: 10.1016/j.scitotenv.2020.138403.
- Chen TD, Jiao JY, Wang HL, Zhao CJ, Lin H. 2020. Progress in research on soil erosion in Qinghai-Tibet Plateau. Acta Pedologica Sinica. 57(03):547–564.
- Dong SC, Li ZH, Li Y, Shi GY, Li FJ, Li F. 2017. The first national science and technology support project of the six “One Belt and One Road” economic corridor launch of the multi-disciplinary joint survey of: China-Mongolia-Russia International Economic Corridor. Res Sci. 39(05):987–989.
- Dong SC, Yang Y, Li FJ, Cheng H, Li J, Bilgaev A, Li ZH, Li Y. 2019. Influencing mechanism and policy suggestions of ChinaMongolia-Russia high-speed railway construction. Geographic J. 74(05):297–311.
- Eigenbrod KD. 1996. Effects of cyclic freezing and thawing on value changes and permeabilities of soft fine-gained soils. Can Geotech J. 33(4):529–537. doi: 10.1139/t96-079-301.
- Fan HD, Lu YF, Hu YL, Fang J, Lv CZ, Xu CQ, Feng XY, Liu YR. 2022. A landslide susceptibility evaluation of highway disasters based on the frequency ratio coupling model. Sustainability. 14(13):7740–7740. doi: 10.3390/su14137740.
- Frauenfeld OW, Zhang T, Barry RG, Gilichinsky D. 2004. Interdecadal changes in seasonal freeze and thaw depths in Russia. J Geophys Res. 109(d5):d05101. doi: 10.1029/2003JD004245.
- Fan ZY, Gou XF, Qin MY, Fan Q, Yu J, Zhao JJ. 2018. Information and logistic regression models based coupling analysis for susceptibility of geological hazards. J Eng Geol. 26(02):340–347.
- Guo B, Chen ST, Han BM, Yang X, Fan YW, He TL. 2019. Quantitative assessment of ecological vulnerability of the silk road economic belt, China based on the partition-integration concept. Yangtze River Basin Res Environ. 28(11):2601–2611.
- Guo B, Jiang L. 2017. Evaluation on freeze-thawing erosion in Qinghai-Tibet Plateau Based on Multi-Source Data. Bulletin Water Soil Conserv. 37(04):12–19.
- Guo B, Luo W, Wang DL, Jiang L. 2017. Spatial and temporal change patterns of freeze-thaw erosion in the three-river source region under the stress of climate warming. J Mt Sci. 14(6):1086–1099. doi: 10.1007/s11629-016-4348-x.
- Harden CP, Mathews LE. 2002. Hillslope Runoff and soil erosion following reforestation in the Copper Basin, Tennessee, USA. Aust Geog Studies. 40(2):130–142. doi: 10.1111/1467-8470.00169.
- Hatami S, Nazemi A. 2022. Compound changes in temperature and snow depth lead to asymmetric and nonlinear responses in landscape freeze–thaw. Sci Rep. 12(1):2196. doi: 10.1038/s41598-022-06320-6.
- Henry HAL. 2007. Soil freeze-thaw cycle experiments: trends, methodological weaknesses and suggested improvements. Soil Biol Biochem. 39(5):977–986. doi: 10.1016/j.soilbio.2006.11.017.
- Henry HAL. 2008. Climate change and soil freezing dynamics: historical trends and projected changes. Clim Change. 87(3–4):421–434. doi: 10.1007/s10584-007-9322-8.
- Iwata Y, Hayashi M, Suzuki S, Hirota T, Hasegawa S. 2010. Effects of snow cover on soil freezing, water movement, and snowmelt infiltration: a paired plot experiment. Water Resour Res. 46(9):W09504. doi: 10.1029/2009WR008070.
- Jing GC, Ren XP, Liu BY, Qi GG. 2003. Freeze-thawing erosion in Heilongjiang Province. Soil Water Conserv Sci China. 1(03):99–101.
- Juselius T, Ravolainen V, Zhang H, Piilo S, Müller M, Gallego-Sala A, Väliranta M. 2022. Newly initiated carbon stock, organic soil accumulation patterns and main driving factors in the High Arctic Svalbard. Norway. Sci Rep. 12(1):4679. doi: 10.1038/s41598-022-08652-9.
- Kuchera SF. 2022. Comprehensive evaluation for chronic pain. Primary Care: Clinics in Office Practice. 49(3):375–385.
- Lee G, Kim G, Min G, Kim M, Jung S, Hwang J, Cho S. 2022. Vegetation Classification In Urban Areas By Combining UAV-based NDVI and thermal infrared image. Applied Sci. 13(1):515. doi: 10.3390/app13010515.
- Liu B, Fan HM, Jiang YZ, Ma RM. 2023. Linking pore structure characteristics to soil strength and their relationships with detachment rate of disturbed Mollisol by concentrated flow under freeze-thaw effects. J Hydrol. 617(PB):129052. doi: 10.1016/j.jhydrol.2022.129052.
- Li CL, Ma JH, Tang ZG, Zhou W. 2011. GIS-based evaluationon intensity of freeze-thaw erosion in headwater region of the three-river-source area. China Soil Water Conserv. 349(04):41–43 + 69.
- Li D, Wei X, Li XG, Li YJ. 2015. Sensitivity evaluation of freeze-thawing erosion in Gansu Province Based on RS and GIS. Soil Soil Conserv Res. 22(02):1–6.
- Luo D, Jin H, Lü L, Wu Q. 2014. Spatiotemporal characteristics of freezing and thawing of the active layer in the source areas of the Yellow River (SAYR). Chin Sci Bull. 59(24):3034–3045. doi: 10.1007/s11434-014-0189-6.
- Luo LG, Pei XJ, Huang RQ, Pei Z. 2020. Spatial-temporal differentiation characteristics and rate of freeze-thaw erosion of rock slopes in periglacial area. J Eng Geol. 28(06):1319–1328.
- Li HW, Xu EQ, Zhang HQ. 2018. Soil resion regionalization in ILi river valley. Agri Res Div China. 39(04):116–124.
- Lu MF, Zhang H, Xie BH. 2022. Risk Assessment of geological disaster by introducing information quantity model AHP: a case study of Songtao County, Guizhou Province. Guizhou Geology. 39(04):392–399.
- Le ND, Zidek JV. 2006. Statistical analysis of environmental space-time processes. New York: Springer Series in Statistics.
- Lu YF, Liu C, Ge Y, Hu YL, Wen Q, Fu ZL, Wang SB, Liu Y. 2021. Spatiotemporal characteristics of freeze-thawing erosion in the source regions of the Chin-Sha, Ya-Lung and Lantsang rivers on the basis of GIS. Remote Sens. 13(2):309. doi: 10.3390/rs13020309.
- Lu YF, Zhang T, Liu Y, You YM, Feng C, Kong WH. 2019. Evaluation and spatial distribution characteristics of freeze-thaw erosion intensity in the Yalu Tsangpo River basin on the basis of geographic information system. Geomatics, Natural Hazards & Risk. 10(1):1047–1069. doi: 10.1080/19475705.2018.1564705.
- Lv YY, Liu ZH, Ye P, Zhang S, Zhang Y. 2016. Evaluation on ecological sensitivity of Chuanxi plateau based on GIS. Soil Soil Conserv Res. 37(04):272–277.
- Li ZG, Liu SZ, Zhang JG, Zhang LX. 2017. Survey method of freeze-thaw erosion in China. Soil Water Conserv Sci China. 10(01):1–5.
- Li ZT, Wang T, Zhou Y, Liu JM, Xin P. 2019. Landslide susceptibility assessment based on information value model, logistic regression model and their integrated model: a case in Shatang River Basin, Qinghai Province. GEOSCIENCE. 33(01):235–245.
- Manzi G, Vizza CD, Badagliacca R. 2022. Comment on Topyła-Putowska et al. echocardiography in pulmonary arterial hypertension: comprehensive evaluation and technical considerations. J Clin Med. 11(12):3337. doi: 10.3390/jcm11123337.
- Mao QX, Hu YP, Liu WL, Weng MZ, Duan XF. 2022. Vulnerability evaluation of slope geological disasters in linear corridor area in Moudao-Jiannan, Lichuan City based on information model. Resour Environ Eng. 36(02):175–180. +217.
- Oliveira M, Miguel M, van Langen SK, Ncube A, Zucaro A, Fiorentino G, Passaro R, Santagata R, Coleman N, Lowe BH, et al. 2021. Circular economy and the transition to a sustainable society: integrated assessment methods for a new paradigm. CircEconSust. 1(1):99–113. doi: 10.1007/s43615-021-00019-y.
- Qiu GQ, Zhou YW, Cheng GD, Guo DX. 2000. Geocryology in China. Beijing: Science Press.
- Sun BY, Li ZB, Xiao JB, Zhang LT, Ma B, Li JM, Cheng DB. 2019. Research progress on the effects of freeze-thaw on soil physical and chemical properties and wind and water erosion. J Appl Ecol. 30(01):337–347.
- Saaty TL. 2008. Relative measurement and its generalization in decision making: why pairwise comparisons are central in mathematics for the measurement of intangible factors the analytic hierarchy/network process. Review of the Royal Academy of Exact, Physical and Natural Sciences, Series A: Mathematics (RACSAM).
- Shi Z, Tao HP, Liu SZ, Liu BT, Guo B. 2012. Research of freeze-thaw erosion in the three-river-source area based on GIS. Transactions Chinese Society Agri Eng (Transactions of the CSAE). 28(19):214–221 + 300.
- Taskin O, Ferhan F. 2003. Effect of freezing and thawing processes on soil aggregate stability. Catena. 52(01):1–8.
- Vecellio DJ, Frauenfeld OW. 2022. Surface and sub-surface drivers of autumn temperature increase over eurasian permafrost. Clim Change. 172(1–2):13. doi: 10.1007/s10584-022-03366-3.
- Wang F, Xiz H, Li LH, Zhang KQ. 2010. How freezing and thawing processes affect black-soil aggregate stability in northeastern China. Scie Cold and Arid Regions. 2(01):67–72.
- Wang FF. 2022. Application of ArcGIS information value modeling method in regional geological hazard risk evaluation. Inner Mongolia Coal Economy. 354(13):178–180.
- Wang LY, Xiao FR, Jiang L, Oyang ZY. 2017. Assessment and analysis of the freeze-thaw erosion sensitivity on the Tibetan Plateau. J Glaciol Geocryol. 39(01):61–69.
- Wang P, Zhao HY, Yan P, Zhu HX, Zhai M, Li XF. 2021. Characteristics of changes of meteorological factors during freezing and thawing period in Heilongjiang Province. J Glaciol Geocryol. 43(06):1764–1772.
- Wang QF, Qi JY, Qiu H, Li J, Cole J, Waldhoff S, Zhang XS. 2021. Pronounced increases in future soil erosion and sediment deposition as influenced by freeze-thaw cycles in the upper mississippi river basin. Environ Sci Technol. 55(14):9905–9915. doi: 10.1021/acs.est.1c02692.
- Wu WZ, Liu FG. 2010. Dynamic analysis and distribution characteristics of freeze-thaw erosion in the three-rivers’ headstream region. J Qinghai Normal Univ (Natural Science Edition). 26(01):57–61.
- Wang YH, Yu XS, Su YL. 2021. Research on comprehensive evaluation of liaoning realestate industry development based on entropy method. J Shenyang Jianzhu Univ (Social Science). 23(4):389–394.
- Wang YJ. 2022. Constructing the comprehensive benefit evaluation model of micro-energy network in residential building. Int J Front Sociol. 4(07):118–125.
- Wang YY. 2019. The environmental issues and cooperation of green development in the building the China-Mongolia-Russia economic corridor. Northeast Asia J. 44(03):69–79 + 148.
- Xu W, Li TF, Hu RL, Wen MS, Liu B. 2011. GIS-based landslide susceptibility assessment at small watershed in Jiasa Town, Ailao Mountain Area. Hydrogeol Eng Geol. 38(05):110–114 + 119.
- Xu XM. 2008. The application of the hierarchical analysis method. Statistics Decision. 253(1):156–158.
- Yang PP, Wang NQ, Guo YJ, Ma X. 2020. Assessment of landslide susceptibility in lintong district using weighted information value model. J Yangtze River Academy Sci. 37(09):50–56.
- Yang ZZ, Ni WK, Li L, Niu FJ. 2022. Grading evaluation of multi factor soil freeze-thaw erosion intensity based on GIS: A case study of the upper reache of the Yangtze River. J Water Resour Archi Eng. 20(06):89–95.
- Yu XH, Sun JH, Xu HL. 2023. Application progress of analytic hierarchy process in marine ecological assessment. J Tianjin Agri Univ. 30(01):80–83.
- Zhu LF, Wu XC, Yin KL, Liu XG. 2004. Risk zonation of landslide in China based on information content model. J Earth Sci Environ. 26(03):52–56.
- Zhang J, Sha ZJ, Song CB, Wang CB, Wang JH, Feng YS. 2011. Freeze-thaw erosion in the Buha River basin. J Earth Environ. 2(06):680–684.
- Zhang JG, Liu SZ. 2005. A new way for definting the freezing-thaw erosion area in Tibet. Geography Geo-Info Sci. 21(02):32–34 + 47.
- Zhang YB, Zeng L, Fu HL, Zhang ZY. 2022. Evaluation of geological hazard susceptibility IinShiQian county based on information model method. J Geol Hazards Environ Preserv. 33(1):44–49.