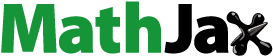
ABSTRACT
The antibiotic resistome is the collection of all antibiotic resistance genes (ARGs) present in an individual. Whether an individual’s susceptibility to infection and the eventual severity of coronavirus disease 2019 (COVID-19) is influenced by their respiratory tract antibiotic resistome is unknown. Additionally, whether a relationship exists between the respiratory tract and gut ARGs composition has not been fully explored. We recruited 66 patients with COVID-19 at three disease stages (admission, progression, and recovery) and conducted a metagenome sequencing analysis of 143 sputum and 97 fecal samples obtained from them. Respiratory tract, gut metagenomes, and peripheral blood mononuclear cell (PBMC) transcriptomes are analyzed to compare the gut and respiratory tract ARGs of intensive care unit (ICU) and non-ICU (nICU) patients and determine relationships between ARGs and immune response. Among the respiratory tract ARGs, we found that Aminoglycoside, Multidrug, and Vancomycin are increased in ICU patients compared with nICU patients. In the gut, we found that Multidrug, Vancomycin, and Fosmidomycin were increased in ICU patients. We discovered that the relative abundances of Multidrug were significantly correlated with clinical indices, and there was a significantly positive correlation between ARGs and microbiota in the respiratory tract and gut. We found that immune-related pathways in PBMC were enhanced, and they were correlated with Multidrug, Vancomycin, and Tetracycline ARGs. Based on the ARG types, we built a respiratory tract-gut ARG combined random-forest classifier to distinguish ICU COVID-19 patients from nICU patients with an AUC of 0.969. Cumulatively, our findings provide some of the first insights into the dynamic alterations of respiratory tract and gut antibiotic resistome in the progression of COVID-19 and disease severity. They also provide a better understanding of how this disease affects different cohorts of patients. As such, these findings should contribute to better diagnosis and treatment scenarios.
Introduction
The global rise in antibiotic resistance poses a major health threat and is responsible for more than 700 000 deaths annually.Citation1 The antibiotic resistance crisis has increased the challenges of antibiotic treatment for bacterial infections,Citation2 largely due to the selection of antibiotic resistance genes (ARGs) collection, in the human gut and respiratory tract. The human gut and respiratory tract are considered important reservoir of ARGs.Citation3,Citation4 A few current studies have explored the function of antibiotic resistome in the development of infection, suggesting possible relationships between the gut ARGs and infection.Citation5–8 The composition of gut ARGs showed differences between patients with COVID-19 and healthy cohorts.Citation9,Citation10 As a major portal of entry for SARS-CoV-2, the human upper respiratory tract is inhabited by an abundant and complex microbiome.Citation11 However, the composition of respiratory tract ARGs and their variation in patients with COVID-19 is largely unknown. In addition, little is known about the association between the respiratory tract ARGs and the gut ARGs, which is one of the most crucial questions regarding ARGs’ contribution to the health of patients with COVID-19.
Given that prior evidence suggested a close link between human gut and respiratory tract microbiome and COVID-19 disease severity,Citation12 in the current study, we investigated the dynamic alterations in the composition of respiratory tract ARGs of patients with COVID-19 and its correlation with ARG composition in the gut. To this end, we recruited 66 COVID-19 patients at three stages of the disease (i.e., admission, progression, and recovery) and conducted metagenome sequencing in sputum and fecal samples. A total of 143 respiratory tract metagenomes, 97 gut metagenomes, and 66 peripheral blood mononuclear cell (PBMC) transcriptomes were sequenced and used to systematically investigate the similarities and differences between the gut and respiratory tract antibiotic resistome in intensive care unit (ICU) and non-ICU (nICU) patients at admission, progression, and recovery stages. Our findings reveal the dynamic alterations in the respiratory tract and gut ARGs during COVID-19 infection and explore their relationship between host immune response and microbiota. The findings of this study provide insights into the role changes in the respiratory tract and gut antibiotic resistome and immune system play at COVID-19 different disease severity and progression stages.
Results
Composition of the respiratory tract and gut antibiotic resistome in patients with COVID-19
To dissect the changes in the ARG composition of COVID-19 patients, we performed metagenome sequencing on 143 sputum and 97 fecal samples from 20 ICU and 46 nICU patients and 16 healthy people. The median ages of nICU patients, ICU patients, and healthy people were 51, 70, and 49 years, respectively; 39% and 100% of the patients in the nICU and ICU groups, respectively, had underlying comorbidities (). The sputum samples were collected from patients at three disease progression stages (admission, progression, and recovery), whereas the fecal samples were collected at two stages (progression and recovery) for the study (). We detected a total of 24 ARG types and 1244 ARG subtypes in the total sample population. After excluding unclassified ARG types or subtypes and 10% prevalence filtering, a total of 17 ARG types and 193 ARG subtypes were validated and subsequently analyzed in the current study. The top three most abundant ARG types identified in the respiratory tract are active against Macrolide-lincosamide-streptogramin (MLS), Beta-lactam, and Tetracycline and are present in both healthy individuals and patients with COVID-19 (). We found that among the top 10 most abundant ARG types in the respiratory tract, the relative abundances of MLS, Vancomycin, Multidrug, and Aminoglycoside increased significantly in patients with COVID-19 compared with healthy cohorts (p< .05) (Figure S1). ICU patients had higher relative abundances of Multidrug, Vancomycin and Aminoglycoside ARGs than nICU patients (). In the gut samples, the top three most abundant ARG types observed are Multidrug, MLS, and Tetracycline. We observed that among the top 10 most abundant ARG types, the relative abundances of Multidrug, Tetracycline, Aminoglycoside, Fosmidomycin and Vancomycin ARGs increased significantly in patients with COVID-19 compared with healthy cohorts (p < .05) (Figure S1). ICU patients had higher relative abundances of Multidrug, Vancomycin and Fosmidomycin ARGs than nICU patients ().
Figure 1. Composition of the respiratory tract and gut antibiotic resistome in patients with COVID-19.

Table 1. Basic demographics of the patients used in this study.
To investigate the diversity of respiratory tract and gut antibiotic resistome among patients with COVID-19, we calculated the Shannon index to measure the alpha diversity for each patient. We found that the Shannon index of the gut antibiotic resistome is much higher than that of the respiratory tract (p < .001) (). We also found that the beta-diversity was significantly higher in the gut antibiotic resistome than respiratory tract (p < .001) (). Furthermore, when we compared the beta-diversity of ICU and nICU patients, we found that there were significant differences between the ICU and the nICU patients in both the respiratory tract and gut antibiotic resistome (p < .01) ().
Dynamic changes in respiratory tract antibiotic resistome and its association with disease severity
We next examined the relative abundances of ARGs in sputum samples of patients at the admission, progression, and recovery stages to investigate the potential dynamic alterations in the respiratory tract antibiotic resistome over the time course of the disease. In the ICU patients, the total relative abundance of ARGs was significantly increased in the progression stage compared to the admission and recovery stages (p < .01) (Figure S2). Moreover, the relative abundances of MLS, Multidrug, Vancomycin, and Aminoglycoside ARGs were higher in the progression-stage samples compared to samples collected at the admission and recovery stages (), consistent with our previous findings (). In nICU patients, the total relative abundance of ARGs was significantly increased in the progression stage compared with the recovery stage (p < .05) (Figure S2). Further comparison of Shannon indices between ICU and nICU patients at different stages indicated that there was a significant difference in antibiotic resistome alpha diversity between ICU and nICU patients at the progression stage (p < .05) ().
Figure 2. Dynamic alterations in respiratory tract antibiotic resistome and its association with disease severity.

To further explore the differences in respiratory tract antibiotic resistome between ICU and nICU patients at different stages of disease progression, we performed multiple linear model regression analyses that included clinical information about patient ICU status, gender, antibiotic usage, age, and smoking history. At the admission stage, Kasugamycin, Vancomycin, and Aminoglycoside ARGs were significantly positively correlated with ICU status. In contrast, Rifamycin ARGs were negatively correlated with ICU status. Besides, at the admission stage, we found that Rifamycin and Aminoglycoside were positively correlated with antibiotic therapy (p < .05) (). At the progression stage, Multidrug, Bacitracin, Quinolone, Aminoglycoside, Chloramphenicol, Fosfomycin, Sulfonamide, Kasugamycin, Fosmidomycin, and Vancomycin ARGs were significantly positively correlated with ICU status (p < .05) (), and we observed that Multidrug and Bleomycin ARGs were positively associated with antibiotic therapy (p < .05) (). At the recovery stage, Sulfonamide, Polymyxin, Fosmidomycin, and Multidrug ARGs were significantly positively correlated with ICU status. In addition, we found that at the recovery stage, the relative abundances of Multidrug and Chloramphenicol ARGs were positively correlated with antibiotic therapy (p < .05) (). Based on these results, we found that Kasugamycin, Vancomycin, and Aminoglycoside ARGs were significantly positively correlated with ICU status in the admission and progression stages. Besides, the relative abundances of Sulfonamide, Fosmidomycin, and Multidrug ARGs were significantly positively correlated with ICU status in the progression and recovery stages. In addition, the results showed that patients’ gender, age, and smoking history did not have a significant influence on the abundance of respiratory tract antibiotic resistome ().
Next, to investigate the relationships between the ARG types at each stage, we performed a relative abundance correlation analysis (). Our results showed that MLS and Tetracycline, MLS and Aminoglycoside, Bacitracin, and Multidrug ARGs were significantly correlated with each other at all stages (p < .05) (Figure S2). Tetracycline ARGs were significantly positively correlated with Vancomycin ARGs both at the admission and progression stages (p < .05) (Figure S2). To determine the ARG subtypes involved in the observed changes, we compared the relative abundances of subtypes between ICU and nICU patients at the admission, progression, and recovery stages (). Among the Chloramphenicol types, the relative abundance of Chloramphenicol_cat increased considerably in ICU patients at the progression stage (p < .01). In the MLS types, the relative abundance of MLS_macA increased significantly in ICU patients at the recovery stage (p < .05). In the Multidrug types, the relative abundances of Multidrug_mdtB, Multidrug_mdtC, and Multidrug_mdtK were significantly increased in ICU patients at the progression stage (p < .05). In the Vancomycin types, the relative abundance of Vancomycin_vanS was significantly increased in ICU patients at the admission and progression stages (p < .05). Thus, the increased relative abundance of certain ARG types, such as Multidrug ARGs was related to alterations in many subtypes, whereas in Chloramphenicol, MLS, and Vancomycin ARGs only one subtype was responsible for the increase in the relative abundance of the types.
Dynamic changes in gut antibiotic resistome of patients with COVID-19 and its association with respiratory tract antibiotic resistome
To analyze the dynamic changes in the gut antibiotic resistome, we investigated the relative abundances of the ARG types in the gut during the progression and recovery stages. In ICU patients, the total relative abundance of ARGs was significantly increased in the progression stage compared to the recovery stage (p < .05) (Figure S3). Moreover, the relative abundances of Multidrug and Fosmidomycin ARGs were higher in the progression stage than in the recovery stage (). In nICU patients, no significant difference in the total relative abundance of ARGs was observed among samples collected at progression and recovery stages (Figure S3).
Figure 3. Dynamic alterations in gut antibiotic resistome of patients with COVID-19 and its association with respiratory tract antibiotic resistome.

To further understand the differences in gut antibiotic resistome between the ICU and nICU patients at different disease stages, we also performed a multiple linear model regression analysis that included information about patient ICU status, gender, antibiotic usage, age, and smoking history. In the progression-stage samples, we found that Multidrug, Bacitracin, Fosmidomycin, Polymyxin, Kasugamycin, Aminoglycoside, Vancomycin, and Fosfomycin ARGs were significantly positively correlated with ICU status (p < .05). At the progression stage, we also observed that Fosmidomycin, Beta-lactam, and Quinolone ARGs were positively correlated with antibiotic therapy (p < .05) (). In the recovery stage, Vancomycin, Aminoglycoside, and Multidrug ARGs were found to be significantly positively correlated with ICU status (p < .05). In addition, at the recovery stage, we observed that Beta-lactam and Chloramphenicol ARGs were positively correlated with antibiotic therapy (p < .05) (). Based on these findings, we concluded that Vancomycin, Multidrug, and Aminoglycoside ARGs were positively correlated with ICU status in both the progression and recovery stages, and Beta-lactam ARGs were positively correlated with antibiotic therapy in both the progression and recovery stages.
Next, we compared the relative abundance of ARG subtypes between ICU and nICU patients at the progression and recovery stages to determine which ARG subtype contributed the most to the alterations in the gut antibiotic resistome (). In the Multidrug type ARGs, the relative abundance of Multidrug_mdtN and Multidrug_mexE subtypes were significantly increased in ICU patients at the progression stage (p < .05). In the Aminoglycoside type, the relative abundance of Aminoglycoside_aph-3I was significantly increased in ICU patients at the progression stage (p < .05). In the Vancomycin type, the relative abundance of Vancomycin_vanY was significantly increased in ICU patients at the progression stage (p < .01). In the Fosmidomycin type, the relative abundance of Fosmidomycin_rosB and Fosmidomycin_rosA subtypes were significantly increased in ICU patients at the progression stage (p < .05). Based on these results, we suggest that the increased relative abundance of the Multidrug and Fosmidomycin ARGs could be attributed to multiple subtypes, whereas in ARG types, such as Vancomycin and Aminoglycoside, only one subtype was responsible for the increased relative abundance.
To investigate the relationship between the antibiotic resistome of the respiratory tract and the gut, we conducted a correlation analysis based on the relative abundances of each ARG type. A significant positive correlation was revealed between the relative abundances of Aminoglycoside, Kasugamycin and Tetracycline ARGs in the respiratory tract and the gut (). In addition, we found that the Multidrug type was significantly increased in the ICU patients in both the gut and respiratory tract (). The relative abundance of Multidrug ARGs in the respiratory tract was significantly lower than that in the gut (). Based on the samples in which Multidrug ARGs were detected, a significant (p < .01) correlation was found between Multidrug ARGs in the respiratory tract and gut ().
Relationship between respiratory tract, gut antibiotic resistome composition, and clinical indices
We compared clinical indices in the ICU and nICU patients and found that the number of WBC was significantly higher in the ICU patients than the nICU patients (p < .05). In contrast, HCT levels were significantly higher in the nICU patients (p < .05) (). Both of these observations are consistent with results from previous studies.Citation13–15 The levels of IL-6 and IL-10 were higher in the ICU patients compared with nICU patients and are both higher compared with healthy people. However, the number of lymphocytes was higher in healthy people compared with patients with COVID-19, and nICU patients have a higher lymphocyte count compared with ICU patients (). We further conducted a Spearman correlation analysis between clinical indices and the relative abundances of ARG subtypes, to explore the relationships between the clinical indices, and respiratory tract and gut antibiotic resistome of patients with COVID-19 (). In the respiratory tract, we found that IL-6 was positively correlated with the subtypes of Multidrug ARGs (p < .05), and PCT level, WBC and Neutrophil numbers were significantly positively correlated with the relative abundance of ARG subtype MLS_macA (p < .01), which was increased in the ICU patients at the recovery stage (). However, the Hb and HCT levels were significantly negatively correlated with the abundance of Chloramphenicol_cat and subtypes of Multidrug ARGs (p < .05), whereas the abundance of Tetracycline_tetO was positively correlated with the Hb and HCT levels (p < .05). The IFN-γlevel was positively correlated with the abundance of Bacitracin_bcrA and Tetracycline_tetO subtypes (p < .05) (). In the gut, PCT level was significantly positively correlated with the abundance of ARG subtypes Multidrug_mexE and Vancomycin_vanY (p < .001), both of which are higher in ICU patients at the progression stage (). IL-6 level was positively correlated with the abundance of many subtypes of Multidrug ARGs (p < .05). Moreover, WBC and Neutrophil numbers were positively correlated with the abundance of Vancomycin_vanY (p < .001), whereas the Hb and HCT levels were negatively correlated with the abundance of ARG subtypes Aminoglycoside_aph3I and Vancomycin_vanY (p < .05).
Figure 4. Relationship between respiratory tract, gut antibiotic resistome composition, and clinical indices.

Co-occurrence patterns between disease-severity-related ARGs and microbial species in respiratory tract and gut
To investigate whether there was a co-occurrence pattern between the disease severity-related ARGs and microbiota in the respiratory tract and gut, we conducted a correlation analysis based on the relative abundance of ARG subtypes and microbiota composition at the genus and species level. In the respiratory tract, at genus level, we found that the relative abundance of Multidrug_mdtB ARGs was positively associated with Lautropia (). Genera associated with pathogenicity, like Lautropia, in conditions of immune exhaustion were predominant in patients with severe infection.Citation16 At the species level, we found that Multidrug_mdtB ARGs were positively associated with Klebsiella pneumoniae, which accounts for a higher proportion of hospital-acquired pneumonia (). Moreover, we found that subtypes of Multidrug ARGs were positively associated with Pseudomonas aeruginosa, which can cause infections in the blood or other parts of the body after surgery (). A prior study showed that patients with severe COVID-19 requiring ECMO had a very high rate of late-onset ventilator-associated pneumonia frequently caused by P. aeruginosa.Citation17 This observation is consistent with our findings that the relative abundance of Multidrug ARGs is associated with disease severity (). We also observed that subtypes of Aminoglycoside and Chloramphenicol_cat ARGs were positively correlated with Candida albicans, a main cause of invasive fungal infections in critical care settings (). A prior study suggested a high prevalence of systemic candidiasis in severe COVID-19-associated pneumonia patients,Citation18 which is consistent with our findings that the relative abundance of Aminoglycoside and Chloramphenicol_cat ARGs was higher in ICU patients and in the samples collected at the progression stage ().
Figure 5. Co-occurrence patterns between disease-severity-related ARGs and microbial species in respiratory tract and gut.

In the gut, at the genus level, we found that the relative abundance of Fosmidomycin_rosA, Vancomycin_vanT, and Multidrug_mexE ARGs were positively correlated with Escherichia, Citrobacter, and Pseudomonas (). At the species level, we note that the relative abundance of Fosmidomycin_rosA and Vancomycin_vanT ARGs was positively correlated with Pseudomonas putida, whereas the relative abundance of Multidrug_mexE ARGs was positively correlated with Pseudomonas aeruginosa. We also observed that the relative abundance of Fosmidomycin_rosA, Multidrug_mdtN, and Multidrug_mexE ARGs were positively associated with Escherichia coli (). Prior studies showed that E. coli was positively correlated with COVID-19 disease severity.Citation19 This observation is consistent with our finding that the relative abundances of Fosmidomycin_rosA, Multidrug_mdtN, and Multidrug_mexE ARGs were significantly increased in ICU patients at the progression stage in the gut (). Moreover, we found that at the species level, the relative abundance of Multidrug_mdtB ARGs was positively correlated with K. pneumoniae in both the respiratory tract and gut ().
Respiratory tract and gut antibiotic resistome features are associated with immune response in PBMC
RNA sequencing was performed on PBMC samples of blood from ICU and nICU patients with COVID-19 at the progression stage, and from 18 healthy individuals to explore the relationships between respiratory tract ARGs, gut ARGs, and immune response-related gene expression. To explore this relationship, we carried out a Spearman correlation analysis between the gene set enrichment analysis (GSEA) score of each pathway and relative abundance of ARGs. In the respiratory tract ARGs, we found that the relative abundances of Vancomycin_vanS and Multidrug_transporter types were positively correlated with “PTK6 promotes HIF1A stabilization” (Figure S4). Moreover, the defensin-related pathways, such as “Defensins,” “Beta defensins,” and “Antimicrobial peptides,” were found to be significantly correlated with the relative abundance of subtypes of Multidrug and Tetracycline ARGs. In the gut ARGs, the results showed that the relative abundance of Fosmidomycin_rosA subtype, which was found to be significantly increased in ICU patients (), was positively correlated with “Synthesis of (16–20)-hydroxyeicosatetraenoic acids (HETE)” (Figure S4). This is consistent with the observation that infection with SARS-CoV-2 causes the imbalance of metabolites of arachidonic acid (AA) such as 20-HETE.Citation20
Respiratory tract and gut antibiotic resistome could accurately classify the disease severity of patients with COVID-19
To identify the antibiotic resistance characteristics associated with COVID-19 disease severity in the respiratory tract and gut, unsupervised random forest classification analysis using a leave-one-out cross-validation method was performed at the types and subtypes levels. We identified antibiotic resistance classifiers distinguishing ICU patients from nICU patients based on the respiratory tract ARGs, gut ARGs, and combined respiratory tract-gut ARGs in patients with COVID-19. At the ARG types level, the random forest classifiers based on the respiratory tract, gut, and combined respiratory tract–gut ARGs achieved area under the receiver operating characteristic curve (AUC) values of 0.918, 0.956, and 0.969, respectively (). The random forest classifier also identified the top ARG types characteristics of ICU and nICU patients. For the respiratory tract classifier, Multidrug, Aminoglycoside and MLS types were the top three characteristic ARGs, which was in line with our finding that relative abundances of Multidrug, Aminoglycoside ARGs in the respiratory tract were higher in the ICU patients (). For the gut classifier, Vancomycin, Aminoglycoside, and Multidrug types were the top three characteristic ARGs, which was in line with our finding that relative abundances of Multidrug and Vancomycin ARGs in the gut were higher in the ICU patients (). For the combined respiratory tract-gut classifier, Aminoglycoside and Vancomycin ARGs in the gut and Multidrug ARGs in the respiratory tract were the top three characteristic ARGs between ICU and nICU patients ().
Figure 6. Respiratory tract and gut antibiotic resistance dynamics during COVID-19 progression and their diagnostic potential for disease severity.

At the ARG subtypes level, the random forest classifiers based on the respiratory tract, gut, and combined respiratory tract–gut ARGs achieved area under the receiver operating characteristic curve (AUC) values of 0.922, 0.958, and 0.965, respectively (). The random forest classifier identified the top characteristic ARG subtypes between the ICU and nICU patients. In the respiratory tract, Multidrug_mdtC, MLS_macB, and Multidrug_mdtG were the top three characteristic ARG subtypes, which was consistent with the finding that the relative abundance of Multidrug_mdtC subtype in the respiratory tract was significantly higher in the ICU patients at the progression stage (). In the gut, Multidrug_adeC, MLS_msrC, and Multidrug_mexF were the top three characteristic subtypes. In the combined respiratory tract–gut classifier, Multidrug_adeC, and Multidrug_transporter in the gut and MLS_mefA in the respiratory tract were the top three characteristic subtypes between ICU and nICU patients ().
Discussion
We have systematically examined the similarities and differences between respiratory tract and gut antibiotic resistome in patients with COVID-19 at different disease progression stages and with different disease severities in the ICU or not (nICU). Our data not only uncovered a shift of the respiratory tract and gut ARGs with the progression of COVID-19 but also demonstrated that relationships exist between ARG compositions and host immune response and microbiota in the respiratory tract and gut. The overuse and misuse of antibiotics has stimulated a more rapid emergence of ARGs in recent years.Citation21 In our study, almost all of the ICU patients received empirical antibiotics, whereas there were 10 patients who received antibiotics in nICU group. We found that there was no significant difference in total relative abundance of ARG types in the respiratory tract and gut of patients who received antibiotics and nICU patients that never received antibiotics (Figure S5). Moreover, the relative abundance of ARGs in the respiratory tract and gut was higher in patients who never received antibiotics in the nICU group compared with healthy individuals (Figure S5).
Although it is difficult to determine whether the ARG features are causally correlated with the COVID-19 disease severity and progression, our data permit reasonable speculation on this matter. For example, we found that the relative abundances of Vancomycin and Multidrug ARGs in the respiratory tract and gut were significantly increased in ICU patients compared with nICU patients, and were higher in the progression stages in ICU patients. Moreover, we found that the relative abundance of Vancomycin_vanS subtype was significantly increased in both respiratory tract and gut in the COVID-19 patients, and was higher in the ICU patients (Figure S6), consistent with the results from a prior study.Citation9 These results reveal a potential link between the relative abundance of Vancomycin and Multidrug ARGs, especially relative abundance of Vancomycin_vanS subtype with COVID-19 disease severity and progression. Vancomycin_vanS is a membrane-bound sensor histidine kinase that has been demonstrated to activate transcription of vancomycin-resistance genes.Citation22
To explore the potential mechanism between ARGs and COVID-19 disease severity, we performed examination on several relationships. Bacterial co-infections are identified in patients with COVID-19 and are significant reasons for morbidity and mortality.Citation23 Our study found that the relative abundance of Multidrug_mdtB subtype was positively correlated with K. pneumoniae in both the respiratory tract and gut. Moreover, we found that the relative abundance of Multidrug_mexE subtype was positively correlated with P. aeruginosa, which is characterized by intrinsic resistance to a variety of antimicrobial agents. This property results from the interplay between broadly specific drug efflux systems, including MexEF-OprN, and the low outer membrane permeability of P. aeruginosa.Citation24 These results were in line with our finding that the relative abundance of Multidrug_mexE and Multidrug_mdtB subtypes was higher in ICU patients at the progression stage in the gut and respiratory tract, respectively ().
Previous evidence showed that IL6 and PCT levels had a highly significant association with COVID-19 disease severity.Citation25 In our study, both were positively correlated with the relative abundance of Multidrug subtypes, which were found to be higher in the ICU patients both in the respiratory tract and gut. The increased immune response caused by viral infection may alter the respiratory tract microbiota, which can further regulate the local immune response.Citation26,Citation27 Taken together, these results provide a potential interpretation for the mechanism behind the ARGs and COVID-19 disease severity and progression. However, based on the current results, it is hard to unequivocally conclude whether higher ARGs, especially Vancomycin_vanS and Multidrug_mdtB abundances, caused changes in disease severity or whether the disease severity alterations caused higher ARGs abundances, especially Vancomycin_vanS and Multidrug_mdtB.
Our findings highlight that COVID-19 patients, especially those with severe disease, may be vulnerable to the accumulation of ARGs due to respiratory tract and gut dysbiosis,Citation12,Citation28 or an enhanced host immune response.Citation29 Respiratory tract dysbiosis in deceased COVID-19 patients characterized by enrichment of conditional fungi such as Candida sp., which will impose considerable comorbidity and aggravate the disease.Citation30,Citation31 Gut dysbiosis in COVID-19 patients is characterized by enrichment of pathogenic bacteria like Klebsiella sp., the most common carriers of ARGs,Citation32 which will result in bacterial coinfections. In addition, K. pneumoniae isolates obtained from COVID-19 patients have high antimicrobial resistance that accelerated the horizontal gene transfer via outer membrane vesicles, and increased the transfer of ARGs from pathogenic bacteria to commensal.Citation33,Citation34 The potential role of an immune balance is supported by the increased levels of inflammatory cytokines and their significant correlation with ARGs alteration. Our study found that Vancomycin_vanS in respiratory tract was positively correlated with “PTK6 promotes HIF1A stabilization” pathway, and HIF1A plays a key role in promoting SARS-CoV-2 infection and inducing pro-inflammatory responses to COVID-19.Citation35 In conclusion, our study suggests that both the overgrowth of pathogenic bacteria and an increased immune response to SARS-CoV-2 infection might play a role in ARG accumulation.
The present study has several strengths. First, we were able to profile the respiratory tract and gut antibiotic resistome configuration of patients with COVID-19 and to the best of our knowledge this has not been previously investigated. Second, while a previous study mainly focused on the relationship between the antibiotic resistome and COVID-19 severity, we also examined the correlation between antibiotic resistome and COVID-19 disease progression. Finally, we compared, for the first time, the respiratory tract and gut antibiotic resistome as prognostic biomarkers for the COVID-19 severity. We also recognize that our study had several limitations. First, this was a single-center study, and, therefore, any generalization of our findings requires validation in other countries and/or ethnicities. Second, our current results did not allow us to derive a detailed mechanism or direct causal relationship between specific aspects of the antibiotic resistome and disease severity. Therefore, a more mechanistic investigation should be undertaken in the future. The current work provides a clear foundation for such exploration.
In summary, the present study shows that dynamic changes existed in the respiratory tract and gut antibiotic resistome of COVID-19 patients with different disease severity and progression, and there are significant changes in the composition of respiratory tract and gut ARGs between ICU and nICU patients. Our findings demonstrate that respiratory tract ARG composition, in particular, changes in the abundance of Aminoglycoside, Vancomycin, and Multidrug subtypes in COVID-19 patients, and gut ARG composition, in particular, changes in the abundance of Multidrug, Vancomycin, and Fosmidomycin subtypes, are correlated with disease severity. Our findings also suggest that respiratory tract and gut antibiotic resistome are closely connected to host immune response and respiratory tract and gut microbiota. Taken together, these novel ARG features provide valuable insight for the future development of protocols and tools for halting the continuing COVID-19 health crisis.
Material and methods
Study design and participants
A total of 66 patients with COVID-19 (46 nICU hospitalized patients and 20 ICU hospitalized patients) and 18 healthy individuals from the First Affiliated Hospital of Zhejiang University were enrolled in this study. Volunteers enrolled in this study varied in age between 13 and 96 years old. The ICU patients were included in the ICU group if they met one of the following conditions: 1) had respiratory failure and required mechanical ventilation, 2) entered into shock, and 3) had complicating nonfunction of other organs.Citation36 Other hospitalized patients were included in the nICU group. We collected sputum and fecal samples from patients at three disease stages (admission, progression, recovery). The disease stages were defined according to a series of clinical symptoms, chest CT scans, laboratory indicators, and virological test results. Patients with the progression of pulmonary inflammatory lesions and higher levels of laboratory inflammation indicators were identified in the progression stage. Patients with improved clinical symptoms, respiratory function, absorption of pulmonary inflammation, and negative virological test results were identified in the recovery stage. In addition, PBMC samples were collected from 48 patients (28 nICU and 20 ICU) in progression stages. Healthy donors were all serologic negative for SARS-CoV-2, and without any comorbidities. All 18 healthy donors have RNA-seq data from PBMCs, and 16 of them have sputum and fecal ARG sequencing data. The study protocol was approved by the Ethics Committee of the First Affiliated Hospital, Zhejiang University School of Medicine, China (2021IIT A0239).
We obtained the patient epidemiological, clinical, and laboratory characteristics, as well as treatment and outcome data, from hospital electronic medical records. A trained team of doctors reviewed the data. Personal lifestyles, comorbidities, symptoms and signs, antibiotic treatment, laboratory indicators, and the progression and resolution of clinical illness were included as clinical data. Laboratory indicators included IL6, IL10, IFN-γ, TNF-α, and PCT levels and lymphocyte, neutrophil, and monocyte numbers. Patient’s smoking status was categorized as either current smoker or nonsmoker. Antibiotic treatment was categorized into used relevant antibiotics and non-used.
Sample collection
Sputum from conscious patients and bronchial aspirates from unconscious patients were included in this study as the respiratory tract samples. Before peripheral blood mononuclear cells (PBMCs) extraction, blood samples were collected in specific collection tubes. PBMCs were then isolated and used for RNA-seq analysis. Fecal samples were collected in the hospital in a special sterile container and stored frozen on dry ice until used. Samples were then incubated in Dulbecco’s phosphate buffered saline, agitated for 15 min, and filtered through a 40-micron filter. During sampling, all medical personnel were equipped with personal protection equipment for biosafety level 3, including solid front-wrap around gowns, goggles, and N95 respirators.
Library generation for PBMC transcriptome sequencing
Total RNAs were extracted from PBMC samples using a QIAamp RNA Blood Mini kit (Qiagen, Valencia, CA) according to the manufacturer’s instructions. Total RNA (1 μg) was used for sequencing library preparation, and the Illumina Total RNA-seq (H/M/R) Library Prep Kit was then used according to manufacturer’s instruction. We used an Agilent Bioanalyzer 2100 to examine library quality. The libraries were pooled together in equimolar amounts to a final concentration of 2 nM. The normalized libraries were denatured with 0.1 M NaOH (Sigma), and pooled denatured libraries were pair-end sequenced with a 150 bps read length on the Illumina NovaSeq 6000 platform.
Library generation for metagenome sequencing
After the host cells were removed using a self-developed host removal kit, DNA was extracted from frozen sputum and fecal samples using a TIANamp Micro DNA kit (DP316, Tiangen Biotech) according to the manufacturer’s recommendations. Agilent 4200 TapeStation (Agilent Technologies) was used to assess DNA quality. DNA libraries were carried out by a standardized procedure for DNA fragmentation, end repair, adapter ligation, and PCR amplification. Agilent Bioanalyzer 2100 was used to assess library quality. Whole-genome shotgun sequencing of the sputum and fecal samples was performed on an Illumina HiSeq 2500 platform. All samples were paired-end sequenced with a 150-bp read length to a targeted data size of 5.0 Gb.
RNA-seq data process
FASTQCCitation37 was used to determine quality control (QC) metrics (base quality distribution) of the initial reads, and we then used NGS QC toolkitsCitation38 to trim low-quality reads. RNA-seq reads were mapped to the reference genome using Tophat.Citation39 Following alignment, Cufflinks was used for transcript assemblyCitation39 and CuffmergeCitation39 was applied to merge transcripts generated from all sequencing samples. The output files were imported separately into CuffdiffCitation39 for further statistical analysis.
Metagenome data process
Sequence reads were passed through the KneadData QC pipeline (http://huttenhower.sph.harvard.edu/kneaddata), which incorporates the TrimmomaticCitation40 and BMTaggerCitation41 filtering and decontamination algorithms to remove low-quality read bases and reads of human origin, respectively: 1) trim non-human reads shorter than 50 nucleotides; 2) exclude samples with <500 000 microbial reads. Taxonomic profiling was performed using the MetaPhlAn2 classifier.Citation42 The classifier relied on approximately 1 million clade-specific marker genes derived from >10 000 microbial genomes to unambiguously classify reads to taxonomies and yield the relative abundances of the taxa identified in the sample.
The ARG types and subtypes were annotated by ARGs-OAP v2.0 with default parameters.Citation43 The abundance of ARG was calculated and normalized by the cell number in the research. We calculated and normalized ARG abundance using the following equation, expressed as ‘copies of ARG per prokaryote’s cell’,
where n is the number of ARG reference sequences belonging to an ARG type or subtype; NARG is the number of the ARG-like sequences aligned to one specific ARG reference sequence; Lreads is the sequencing length of metagenomic reads; LARG-ref is the length of the corresponding specific ARG reference sequences. The cell number was computed based on the following equation.Citation44
where m is the total taxa detected from the metagenomics dataset based on the extracted hypervariable region information; Mi represents the number of copies of taxon i from the CopyRighter database; ai is the number of aligned hypervariable sequences of taxon i in the metagenomics dataset; A is the total number of aligned hypervariable sequences in all m taxa. ARG types or subtypes were excluded that were present in less than 10% of the samples; moreover, unclassified ARG types and subtypes were also excluded in the study.
Statistical analysis
The alpha-diversity of the respiratory tract and gut antibiotic resistome was represented by the Shannon index in the study, which were calculated by the R software (ver.4.1.3) package “vegan.”Citation45 The differences in alpha-diversity between groups were statistically measured using permutational multivariate ANOVA. Fisher exact tests and Mann–Whitney U tests were used to compare categorical variables and continuous variables, respectively. Beta diversity was used to evaluate differences in the antibiotic resistome among samples. Principal coordinates analysis (PCoA) on Bray–Curtis distance was performed to obtain visual representations. This analysis was implemented in the R “vegan” packageCitation45 and visualized using scatter plots. We then performed a multiple linear model regression analysis to investigate associations between ARG types and clinical information in the respiratory tract and the gut. Network analysis was used to explore the co-occurrence of ARGs in the COVID-19 patients based on Spearman correlation analysis. Networks were visualized and analyzed using Cytoscape.Citation46 Spearman correlation analysis was also performed to investigate the relationship between clinical indices, immune-related pathways, and ARG subtypes, the association between gut and respiratory tract ARGs, and the relationship between ARGs and microbial genus and species. Correlation coefficients and significant P-values were computed using R software.
Identification of ARG markers for predicting COVID-19 severity based on respiratory tract and gut metagenome data
To identify ARG markers that distinguish samples from ICU and nICU patients, classification models were performed based on ARG types and subtypes profiles using random-forest models. The models were validated by 10-fold stratified cross-validation testing. In each test, the accuracy of the model was examined by receiver operating characteristic. We conducted two steps in the random-forest model. In the first step, the model was constructed using each of the two antibiotic resistance profiles (i.e., respiratory tract and gut) independently. All the features were taken to conduct a random-forest function using the indicated parameters (500 trees, balanced class weight) and to compute the “feature importance” (Mean Decrease Gini). The optimal number of features was determined using the recursive feature elimination method.Citation47 In the second step, the combination model was constructed by features selected from the separate models (respiratory tract and gut). The features were then selected by the recursive feature elimination method.Citation47 We used the R software (ver4.1.3) package “randomForest” to perform all analyses.
Author contributions
YC was the principal investigator who designed and supervised the study. SZ, FY, DZ, QZ, MX, LY, BL, GX, XY, WC, QW, YT, YD, LH, and WW had roles in recruitment, data collection, and clinical management. YS, WQ, SZ, and YC did bioinformatics, statistical analysis, and data interpretation. YS, WQ, SZ, YC, XC, MT, JB, DH, CL, and MT had roles in results discussion. YS, WQ, SZ, YC, and MT drafted the article, and all authors contributed to review and revision and have seen and approved the final version. The corresponding author attests that all listed authors meet authorship criteria and that no others meeting the criteria have been omitted.
Data sharing
The raw metagenomics sequencing dataset was deposited in the Sequence Read Archive (SRA) under BioProject accession PRJNA950577. Access will be granted after an appropriate request to corresponding author.
Ethical approval
The study protocol was approved by the Ethics Committee of the First Affiliated Hospital, Zhejiang University School of Medicine, China (2021IITA0239). The authors gratefully acknowledge the support of the high-performance computing cluster and the help of Enhui Shen from Center for Bioinformatics and Big data Technology, Zhejiang University.
Supplemental Material
Download Zip (2.1 MB)Acknowledgments
We acknowledge the contributions of other clinical and technical staff of the First Affiliated Hospital, College of Medicine, Zhejiang University.
Disclosure statement
No potential conflict of interest was reported by the author(s).
Supplementary material
Supplemental data for this article can be accessed online at https://doi.org/10.1080/19490976.2023.2223340
Additional information
Funding
References
- Choudhury S, Medina-Lara A, Smith R. Antimicrobial resistance and the COVID-19 pandemic. Bull World Health Organ. 2022;100(5):295–20. doi:10.2471/blt.21.287752.
- Bassetti M, Mularoni A, Giacobbe DR, Castaldo N, Vena A. New antibiotics for hospital-acquired pneumonia and ventilator-associated pneumonia. Semin Respir Crit Care Med. 2022;43(2):280–294. doi:10.1055/s-0041-1740605.
- Feng J, Li B, Jiang X, Yang Y, Wells GF, Zhang T, Li X. Antibiotic resistome in a large-scale healthy human gut microbiota deciphered by metagenomic and network analyses. Environ Microbio. 2018;20(1):355–368. doi:10.1111/1462-2920.14009.
- Crits-Christoph A, Hallowell HA, Koutouvalis K, Suez J. Good microbes, bad genes? The dissemination of antimicrobial resistance in the human microbiome. Gut Microbes. 2022;14(1):2055944. doi:10.1080/19490976.2022.2055944.
- Donà D, Di Chiara C, Sharland M. Multi-drug-resistant infections in the COVID-19 era: a framework for considering the potential impact. J Hosp Infect. 2020;106(1):198–199. doi:10.1016/j.jhin.2020.05.020.
- Rezasoltani S, Yadegar A, Hatami B, Asadzadeh Aghdaei H, Zali MR. Antimicrobial resistance as a hidden menace lurking behind the COVID-19 outbreak: the global impacts of too much hygiene on AMR. Front Microbiol. 2020;11:590683. doi:10.3389/fmicb.2020.590683.
- Monnet DL, Harbarth S. Will coronavirus disease (COVID-19) have an impact on antimicrobial resistance? Euro Surveill. 2020;25(45). doi:10.2807/1560-7917.Es.2020.25.45.2001886.
- Cantón R, Gijón D, Ruiz-Garbajosa P. Antimicrobial resistance in ICUs: an update in the light of the COVID-19 pandemic. Curr Opin Crit Care. 2020;26(5):433–441. doi:10.1097/mcc.0000000000000755.
- Kang Y, Chen S, Chen Y, Tian L, Wu Q, Zheng M, Li Z. Alterations of fecal antibiotic resistome in COVID-19 patients after empirical antibiotic exposure. Int J Hyg Environ Health. 2022;240:113882. doi:10.1016/j.ijheh.2021.113882.
- Su Q, Liu Q, Zhang L, Xu Z, Liu C, Lu W, Ching JY, Li A, Mak JWY, Lui GCY, et al. Antibiotics and probiotics impact gut antimicrobial resistance gene reservoir in COVID-19 patients. Gut Microbes. 2022;14(1):2128603. doi:10.1080/19490976.2022.2128603.
- Merenstein C, Bushman FD, Collman RG. Alterations in the respiratory tract microbiome in COVID-19: current observations and potential significance. Microbiome. 2022;10(1):165. doi:10.1186/s40168-022-01342-8.
- Shen Y, Yu F, Zhang D, Zou Q, Xie M, Chen X, Yuan L, Lou B, Xie G, Wang R, et al. Dynamic alterations in the respiratory tract microbiota of patients with covid-19 and its association with microbiota in the gut. Adv Sci. 2022;9(27):e2200956. doi:10.1002/advs.202200956.
- Broman N, Rantasärkkä K, Feuth T, Valtonen M, Waris M, Hohenthal U, Rintala E, Karlsson A, Marttila H, Peltola V, et al. IL-6 and other biomarkers as predictors of severity in COVID-19. Ann Med. 2021;53(1):410–412. doi:10.1080/07853890.2020.1840621.
- Mittal R, Chourasia N, Bharti V, Singh S, Sarkar P, Agrawal A, Ghosh A, Pal R, Kanwar J, Kotnis A, et al. Blood-based biomarkers for diagnosis, prognosis, and severity prediction of COVID-19: opportunities and challenges. Fam Med Prim Care Rev. 2022;11(8):4330–4341. doi:10.4103/jfmpc.jfmpc_2283_21.
- Kesmez Can F, Özkurt Z, Öztürk N, Sezen S. Effect of IL-6, IL-8/CXCL8, IP-10/CXCL 10 levels on the severity in COVID-19 infection. Int J Clin Pract. 2021;75(12):e14970. doi:10.1111/ijcp.14970.
- Philips CA, Ahamed R, Abduljaleel JKP, Rajesh S, Augustine P. Identification and analysis of Gut Microbiota and functional metabolism in decompensated cirrhosis with infection. J Clin Transl Hepatol. 2023;11(1):15–25. doi:10.14218/jcth.2021.00428.
- Luyt CE, Sahnoun T, Gautier M, Vidal P, Burrel S, Pineton de Chambrun M, Chommeloux J, Desnos C, Arzoine J, Nieszkowska A, et al. Ventilator-associated pneumonia in patients with SARS-CoV-2-associated acute respiratory distress syndrome requiring ECMO: a retrospective cohort study. Ann Intensive Care. 2020;10(1):158. doi:10.1186/s13613-020-00775-4.
- Segrelles-Calvo G, de S Araújo GR, Llopis-Pastor E, Carrillo J, Hernández-Hernández M, Rey L, Melean NR, Escribano I, Antón E, Zamarro C, et al. Candida spp. co-infection in COVID-19 patients with severe pneumonia: prevalence study and associated risk factors. Respir Med. 2021;188:106619. doi:10.1016/j.rmed.2021.106619.
- Zhou T, Wu J, Zeng Y, Li J, Yan J, Meng W, Han H, Feng F, He J, Zhao S, et al. SARS-CoV-2 triggered oxidative stress and abnormal energy metabolism in gut microbiota. MedComm. 2022;3(1):e112. doi:10.1002/mco2.112.
- Shoieb SM, El-Ghiaty MA, El-Kadi AOS. Targeting arachidonic acid–related metabolites in COVID-19 patients: potential use of drug-loaded nanoparticles. Emerg Mater. 2021;4(1):265–277. doi:10.1007/s42247-020-00136-8.
- Qiao M, Ying GG, Singer AC, Zhu YG. Review of antibiotic resistance in China and its environment. Environ Int. 2018;110:160–172. doi:10.1016/j.envint.2017.10.016.
- Guffey AA, Loll PJ. Regulation of resistance in vancomycin-resistant enterococci: the VanRS two-component system. Microorganisms. 2021;9(10):2026. doi:10.3390/microorganisms9102026.
- Mahmoudi H. Bacterial co-infections and antibiotic resistance in patients with COVID-19. GMS Hyg Infect Control.2020; 15. Doc35. doi:10.3205/dgkh000370.
- Li XZ, Barré N, Poole K. Influence of the MexA-MexB-oprM multidrug efflux system on expression of the MexC-MexD-oprJ and MexE-MexF-oprN multidrug efflux systems in Pseudomonas aeruginosa. J Antimicrob Chemother. 2020;46(6):885–893. doi:10.1093/jac/46.6.885.
- Markovic SS, Jovanovic M, Gajovic N, Jurisevic M, Arsenijevic N, Jovanovic M, Jovanovic M, Mijailovic Z, Lukic S, Zornic N, et al. IL 33 correlates with COVID-19 severity, radiographic and clinical finding. Front Med. 2021;8:749569. doi:10.3389/fmed.2021.749569.
- Dickson RP, Erb-Downward JR, Falkowski NR, Hunter EM, Ashley SL, Huffnagle GB. The lung microbiota of healthy mice are highly variable, cluster by environment, and reflect variation in baseline lung innate immunity. Am J Respir Crit Care Med. 2018;198(4):497–508. doi:10.1164/rccm.201711-2180OC.
- Segal LN, Clemente JC, Tsay J-CJ, Koralov SB, Keller BC, Wu BG, Li Y, Shen N, Ghedin E, Morris A, et al. Enrichment of the lung microbiome with oral taxa is associated with lung inflammation of a Th17 phenotype. Nat Microbiol. 2016;1:16031. doi:10.1038/nmicrobiol.2016.31.
- Dhar D, Mohanty A. Gut microbiota and Covid-19- possible link and implications. Virus Res. 2020;285:198018. doi:10.1016/j.virusres.2020.198018.
- Azkur AK, Akdis M, Azkur D, Sokolowska M, Veen W, Brüggen M-C, O’Mahony L, Gao Y, Nadeau K, Akdis CA, et al. Immune response to SARS-CoV-2 and mechanisms of immunopathological changes in COVID-19. Allergy. 2020;75(7):1564–1581. doi:10.1111/all.14364.
- Hoenigl M, Seidel D, Sprute R, Cunha C, Oliverio M, Goldman GH, Ibrahim AS, Carvalho A. COVID-19-associated fungal infections. Nat Microbiol. 2022;7(8):1127–1140. doi:10.1038/s41564-022-01172-2.
- Ren Z, Wang H, Cui G, Lu H, Wang L, Luo H, Chen X, Ren H, Sun R, Liu W, et al. Alterations in the human oral and gut microbiomes and lipidomics in COVID-19. Gut. 2021;70(7):1253–1265. doi:10.1136/gutjnl-2020-323826.
- Partridge SR, Kwong SM, Firth N, Jensen SO. Mobile genetic elements associated with antimicrobial resistance. Clin Microbiol Rev. 2018;31(4). doi:10.1128/cmr.00088-17.
- Ghanizadeh A, Najafizade M, Rashki S, Marzhoseyni Z, Motallebi M, Tsai F-M. Genetic diversity, antimicrobial resistance pattern, and biofilm formation in Klebsiella pneumoniae isolated from patients with coronavirus disease 2019 (COVID-19) and ventilator-associated pneumonia. Biomed Res Int. 2021;2021:1–11. doi:10.1155/2021/2347872.
- Dell’annunziata F, Dell’aversana C, Doti N, Donadio G, Dal Piaz F, Izzo V, De Filippis A, Galdiero M, Altucci L, Boccia G, et al. Outer membrane vesicles derived from Klebsiella pneumoniae are a driving force for horizontal gene transfer. Int J Mol Sci. 2021;22(16):8732. doi:10.3390/ijms22168732.
- Tian M, Liu W, Li X, Zhao P, Shereen MA, Zhu C, Huang S, Liu S, Yu X, Yue M, et al. HIF-1α promotes SARS-CoV-2 infection and aggravates inflammatory responses to COVID-19. Signal Transduct Target Ther. 2021;6(1):308. doi:10.1038/s41392-021-00726-w.
- Köstenberger M, Hasibeder W, Dankl D, Germann R, Hörmann C, Joannidis M, Markstaller K, Müller-Muttonen S-O, Neuwersch-Sommeregger S, Schaden E, et al. SARS-CoV-2: recommendations for treatment in intensive care medicine. Wien Klin Wochenschr. 2020;132(21–22):664–670. doi:10.1007/s00508-020-01734-6.
- Andrews S. FastQC a quality control tool for high throughput sequence data. 2014.
- Patel RK, Jain M, Liu Z. NGS QC Toolkit: a toolkit for quality control of next generation sequencing data. PLos One. 2012;7(2):e30619. doi:10.1371/journal.pone.0030619.
- Trapnell C, Roberts A, Goff L, Pertea G, Kim D, Kelley DR, Pimentel H, Salzberg SL, Rinn JL, Pachter L, et al. Differential gene and transcript expression analysis of RNA-seq experiments with TopHat and Cufflinks. Nat Protoc. 2012;7(3):562–578. doi:10.1038/nprot.2012.016.
- Bolger AM, Lohse M, Usadel B. Trimmomatic: a flexible trimmer for Illumina sequence data. Bioinformatics. 2014;30(15):2114–2120. doi:10.1093/bioinformatics/btu170.
- Rotmistrovsky K, Agarwala R. Bmtagger: best match tagger for removing human reads from metagenomics datasets.
- Truong DT, Franzosa EA, Tickle TL, Scholz M, Weingart G, Pasolli E, Tett A, Huttenhower C, Segata N. MetaPhlAn2 for enhanced metagenomic taxonomic profiling. Nat Methods. 2015;12(10):902–903. doi:10.1038/nmeth.3589.
- Yin X, Jiang X-T, Chai B, Li L, Yang Y, Cole JR, Tiedje JM, Zhang T. Args-OAP v2.0 with an expanded SARG database and hidden Markov models for enhancement characterization and quantification of antibiotic resistance genes in environmental metagenomes. Bioinformatics. 2018;34(13):2263–2270. doi:10.1093/bioinformatics/bty053.
- Yang Y, Jiang X, Chai B, Ma L, Li B, Zhang A, Cole JR, Tiedje JM, Zhang T. Args-OAP: online analysis pipeline for antibiotic resistance genes detection from metagenomic data using an integrated structured ARG-database. Bioinformatics. 2016;32(15):2346–2351. doi:10.1093/bioinformatics/btw136.
- Oksanen J, Kindt R, Legendre P, O’Hara B, Wagner H. The VEGAN package: community ecology package. 2008.
- Smoot ME, Ono K, Ruscheinski J, Wang PL, Ideker T. Cytoscape 2.8: new features for data integration and network visualization. Bioinformatics. 2011;27(3):431–432. doi:10.1093/bioinformatics/btq675.
- RColorBrewer S, Liaw MA. Package ‘randomForest’. Berkeley (CA):University of California,Berkeley; 2018.