ABSTRACT
Fungal communities (mycobiome) have an important role in sustaining the resilience of complex microbial communities and maintenance of homeostasis. The mycobiome remains relatively unexplored compared to the bacteriome despite increasing evidence highlighting their contribution to host-microbiome interactions in health and disease. Despite being a small proportion of the total species, fungi constitute a large proportion of the biomass within the human microbiome and thus serve as a potential target for metabolic reprogramming in pathogenesis and disease mechanism. Metabolites produced by fungi shape host niches, induce immune tolerance and changes in their levels prelude changes associated with metabolic diseases and cancer. Given the complexity of microbial interactions, studying the metabolic interplay of the mycobiome with both host and microbiome is a demanding but crucial task. However, genome-scale modelling and synthetic biology can provide an integrative platform that allows elucidation of the multifaceted interactions between mycobiome, microbiome and host. The inferences gained from understanding mycobiome interplay with other organisms can delineate the key role of the mycobiome in pathophysiology and reveal its role in human disease.
Introduction
Until recently, fungal infections, unlike bacterial and viral infections, had been largely neglected despite being a significant cause of global morbidity and mortality.Citation1,Citation2 The importance of fungi in the host has been largely overlooked as the number of fungal genes in the gut is relatively low compared to bacterial genes, as reported by the Human Microbiome Project (HMP).Citation3 With the advent of high throughput sequencing technologies, our knowledge of the bacterial communities of the microbiome has expanded exponentially, along with our understanding of their potential roles in disease pathology. In contrast, the mycobiome (fungal community) study remains in its formative years.Citation4–6 To date, there are substantially fewer studies focused on fungi within the microbiome due to difficulties in nucleic acid extraction, rudimentary fungal genome annotations and limited bioinformatic tools to analyse these data or established standards. Early studies using culture-dependent studies suggested that only 70% of the population harboured a fungal population.Citation7 A total of 267 fungal taxa have been identified in the human gut mycobiome by several studies. However, the validity of some of these fungal species (e.g. Penicillium) is unclear as they cannot be grown under human gut conditions in the laboratory.Citation8 The long-term residence of fungi in the gut microbiome is thus still the subject of debate as the longevity of fungal species within a mycobiome is undetermined.Citation8 Further, fungal genes within the microbial metagenome have been estimated to only make up 0.1% of the total microbial genes.Citation9 However, the importance of fungi to the host cannot be determined by measures of the overall relative abundance of their genetic material within the microbiome. Production of metabolites and small molecules by fungi potentially has a more significant impact in the microbiota and host compared to bacterial production, owing to the greater fungal biomass and associated higher occupation of surface area, however, this remains largely unproven.Citation9–11 A summary table of mycobiome studies associated with dysbiosis highlights the increasing relevance of fungi ().
Table 1. The effect of fungal species in human health. The table indicates the fungal species causing diseases with references.
The variation in species seen within the mycobiome is attributable to dietary changes, skin contamination, drug therapy, geographical location and oral hygiene.Citation8,Citation12,Citation13 There are, however, some common features to the different mycobiome communities. Candida and Saccharomyces are the most common fungal genera in the gut, with their presence detected from infancy, indicating they are likely to be permanent colonisers.Citation3,Citation4,Citation8,Citation12,Citation14–17 Aspergillus and Penicillium genera are less common, indicating the transient nature of these genera, whilst reports of Mucor, Cladosporium and Cyberlindera presence are inconsistent. The disparate nature of fungal community structure is due to culture-dependent and -independent approaches lacking gold standards.Citation18 For example, varying extraction methods and primer selection can skew mycobiome analyses. In essence, there is a lack of reference gene catalogues and bioinformatic tools for consistent taxonomic profiling and reconstitute fungal metagenome species.
Although increasingly being seen as important, investigations of the impact of the mycobiota on both host and microbiome are in their infancy. In particular, our understanding of host-fungal metabolic interactions is limited in both health and disease. Although host-microbiome metabolic interactions have been studied, further development is needed in understanding the impact of fungal biochemical pathways and their downstream metabolites in these interactions.Citation19 In this review, we explore the role of fungal metabolism in human disease, and this will help us understand the extent of how mycobiome interacts with its environment. This review will go onto explore the impact of secondary metabolites and how we can use systems-level approaches to explore this deeper level of functioning of the mycobiome for therapeutic use.
Mycobiome and importance in health and disease
In the last decade, fungal infection research has exploded, focussing on the host immune response towards these microbes, including adaptive and innate immunity.Citation20,Citation21 Even though fungal disease leads to inflammation, the mechanisms at mucosal surfaces are not well elucidated.Citation2 However, the host immune response and subsequent imbalance of homeostatic microbial communities can lead to dysbiosis. Dysbiosis is the unfavourable changes/imbalance in the community of organisms within the microbiota, leading to the progression of the disease. This term is highly used in microbiome research and infers misunderstanding as the biological context is undetermined as healthy microbiota remains unknown and not specific to the presence of a particular genus or community distribution.Citation22 Detailed information of data source was tabulated for in vivo and in vitro mycobiome studies ().
Table 2. Mycobiome tabulated data types. The table indicates references with fungal data types for discerning fungal genera.
Fungal by-products have diverse uses, including agricultural supplements, food preservation, environmental farming, biotechnology and medicines.Citation10,Citation23 Routine consumption of fungal derivatives has inevitably resulted in changes to our ecosystem and adaptation of the human mycobiome. For example, fungal species have been used as probiotics, with Saccharomyces boulardii currently being used as a probiotic for the treatment of gastrointestinal disorders.Citation24 Meanwhile, S. cerevisiae isolated from food sources inhibits the adhesion, filamentation and biofilm formation of multiple Candida species.Citation25 Additionally, exposure to environmental factors including age, gender, diet, host genetics, therapeutic intervention and host lifestyle affect individual mycobiome composition.Citation5,Citation8,Citation14,Citation26 Diets with high carbohydrates can drive the increased gut presence of Candida species.Citation14,Citation27,Citation28 Likewise, obesity is associated with an increased abundance of Ascomycota and a parallel decrease in gut mycobiome richness.Citation29
Fungal dysbiosis potentially affects a significant portion of the population, being implicated in irritable bowel syndrome (IBS) and Crohn’s disease (CD) and a direct contributing factor in IBDCitation30 (see ). For example, greater fungal diversity occurs in CD patients compared to healthy controls,Citation31,Citation32 whilst C. albicans overgrowth in the gastrointestinal (GI) tract is a contributing factor to IBD onset.Citation31–36 Further, a shift in Ascomycota and Basidiomycota proportions potentially drives inflammatory processes in IBD.Citation31,Citation32,Citation37 The distal effect of mycobiome dysbiosis is chronic immune-mediated inflammation and appears to be related to central nervous system (CNS) infection associated with neurological diseases.Citation38–40 Fungal products have been found in Alzheimer patients,Citation41 whilst another study confirmed the presence of 14 fungal species, including Malassezia, Candida, Alternaria and Botrytis,Citation42 in different brain regions of Alzheimer’s patients.Citation43 Similarly, fungi have been associated as triggers of autoimmunity and multiple sclerosis,Citation44 whilst the presence of C. albicans and S. cerevisiae antibodies in schizophrenia patients has been linked to the gastrointestinal disorder, supporting the hypothesis of a gut-brain axis.Citation5,Citation38,Citation39,Citation45
Mycobiome dysbiosis is a predisposing factor in other diseases as well.Citation37,Citation40 For example, the mycobiome is associated with cancer development and progression,Citation46 where Malassezia spp. are increased in pancreatic ductal adenocarcinoma (PDA) in an in vivo (murine) study.Citation46 In contrast, Candida tropicalis, another commensal fungus, is associated with slower or lack of tumour development.Citation46 This study, among others, indicates that mycobiome composition is paramount to host health and disease status. However, specific fungal species do not exclusively drive these interactions. In order to survive, fungi adapt to their local environment; this includes adjusting to acidic environments through morphology switching and metabolic plasticity. Biofilms are a classic example of fungi changing their growth pattern in response to host responses and environmental cues. Fungi-microbe interactions play a key role in adaptation. Fungi cooperate with bacteria to increase fungal biofilm potential, resulting in significant increases in the severity of inflammatory diseases – most notably in IBD.Citation37 Alternatively, Pichia spp. interaction with Candida results in the reduction of biofilm due to competition in the environment.Citation47 Thus, mycobiome composition plays a significant role in the maintenance of the homeostatic relationship within the microbiota. Determining whether fungal-bacterial-host relationships drive compositional changes and whether controlling each element in these networks could potentially prevent disease is a fundamental goal for future microbiome research.
Mycobiome and it’s environment
The interplay between the mycobiome and host immune responses involves physical interactions at the cell surface, metabolic reprogramming, regulatory pathways, and secretion of small molecules. Exposure to host responses and environmental stressors provides a platform for metabolic plasticity to manipulate host interactions and promote invasionCitation48 or modulate immunological responses.Citation49 These host immune responses to fungal infection have been reviewed extensively elsewhere.Citation20,Citation50–54 To creates symbiotic relationship with microbiota for host immune-tolerance, there is a positive exchange of compounds and nutrients. With evidence of fungi producing vitamins and fatty acids,Citation55–57 there is an opportunity and potential for these exchanges with the mycobiome that remains to be investigated.
Microbial metabolism, including fungal metabolism, has been associated with driving inflammation, various diseases and even cancer progression.Citation58–60 For instance, short-chain fatty acids (SCFA), tryptophan and branched-chain amino acids have all been identified as playing a role in immune and homeostatic responses.Citation61 Short-chain fatty acids (SCFAs) available within the host are necessary for regulating some fungal growth as well as modulating immune responses. Fungi are capable of producing vitamins, notably including vitamin D and B6, that can impact the immune system.Citation62–66 The different fungal moieties, such as cell wall polysaccharides and melanin,Citation67 drive metabolic reprogramming of innate immune cells to confer protective inflammation and activate antimicrobial defences.Citation68–70 Equally, contact with host cells drives shifts in fungal metabolism.Citation52 For instance, host recognition of ß-glucan from fungal cell walls reprogrammes macrophage glucose metabolism towards aerobic glycolysis, promoting more rapid ATP creation.Citation67 Importantly, however, C. albicans undergoes a similar metabolic reprogramming, meaning that local glucose is rapidly depleted, leading to the premature death of macrophages.Citation52 As C. albicans has a significant degree of metabolic plasticity, it can then revert to using alternative carbon sources to survive. Melanin produced by Aspergillus fumigatus is recognised by macrophages via MelLec (a C-type melanin-sensing receptor) via calcium sequestering in phagosomes, leading to activation of antifungal defences within the host.Citation71 A. fumigatus responds further by reducing melanin for fungal persistence, leading to conidia germination and increased glycolysis allows metabolic repurposing of macrophage response for effective fungal defence.Citation70 Hosts defensive metabolic responses and the parallel evasive mechanistic pathways in fungi demonstrate a need to understand metabolic networks in host mycobiome in health and disease.Citation52
Bacteriome-mycobiome interaction
In a series of animal model studies, the interaction between host-mycobiome and fungus-bacterium has been linked to the health status of the host (expansion of the in vivo data set sources is listed in ).Citation37,Citation72–74 The interaction of fungi and bacteria occurs on several different levels. Physical contact can occur between members of microbial communities leading to the exchange of secreted products and communication between the two cell surfaces. Cell signalling between microbes also provides regulation and trade of metabolites and genetic materials through different mechanisms, such as horizontal gene transfer, signal transduction and interaction with the host.Citation75,Citation76 This exchange allows for an interplay between microbes that can maintain the ecosystem’s resilience or drive a dysbiosis state. This interplay within the host means there is a knock-on effect for the host, potentially resulting in disease pathology. For example, C. tropicalis is significantly more abundant in Crohn’s disease (CD) patients in comparison to non-CD relatives.Citation37 This increased abundance correlates with increased abundance of some bacterial species and related improved biofilm formation and disease severity. These interactions can potentially be bi-directional. For example, Escherichia coli, Serratia marcescens, and Candida tropicalis together exhibit enhanced biofilm formation, giving a thicker, more robust film.Citation37 Furthermore, the production of bile acids (e.g. cholic acid) from the microbiota and environment have been shown to promote the growth and morphogenetic plasticity of C. albicans,Citation77 whilst hexadecanedioic and caproic acids have demonstrated antifungal activity.Citation29
Recently, multiple studies have begun to elucidate how our bacteriome and mycobiome interact with each other and the contribution of these interactions to human health and disease. In particular, the bacteriome and mycobiome can alter each other’s composition.Citation28 For example, competition between bacteria and fungi leads to stunted Candida growth.Citation78 The release of various compounds can drive these interactions. For instance, Pseudomonas aeruginosa releases bacterial compounds called phenazines, in particular pyocyanin, that suppress A. fumigatus, C. albicans and Cryptococcus neoformans growth in the lungs of cystic fibrosis patients (in vitro; ).Citation79 Nor are these interactions purely suppressive. Dimethyl sulphide, a volatile compound released by strains of P. aeruginosa derived from human sputum samples, stimulates the growth of A. fumigatus within in vitro experiments.Citation80 These bacterial-produced compounds demonstrate the varied effects of secondary metabolites produced by the bacteriome on the mycobiome. Moreover, P. aeruginosa has been shown to attach to C. albicans hyphae, killing them via type IV pili.Citation81 Similarly, Staphylococcus aureus and C. albicans are frequently co-isolated in immunocompromised patients.Citation82,Citation83 Serum analysis showed S. aureus has preferential adherence to C. albicans driving hyphae formation. Interestingly, S. aureus formed microcolonies with a halo formation on the surface of C. albicans biofilm, resulting in an increase in vancomycin resistance.Citation84 C. albicans hyphae initiates innate responses in attracting phagocyte cells for transporting the fungi into a protected intracellular host environment. S. aureus is shown to take advantage of this by escaping intracellular killing and disseminating to cause sepsis.Citation85
Alteration of the bacteriome composition drives diverse changes in metabolite levels in the gut and effects on the mycobiome. For example, antibiotic-treated mice have increased levels of C. albicans in their gut.Citation77 Whilst it could be argued that this is purely due to an increase in the available nutrients, this is unlikely to be the sole reason, given the lack of presence of this species in mice under normal conditions. There is evidence of SCFA release by bacteria incurring modifications in host transcription activation,Citation86–88 signallingCitation89,Citation90 and chromatin changesCitation91 in the host. The increased fungal presence may be due to bacterial species reduction resulting in diminished SFCA production, giving rise to changes in pH. Therefore, the changes in bacterial abundance due to antibiotics drive shifts in gut bacteriome composition and abundance, altering cross-talk with the mycobiome. There is further potential for fungi to interact with SCFAs. Aspergillus can produce SCFAs from aromatic compounds biodegradation. A. fumigatus degrades these compounds to produce acetic acid, among others.Citation92 Investigation of the effects of SCFAs on C. albicans in the presence of bacteria found they control yeast growth.Citation93 Further investigation revealed that butyrate and lactic acid inhibit hyphal germinationCitation94,Citation95 whilst sodium butyrate increases phagocytic macrophage rates along with increasing production of nitric acid.Citation96 SCFAs also induce biofilm formation in C. albicans, decreasing its metabolic activity.Citation77 Acetate exhibited the most potent inhibitory effects on C. albicans, directly preventing germ tube formation and adherence independent of pH.Citation77 These studies indicate that microbially-produced SCFAs (from either bacteriome or mycobiome) affect both confirmation and community structure of the mycobiome, potentially leading to dysbiosis.
Mycobiome metabolism
Fungi produce an expansive variety of metabolites with varied impacts on their survival, as well as host and microbiota interactions. For example, the fungal metabolite N-acetyl-L-glutamic acid has hypotensive effects.Citation29 The natural ability of fungi to produce this cornucopia leads to ripple effects in their environment.Citation97 The metabolic activity of fungal communities has a pivotal role in microbiome modulation, contributing to disease pathogenesis and pathology. Fungi can catabolise a panoply of different substrates, including carbohydrates, amino acids, lipids, proteins, and vitamins utilising both primary and secondary metabolism. Primary metabolism is involved in the growth and development of the cell, whilst secondary metabolism increases fungal viability in the environment. Fungi produce a variety of secondary metabolites, including acids, toxins, polyols and sugars that mediate cross-talk and are considered a by-product of other reactions.Citation98
Secondary metabolites are key by-products of primary reactions and play a crucial role in shaping the interaction within a niche. In the elucidation of enzymatic processes, fungal secondary metabolites have been classed into several groups depending on the biosynthesis of these products. The most notable involves polyketide synthases (PKS), non-ribosomal peptides synthases (NRPS), fatty acid derivatives, terpenoids, and steroids.Citation99–102 Polyketide (PKS) class consists of derivatives of fatty acids and the output of acetyl CoA pathways that allow for various structures and functions.Citation102,Citation103 Non-ribosomal peptides (NRPS) are derived from amino acids and cannot perform RNA transcription.Citation99,Citation101,Citation102,Citation104 Terpenes class are generated from terpene synthases, a product of the mevalonic acid pathway and produced as steroid compounds.Citation105 Terpenoids are a modified class of terpenes. Dimethyallyl tryptophan (DMATs) class is comparable to the ergot alkaloid class and are usually pharmacologically active compounds responsible for producing indoles.Citation106–108 As the detection of growing numbers of metabolites progresses, methods in finding genes responsible for secondary metabolites are also being developed. These genes are called biosynthetic gene clusters (BGCs) and are based on the chromosomal arrangement of the synthesis of secondary metabolites. These BGCs ranges from smaller clusters relating to synthases to a larger cluster of genes that include transcription factors, as detailed in Keller et al. (2019). These genes are globally regulated by transcriptional activity, not all necessarily from within the cluster, thus indicating that transcription and epigenetic activation of BGCs is dependent on external factors. These factors include environmental stimuli, metal presence, nutritional availability, temperature, pH and light, triggered by regulatory pathways.Citation76
The mycobiome includes metabolic processes with an extensive pool of secondary metabolite production that can be key in driving or preventing a pathogenic switch. For instance, metabolites and small molecules can activate the host immune system via recognition of foreign material or the release of toxins, driving inflammatory responses. Potential effects of known metabolites produced by Candida, Penicillium and Aspergillus species and their possible metabolic pathways have been illustrated in , demonstrating a likely impact on the host during infection.
Figure 1. Fundamental metabolic interactions between host-mycobiome in health and disease. A. indicates Candida contribution to the production of γ-amino butyric acid (GABA) through the tricarboxylic acid cycle to produce succinate may perceptively occur during systemic infection. GABA has a role in dampening the effect of the nervous system, and generally, deficiency involves epilepsy.Citation109 Indirectly targeting mycobiome in specific regions such as Candida species can have a better effect than providing analogues of GABA that has been identified to lead to anxiety, stress and seizures. Exploring this metabolic pathway of the mycobiome, especially targeting the succinate pathway, the production of semi-aldehydrase could lead to alteration of disease with better regulation from the microbiota, as alternatively, high levels of GABA have been linked to Alzheimer’s disease.Citation110 B. Penicillium species’ ability to produce the mycotoxin called citrinin is toxic to cause renal nephrosis.Citation111–113 Drugs have been developed to inhibit the effect of citrinin in hepatoma, and several animal studies have shown the benefit.Citation114 Ostry et al., 2013 have highlighted the dangerous effect of citrinin in dietary sources.Citation115 There has been no established link of mycobiome being the contributor of toxin in humans and whether diverting the polyketide pathway can reduce renal cell toxicity. C Aflatoxin is a common secondary metabolite of the Aspergillus family. It has been associated with cancer morbidity and inducing tumour suppressor gene p53.Citation116–119 The potential effect of the presence of Aspergillus needs to be investigated and whether manipulating the metabolic pathway can give a better indicator of being able to reverse the effects of aflatoxin by reducing the levels of the malonyl-coA pathway. All these potential pathways give us a clear indication of how the study of the metabolism of the mycobiome is essential in understanding pathogenesis across a wide breadth of diseases.
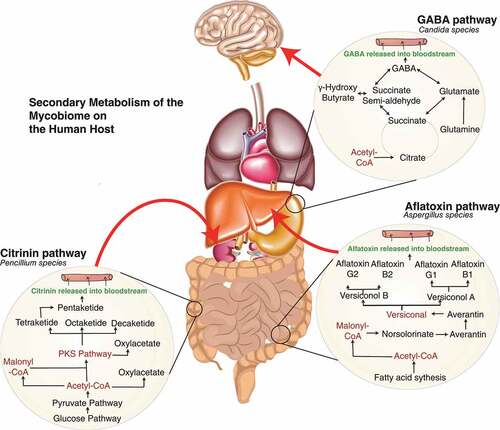
Secondary metabolites can have a knock-on effect on host metabolism, and this has been used extensively for therapeutic effects (). One metabolite isolated from Aspergillus species is naptho-γ-pyrones. This natural metabolite has various biological effects, including anti-bacterial and anti-oxidant activity.Citation120–124 The wide variety of fungal metabolite functions makes them ideal targets for therapeutic use. This can be done by targeting metabolic pathways to derive benefits. For example, by driving immunometabolic shifts that affect glucose competition and redirecting pathways for cancer treatment.Citation125 As well as direct impacts on the host whether as colonisers or during infection, these metabolites are ideal potential drugs for treating the microbiome directly. For example Penicillium produces a mycotoxin called citrinin that has antibiotic properties against bacteria and can have a toxic effect on kidney and liver.Citation126–128 Secondary metabolites can thus conceivably both provide diagnostic markers for the detection of disease and manipulate metabolic pathways, altering host health status. System biology contributes useful tools in investigating metabolic pathways in fungi in the context of the human mycobiome.
Table 3. Highlight the effect of metabolites on the host. Identification of a wide variety of secondary metabolites from various fungal species, the class grouping attributed to the metabolite, based upon the synthesis, fungal species responsible for chemically engineering the metabolite and the effect on the human host. The properties include anti-bacterial activity to cancerous effect. The extended table includes the effect on the environment, marine biology and produce. *IL = interleukin ** blue highlight indicates human effect.
Systems and synthetic biology approach to elucidate interactions
To date, mycobiome studies have primarily been grounded in culture-based techniques that are hostage to the diverse requirements of each community member, meaning many fungi remain undetected and undiscovered.Citation4 The development of molecular techniques such as 18S ribosomal RNA and inter transcribed spacer (ITS) sequencing has profoundly impacted the detection of fungi and the characterisation of the mycobiome. However, these techniques are limited in understanding the mycobiome community’s overall structure, function, and dynamic existence on a large-scale platform.Citation129 Many mycologists face the obstacle of limited bioinformatic tools and databases available compared to bacteriologists. With improving next generation sequencing (NGS) technologies and lower costs, multi-omics data generation is increasing rapidly. Multi-omics data includes metagenomics, transcriptomics, proteomics, metabolomics and fluxomics that impart in-depth information that has enabled us to learn about microbiota interactions ().Citation130–132 Each multi-omics dataset provides a different view on either single species or communities of varied microbes.Citation133,Citation134 Experimental studies and multi-omics data generate abundant information; however, we currently lack the ability to fully integrate this information to give us a systematic overview. Individual data have so far failed to elucidate the interactions involved in fungi-fungi, fungi-bacteria and fungi-host relationships. Systems biology, by its holistic approach and mathematical models, provides a computational platform to integrate the different multi-omics datasets, presenting a better structure to understand the relationship between gene, protein and metabolites, and additionally to explore the interactions between organisms in a complex community.Citation33,Citation135–137 Among the mathematical models as the core of the systems biology approach, genome-scale metabolic models (GSMM) has proven to enable the genotype-phenotype relationships through a series of connected gene-protein-reaction links, providing a powerful predictive toolkit.Citation135 GSMM have been applied in different microbial and human studies as a discovery platform to propose novel markers, treatments and bioproduction enhancement.Citation138–141 GSMM predictions and discovery can be further evaluated and validated using synthetic biology, such as CRISPR-cas systems (Clustered Regularly Interspace Short Palindromic Repeat) for genome editing to test and manipulate molecular and metabolic pathways.Citation142
Figure 2. System and synthetic biology approach in investigating mycobiome’s important role in the gut-liver axis. This image shows the interaction of the microbiome-mycobiome with the host cells in the gut, translocation of intermediaries through the blood, and prospectively leading to the composition of the liver environment to change. The biological knowledge from the collection of omics data such as proteome, metabolome and transcriptome generates information that can be integrated into functional mathematical models. The structural data is placed into the stoichiometric matrix and after several steps of adding biochemical information the draft of the GSMM can be reconstructed and further validated for new biological interpretation and predictions. Different constraint can be applied on the GSMMs to investigate the models for novel discovery. CRISPR as one of the gene editing approaches in validating predictions based on GSMM; these can be done using the CRISPR-cas9 system to perform genome modification such as gene slicing, gene mutation, secondary metabolite modification, base editing, and gene editing tagging and gene overexpression, down-regulation methods. The application can be used in tackling drug resistance, alteration of the metabolic pathway with the possibility of amending toxic pathways in pathogenesis, rationalising gene essentiality and genome-wide screening. The application of these potential methods in assessing the mycobiome community and particularly within the gut-liver axis.
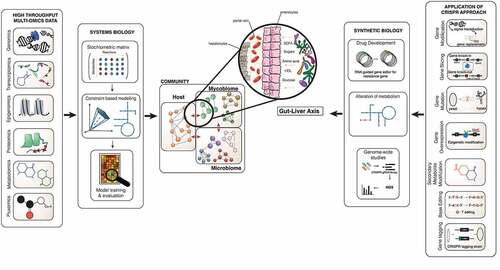
The S. cerevisiae model is the benchmarking fungal GSMM with a comprehensive network structure including the 3D structure and protein kinetics.Citation143 This GSMM is a reference model used to generate the scaffold of other fungal species models.Citation30 Other well-constructed fungal reference models that have been generated include Scheffersomyces stipitis,Citation144 C. glabrataCitation145,Citation146 and Pichia pastoris.Citation147 Fungal GSMM are mainly used to inform the metabolic engineering experiments, increase the efficiency of the process and markers, such as modelling yeast suggested methods for increasing vanillin production by 5-fold.Citation148 Similarly, the model’s biological interpretation of Yarrowia lipolytica metabolic pathways identified that this fungal species is an ideal producer of di-carboxylic acid using a lipid pathway.Citation149
The application of GSMM technologies to the human microbiome has identified cross-feeding of metabolites between host and microbiota in health and disease.Citation139 This has led to a recent increase in studies predicting biological behaviour, the use of biotics for metabolic diseases, personalised medicine, and drug targets for pathogens.Citation149–154 Modelling has implicated the production of SCFAs from microbial fermentation in the host in CD aetiology; thus, adjusting diet can control the presence of SCFAs in patients and modulate disease.Citation155 Exploring IBD compared to healthy cohorts showed an increase in vitamin metabolism in IBD patients, whilst a closer inspection of species in the microbiome using GSMMs unveiled vitamin and biotin producing gut organisms in those suffering from IBD,Citation156 whilst using modelling of the microbiome in neurological diseases including Parkinson’s DiseaseCitation157–160 and Alzheimer’s diseaseCitation161,Citation162 has identified microbiome perturbations, causing flux changes in pathways and production of SCFAs that ultimately potentially cause disease. Studies on community modelling of the microbiome predict metabolic interactions between species with the overall metabolic contribution of each species determined.Citation163–165 Research in this area remains in its infancy as the development of algorithms and tools used to explore community modelling continues, but the potential is beyond doubt.
As there are several available fungal GSMM, they could be implemented in studying the mycobiome to elucidate the complex interactions between mycobiome, bacteriome and host, thereby explaining the metabolic role of fungi in disease pathophysiology. Exploring the mycobiome with GSMM will significantly improve our understanding of fungal interactions with the host in health and disease. Synthetic biology can then be used to validate and assess genetic circuits and metabolic frameworks for complex interactions. Recently, CRISPR-based systems for repression and activation in C. albicans have been used to investigate its regulatory system in the clinical context.Citation166
The difficulties lie in exploiting fungal models and synthetic biology due to annotation accuracy with high plasticity of metabolic pathways, diverse morphology, and evolutionary adaptive traits. There is a lagging development of fungal GSMM and applying CRISPR system in clinical and healthcare settings due to a lack of quantitative tools to study fungal genomes a lack of annotation and validation in the model. The global overview provided by this approach offers the potential to drive strategies to manipulate the mycobiome to make predictions, test hypotheses, propose clinical areas for manipulation, identify new diagnostics target areas with better understanding, and discover novel therapeutic targets.Citation135,Citation136,Citation167
Conclusion
Understanding the behaviour of fungi, both individually and as a community, is essential in elucidating the role of the mycobiome in health and disease. Untethering the distinct metabolic interactions and effects of secondary metabolism provide an opportunity for disease intervention, determining the point at which dysbiosis arises, identifying biomarkers for better diagnostics and novel therapeutic targets. Metabolism is a holistic and integrative subject that combines genetics, molecular pathways, signalling and environmental factors. Multi-disciplinary tools and methods such as system biology are needed to develop in silico models as a predictive tool to validate current datasets and knowledge. In doing so, we will generate a platform to answer how mycobiome metabolism affects the host in health and disease.
Background information – what is a genome-scale metabolic model (GSMM) and CRISPR
Genome-scale metabolic models (GSMM) are a systematic and curated method to establish genotype-phenotype relationships. GSMM aim to bridge together the complex network of genes, reactions and thousands of metabolites in silico while sustaining full metabolic flux functionality of the system. The functionality of a model refers to the natural ability of the model to undertake reactions, a realistic rate of energy consumption, rate of energy production and apply physio-chemical laws and environmental input to create a system that is true-to-life.Citation168 The conversion of the reactions into a stoichiometric matrix allows mathematical inferences to integrate data into a predictive biological framework called constraint-based modelling.Citation169 This developmental process of integration requires automated and manual curation for efficient quality standards. The GSMM community has developed a MEMOTE suite package to ensure the models’ standardisation and functional feasibility.Citation170 With synthetic biology approach of genome engineering techniques pave the way for validating fungal species’ biological mechanisms underlying these systematic alterations in response to different environmental changes such as response to anti-fungal drug and the host organism’s reaction during fungal infection (Martins-Santana et al., 2018).Citation142 demonstrates the step-by-step inferences for creating a GSMM and synthetic biology to determine the interaction of mycobiome within the community.
The creation of a GSMM includes two methods: firstly, a top-down approach, which integrates experimental data into a mathematical model. This method makes it possible to create a network of reactions and metabolic outputs through pathways. The study of metabolism can provide more accuracy and specificity with further predictive analysis and assessment. Secondly, the bottom-up approach includes defined knowledge and information sourced from readily available studies and the input of current literature (Machado et al., 2018).Citation171 The current approach to reconstructing GSMM combines top-down and bottom-up approaches using automated tools such as the RAVEN toolbox (Agren et al., 2013).Citation172 GSMM can be used in constraint-based modelling to predict the flux distribution and secretion of metabolites within the organism under certain constraints such as the intake of substrates and governing specific objective functions such as growth, thereby providing information that would be difficult to generate in vitro experiments (Bordbar et al., 2014).Citation169 As of 2019, there are reconstructed GSMM for 434 bacteria, 40 archaea and 117 eukaryotes (Gu et al., 2019).Citation30 The majority of these GSMM are used in the simulation of a single organism. However, their use can go further to study the metabolic cross-talk of microbial communities.
CRISPR (Clustered Regularly Interspace Short Palindromic Repeat-associated (Cas) is a robust and customised method of gene editing which is adapted from the immune mechanism in bacteria and archaea against viral (bacteriophage) invasion (Mojica et al. 2005).Citation173 What makes Type-II CRISPR-Cas9 a well-established CRISPR approach in the genome-editing field is the ability of manipulation at genome level using just chimeric single guide RNA, making double-strand breaks to insert-deletion gene (indel) using cas9 nuclease, followed by homologous/non-homologues recombination and multiple genes indel (Pourcel et al. 2005; Brouns et al. 2008).Citation109–119,Citation174,Citation175
Supplemental Material
Download Zip (1.2 MB)Acknowledgments
The review was supported by the Engineering and Physical Sciences Research Council (EPSRC) EP/S001301/1 and BBSRC grant -BB/S016899/1. NB is supported by a KCL-FoDOCs studentship. SS and DLM are part-funded by Unilever and Sanofi.
Disclosure statement
The authors declare no conflict of interest.
Supplementary material
Supplemental data for this article can be accessed online at https://doi.org/10.1080/19490976.2022.2121576.
Additional information
Funding
References
- Brown GD, Denning DW, Gow NAR, Levitz SM, Netea MG, White TC. Hidden killers: human fungal infections. Sci Transl Med. 2012;4(165):165rv13–19. doi:10.1126/scitranslmed.3004404.
- Brown GD, Denning DW, Levitz SM. Tackling human fungal infections. Science. 2012;336(6082):647. doi:10.1126/science.1222236.
- Nash AK, Auchtung TA, Wong MC, Smith DP, Gesell JR, Ross MC, Stewart CJ, Metcalf GA, Muzny DM, Gibbs RA, et al. The gut mycobiome of the Human Microbiome Project healthy cohort. Microbiome. 2017;5(1):153. doi:10.1186/s40168-017-0373-4.
- Seed PC. The human mycobiome. Cold Spring Harb Perspect Med. 2015;5(5):a019810–a019810. doi:10.1101/cshperspect.a019810.
- Forbes JD, Bernstein CN, Tremlett H, Van Domselaar G, Knox NC. A fungal world: could the gut mycobiome be involved in neurological disease?. Front Microbiol. 2019;10:3249. doi:10.3389/fmicb.2018.03249.
- Chin VK, Yong VC, Chong PP, Amin Nordin S, Basir R, Abdullah M. Mycobiome in the gut: a multiperspective review. Mediators Inflamm. 2020;2020:1–16. doi:10.1155/2020/9560684.
- Schulze J, Sonnenborn U. Yeasts in the gut: from commensals to infectious agents. Dtsch Arzteblatt Int. 2009;106:837–842.
- Suhr MJ, Hallen-Adams HE. The human gut mycobiome: pitfalls and potentials-a mycologist’ s perspective the human gut mycobiome: pitfalls and potentials-a mycologist’s perspective. 2015. doi:10.3852/15-147.
- Qin J, Li R, Raes J, Arumugam M, Burgdorf KS, Manichanh C, Nielsen T, Pons N, Levenez F, Yamada T, et al. A human gut microbial gene catalogue established by metagenomic sequencing. Nature. 2010;464(7285):59–65. doi:10.1038/nature08821.
- Huffnagle GB, Noverr MC. The emerging world of the fungal microbiome. Trends Microbiol. 2013;21(7):334–341. doi:10.1016/j.tim.2013.04.002.
- Baker JL, Bor B, Agnello M, Shi W, He X. Ecology of the Oral Microbiome: beyond Bacteria. Trends Microbiol. 2017;25(5):362–374. doi:10.1016/j.tim.2016.12.012.
- Auchtung TA, Fofanova TY, Stewart CJ, Nash AK, Wong MC, Gesell JR, Auchtung JM, Ajami NJ, Petrosino JF. Investigating colonization of the healthy adult gastrointestinal tract by fungi. mSphere. 2018;3(2):e00092–18. doi:10.1128/mSphere.00092-18.
- Perrone G, Gallo A. Aspergillus species and their associated mycotoxins. In: Moretti A, Susca A, editors. Mycotoxigenic fungi: methods and protocols. Springer;2017. p. 33–49. doi:10.1007/978-1-4939-6707-0_3.
- Hoffmann C, Dollive S, Grunberg S, Chen J, Li H, Wu GD, Lewis JD, Bushman FD. Archaea and fungi of the human gut microbiome: correlations with diet and bacterial residents. PLoS ONE. 2013;8(6):e66019. doi:10.1371/journal.pone.0066019.
- Strati F, Di Paola M, Stefanini I, Albanese D, Rizzetto L, Lionetti P, Calabrò A, Jousson O, Donati C, Cavalieri D, De Filippo C. Age and gender affect the composition of fungal population of the human gastrointestinal tract. Front Microbiol. 2016;7. doi:10.3389/fmicb.2016.01227
- Borges FM, de Paula TO, Sarmiento MRA, de Oliveira MG, Pereira MLM, Toledo IV, Nascimento TC, Ferreira-Machado AB, Silva VL, Diniz CG. Fungal diversity of human gut microbiota among eutrophic, overweight, and obese individuals based on aerobic culture-dependent approach. Curr Microbiol. 2018;75(6):726–735. doi:10.1007/s00284-018-1438-8.
- Suhr MJ, Banjara N, Hallen‐Adams HE. Sequence-based methods for detecting and evaluating the human gut mycobiome. Lett Appl Microbiol. 2016;62(3):209–215. doi:10.1111/lam.12539.
- Huseyin CE, Rubio RC, O’Sullivan O, Cotter PD, Scanlan PD. The fungal frontier: a comparative analysis of methods used in the study of the human gut mycobiome. Front Microbiol. 2017;8. doi:10.3389/fmicb.2017.01432.
- Wisecaver JH, Slot JC, Rokas A, Stajich JE. The evolution of fungal metabolic pathways. PLOS Genet. 2014;10(12):e1004816. doi:10.1371/journal.pgen.1004816.
- Richardson JP, Moyes DL. Adaptive immune responses to Candida albicans infection. Virulence. 2015;6(4):327–337. doi:10.1080/21505594.2015.1004977.
- Moyes DL, Richardson JP, Naglik JR. Candida albicans- epithelial interactions and pathogenicity mechanisms: scratching the surface. Virulence. 2015;6(4):338–346. doi:10.1080/21505594.2015.1012981.
- Shanahan F, Hill C. Language, numeracy and logic in microbiome science. Nat Rev Gastroenterol Hepatol. 2019;16(7):387–388. doi:10.1038/s41575-019-0163-5.
- Frey-Klett P, Burlinson P, Deveau A, Barret M, Tarkka M, Sarniguet A. Bacterial-fungal interactions: hyphens between agricultural, clinical, environmental, and food microbiologists. Microbiol Mol Biol Rev. 2011;75(4):583–609. doi:10.1128/MMBR.00020-11.
- Kelesidis T, Pothoulakis C. Efficacy and safety of the probiotic Saccharomyces boulardii for the prevention and therapy of gastrointestinal disorders: ther. Adv Gastroenterol. 2011. doi:10.1177/1756283X11428502.
- Kurrey NK, Anu-Appaiah KA, Rao RP. Probiotic yeasts inhibit virulence of non-albicans candida species lohith kunyeit,a,b,c. mBio. 2019;10.
- Huseyin CE, O’Toole PW, Cotter PD, Scanlan PD. Forgotten fungi-the gut mycobiome in human health and disease. FEMS Microbiol Rev. 2017;41(4):479–511. doi:10.1093/femsre/fuw047.
- David LA, Maurice CF, Carmody RN, Gootenberg DB, Button JE, Wolfe BE, Ling AV, Devlin AS, Varma Y, Fischbach MA. Diet rapidly and reproducibly alters the human gut microbiome. Nature. 2014;505(7484):559–563. doi:10.1038/nature12820.
- Sam Q, Chang M, Chai L. The fungal mycobiome and its interaction with gut bacteria in the host. Int J Mol Sci. 2017;18(2):330. doi:10.3390/ijms18020330.
- Mar Rodríguez M, Marin-Garcia P, Xifra G, Vendrell J, Jové M, Pamplona R, Ricart W. Obesity changes the human gut mycobiome. Sci Rep. 2015;5(1):14600. doi:10.1038/srep14600.
- Gu C, Kim GB, Kim WJ, Kim HU, Lee SY. Current status and applications of genome-scale metabolic models. Genome Biol. 2019;20(1):121. doi:10.1186/s13059-019-1730-3.
- Sokol H, Leducq V, Aschard H, Pham H-P, Jegou S, Landman C, Cohen D, Liguori G, Bourrier A, Nion-Larmurier I. Fungal microbiota dysbiosis in IBD. Gut. 2017;66(6):1039–1048. doi:10.1136/gutjnl-2015-310746.
- Ott SJ, Kühbacher T, Musfeldt M, Rosenstiel P, Hellmig S, Rehman A, Drews O, Weichert W, Timmis KN, Schreiber S. Fungi and inflammatory bowel diseases: alterations of composition and diversity. Scand J Gastroenterol. 2008;43(7):831–841. doi:10.1080/00365520801935434.
- Witherden EA, Moyes DL, Bruce KD, Ehrlich SD, Shoaie S. Using systems biology approaches to elucidate cause and effect in host–microbiome interactions. Curr Opin Syst Biol. 2017;3:141–146. doi:10.1016/j.coisb.2017.05.003.
- Kumamoto CA. Inflammation and gastrointestinal Candida colonization. Curr Opin Microbiol. 2011;14(4):386–391. doi:10.1016/j.mib.2011.07.015.
- Gerard R, Sendid B, Colombel JF, Poulain D, Jouault T. An immunological link between Candida albicans colonization and Crohn’s disease. Crit Rev Microbiol. 2015;41(2):135–139. doi:10.3109/1040841X.2013.810587.
- Trojanowska D, Zwolińska-Wcisło M, Tokarczyk M, Kosowski K, Mach T, Budak A. The role of Candida in inflammatory bowel disease. Estimation of transmission of C. albicans fungi in gastrointestinal tract based on genetic affinity between strains. Med Sci Monit Int Med J Exp Clin Res. 2010;16:CR451–457.
- Hoarau G, Mukherjee PK, Gower-Rousseau C, Hager C, Chandra J, Retuerto MA, Neut C, Vermeire S, Clemente J, Colombel JF. Bacteriome and mycobiome interactions underscore microbial dysbiosis in familial Crohn’s disease. mBio. 2016;7(5). doi:10.1128/mBio.01250-16.
- Severance EG, Alaedini A, Yang S, Halling M, Gressitt KL, Stallings CR, Origoni AE, Vaughan C, Khushalani S, Leweke FM. Gastrointestinal inflammation and associated immune activation in schizophrenia. Schizophr Res. 2012;138(1):48–53. doi:10.1016/j.schres.2012.02.025.
- Severance EG, Gressitt KL, Stallings CR, Katsafanas E, Schweinfurth LA, Savage CL, Adamos MB, Sweeney KM, Origoni AE, Khushalani S, Leweke FM. Candida albicans exposures, sex specificity and cognitive deficits in schizophrenia and bipolar disorder. Npj Schizophr. 2016;2(1):1–7. doi:10.1038/npjschz.2016.18.
- Enaud R, Vandenborght L-E, Coron N, Bazin T, Prevel R, Schaeverbeke T, Berger P, Fayon M, Lamireau T, Delhaes L. The mycobiome: a neglected component in the microbiota-gut-brain axis. Microorganisms. 2018;6(1):22. doi:10.3390/microorganisms6010022.
- Alonso R, Pisa D, Marina AI, Morato E, Rabano A, Carrasco L. Fungal infection in patients with Alzheimer’s disease. J Alzheimers Dis JAD. 2014;41(1):301–311. doi:10.3233/JAD-132681.
- Alonso R, Pisa D, Aguado B, Carrasco L. Identification of fungal species in brain tissue from alzheimer’s disease by next-generation sequencing. J Alzheimers Dis JAD. 2017;58(1):55–67. doi:10.3233/JAD-170058.
- Pisa D, Alonso R, Rábano A, Rodal I, Carrasco L. Different brain regions are infected with fungi in alzheimer’s disease. Sci Rep. 2015;5(1):1–13. doi:10.1038/srep15015.
- Benito-León J, Laurence M. The role of fungi in the etiology of multiple sclerosis. Front Neurol. 2017;8:535. doi:10.3389/fneur.2017.00535.
- Severance EG, Gressitt KL, Stallings CR, Katsafanas E, Schweinfurth LA, Savage CL, Adamos MB, Sweeney KM, Origoni AE, Khushalani S, Dickerson FB. Probiotic normalization of Candida albicans in schizophrenia: a randomized, placebo-controlled, longitudinal pilot study. Brain Behav Immun. 2017;62:41–45. doi:10.1016/j.bbi.2016.11.019.
- Aykut B, Pushalkar S, Chen R, Li Q, Abengozar R, Kim JI, Shadaloey SA, Wu D, Preiss P, Verma N, Guo Y. The fungal mycobiome promotes pancreatic oncogenesis via activation of MBL. Nature. 2019;1–4. doi:10.1038/s41586-019-1608-2.
- Mukherjee PK, Chandra J, Retuerto M, Sikaroodi M, Brown RE, Jurevic R, Salata RA, Lederman MM, Gillevet PM, Ghannoum MA. Oral mycobiome analysis of HIV-infected patients: identification of pichia as an antagonist of opportunistic fungi. PLoS Pathog. 2014;10(3):e1003996. doi:10.1371/journal.ppat.1003996.
- Remmele CW, Luther CH, Balkenhol J, Dandekar T, Müller T, Dittrich MT. Integrated inference and evaluation of host-fungi interaction networks. Front Microbiol. 2015;6:764. doi:10.3389/fmicb.2015.00764.
- Jiang TT, Shao T-Y, Ang WXG, Kinder JM, Turner LH, Pham G, Whitt J, Alenghat T, Way SS. Commensal fungi recapitulate the protective benefits of intestinal bacteria. Cell Host Microbe. 2017;22(6):809–816.e4. doi:10.1016/j.chom.2017.10.013.
- Brown GD. Innate antifungal immunity: the key role of phagocyteS. Annu Rev Immunol. 2011;29(1):1–21. doi:10.1146/annurev-immunol-030409-101229.
- Richardson JP, Moyes DL, Ho J, Naglik JR. Candida innate immunity at the mucosa. Semin Cell Dev Biol. 2019;89:58–70. doi:10.1016/j.semcdb.2018.02.026.
- Tucey TM, Verma J, Harrison PF, Snelgrove SL, Lo TL, Scherer AK, Barugahare AA, Powell DR, Wheeler RT, Hickey MJ. Glucose homeostasis is important for immune cell viability during candida challenge and host survival of systemic fungal infection. Cell Metab. 2018;27(5):988–1006.e7. doi:10.1016/j.cmet.2018.03.019.
- Chai LYA, Netea MG, Vonk AG, Kullberg B-J. Fungal strategies for overcoming host innate immune response. Med Mycol. 2009;47(3):227–236. doi:10.1080/13693780802209082.
- Netea MG, Brown GD, Kullberg BJ, Gow NAR. An integrated model of the recognition of Candida albicans by the innate immune system. Nat Rev Microbiol. 2008;6(1):67–78. doi:10.1038/nrmicro1815.
- Mogilnicka I, Ufnal M. Gut mycobiota and fungal metabolites in human homeostasis. Curr Drug Targets. 2019;20(2):232–240. doi:10.2174/1389450119666180724125020.
- Huć T, Nowinski A, Drapala A, Konopelski P, Ufnal M. Indole and indoxyl sulfate, gut bacteria metabolites of tryptophan, change arterial blood pressure via peripheral and central mechanisms in rats. Pharmacol Res. 2018;130:172–179. doi:10.1016/j.phrs.2017.12.025.
- Huc T, Konop M, Onyszkiewicz M, Podsadni P, Szczepańska A, Turło J, Ufnal M. Colonic indole, gut bacteria metabolite of tryptophan, increases portal blood pressure in rats. Am J Physiol Regul Integr Comp Physiol. 2018;315(4):R646–R655. doi:10.1152/ajpregu.00111.2018.
- Tremaroli V, Bäckhed F. Functional interactions between the gut microbiota and host metabolism. Nature. 2012;489(7415):242–249. doi:10.1038/nature11552.
- Claesson MJ, Jeffery IB, Conde S, Power SE, O’Connor EM, Cusack S, Harris HMB, Coakley M, Lakshminarayanan B, O’Sullivan O. Gut microbiota composition correlates with diet and health in the elderly. Nature. 2012;488(7410):178–184. doi:10.1038/nature11319.
- Koh A, De Vadder F, Kovatcheva-Datchary P, Bäckhed F. From dietary fiber to host physiology: short-chain fatty acids as key bacterial metabolites. Cell. 2016;165(6):1332–1345. doi:10.1016/j.cell.2016.05.041.
- Raffa N, Keller NP, Sheppard DC. A call to arms: mustering secondary metabolites for success and survival of an opportunistic pathogen. PLoS Pathog. 2019;15(4):e1007606. doi:10.1371/journal.ppat.1007606.
- Dietl A-M, Meir Z, Shadkchan Y, Osherov N, Haas H. Riboflavin and pantothenic acid biosynthesis are crucial for iron homeostasis and virulence in the pathogenic mold Aspergillus fumigatus. Virulence. 2018;9(1):1036–1049. doi:10.1080/21505594.2018.1482181.
- Meir Z, Osherov N. Vitamin biosynthesis as an antifungal target. J Fungi. 2018;4(72):72. doi:10.3390/jof4020072.
- Björn LO, Wang T. Vitamin D in an ecological context. Int J Circumpolar Health. 2000;59:26–32.
- Yamamoto EA, Jørgensen TN. Relationships between vitamin D, gut microbiome, and systemic autoimmunity. Front Immunol. 2020;10. doi:10.3389/fimmu.2019.03141.
- Klassert TE, Bräuer J, Hölzer M, Stock M, Riege K, Zubiría-Barrera C, Müller MM, Rummler S, Skerka C, Marz M. Differential effects of vitamins A and D on the transcriptional landscape of human monocytes during infection. Sci Rep. 2017;7(1):40599. doi:10.1038/srep40599.
- Weerasinghe H, Traven A. Immunometabolism in fungal infections: the need to eat to compete. Curr Opin Microbiol. 2020;58:32–40. doi:10.1016/j.mib.2020.07.001.
- Domínguez-Andrés J, Arts RJW, ter Horst R, Gresnigt MS, Smeekens SP, Ratter JM, Lachmandas E, Boutens L, van de Veerdonk FL, Joosten LAB. Rewiring monocyte glucose metabolism via C-type lectin signaling protects against disseminated candidiasis. PLOS Pathog. 2017;13(9):e1006632. doi:10.1371/journal.ppat.1006632.
- Lachmandas E, Beigier-Bompadre M, Cheng S-C, Kumar V, van Laarhoven A, Wang X, Ammerdorffer A, Boutens L, de Jong D, Kanneganti T-D. Rewiring cellular metabolism via the AKT/mTOR pathway contributes to host defence against Mycobacterium tuberculosis in human and murine cells. Eur J Immunol. 2016;46(11):2574–2586. doi:10.1002/eji.201546259.
- Gonçalves SM, Duarte-Oliveira C, Campos CF, Aimanianda V, ter Horst R, Leite L, Mercier T, Pereira P, Fernández-García M, Antunes D. Phagosomal removal of fungal melanin reprograms macrophage metabolism to promote antifungal immunity. Nat Commun. 2020;11(1):2282. doi:10.1038/s41467-020-16120-z.
- Stappers MHT, Clark AE, Aimanianda V, Bidula S, Reid DM, Asamaphan P, Hardison SE, Dambuza IM, Valsecchi I, Kerscher B. Recognition of DHN-melanin by a C-type lectin receptor is required for immunity to aspergillus. Nature. 2018;555(7696):382–386. doi:10.1038/nature25974.
- Nagpal R, Neth BJ, Wang S, Mishra SP, Craft S, Yadav H. Gut mycobiome and its interaction with diet, gut bacteria and alzheimer’s disease markers in subjects with mild cognitive impairment: a pilot study. EBioMedicine. 2020;59:102950. doi:10.1016/j.ebiom.2020.102950.
- Lai GC, Tan TG, Pavelka N. The mammalian mycobiome: a complex system in a dynamic relationship with the host. Wiley Interdiscip Rev Syst Biol Med. 2018;11.
- Witherden EA, Shoaie S, Hall RA, Moyes DL. The human mucosal mycobiome and fungal community interactions. J Fungi. 2017;3(4):56. doi:10.3390/jof3040056.
- Calvo AM, Wilson RA, Bok JW, Keller NP. Relationship between secondary metabolism and fungal development. Microbiol Mol Biol Rev MMBR. 2002 table of contents;66(3):447–459. doi:10.1128/MMBR.66.3.447-459.2002.
- Keller NP. Fungal secondary metabolism: regulation, function and drug discovery. Nat Rev Microbiol. 2019;17(3):167–180. doi:10.1038/s41579-018-0121-1.
- Guinan J, Wang S, Hazbun TR, Yadav H, Thangamani S. Antibiotic-induced decreases in the levels of microbial-derived short-chain fatty acids correlate with increased gastrointestinal colonization of Candida albicans. Sci Rep. 2019;9(1):8872. doi:10.1038/s41598-019-45467-7.
- Kennedy MJ, Volz PA. Ecology of Candida albicans gut colonization: inhibition of Candida adhesion, colonization, and dissemination from the gastrointestinal tract by bacterial antagonism. Infect Immun. 1985;49(3):654–663. doi:10.1128/iai.49.3.654-663.1985.
- Kaur J, Pethani BP, Kumar S, Kim M, Sunna A, Kautto L, Penesyan A, Paulsen IT, Nevalainen H. Pseudomonas aeruginosa inhibits the growth of Scedosporium aurantiacum, an opportunistic fungal pathogen isolated from the lungs of cystic fibrosis patients. Front Microbiol. 2015;6:866. doi:10.3389/fmicb.2015.00866.
- Briard B, Heddergott C, Latgé JP. Volatile compounds emitted by pseudomonas aeruginosa stimulate growth of the fungal pathogen aspergillus fumigatus. mBio. 2016;7(2). doi:10.1128/mBio.00219-16.
- Hogan DA, Kolter R. Pseudomonas - candida interactions: an ecological role for virulence factors. Science. 2002;296(5576):2229–2232. doi:10.1126/science.1070784.
- Klotz SA, Chasin BS, Powell B, Gaur NK, Lipke PN. Polymicrobial bloodstream infections involving Candida species: analysis of patients and review of the literature. Diagn Microbiol Infect Dis. 2007;59(4):401–406. doi:10.1016/j.diagmicrobio.2007.07.001.
- Gupta N, Haque A, Mukhopadhyay G, Narayan RP, Prasad R. Interactions between bacteria and Candida in the burn wound. Burns J Int Soc Burn Inj. 2005;31(3):375–378. doi:10.1016/j.burns.2004.11.012.
- Harriott MM, Noverr MC. Candida albicans and Staphylococcus aureus form polymicrobial biofilms: effects on antimicrobial resistance. Antimicrob Agents Chemother. 2009;53(9):3914–3922. doi:10.1128/AAC.00657-09.
- Allison DL, Scheres N, Willems HME, Bode CS, Krom BP, Shirtliff ME. The host immune system facilitates disseminated Staphylococcus aureus disease due to phagocytic attraction to candida albicans during coinfection: a case of bait and switch. Infect Immun. 2019;87(11):e00137–19. doi:10.1128/IAI.00137-19.
- Parada Venegas D, De la Fuente MK, Landskron G, González MJ, Quera R, Dijkstra G, Harmsen HJ, Faber KN, Hermoso MA. Short Chain Fatty Acids (SCFAs)-mediated gut epithelial and immune regulation and its relevance for inflammatory bowel diseases. Front Immunol. 2019;10.
- Bhaskaran N, Quigley C, Paw C, Butala S, Schneider E, Pandiyan P. Role of short chain fatty acids in controlling tregs and immunopathology during mucosal infection. Front Microbiol. 2018;9:1995. doi:10.3389/fmicb.2018.01995.
- Ciarlo E, Heinonen T, Herderschee J, Fenwick C, Mombelli M, Le Roy D, Roger T. Impact of the microbial derived short chain fatty acid propionate on host susceptibility to bacterial and fungal infections in vivo. Sci Rep. 2016;6(1):37944. doi:10.1038/srep37944.
- Liu P, Wang Y, Yang G, Zhang Q, Meng L, Xin Y, Jiang X. The role of short-chain fatty acids in intestinal barrier function, inflammation, oxidative stress, and colonic carcinogenesis. Pharmacol Res. 2021;165:105420. doi:10.1016/j.phrs.2021.105420.
- Yoo JY, Groer M, Dutra SVO, Sarkar A, McSkimming DI. Gut microbiota and immune system interactions. Microorganisms. 2020;8(10):1587. doi:10.3390/microorganisms8101587.
- Fellows R, Varga-Weisz P. Chromatin dynamics and histone modifications in intestinal microbiota-host crosstalk. Mol Metab. 2020;38:100925. doi:10.1016/j.molmet.2019.12.005.
- Baltierra-Trejo E, Sánchez-Yáñez JM, Buenrostro-Delgado O, Márquez-Benavides L. Production of short-chain fatty acids from the biodegradation of wheat straw lignin by aspergillus fumigatus. Bioresour Technol. 2015;196:418–425. doi:10.1016/j.biortech.2015.07.105.
- Cottier F, Tan ASM, Yurieva M, Liao W, Lum J, Poidinger M, Zolezzi F, Pavelka N. The transcriptional response of candida albicans to weak organic acids, carbon source, and MIG1 inactivation unveils a role for HGT16 in mediating the fungistatic effect of acetic acid. G3 Bethesda Md. 2017;7(11):3597–3604. doi:10.1534/g3.117.300238.
- Noverr MC, Huffnagle GB. Regulation of candida albicans morphogenesis by fatty acid metabolites. Infect Immun. 2004;72(11):6206–6210. doi:10.1128/IAI.72.11.6206-6210.2004.
- García C, Tebbji F, Daigneault M, Liu -N-N, Köhler JR, Allen-Vercoe E, Sellam A. The human gut microbial metabolome modulates fungal growth via the TOR signaling pathway. mSphere. 2017;2(6):e00555–17. doi:10.1128/mSphere.00555-17.
- Nguyen LN, Lopes LCL, Cordero RJB, Nosanchuk JD. Sodium butyrate inhibits pathogenic yeast growth and enhances the functions of macrophages. J Antimicrob Chemother. 2011;66(11):2573–2580. doi:10.1093/jac/dkr358.
- Goyal S, Ramawat KG, Mérillon J-M. Different shades of fungal metabolites: an overview. In: Fungal metabolites. Springer International Publishing; 2017. 1–29. doi:10.1007/978-3-319-25001-4_34.
- Deveau A, Bonito G, Uehling J, Paoletti M, Becker M, Bindschedler S, Hacquard S, Hervé V, Labbé J, Lastovetsky OA, et al. Bacterial-fungal interactions: ecology, mechanisms and challenges. FEMS Microbiol Rev. 2018;42(3):335–352. doi:10.1093/femsre/fuy008.
- Keller NP, Turner G, Bennett JW. Fungal secondary metabolism — from biochemistry to genomics. Nat Rev Microbiol. 2005;3(12):937–947. doi:10.1038/nrmicro1286.
- Fox EM, Howlett BJ. Secondary metabolism: regulation and role in fungal biology. Curr Opin Microbiol. 2008;11(6):481–487. doi:10.1016/j.mib.2008.10.007.
- Pusztahelyi T, Holb IJ, Pãcsi I. Secondary metabolites in fungus-plant interactions. Front Plant Sci. 2015;6:573. doi:10.3389/fpls.2015.00573.
- Thirumurugan D, Cholarajan A, Raja SSS, Vijayakumar R. An introductory chapter: secondary metabolites. In: Secondary metabolites - sources and applications. InTech; 2018. doi:10.5772/intechopen.79766
- Zhang Y-Q, Brock M, Keller NP. Connection of propionyl-CoA metabolism to polyketide biosynthesis in Aspergillus nidulans. Genetics. 2004;168(2):785–794. doi:10.1534/genetics.104.027540.
- Oide S, Moeder W, Krasnoff S, Gibson D, Haas H, Yoshioka K, Turgeon BG. NPS6, encoding a nonribosomal peptide synthetase involved in siderophore-mediated iron metabolism, is a conserved virulence determinant of plant pathogenic ascomycetes. Plant Cell. 2006;18(10):2836–2853. doi:10.1105/tpc.106.045633.
- Schmidt-Dannert C. Biosynthesis of terpenoid natural products in fungi. Cham: Springer; 2014. 19–61. doi:10.1007/10_2014_283.
- Metzger U, Schall C, Zocher G, Unsöld I, Stec E, Li S-M, Heide L, Stehle T. The structure of dimethylallyl tryptophan synthase reveals a common architecture of aromatic prenyltransferases in fungi and bacteria. Proc Natl Acad Sci U S A. 2009;106(34):14309–14314. doi:10.1073/pnas.0904897106.
- Steffan N, Unsöld IA, Li SM. Chemoenzymatic synthesis of prenylated indole derivatives by using a 4-dimethylallyltryptophan synthase from aspergillus fumigatus. ChemBioChem. 2007;8(11):1298–1307. doi:10.1002/cbic.200700107.
- de Groot AN, van Dongen PW, Vree TB, Hekster YA, van Roosmalen JEA. Current status and review of clinical pharmacology and therapeutic use compared with other oxytocics in obstetrics and gynaecology. Drugs. 1998;56(4):523–535. doi:10.2165/00003495-199856040-00002.
- Wong CGT, Bottiglieri T, Iii OCS. Neurological disease. Ann Neurol. 2003;65(S6):3–12. doi:10.1002/ana.10696.
- Jo S, Yarishkin O, Hwang YJ, Chun YE, Park M, Woo DH, Bae JY, Kim T, Lee J, Chun H, et al. GABA from reactive astrocytes impairs memory in mouse models of alzheimer’s disease. Nat Med. 2014;20(8):886–896. doi:10.1038/nm.3639.
- Flajs D, Peraica M. Toxicological properties of citrinin. doi:10.2478/10004-1254-60-2009-1992.
- Kumar A, Asthana M, Gupta A, Nigam D, Mahajan S. Secondary metabolism and antimicrobial metabolites of penicillium. In: New and future developments in microbial biotechnology and bioengineering. Elsevier; 2018. 47–68. doi:10.1016/b978-0-444-63501-3.00003-x.
- Tammer B, Lehmann I, Nieber K, Altenburger R. Combined effects of mycotoxin mixtures on human T cell function. Toxicol Lett. 2007;170(2):124–133. doi:10.1016/j.toxlet.2007.02.012.
- Chen CC, Chan WH. Inhibition of citrinin-induced apoptotic biochemical signaling in human hepatoma G2 cells by resveratrol. Int J Mol Sci. 2009;10(8):3338–3357. doi:10.3390/ijms10083338.
- Ostry V, Malir F, Ruprich J. Producers and important dietary sources of ochratoxin A and citrinin. Toxins. 2013;5(9):1574–1586. doi:10.3390/toxins5091574.
- Groopman JD, Cain LG, Kensler TW, Harris CC. CRC critical reviews in toxicology aflatoxin exposure in human populations: measurements and relationship to cancer. 2008. doi:10.3109/10408448809014902.
- Deger GE. Aflatoxin: human colon carcinogenesis?. Ann Intern Med. 1976;85(2):204–205. doi:10.7326/0003-4819-85-2-204.
- Aguilar F, Hussain SP, Cerutti P. Aflatoxin B1 induces the transversion of G-->T in codon 249 of the p53 tumor suppressor gene in human hepatocytes. Proc Natl Acad Sci U S A. 1993;90(18):8586–8590. doi:10.1073/pnas.90.18.8586.
- Buening MK, Chang RL, Huang MT, Fortner JG, Wood AW, Conney AH. Activation and inhibition of benzo(a)pyrene and aflatoxin B1 metabolism in human liver microsomes by naturally occurring flavonoids. Cancer Res. 1981;41:67–72.
- Samson RA, Noonim P, Meijer M, Houbraken J, Frisvad JC, Varga J. Diagnostic tools to identify black aspergilli. Stud Mycol. 2007;59:129–145. doi:10.3114/sim.2007.59.13.
- Siddiqui MS, Thodey K, Trenchard I, Smolke CD. Advancing secondary metabolite biosynthesis in yeast with synthetic biology tools. FEMS Yeast Res. 2012;12(2):144–170. doi:10.1111/j.1567-1364.2011.00774.x.
- Song YC, Li H, Ye YH, Shan CY, Yang YM, Tan RX. Endophytic naphthopyrone metabolites are co-inhibitors of xanthine oxidase, SW1116 cell and some microbial growths. FEMS Microbiol Lett. 2004;241(1):67–72. doi:10.1016/j.femsle.2004.10.005.
- Koyama K, Ominato K, Natori S, Tashiro T, Tsuruo T. Cytotoxicity and antitumor activities of fungal bis (naphtho-γ-pyrone) derivatives. J Pharmacobiodyn. 1988;11(9):630–635. doi:10.1248/bpb1978.11.630.
- Kim S-K, Dewapriya P. Anticancer potentials of marine-derived fungal metabolites. In: Marine microbiology. John Wiley & Sons, Ltd; 2013. 237–245. doi:10.1002/9783527665259.ch14.
- Traven A, Naderer T. Central metabolic interactions of immune cells and microbes: prospects for defeating infections. EMBO Rep. 2019;20.
- Bouslimi A, Ouannes Z, Golli EE, Bouaziz C, Hassen W, Bacha H. Cytotoxicity and oxidative damage in kidney cells exposed to the mycotoxins ochratoxin a and citrinin: individual and combined effects. Toxicol Mech Methods. 2008;18(4):341–349. doi:10.1080/15376510701556682.
- Hetherington AC, Raistrick H. Studies in the biochemistry of micro-organisms. part XIV.—On the production and chemical constitution of a new yellow colouring mater, citrinin, produced from glucose by penicillium. Philos Trans R Soc Lond Ser B Contain Pap Biol Character. 1931;220:269–295.
- Zain ME. Impact of mycotoxins on humans and animals. J Saudi Chem Soc. 2011;15(2):129–144. doi:10.1016/j.jscs.2010.06.006.
- Kong HH, Morris A. The emerging importance and challenges of the human mycobiome. Virulence. 2017;8(3):310–312. doi:10.1080/21505594.2017.1279780.
- Joyce AR, Palsson B. The model organism as a system: integrating ‘omics’ data sets. Nat Rev Mol Cell Biol. 2006;7(3):198–210. doi:10.1038/nrm1857.
- Gehlenborg N, O’Donoghue SI, Baliga NS, Goesmann A, Hibbs MA, Kitano H, Kohlbacher O, Neuweger H, Schneider R, Tenenbaum D. Visualization of omics data for systems biology. Nat Methods. 2010;7(S3):S56–S68. doi:10.1038/nmeth.1436.
- Berger B, Peng J, Singh M. Computational solutions for omics data. Nat Rev Genet. 2013;14(5):333–346. doi:10.1038/nrg3433.
- Vogel C, Marcotte EM. Insights into the regulation of protein abundance from proteomic and transcriptomic analyses. Nat Rev Genet. 2012;13(4):227–232. doi:10.1038/nrg3185.
- de Bekker C, Smith PB, Patterson AD, Hughes DP, Jacobsen ID. Metabolomics reveals the heterogeneous secretome of two entomopathogenic fungi to ex vivo cultured insect tissues. PLoS ONE. 2013;8(8):e70609. doi:10.1371/journal.pone.0070609.
- Palsson B. Metabolic systems biology. FEBS Lett. 2009;583(24):3900–3904. doi:10.1016/j.febslet.2009.09.031.
- Systems biology - bernhard palsson -. Google Books.
- Shoaie S, Nielsen J. Elucidating the interactions between the human gut microbiota and its host through metabolic modeling. Front Genet. 2014;5. doi:10.3389/fgene.2014.00086.
- Mardinoglu A, Gatto F, Nielsen J. Genome-scale modeling of human metabolism - a systems biology approach. Biotechnol J. 2013;8(9):985–996. doi:10.1002/biot.201200275.
- Magnúsdóttir S, Heinken A, Kutt L, Ravcheev DA, Bauer E, Noronha A, Greenhalgh K, Jäger C, Baginska J, Wilmes P. Generation of genome-scale metabolic reconstructions for 773 members of the human gut microbiota. Nat Biotechnol. 2017;35(1):81–89. doi:10.1038/nbt.3703.
- Noronha A, Modamio J, Jarosz Y, Guerard E, Sompairac N, Preciat G, Daníelsdóttir AD, Krecke M, Merten D, Haraldsdóttir HS. The virtual metabolic human database: integrating human and gut microbiome metabolism with nutrition and disease. Nucleic Acids Res. 2019;47(D1):D614–D624. doi:10.1093/nar/gky992.
- Ghaffari P, Mardinoglu A, Asplund A, Shoaie S, Kampf C, Uhlen M, Nielsen J. Identifying anti-growth factors for human cancer cell lines through genome-scale metabolic modeling. Sci Rep. 2015;5(1):8183. doi:10.1038/srep08183.
- Martins-Santana L, Nora LC, Sanches-Medeiros A, Lovate GL, Cassiano MHA, Silva-Rocha R. Systems and synthetic biology approaches to engineer fungi for fine chemical production. Front Bioeng Biotechnol. 2018;6:117. doi:10.3389/fbioe.2018.00117.
- Lu H, Li F, Sánchez BJ, Zhu Z, Li G, Domenzain I, Marcišauskas S, Anton PM, Lappa D, Lieven C. A consensus S. cerevisiae metabolic model Yeast8 and its ecosystem for comprehensively probing cellular metabolism. Nat Commun. 2019;10(1):3586. doi:10.1038/s41467-019-11581-3.
- Caspeta L, Shoaie S, Agren R, Nookaew I, Nielsen J. Genome-scale metabolic reconstructions of Pichia stipitis and Pichia pastoris and in silico evaluation of their potentials. BMC Syst Biol. 2012;6(1):24. doi:10.1186/1752-0509-6-24.
- Sohn SB, Kim TY, Lee JH, Lee SY. Genome-scale metabolic model of the fission yeast Schizosaccharomyces pombe and the reconciliation of in silico/in vivo mutant growth. BMC Syst Biol. 2012;6(1):49. doi:10.1186/1752-0509-6-49.
- Xu N, Liu L, Zou W, Liu J, Hua Q, Chen J. Reconstruction and analysis of the genome-scale metabolic network of Candida glabrata. Mol Biosyst. 2013;9(2):205–216. doi:10.1039/C2MB25311A.
- Chung BK, Selvarasu S, Camattari A, Ryu J, Lee H, Ahn J, Lee H, Lee D-Y. Genome-scale metabolic reconstruction and in silico analysis of methylotrophic yeast Pichia pastoris for strain improvement. Microb Cell Factories. 2010;9(1):50. doi:10.1186/1475-2859-9-50.
- Brochado AR, Matos C, Møller BL, Hansen J, Mortensen UH, Patil KR. Improved vanillin production in baker’s yeast through in silico design. Microb Cell Factories. 2010;9(1):84. doi:10.1186/1475-2859-9-84.
- Mishra P, Lee N-R, Lakshmanan M, Kim M, Kim B-G, Lee D-Y. Genome-scale model-driven strain design for dicarboxylic acid production in Yarrowia lipolytica. BMC Syst Biol. 2018;12(S2):12. doi:10.1186/s12918-018-0542-5.
- Frezza C, Zheng L, Folger O, Rajagopalan KN, MacKenzie ED, Jerby L, Micaroni M, Chaneton B, Adam J, Hedley A. Haem oxygenase is synthetically lethal with the tumour suppressor fumarate hydratase. Nature. 2011;477(7363):225–228. doi:10.1038/nature10363.
- Lewis NE, Schramm G, Bordbar A, Schellenberger J, Andersen MP, Cheng JK, Patel N, Yee A, Lewis RA, Eils R. Large-scale in silico modeling of metabolic interactions between cell types in the human brain. Nat Biotechnol. 2010;28(12):1279–1285. doi:10.1038/nbt.1711.
- Agren R, Mardinoglu A, Asplund A, Kampf C, Uhlen M, Nielsen J. Identification of anticancer drugs for hepatocellular carcinoma through personalized genome-scale metabolic modeling. Mol Syst Biol. 2014;10(3):721. doi:10.1002/msb.145122.
- Kumar M, Ji B, Babaei P, Das P, Lappa D, Ramakrishnan G, Fox TE, Haque R, Petri WA, Bäckhed F. Gut microbiota dysbiosis is associated with malnutrition and reduced plasma amino acid levels: lessons from genome-scale metabolic modeling. Metab Eng. 2018;49:128–142. doi:10.1016/j.ymben.2018.07.018.
- Granata I, Troiano E, Sangiovanni M, Guarracino MR. Integration of transcriptomic data in a genome-scale metabolic model to investigate the link between obesity and breast cancer. BMC Bioinform. 2019;20(S4):162. doi:10.1186/s12859-019-2685-9.
- Bauer E, Thiele I. From metagenomic data to personalized in silico microbiotas: predicting dietary supplements for Crohn’s disease. Npj Syst Biol Appl. 2018;4(1):1–9. doi:10.1038/s41540-018-0063-2.
- Das P, Babaei P, Nielsen J. Metagenomic analysis of microbe-mediated vitamin metabolism in the human gut microbiome. BMC Genomics. 2019;20(1):208. doi:10.1186/s12864-019-5591-7.
- Baldini F, Hertel J, Sandt E, Thinnes CC, Neuberger-Castillo L, Pavelka L, Betsou F, Krüger R, Thiele I. Parkinson’s disease-associated alterations of the gut microbiome predict disease-relevant changes in metabolic functions. BMC Biol. 2020;18(1):62. doi:10.1186/s12915-020-00775-7.
- Miraglia F, Colla EM. Parkinson’s disease and molecular mimicry. Cells. 2019;8(3):E222. doi:10.3390/cells8030222.
- Nuzum ND, Loughman A, Szymlek-Gay EA, Hendy A, Teo WP, Macpherson H. Gut microbiota differences between healthy older adults and individuals with Parkinson’s disease: a systematic review. Neurosci Biobehav Rev. 2020;112:227–241. doi:10.1016/j.neubiorev.2020.02.003.
- Rosario D, Bidkhori G, Lee S, Bedarf J, Hildebrand F, Le Chatelier E, Uhlen M, Ehrlich SD, Proctor G, Wüllner U, Mardinoglu A. Systematic analysis of gut microbiome reveals the role of bacterial folate and homocysteine metabolism in Parkinson’s disease. Cell Rep. 2021;34(9):108807. doi:10.1016/j.celrep.2021.108807.
- MahmoudianDehkordi S, Arnold M, Nho K, Ahmad S, Jia W, Xie G, Louie G, Kueider‐Paisley A, Moseley MA, Thompson JW. Altered bile acid profile associates with cognitive impairment in Alzheimer’s disease-An emerging role for gut microbiome. Alzheimers Dement J Alzheimers Assoc. 2019;15(1):76–92. doi:10.1016/j.jalz.2018.07.217.
- Baloni P, Funk CC, Yan J, Yurkovich JT, Kueider-Paisley A, Nho K, Heinken A, Jia W, Mahmoudiandehkordi S, Louie G, Saykin AJ. Identifying differences in bile acid pathways for cholesterol clearance in Alzheimer’s disease using metabolic networks of human brain regions. 2019, p. 782987. doi:10.1101/782987.
- Zomorrodi AR, Maranas CD. OptCom: a multi-level optimization framework for the metabolic modeling and analysis of microbial communities. PLoS Comput Biol. 2012;8(2):e1002363. doi:10.1371/journal.pcbi.1002363.
- Shoaie S, Karlsson F, Mardinoglu A, Nookaew I, Bordel S, Nielsen J. Understanding the interactions between bacteria in the human gut through metabolic modeling. Sci Rep. 2013;3(1):1–10. doi:10.1038/srep02532.
- Shoaie S, Ghaffari P, Kovatcheva-Datchary P, Mardinoglu A, Sen P, Pujos-Guillot E, De Wouters T, Juste C, Rizkalla S, Chilloux J, Hoyles L. Quantifying diet-induced metabolic changes of the human gut microbiome. Cell Metab. 2015;22(2):320–331. doi:10.1016/j.cmet.2015.07.001.
- Román E, Coman I, Prieto D, Alonso-Monge R, Pla J. Implementation of a CRISPR-based system for gene regulation in candida albicans. mSphere. 2019;4(1):e00001–19. doi:10.1128/mSphere.00001-19.
- Gonzalez-Angulo AM, Hennessy BTJ, Mills GB. Future of personalized medicine in oncology: a systems biology approach. J Clin Oncol. 2010;28(16):2777–2783. doi:10.1200/JCO.2009.27.0777.
- Norsigian CJ, Fang X, Seif Y, Monk JM, Palsson BO. A workflow for generating multi-strain genome-scale metabolic models of prokaryotes. Nat Protoc. 2020;15(1):1–14. doi:10.1038/s41596-019-0254-3.
- Bordbar A, Monk JM, King ZA, Palsson BO. Constraint-based models predict metabolic and associated cellular functions. Nat Rev Genet. 2014;15(2):107–120. doi:10.1038/nrg3643.
- Lieven C, Beber ME, Olivier BG, Bergmann FT, Ataman M, Babaei P, Bartell JA, Blank LM, Chauhan S, Correia K, Diener C. MEMOTE for standardized genome-scale metabolic model testing. Nat Biotechnol. 2020;38(3):272–276. doi:10.1038/s41587-020-0446-y.
- Machado D, Andrejev S, Tramontano M, Patil KR. Fast automated reconstruction of genome-scale metabolic models for microbial species and communities. Nucleic Acids Res. 2018;46(15):7542–7553. doi:10.1093/nar/gky537.
- Agren R, Liu L, Shoaie S, Vongsangnak W, Nookaew I, Nielsen J. The RAVEN toolbox and its use for generating a genome-scale metabolic model for Penicillium chrysogenum. PLoS Comput Biol. 2013;9(3):e1002980. doi:10.1371/journal.pcbi.1002980.
- Mojica FJM, Díez-Villaseñor C, García-Martínez J, Soria E. Intervening sequences of regularly spaced prokaryotic repeats derive from foreign genetic elements. J Mol Evol. 2005;60(2):174–182. doi:10.1007/s00239-004-0046-3.
- Pourcel C, Salvignol G, Vergnaud G. CRISPR elements in Yersinia pestis acquire new repeats by preferential uptake of bacteriophage DNA, and provide additional tools for evolutionary studies. Microbiol Read Engl. 2005;151(3):653–663. doi:10.1099/mic.0.27437-0.
- Brouns SJJ, Jore MM, Lundgren M, Westra ER, Slijkhuis RJH, Snijders APL, Dickman MJ, Makarova KS, Koonin EV, van der Oost J. Small CRISPR RNAs guide antiviral defense in prokaryotes. Science. 2008;321(5891):960–964. doi:10.1126/science.1159689.