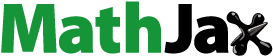
ABSTRACT
Virulent genes present in Escherichia coli (E. coli) can cause significant human diseases. These enteropathogenic E. coli (EPEC) and enterotoxigenic E. coli (ETEC) isolates with virulent genes show different expression levels when grown under diverse laboratory conditions. In this research, we have performed differential gene expression analysis using publicly available RNA-seq data on three pathogenic E. coli hybrid isolates in an attempt to characterize the variation in gene interactions that are altered by the presence or absence of virulent factors within the genome. Almost 26.7% of the common genes across these strains were found to be differentially expressed. Out of the 88 differentially expressed genes with virulent factors identified from PATRIC, nine were common in all these strains. A combination of Weighted Gene Co-Expression Network Analysis and Gene Ontology Enrichment Analysis reveals significant differences in gene co-expression involving virulent genes common among the three investigated strains. The co-expression pattern is observed to be especially variable among biological pathways involving metabolism-related genes. This suggests a potential difference in resource allocation or energy generation across the three isolates based on genomic variation.
Introduction
Enteropathogenic E. coli (EPEC) is a public health concern as infection can lead to severe cases of diarrhea and is especially lethal among young children in developing countries.Citation1–5 The major components of EPEC pathogenesis include the type III secretion system (T3SS) encoded by the locus of enterocyte effacement (LEE) island cluster region. A second pathogenicity island that encodes EspC is inserted into the genome by insertion elements or phages and helps develop the bundle forming pilus (BFP).Citation6–11 These elements are involved in translocation of bacterial factors into host cells and host cell adherence. In the past, EPEC pathogenesis and virulence factor (VF) regulation have primarily focused on only a few prototype isolates (E2348/69, B171, E22, and E110019).Citation12–14 More recently, global transcriptional analysis of pathogens has identified genome-wide transcription that promotes pathogenesis or novel virulence-associated factors.Citation15–20
Global gene expression of EHEC has been investigated under several conditions, such as (1) response to nutrient limitation, (2) exposure to host cells, and (3) during growth phases.Citation15,Citation21–23
ETEC is a significant contributor to bacterial diarrheal disease. ETEC creates two unique toxins (LT-heat labile toxin and ST-heat stable toxin) that cause diarrhea by inducing the intestines to discharge an excessive amount of fluid. Frequent, watery diarrhea, cramping in the abdomen, fever, and nausea are some of the signs of an ETEC infection. EPEC and some Shiga toxin-producing E. coli (STEC) are two types of E. coli that cause enteric disease. Both types of E. coli have a gene cluster LEE, which is found on a chromosomal pathogenicity island. EPEC frequently causes sporadic diarrhea in adults and frequent infantile diarrhea (outbreaks). It is, however, noninvasive and nontoxic. The chromosomal LEE gene is responsible for the production of attaching and effacing lesions, which damage the brush border epithelium and result in increased secretion and watery diarrhea.Citation10,Citation24,Citation25 Each of the nine definite E. coli pathotypes, including EPEC and ETEC, has a specific set of virulence factors (some in common across several pathotypes) that lead to the observed clinical virulence and pathogenicity.Citation26 A recent review summarizes our current understanding of the activity/function that different virulence factors have across the nine E. coli pathotypes.Citation27
The regulation of EPEC virulence genes involves numerous transcriptional factors and can be affected by environmental conditions and cell density.Citation28–31,Citation7,Citation32–37 Several genes have been identified as important regulators of VFs. The first gene in the LEE1 operon, LEE-encoded regulator (Ler), positively modulates the expression of other LEE operons through promoter activation.Citation38 In addition to regulation by external factors such as host body temperature and growth conditions, Ler can auto-regulate thereby utilizing an internal balance mechanism to maximize colonization efficiency while limiting host immune detection.Citation39–41 Global regulators of Ler operon, GrlA and GrlR, promote and repress the expression of virulence genes, respectively.Citation42 The EPEC adherence factor (EAF) plasmid can contain a plasmid-encoded regulator (Per) operon that is transcribed as a single polycistronic mRNA and independent of GrlA can activate LEE1.Citation43–45,Citation32
In this study, our objective was to identify which genes across three EPEC/ETEC hybrid strains were correlated with differentially expressed virulent genes in an attempt to understand the impact of the presence of virulent factors on E. coli biological processes. Additionally, we wanted to determine the impact of media (i.e., environment) on the expression of these virulent genes and the correlation structure between virulence genes and other differentially expressed genes. The aim was to identify how different E. coli strains may alter resource allocation to different biological processes under different conditions. Such interaction may also allow the investigation of virulence gene expression in emerging virulent multidrug resistance (MDR) strains. MDR has increased all over the world which is considered a public health threat. Several recent investigations reported the emergence of virulent multidrug-resistant bacterial pathogens from different origins that increase the necessity for the proper use of antibiotics.Citation46–50 Results from differential expression combined with a gene enrichment analysis allow us to hypothesize the potential role environmental nutrient availability may play in virulent gene expression, E. coli strain pathogenicity, and provide a gene list of potential treatment targets.
Materials and methods
RNA-seq data
In the first stage of our research, 24 publicly available RNA-seq expressions were downloaded for three EPEC/ETEC hybrid isolates, namely 102651, 102712, and 401140 (). These hybrid isolates were reported by Hazen et al.Citation51 The paper analyzed these three hybrid isolates. Since they contained the LEE region, these hybrid isolates possessed the features of EPEC. 102651 and 102712 also possessed the eltA and eltB genes responsible for heat-labile toxin which is common for many ETECs. 401140 contained the eatA gene which is also a feature of ETECs. The presence of features from both EPEC and ETEC makes them hybrid isolates. After performing global transcriptional analysis through RNA-sequencing, Hazen et al.Citation51 identified these hybrid isolates to show variation in virulent gene content based on laboratory growth conditions. The authors also reported based on phylogenomic analysis that these hybrid isolates originated from EPEC and acquired ETEC virulent genes. Based on their research findings and the presence of both ETEC/EPEC virulent factors, these three strains were selected for our research. These strains also showed variations in their degree of evolutionary genomic differences and that relationship is visualized in . shows sample information and experimental conditions.
Figure 1. Phylogenetic tree depicting natural history of the E. coli genomes. 102651, 1026712, and 401140 are in red. Core genes were aligned using Multiple Sequence Alignment based on Fast Fourier transform (MAFFT)Citation52 in the Rapid Large-Scale Prokaryote Pan Genome Analysis (Roary)Citation53 pipeline. RAxML was used for tree generation using 1000 trees for bootstrapping, and bootstrap convergence assessment using autoMRE criterion stopped after 200 trees. Hybrid strains were evaluated by passing the output of RAxML through ClonalFrameML using a transition/transversion ratio of 2.5.

Table 1. RNA-seq sample information for the strains and experimental conditions selected for use in this studyCitation51
Differential expression analysis
The first goal in analyzing these RNA-seq datasets is to identify differentially expressed genes across and within growth conditions. Mapping of the FASTQ files to the reference genomes available in the NCBI database was completed using Burrows-Wheeler Alignment Tool. This tool provides the BWA-MEM algorithm,Citation54 minimizes sequencing errors, and is able to map sequences of around 100 bp with superior performance. After this step in the analysis, new alignment files are generated in Sequence Alignment Map (SAM) format which is converted to Binary Alignment Map (BAM) format to save on storage space. With the genomecov function in bedtools,Citation55 we were able to compute the coverage of sequence alignments in BAM files using the reference genome. The total count data across multiple conditions per sample were combined, and genome annotation for each strain was completed using a General Feature Format (GFF) files generated by Prokka.Citation56 Finally, these annotated files were used to identify common genes across all strains using the RoaryCitation53 pan genome pipeline. Processing was completed on North Dakota State University CCAST Servers.
Further analysis to obtain differentially expressed genes using these count data was completed using DESeq2.Citation57 The process began by discarding reads from rRNA (all of them) since they distort the distribution of the other RNAs. An additional technical problem with estimating rRNA expression is that the mapping algorithm will mainly pile up the rRNA reads on the first copy in the genome and fail to distinguish expression from that first operon compared to the others (E. coli genomes range from 6 to 10 copies of rRNA operons). We used the regularized-logarithm (rlog) transformation offered by DESeq2 on the raw read counts. This transformation ensures that the genes with high counts do not affect the results extensively, while genes with lower counts have values attenuated toward genes’ averages. The rlog transformation uses ridge penalty to perform this operation ensuring that the generated read counts are approximately homoskedastic. The scatter plot in (see Appendix) shows the difference in values before and after transformation for isolate 102651. Then, differential expression (DE) of these genes was returned by the function for all three isolates. Any gene without expression data across all mediums was excluded in this study along with rRNA read counts. Differential expression was conducted as a pairwise comparison between two groups in every isolate. False Discovery Rate (FDR) values of <0.05 were considered to represent a significant differential expression for a gene.
Co-expression network analysis
A co-expression network for each of these isolates was developed to further understand gene interactions using the Weighted Gene Co-expression Network Analysis (WGCNA) package.Citation58 The first step toward WGCNA analysis is generating an undirected and weighted co-expression network where nodes are genes and edges represent the pairwise correlation between gene expressions. WGCNA offers the application of a soft-thresholding power, , on the co-expression network to make it a scale-free topology.
As shown in Equation 1, through an iterative process, we raise the correlation of the genes and
in the adjacency matrix to an extent that reduces noise in the dataset. For our experiments, we obtained
= 14, 14, and 12 for 102651, 102712, and 401140 respectively. This is followed by transforming the adjacency matrix into a Topological Overlap Matrix (TOM) which further reduces noise. The TOM output is then used to perform a hierarchical clustering of the genes using agglomerative clustering with average linkage. Highly co-expressed genes in branches are grouped together in a single cluster using a minimum set of genes per cluster. This is followed by merging groups/modules based on highly co-expressed profiles. Finally, CytoscapeCitation59 is used for visualizing these network modules.
Enrichment analysis
After the identification of differentially expressed genes and WGCNA modules, an enrichment analysis was performed separately on the modules that are associated with virulent genes. This technique reveals biological pathways that are more prevalent than would be predicted by random in a gene list.Citation60 The tool used for this step was clusterProfilerCitation61 which performed gene set enrichment analysis using the pathways reported in Gene Ontology (GO).Citation62
Results
The following section demonstrates the results obtained from analyzing the hybrid isolates and associated genes with virulent factors. Group variability in samples is done using PCA followed by differential gene expression analysis across four mediums. This is followed by the identification of genes with virulence factors followed by co-expression network analysis of these genes across the strains.
Group variability in samples
After discarding rRNA reads and rlog transformation, principal component analysis (PCA) was applied which demonstrated that most of the replicates were grouped based on media type as shown in (see Appendix). In 102651 and 102712, the PCA was able to represent 52.9% of the variation, while 65.2% of the variation was demonstrated in 401140 using PC1 and PC2. A combined PCA of all three strains showed that 59.7% of variation could be explained using the first two principal components. The clustering of 3740 genes that were common across all strains is shown in . The overall gene expression pattern here also demonstrates that the transcriptional pattern is heavily impacted by the growth medium. We also notice that the unique genomic content of each isolate plays a significant role in transcription.
Figure 2. Heatmap showing hierarchical clustering of 3740 common genes (y-axis) in strains across all mediums. The x-axis labels take the format medium_sample_strain. The four medium types are DMEMB, DMEM, LBB, and LB, and the three E.Coli strains are 401140, 102651, and 102712. The colors in the heatmap represent rlog expression values with green having the lowest value and red the highest.

Differential expression analysis
shows the number of differentially expressed genes across all three isolates. Differential expression analysis on the 3740 common genes in these hybrid E. coli isolates revealed 394 (10.5%) differentially expressed genes across DMEMB and DMEM and 510 (13.6%) across LB and DMEM medium. A total of 611 (16.3%) genes were differentially expressed while comparing isolates grown in LBB and DMEMB, while only 206 (5.5%) genes were observed to be differentially expressed for isolates grown in LBB and LB. In total, we observed that 998 of the 3740 (26.7%) common genes were differentially expressed in a minimum of one medium comparison.
Table 2. Differentially expressed genes across all mediums for the isolates 102651, 102712, and 401140. The first column shows which conditions were compared for the analysis. Results from the gray highlighted row were used for further analysis as it compared variation across all four mediums LB, LBB, DMEM, and DMEMB.
Virulence gene analysis
We explored how genes with virulent factors are distributed across these hybrid isolates. Information about specialty genes associated with these strains was derived from Pathosystems Resource Integration Center (PATRIC)Citation63 database. After extracting specialty genes labeled as virulent factors, we were able to identify 115 virulent genes across these three isolates. highlights the distribution of virulent genes across these strains. After identifying the differentially expressed genes in these isolates, we filtered the dataset to obtain virulent genes that were differentially expressed again using FDR < 0.05. These data are shown in where differentially expressed virulent genes are either in blue or red. The higher the intensity of the blue, the higher the log fold change (LFC) gene expression, and the higher the intensity of red, the more negative the LFC gene expression.
Figure 3. (a) Presence of virulent genes where yellow represents if they are present and blue if absent (b) their log-fold change (LFC) expression values across all three strains. The higher the intensity of the blue, the higher the log fold change (LFC) expression, and the higher the intensity of red, the more negative the LFC expression.

The venn-diagram in displays the total number of virulent genes common in these strains, while the venn-diagram in shows the number of virulent genes that are differentially expressed. For the next stage of this research, we focused on how these differentially expressed virulent genes interact with other genes within each isolate. We see across all three strains that there are 88 virulent genes in common, but only nine (10.2%) of these genes are differentially expressed across all three strains.
Figure 4. Venn diagram showing the number of virulent genes across strains before and after differential expression analysis. (a) Count of virulent genes appearing in each corresponding genome. (b) Count of significant differentially expressed virulent genes appearing in each corresponding genome.

lists the nine differentially expressed genes with virulent factors found in all three isolates as identified in the previous section. We will focus our attention on how these virulent genes correlate with other differentially expressed genes in their respective strain genomes. To analyze this interaction, correlation networks were generated using Weighted Gene Co-Expression Network Analysis (WGCNA). A detailed explanation of the pipeline is provided in the next section.
Table 3. Log fold change (LFC) expression values for the nine virulent genes present in all three isolates 102651, 102712, and 401140 and differentially expressed across the three strains based on the FDR value.
Co-expression network analysis
WGCNA analysis using soft-thresholding power was used to generate Topological Overlap Matrices (TOM) for all three strains. After analyzing the dendrograms in , the minimum number of genes per module size was set to 100 to group genes with similar patterns and to facilitate network interaction. This process was able to identify 20 modules in 102651, 23 in 102712, and 17 for 401140. The dynamic tree-cut process was implemented. This approach merges modules that have a high degree of correlation in their genes. Modules with a degree of correlation of 0.75 or above were merged together into a single module. This step reduced the number of modules to 14, 14, and 9 in 102651, 102712, and 401140, respectively. The results of the clustering are shown in
Figure 5. WGCNA results after clustering (top color band) and merging (bottom color band) groups for three isolates a: 102651, b: 102712, and c: 401140. These networks will be explored to study virulent gene interactions.

A summary of the modules is provided in . It is observed that differentially expressed virulent genes are usually clustered in a single module showing a very high level of interaction. In strain 102651, there are four virulent genes in the brown module; in strain 102712, there are 14 differentially expressed virulent genes in the cyan module, while strain 401140 has nine virulent genes in the black module. In some situations, we also observed the virulent genes as hub genes (genes with the highest number of connections) for modules having a significant impact. This is the case for the black module in strain 102651 where the hub gene ompA is virulent, with strain 102712 for genes cesT, entA, and entE in brown and cyan modules. Based on the results in , we have four modules from strain 102651, five modules from strain 102712, and six modules from strain 401140 containing virulent genes highlighted in . These modules are highlighted in yellow in . The common genes are in red. After extraction, strains 102651, 102712, and 401140 had a total of 248, 223, and 263 differentially expressed genes that were used to perform enrichment analysis. Together, the selected genes accounted for above 73%, 71%, and 58% of all the differentially expressed genes identified in the strains by DESeq2.
Table 4. Module hub genes in 102651 that were differentially expressed with functions and virulent genes identified by WGCNA. Yellow identifies the modules which contain virulent genes included in .
Table 5. Module hub genes in 102712 that were differentially expressed with functions and virulent genes identified by WGCNA. Yellow identifies the modules which contain virulent genes included in .
Table 6. Module hub genes in 401140 that were differentially expressed with functions and virulent genes identified by WGCNA. Yellow identifies the modules which contain virulent genes included in .
To further analyze strain differences, differentially expressed genes associated with dppA, narG, and ydeP were explored. These genes were selected based on their expression levels: showing the highest negative expression, highest positive expression, and significant difference in expression across all strains, respectively. shows how WGCNA modules are related to each other in these strains. It is observed that in strain 102651, dppA belongs to the brown module which is closely related to the light green module. Genes narG and ydeP are in green module which is significantly different from the brown module. In strain 102712, dppA was found in a blue module which is closely related to purple module and black module housing both narG and ydeP. Finally, in strain 401140, dppA and ydeP were both found in black module. However, the midnightblue module housing narG was significantly different in the clustering diagram. Hence, narG was analyzed separately. show the first neighbors based on the association between differentially expressed genes and dppA, narG, and ydeP derived using Cytoscape.Citation64 The networks were filtered using the top 50th percentile of edge weights between these nodes.
Figure 6. WGCNA hierarchical clustering of modules for three isolates (a) 102651, (b) 102712, and (c) 401140. The selected virulent genes (dppA, narG, ydeP) are identified in red in each tree. These virulent genes were differentially expressed.

Figure 7. Network visualization of first neighbors using Cytoscape for strain 102651. Node size resembles its degree. It is observed that dppA is highly connected in the module. ydeP shows least connections. A larger node represents a higher number of connections. Larger edge weights are represented with thicker and blue lines.

Enrichment analysis
To identify the difference in the interaction of virulent genes across strains, we performed an enrichment analysis for differentially expressed genes associated with dppA, narG, and ydeP. show the results of the enrichment analysis in these three strains highlighting the top 25 biological processes associated with the three virulent genes. The analysis was performed using the clusterProfilerCitation61,Citation65 tool. This tool performed GO enrichment analysisCitation62 by comparing genes identified in WGCNA modules with all the genes in the particular strain (referred as background genes). We compared these genes using the entire genome-wide annotation set for E. coli strain K12 available from org.EcK12.eg.db.Citation66 The enrichment analysis uses hypergeometric testing to determine if a group of genes share similar function and are associated with any pathway. Prior to performing an enrichment analysis, genes in these strains were mapped to their corresponding Entrez IDs using clusterProfiler.Citation65 There were only 22 biological pathways identified in green module of strain 102651 having narG and ydeP. In brown module of strain 102651 with gene dppA, 59 biological processes were identified. The GO categories were obtained using pvalues adjusted by Benjamini-Hochberg procedure with cutoff <0.05. For strain 102712, due to a high degree of correlation between black, blue, and purple modules, a GO enrichment analysis on the combined genes identified 52 biological processes. Finally, in strain 401140, the black module with genes dppA and ydeP was observed to have 84 biological processes, and genes in midnight blue module with narG were associated with 26 biological processes.
Figure 10. Biological pathways identified using genes from WGCNA modules with virulent genes for two isolates (a) 102651, and (b) 102712. The x-axis represents the gene ratio and the y-axis represents the identified biological pathways. The color and size of the plots represent the FDR and the count of genes in those biological pathways, respectively.

Figure 11. Biological pathways identified using genes from WGCNA modules with virulent genes for isolate 401140. The x-axis represents the gene ratio and the y-axis represents the identified biological pathways. The color and size of the plots represent the FDR and the count of genes in those biological pathways, respectively.

Enrichment analysis revealed interesting patterns in how the virulent genes were interacting with the other genes in the modules. Of the three virulence genes, only dppA and NarG formed unique interacting gene modules. The dppA gene in E. coli is a dipeptide-binding protein (DBP). Dipeptide-binding proteins inhibit heme binding. Some functions of this dppA gene include dipeptide/peptide transmembrane transporter activity and binding to heme and peptides.Citation67 In , dppA is involved with amino acid uptake and metabolism genes. Many of them are co-transcribed. As these are growing in different media – though both with amino acids in abundance. A comparative analysis of the biological processes associated with the WGCNA module with dppA is shown in . We noticed a pattern where the biological processes are similar for strain 102651 and strain 401140 but not strain 102712.
Table 7. Biological processes associated with dppA identified by GO enrichment analysis.
Respiratory nitrate reductase 1 alpha chain (narG) is an enzyme complex that uses nitrate as an electron acceptor during anaerobic growth. NarG has many functions including, but not limited to, electron transfer activity, metal ion binding, nitrate assimilation, and nitrate metabolic process. NarG is also involved in GspD assembly and LF secretion by introducing nitrate into the system as shown in . Similarity was also observed here between 102651 and 401140.
Table 8. Biological processes associated with narG identified by GO enrichment analysis.
Discussion
We observed that among the three EPEC/ETEC strains there were unique gene expression patterns. When looking at the 3,740 common genes, expression patterns varied across medium and across strain with most expression differences occurring for the comparison of LBB to DMEMB. Constructing gene expression networks demonstrated high clustering to the same module among virulence genes. Of the virulence genes, nine were common virulence genes identified to be differentially expressed in each of the three strains. Building gene networks for three of these virulence genes (dppA, narG, and ydeP) which demonstrated the largest differences showed both unique and common gene correlations with other genes across the E. coli genome.
The differences we observed in the co-expression of virulence factors and metabolism-related genes across the three E. coli isolates suggest that gene networks are dependent on genome content in different strains. This further suggests that potential clinical treatments may be less effective for specific isolates because of the differences in these gene interactions, but further research is needed.
Previous studies have provided evidence that resource/nutrient allocation is directed toward either the promotion of virulence factor or other cellular functions like growth depending on the environmental conditions.Citation9,Citation68,Citation69 The regulators of virulence factors include both those that are pathogenic-specific and those that are common across E. coli strains.Citation7 Our research suggests that there is variation in this process across E. coli strains, potentially leading to variations in the severity of disease within the host. Observations that the correlations between virulence factors and biological pathways varied by strain further support this hypothesis. Among the three virulence factors investigated in more detail here, dppA is uniquely highly correlated with pathways related to metal transporter genes while narG is uniquely highly correlated with pathways related to respiration and energy production.
Research byAlteri, Smith, & MobleyCitation70 investigated the importance of bacterial metabolism while invading host cells and was able to identify the importance of mutant strains dppA and oppA as important to the fitness of uropathogenic E. coli (UPEC) as they are responsible for peptide transport. Xu et al.Citation71 also noted the significance of dppA in creating more outer membrane proteins (OMPs) associated with cell adhesion. In our study investigating EPEC/ETEC gene expression, we also observed that dppA was highly correlated with many transport and homeostasis genes, metabolic, catabolic, and processing genes.
Lu, Fu, Xie, & JinCitation72 identified that narG plays a significant role in the proper functioning of terminal electron acceptors, which are crucial to the secretion of heat-labile enterotoxin (LT) in ETEC strains under anaerobic conditions. LT is a major virulence factor and can cause severe diarrhea. In strain 102651, narG identified in the green module was associated with 10 out of 22 biological processes. A similar pattern was observed in strain 401140 where it was associated with nine of the 26 biological processes in the midnight blue module. However, we did not observe significant interaction of narG in 102712, where it was only associated with three of the 52 biological processes identified in the black, blue, and purple modules.
Masuda & ChurchCitation73 stated that ydeP is a novel gene related to acid resistance. It has many cofactor binding sites and responds to acidic pH levels. It works with cluster binding and formate dehydrogenase activity. This gene is a part of the prokaryotic molybdopterin containing oxidoreductase family. While ydeP showed significant variation in expression level, we noticed any unique biological processes that it was associated with the enrichment analysis. This may be due to its lower expression level across all the strains.
Conclusion
In this research, we were able to identify how virulent genes show differences in expression levels across various strains even within the same growth medium. Differential expression analysis followed by the generation of WGCNA modules further demonstrates potential differential gene correlations with virulence factor expression across strains. Enrichment analysis is used to provide a potential biological impact that these virulent gene expression-level variations may have and provides a reduced list of future treatment targets in research. A limitation of the current study is that the publicly available dataset only included duplicate instead of triplicate data. This limits our ability to provide and demonstrate our results in a more robust way. Future work will explore these hybrid EPEC/ETEC isolates along with samples having more number of replicates. This will enable a robust differential expression analysis.Citation74 Coupled with modern gene regulatory algorithms,Citation75 we will explore molecular biology from these gene interactions in an optimized manner.
Disclosure statement
No potential conflict of interest was reported by the author(s).
Data availability statement
This is a secondary data analysis. The original data that support the findings of this study are openly available at https://www.ncbi.nlm.nih.gov/. The accession numbers can be found inCitation51 https://doi.org/10.1038/s41598-017-03489-z.
Additional information
Funding
References
- Bittinger K, Zhao C, Li Y, Ford E, Friedman ES, Ni J, Kulkarni CV, Cai J, Tian Y, Liu Q, et al. Bacterial colonization reprograms the neonatal gut metabolome. Nature Microbiol. 2020;5(6):838–22. doi:10.1038/s41564-020-0694-0.
- Kotloff KL, Nataro JP, Blackwelder WC, Nasrin D, Farag TH, Panchalingam S, Wu Y, Sow SO, Sur D, Breiman RF, et al. Burden and aetiology of diarrhoeal disease in infants and young children in developing countries (the global enteric multicenter study, gems): a prospective, case-control study. Lancet. 2013;382(9888):209–222. doi:10.1016/S0140-6736(13)60844-2.
- Ochoa TJ, Contreras CA. Enteropathogenic “E. coli” (epec) infection in children. Curr Opin Infect Dis. 2011;24(5):478. doi:10.1097/QCO.0b013e32834a8b8b.
- Snehaa K, Singh T, Dar SA, Haque S, Ramachandran VG, Saha R, Shah D, Das S. Typical and atypical enteropathogenic Escherichia coli in children with acute diarrhoea: changing trend in east delhi. Biomed J. 2021;44(4):471–478. doi:10.1016/j.bj.2020.03.011.
- Stoll BJ, Puopolo KM, Hansen NI, Sánchez PJ, Bell EF, Carlo WA, Cotten CM, D’Angio CT, Kazzi SNJ, Poindexter BB, et al. Early-onset neonatal sepsis 2015 to 2017, the rise of Escherichia coli, and the need for novel prevention strategies. JAMA Pediatr. 2020;174(7):e200593–e200593. doi:10.1001/jamapediatrics.2020.0593.
- Garcia BG, Castro FS, Vieira MA, Girão DM, Uenishi LT, Cergole-Novella MC, dos Santos LF, Piazza RMF, Hernandes RT, Gomes TAT, et al. Distribution of the pils gene in Escherichia coli pathovars, its transfer ability and influence in the typical enteropathogenic “E. coli” adherence phenotype. Int J Med Microbiol. 2019;309(1):66–72. doi:10.1016/j.ijmm.2018.12.001.
- Kaper JB, Nataro JP, Mobley HL. Pathogenic Escherichia coli. Nat Rev Microbiol. 2004;2(2):123–140. doi:10.1038/nrmicro818.
- McDaniel TK, Jarvis KG, Donnenberg MS, Kaper JB. A genetic locus of enterocyte effacement conserved among diverse enterobacterial pathogens. Proc Natl Acad Sci USA. 1995;92(5):1664–1668. doi:10.1073/pnas.92.5.1664.
- Mellies JL, Barron AM, Carmona AM. Enteropathogenic and enterohemorrhagic Escherichia coli virulence gene regulation. Infect Immun. 2007;75(9):4199–4210. doi:10.1128/IAI.01927-06.
- Nataro JP, Kaper JB. Diarrheagenic Escherichia coli. Clin Microbiol Rev. 1998;11(1):142–201. doi:10.1128/CMR.11.1.142.
- Nisa S, Hazen TH, Assatourian L, Nougayrède J-P, Rasko DA, Donnenberg MS. In vitro evolution of an archetypal enteropathogenic Escherichia coli strain. J Bacteriol. 2013;195(19):4476–4483. doi:10.1128/JB.00704-13.
- Iguchi A, Thomson NR, Ogura Y, Saunders D, Ooka T, Henderson IR, Harris D, Asadulghani M, Kurokawa K, Dean P, et al. Complete genome sequence and comparative genome analysis of enteropathogenic Escherichia coli O127:H6 strain e2348/69. J Bacteriol. 2009;191(1):347–354. doi:10.1128/JB.01238-08.
- Rasko DA, Rosovitz M, Myers GS, Mongodin EF, Fricke WF, Gajer P, Crabtree J, Sebaihia M, Thomson NR, Chaudhuri R, et al. The pangenome structure of Escherichia coli: comparative genomic analysis of “E. coli” commensal and pathogenic isolates. J Bacteriol. 2008;190(20):6881–6893. doi:10.1128/JB.00619-08.
- Viljanen M, Peltola T, Kuistila M, Huovinen P, Junnila S, Olkkonen L, Järvinen H. Outbreak of diarrhoea due to Escherichia coli 0111: B4 in schoolchildren and adults: association of vi antigen-like reactivity. Lancet. 1990;336(8719):831–834. doi:10.1016/0140-6736(90)92337-H.
- Bergholz TM, Wick LM, Qi W, Riordan JT, Ouellette LM, Whittam TS. Global transcriptional response of Escherichia coli O157:H7 to growth transitions in glucose minimal medium. BMC Microbiol. 2007;7(1):1–27. doi:10.1186/1471-2180-7-97.
- Camarena L, Bruno V, Euskirchen G, Poggio S, Snyder M, Roy CR. Molecular mechanisms of ethanol-induced pathogenesis revealed by RNA-sequencing. PLoS Pathog. 2010;6(4):e1000834. doi:10.1371/journal.ppat.1000834.
- Chugani S, Kim BS, Phattarasukol S, Brittnacher MJ, Choi SH, Harwood CS, Greenberg EP. Strain-dependent diversity in the Pseudomonas aeruginosa quorum-sensing regulon. Proc Natl Acad Sci USA. 2012;109(41):E2823–E2831. doi:10.1073/pnas.1214128109.
- Lang C, Fruth A, Holland G, Laue M, Mühlen S, Dersch P, Flieger A. Novel type of pilus associated with a Shiga-toxigenic “E. coli” hybrid pathovar conveys aggregative adherence and bacterial virulence. Emerging Microbes Infect. 2018;7(1):1–16. doi:10.1038/s41426-018-0209-8.
- Mandlik A, Livny J, Robins WP, Ritchie JM, Mekalanos JJ, Waldor MK. RNA-seq-based monitoring of infection-linked changes in vibrio cholerae gene expression. Cell Host Microbe. 2011;10(2):165–174. doi:10.1016/j.chom.2011.07.007.
- Sahl JW, Rasko DA, Payne SM. Analysis of global transcriptional profiles of enterotoxigenic Escherichia coli isolate e24377a. Infect Immun. 2012;80(3):1232–1242. doi:10.1128/IAI.06138-11.
- Abu-Ali GS, Ouellette LM, Henderson ST, Lacher DW, Riordan JT, Whittam TS, Manning SD. Increased adherence and expression of virulence genes in a lineage of Escherichia coli O157:H7 commonly associated with human infections. PLoS One. 2010;5(4):e10167. doi:10.1371/journal.pone.0010167.
- Bingle LE, Constantinidou C, Shaw RK, Islam MS, Patel M, Snyder LA, Lee DJ, Penn CW, Busby SJW, Pallen MJ, et al. Microarray analysis of the ler regulon in enteropathogenic and enterohaemorrhagic Escherichia coli strains. PLoS One. 2014;9(1):e80160. doi:10.1371/journal.pone.0080160.
- Jandu N, Ho NK, Donato KA, Karmali MA, Mascarenhas M, Duffy SP, Tailor C, Sherman PM. Enterohemorrhagic Escherichia coli O157∶H7 gene expression profiling in response to growth in the presence of host Eithelia. PLoS One. 2009;4(3):e4889. doi:10.1371/journal.pone.0004889.
- Parija SC. 2012. Textbook of microbiology & immunology. New Delhi: Elsevier.
- Scaletsky IC, Fabbricotti SH, Carvalho RL, Nunes CR, Maranhao HS, Morais MB, Fagundes-Neto U. Diffusely adherent Escherichia coli as a cause of acute diarrhea in young children in Northeast Brazil: a case-control study. J Clin Microbiol. 2002;40(2):645–648. doi:10.1128/JCM.40.2.645-648.2002.
- Mainil J. Escherichia coli virulence factors. Vet Immunol Immunopathol. 2013;152(1–2):2–12. doi:10.1016/j.vetimm.2012.09.032.
- Pakbin B, Brück WM, Rossen JW. Virulence factors of enteric pathogenic Escherichia coli: a review. Int J Mol Sci. 2021;22(18):9922. doi:10.3390/ijms22189922.
- Bhatt S, Anyanful A, Kalman D. CSRA and tnab coregulate tryptophanase activity to promote exotoxin-induced killing of Caenorhabditis elegans by enteropathogenic Escherichia coli. J Bacteriol. 2011;193(17):4516–4522. doi:10.1128/JB.05197-11.
- Deng W, Puente JL, Gruenheid S, Li Y, Vallance BA, Vázquez A, Barba J, Ibarra JA, O’Donnell P, Metalnikov P, et al. Dissecting virulence: systematic and functional analyses of a pathogenicity island. Proc Natl Acad Sci USA. 2004;101(10):3597–3602. doi:10.1073/pnas.0400326101.
- Elliott SJ, Sperandio V, Girón JA, Shin S, Mellies JL, Wainwright L, Hutcheson SW, McDaniel TK, Kaper JB. The Locus of Enterocyte Effacement (LEE)-encoded regulator controls expression of both LEE- and Non-LEE-Encoded virulence factors in enteropathogenic and enterohemorrhagic Escherichia coli. Infect Immun. 2000;68(11):6115–6126. doi:10.1128/IAI.68.11.6115-6126.2000.
- Garmendia J, Frankel G, Crepin VF. Enteropathogenic and enterohemorrhagic Escherichia coli infections: translocation, translocation, translocation. Infect Immun. 2005;73(5):2573–2585. doi:10.1128/IAI.73.5.2573-2585.2005.
- Mellies JL, Elliott SJ, Sperandio V, Donnenberg MS, Kaper JB. The per regulon of enteropathogenic Escherichia coli: identification of a regulatory cascade and a novel transcriptional activator, the locus of enterocyte effacement (lee)-encoded regulator (ler). Mol Microbiol. 1999;33(2):296–306. doi:10.1046/j.1365-2958.1999.01473.x.
- Puente C, Romeu J, Pous R, Garcia X, Benitez F. Fractal multiband antenna based on the Sierpinski gasket. Electron Lett. 1996;32(1):1–2. doi:10.1049/el:19960033.
- Shin S, Castanie-Cornet M-P, Foster JW, Crawford JA, Brinkley C, Kaper JB. An activator of glutamate decarboxylase genes regulates the expression of enteropathogenic Escherichia coli virulence genes through control of the plasmid-encoded regulator, per. Mol Microbiol. 2001;41(5):1133–1150. doi:10.1046/j.1365-2958.2001.02570.x.
- Sperandio V, Mellies JL, Nguyen W, Shin S, Kaper JB. Quorum sensing controls expression of the type iii secretion gene transcription and protein secretion in enterohemorrhagic and enteropathogenic Escherichia coli. Proc Natl Acad Sci USA. 1999;96(26):15196–15201. doi:10.1073/pnas.96.26.15196.
- Wong AR, Pearson JS, Bright MD, Munera D, Robinson KS, Lee SF, Frankel G, Hartland EL. Enteropathogenic and enterohaemorrhagic Escherichia coli: even more subversive elements. Mol Microbiol. 2011;80(6):1420–1438. doi:10.1111/j.1365-2958.2011.07661.x.
- Yang F, Yang L, Chang Z, Chang L, Yang B. Regulation of virulence and motility by acetate in enteropathogenic Escherichia coli. Int J Med Microbiol. 2018;308(7):840–847. doi:10.1016/j.ijmm.2018.07.010.
- Yerushalmi G, Litvak Y, Gur-Arie L, Rosenshine I. Dynamics of expression and maturation of the type iii secretion system of enteropathogenic Escherichia coli. J Bacteriol. 2014;196(15):2798–2806. doi:10.1128/JB.00069-14.
- Berdichevsky T, Friedberg D, Nadler C, Rokney A, Oppenheim A, Rosenshine I. Ler is a negative autoregulator of the lee1 operon in enteropathogenic Escherichia coli. J Bacteriol. 2005;187(1):349–357. doi:10.1128/JB.187.1.349-357.2005.
- Bhat A, Shin M, Jeong J-H, Kim H-J, Lim H-J, Rhee JH, Paik S-Y, Takeyasu K, Tobe T, Yen H, et al. DNA looping- dependent autorepression of lee1 p1 promoters by ler in enteropathogenic Escherichia coli (epec). Proc Natl Acad Sci USA. 2014;111(25):E2586–E2595. doi:10.1073/pnas.1322033111.
- Platenkamp A, Mellies JL. Environment controls lee regulation in enteropathogenic Escherichia coli. Front Microbiol. 2018;9:1694. doi:10.3389/fmicb.2018.01694.
- Padavannil A, Jobichen C, Mills E, Velazquez-Campoy A, Li M, Leung KY, Mok YK, Rosenshine I, Sivaraman J. Structure of grlr–grla complex that prevents grla activation of virulence genes. Nat Commun. 2013;4(1):1–10. doi:10.1038/ncomms3546.
- Bustamante VH, Villalba MI, García-Angulo VA, Vázquez A, Martínez LC, Jiménez R, Puente JL. Perc and grla independently regulate ler expression in enteropathogenic Escherichia coli. Mol Microbiol. 2011;82(2):398–415. doi:10.1111/j.1365-2958.2011.07819.x.
- Hernandes RT, Elias WP, Vieira MA, Gomes TA. An overview of atypical enteropathogenic Escherichia coli. FEMS Microbiol Lett. 2009;297(2):137–149. doi:10.1111/j.1574-6968.2009.01664.x.
- Lara-Ochoa C, González-Lara F, Romero-González LE, Jaramillo-Rodríguez JB, Vázquez-Arellano SI, Medrano-López A, Cedillo-Ramírez L, Martínez-Laguna Y, Girón JA, Pérez-Rueda E, et al. The transcriptional activator of the bfp operon in epec (pera) interacts with the RNA polymerase alpha subunit. Sci Rep. 2021;11(1):1–11. doi:10.1038/s41598-021-87586-0.
- Algammal A, Hetta HF, Mabrok M, Behzadi P. Emerging multidrug-resistant bacterial pathogens superbugs: a rising public health threat. Front Microbiol. 2023;14. doi:10.3389/fmicb.2023.1135614.
- Algammal AM, Eidaroos NH, Alfifi KJ, Alatawy M, Al-Harbi AI, Alanazi YF, Ghobashy MO, Khafagy AR, Esawy AM, El-Sadda SS, et al. Opr l gene sequencing, resistance patterns, virulence genes, quorum sensing and antibiotic resistance genes of xdr Pseudomonas aeruginosa isolated from broiler chickens. Infect Drug Resist. 2023;16:853–867. doi:10.2147/IDR.S401473.
- Algammal AM, Ibrahim RA, Alfifi KJ, Ghabban H, Alghamdi S, Kabrah A, Khafagy AR, Abou-Elela GM, Abu-Elala NM, Donadu MG, et al. A first report of molecular typing, virulence traits, and phenotypic and genotypic resistance patterns of newly emerging XDR and MDR Aeromonas veronii in mugil seheli. Pathogens. 2022;11(11):1262. doi:10.3390/pathogens11111262.
- Beceiro A, Tomás M, Bou G. Antimicrobial resistance and virulence: a successful or deleterious association in the bacterial world? Clin Microbiol Rev. 2013;26(2):185–230. doi:10.1128/CMR.00059-12.
- Shafiq M, Zeng M, Permana B, Bilal H, Huang J, Yao F, Algammal AM, Li X, Yuan Y, Jiao X, et al. Coexistence of blandm–5 and tet (x4) in international high-risk Escherichia coli clone st648 of human origin in china. Front Microbiol. 2022;13. doi:10.3389/fmicb.2022.1031688.
- Hazen TH, Michalski J, Luo Q, Shetty AC, Daugherty SC, Fleckenstein JM, Rasko DA. Comparative genomics and transcriptomics of Escherichia coli isolates carrying virulence factors of both enteropathogenic and enterotoxigenic “E. coli”. Sci Rep. 2017;7(1):1–17. doi:10.1038/s41598-017-03489-z.
- Katoh K, Misawa K, Kuma K-I, Miyata T. Mafft: a novel method for rapid multiple sequence alignment based on fast Fourier transform. Nucleic Acids Res. 2002;30(14):3059–3066. doi:10.1093/nar/gkf436.
- Page AJ, Cummins CA, Hunt M, Wong VK, Reuter S, Holden MT, Fookes M, Falush D, Keane JA, Parkhill J, et al. Roary: rapid large-scale prokaryote pan genome analysis. Bioinformatics. 2015;31(22):3691–3693. doi:10.1093/bioinformatics/btv421.
- Li H. Aligning sequence reads, clone sequences and assembly contigs with bwa-mem. arXiv Preprint arXiv: 13033997. 2013;1–3.
- Quinlan AR, Hall IM. Bedtools: a flexible suite of utilities for comparing genomic features. Bioinformatics. 2010;26(6):841–842. doi:10.1093/bioinformatics/btq033.
- Seemann T. Prokka: rapid prokaryotic genome annotation. Bioinformatics. 2014;30(14):2068–2069. doi:10.1093/bioinformatics/btu153.
- Love MI, Huber W, Anders S. Moderated estimation of fold change and dispersion for RNA-seq data with deseq2. Genome Biol. 2014;15(12):1–21. doi:10.1186/s13059-014-0550-8.
- Langfelder P, Horvath S. Wgcna: an R package for weighted correlation network analysis. BMC Bioinform. 2008;9(1):1–13. doi:10.1186/1471-2105-9-559.
- Shahan R, Zawora C, Wight H, Sittmann J, Wang W, Mount SM, Liu Z. Consensus coexpression network analysis identifies key regulators of flower and fruit development in wild strawberry. Plant Physiol. 2018;178(1):202–216. doi:10.1104/pp.18.00086.
- Reimand J, Isserlin R, Voisin V, Kucera M, Tannus-Lopes C, Rostamianfar A, Wadi L, Meyer M, Wong J, Xu C, et al. Pathway enrichment analysis and visualization of omics data using g: profiler, gsea, Cytoscape and enrichmentmap. Nat Protoc. 2019;14(2):482–517. doi:10.1038/s41596-018-0103-9.
- Wu T, Hu E, Xu S, Chen M, Guo P, Dai Z, Feng T, Zhou L, Tang W, Zhan L, et al. Clusterprofiler 4.0: a universal enrichment tool for interpreting omics data. Innov. 2021;2(3):100141. doi:10.1016/j.xinn.2021.100141.
- Ashburner M, Ball CA, Blake JA, Botstein D, Butler H, Cherry JM, Davis AP, Dolinski K, Dwight SS, Eppig JT, et al. Gene ontology: tool for the unification of biology. Nat Genet. 2000;25(1):25–29. doi:10.1038/75556.
- Wattam AR, Abraham D, Dalay O, Disz TL, Driscoll T, Gabbard JL, Gillespie JJ, Gough R, Hix D, Kenyon R, et al. Patric, the bacterial bioinformatics database and analysis resource. Nucleic Acids Res. 2014;42(D1):D581–D591. doi:10.1093/nar/gkt1099.
- Shannon P, Markiel A, Ozier O, Baliga NS, Wang JT, Ramage D, Amin N, Schwikowski B, Ideker T. Cytoscape: a software environment for integrated models of biomolecular interaction networks. Genome Res. 2003;13(11):2498–2504. doi:10.1101/gr.1239303.
- Yu G, Wang L-G, Han Y, He Q-Y. Clusterprofiler: an R package for comparing biological themes among gene clusters. Omics. 2012;16(5):284–287. doi:10.1089/omi.2011.0118.
- Carlson M. 2019. Org. eck12. eg. db: genome wide annotation for e coli strain k12. R package version 3.8. 2.
- Uniprot. (n.d.). https://www.uniprot.org/
- Bäumler AJ, Sperandio V. Interactions between the microbiota and pathogenic bacteria in the gut. Nature. 2016;535(7610):85–93. doi:10.1038/nature18849.
- Turner NC, Connolly JP, Roe AJ. Control freakssignals and cues governing the regulation of virulence in attaching and effacing pathogens. Biochem Soc Trans. 2019;47(1):229–238. doi:10.1042/BST20180546.
- Alteri CJ, Smith SN, Mobley HL, Galán JE. Fitness of Escherichia coli during urinary tract infection requires gluconeogenesis and the TCA cycle. PLoS Pathog. 2009;5(5):e1000448. doi:10.1371/journal.ppat.1000448.
- Xu X, Chen J, Huang X, Feng S, Zhang X, She F, Wen Y. The role of a dipeptide transporter in the virulence of human pathogen, helicobacter pylori. Front Microbiol. 2021;12. doi:10.3389/fmicb.2021.633166.
- Lu X, Fu E, Xie Y, Jin F, Young VB. Electron acceptors induce secretion of enterotoxigenic Escherichia coli heat-labile enterotoxin under anaerobic conditions through promotion of gspd assembly. Infect Immun. 2016;84(10):2748–2757. doi:10.1128/IAI.00358-16.
- Masuda N, Church GM. Escherichia coli gene expression responsive to levels of the response regulator evga. J Bacteriol. 2002 11;184(22):6225–6234. doi:10.1128/JB.184.22.6225-6234.2002.
- Lamarre S, Frasse P, Zouine M, Labourdette D, Sainderichin E, Hu G, Le Berre-Anton V, Bouzayen M, Maza E. Optimization of an RNA-seq differential gene expression analysis depending on biological replicate number and library size. Front Plant Sci. 2018;9:108. doi:10.3389/fpls.2018.00108.
- Yang B, Bao W, Chen B. Pgrnig: novel parallel gene regulatory network identification algorithm based on gpu. Brief Funct Genomics. 2022;21(6):441–454. doi:10.1093/bfgp/elac028.