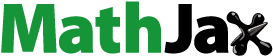
ABSTRACT
The gut microbiota is vital for human body development and function. Its development in early life is influenced by various environmental factors. In this randomized controlled trial, the gut microbiota was obtained as a secondary outcome measure in a study on the effects of one hour of daily skin-to-skin contact (SSC) for five weeks in healthy full-term infants. Specifically, we studied the effects on alpha/beta diversity, volatility, microbiota maturation, and bacterial and gut-brain-axis-related functional abundances in microbiota assessed thrice in the first year. Pregnant Dutch women (n = 116) were randomly assigned to the SSC or care-as-usual groups. The SSC group participants engaged in one hour of daily SSC from birth to five weeks of age. Stool samples were collected at two, five, and 52 weeks and the V4 region was sequenced. We observed significant differences in the microbiota composition, bacterial abundances, and predicted functional pathways between the groups. The SSC group exhibited lower microbiota volatility during early infancy. Microbiota maturation was slower in the SSC group during the first year and our results suggested that breastfeeding duration may have partially mediated this relation. Our findings provide evidence that postpartum SSC may influence microbiota development. Replication is necessary to validate and generalize these results. Future studies should include direct stress measurements and extend microbiota sampling beyond the first year to investigate stress as a mechanism and research SSC’s impact on long-term microbiota maturation trajectories.
Introduction
The human gastrointestinal tract is inhabited by a complex population of bacteria. These bacteria allow the digestion of dietary fibers, providing absorption of nutrients and energy.Citation1 They play an important role in intestinal integrity and immune functioning.Citation2,Citation3 In addition, gut bacteria can influence the brain via the gut-brain axis, a bidirectional pathway between the gut and the brain.Citation4,Citation5 The complex mechanisms underlying this bidirectional communication are still subject of study and have been thoroughly summarized elsewhere.Citation4 Briefly, the gut microbiota can influence human physical and mental development via the immune system, tryptophan metabolism, the vagus nerve and the enteric nervous system. This communication involves microbial metabolites such as short-chain fatty acids, branched chain amino acids and peptidoglycans.
During the early stages of life, the gut microbiota and other co-evolving systems are particularly sensitive to environmental disturbances. Furthermore, the establishment of a healthy gut microbiota during early life is important for the functioning of other systems, such as the immune system.Citation6,Citation7 Therefore, it is important to understand how the gut microbiota develops and how it is influenced during infancy. The present study investigated the potential effects of a randomized controlled trial (RCT) involving daily skin-to-skin contact (SSC) between mothers and their full-term infants on the developing gut microbiome.
The fetal gut is thought to be virtually sterile, although the sterility of the intrauterine environment and meconium is still subject of debate.Citation8 Starting from birth, the mother is the infant’s main source of microbial gut colonization via vaginal delivery, breastfeeding, and frequent close contact. This transmission of bacteria will remain detectable even at older ages.Citation9–11 Subsequently, other household members, close contacts, and pets may become sources of bacteria.Citation11,Citation12 The infant’s gut microbiota starts to increase in diversity and develops toward an adult-like microbiota as solid foods are introduced and breastfeeding is cessated,Citation13 although important changes are still seen in middle childhood.Citation14 Finally, infant health, use of antibiotics, hygiene, and infant genetics are important factors that contribute to the development of the gut microbiome.Citation9,Citation15
During SSC, the naked infant, dressed only in a diaper, is placed on the bare chest of the mother.Citation16 SSC can be considered de-stressingCitation17–19 and has been shown to be beneficial for both mother and infant. In preterm infants, SSC immediately after birth is associated with improved health outcomes, as well as a reduced mortality rate for the infant, and improved caregiving behavior and lower postpartum depression for the mother.Citation20–29 After the first postnatal hours, daily SSC with pre-terms is associated with better physical outcomes and improved development of the brain and the cardiovascular system.Citation22,Citation30,Citation31 Interestingly, the effect of daily SSC on cognitive function in pre-terms was still seen in young adulthood.Citation32,Citation33 While the focus of these studies remains on preterm infants, some studies have investigated full-term infants, although these studies are mostly limited to SSC in the first hours after birth. They have indicated several benefits of SSC right after birth, such as improved cardiovascular health, improved sleep, and weight gain.Citation19,Citation29 Concerning the mother, SSC performed on full-term infants right after birth is associated with a decrease in anxiety and longer breastfeeding duration.Citation29,Citation34,Citation35 Prolonged, daily SSC is associated with a reduction in maternal depressive symptoms,Citation36 anxiety and stress.Citation37 The SKIPPY study, of which this current study is part, was the first to perform an RCT to study an SSC intervention on both maternal and infant outcomes in a healthy, full-term sample.Citation38 The study showed that performing a daily hour of SSC during the first five postnatal weeks may reduce maternal anxiety and fatigue symptoms, increase infant sleep and reduce infant crying, and extend exclusive and continued breastfeeding durations.Citation39–41 At three years of age, the children that had received the SSC intervention also showed fewer internalizing and externalizing behavioral problems.Citation42 However, the results did not indicate that this daily hour of SSC influenced mother-infant interaction quality and maternal depressive, stress, and pain symptoms, as was found in earlier intervention studies.Citation39,Citation43
There are multiple pathways in which mother-infant SSC could potentially influence the infant gut microbiome. Firstly, as mentioned before, a daily hour of SSC was associated with fewer maternal anxiety and fatigue symptoms.Citation39 Previous studies have indicated a link between maternal postnatal distress (including anxiety) and breast milk microbiota, which in turn could influence the gut microbiome of breastfed infants.Citation44,Citation45 Secondly, breastfeeding shapes the infant gut microbiome.Citation46 The extension of exclusive and continued breastfeeding duration caused by a daily hour of SSC could therefore influence the infant gut microbiome. Third, SSC may also be de-stressing for infants.Citation17–19 Stress during the early developmental stages has been found to influence the gut microbiota and gut-brain axis in rodents.Citation47,Citation48 Therefore, a de-stressing practice such as SSC could potentially alter the colonization of the gut.Citation49–51 Lastly, SSC could also provide an additional opportunity for the exchange of microbes between the mother and child. Previous studies have shown that constant contact between microbial communities increases their similarityCitation52,Citation53 and the maternal skin microbiome has been shown to provide a source of bacteria for infants.Citation54,Citation55
To the best of our knowledge, there have been no previous studies on the effects of SSC on the infant gut microbiome. The current study investigated the effects of a five-week daily hour SSC between mothers and full-term infants, compared to care-as-usual (CAU), on the infant gut microbiome. We hypothesized that the treatment and the control group differ in (1) alpha diversity, (2) beta diversity, (3) genus level abundances, (4) volatility, (5) microbiota age and (6) functional pathways that are related to gut-brain communication. While most hypotheses are non-directional, we hypothesized that SSC infants have less volatile microbiota, since stress has been shown to increase gut microbiota volatility.Citation56
Results
Descriptive statistics
Participation in stool sample collection and eligibility resulted in 116 participants (). For one stool sample, PCR amplification did not provide enough material for sequencing. While there was no loss to follow-up in this RCT, some mothers did not provide stool samples for all time points leading to a total of 315 analyzed samples: 105 at week two, 107 at week five and 103 at week 52. Across all time points, 11 phylum-level groups and 162 genus-level groups were identified. The SSC group provided longer total SSC duration (intention-to-treat (ITT): 2067.67 ± 850.65 min; per protocol (PP): 2905.90 ± 497.52 min) than the CAU group (ITT: 308.17 ± 442.41; PP: 308.17 ± 442.41) according to independent sample t-tests (p < 0.001). The average daily SSC duration between groups is furthermore depicted in . An overview of the baseline characteristics of the infants and their mothers, including information on missing data, is presented in . In the following, we present for each preregistered feature of the microbiota the results of the ITT analysis and in case of meaningful deviation, the PP analysis. Relevant parameter estimates, such as β-coefficients of the Bayesian robust linear models, are mentioned in the text. To inspect all model coefficients, see supplementary Figures S1–6.
Figure 1. Participant flow diagram, including the number of participants at each of the trial stages. SSC = skin-to-skin contact. CAU = care-as-usual.

Figure 2. Mean daily skin-to-skin contact (SSC) duration in the SSC and care-as-usual (CAU) condition based on data derived from the intention-to-treat selection.

Table 1. Descriptive statistics and group comparisons of raw data for mother – infant dyads of the SKPPY study.
Main results
Alpha diversity
Our results indicated that SSC had no effect on alpha diversity across different alpha diversity indices, time points, and ITT and PP analyses (). Infants with siblings had lower Shannon alpha diversity scores ( = −0.31, 95% HDI [−0.52;-0.09],
= 0.997) in early infancy (2 and 5 week samples), but no longer in late infancy (1 year samples). Results regarding siblings were similar for Chao1 and the inverse Simpson, but the difference disappeared mostly when the Faith index was used.
Beta diversity
shows the results of a PCA of the centered-log-ratio transformed abundances (Aitchison distance). Samples obtained at one year (triangles) were clearly separated from samples obtained at two and five weeks. There seems to be no apparent separation of SSC and CAU samples considering all samples, also not when other principal components were inspected (not shown here). Within the 1-year samples (triangles), the SSC samples (gray) may occur more frequently closer to the early infancy samples than the CAU samples. Statistically, PERMANOVA identified that the microbiota composition of CAU and SSC samples differed significantly in the early infancy samples (p = 0.016), but not in the late infancy samples (p = .087). To derive the direct (rather than the total) effect of SSC under the assumed directed acyclic graph (Figure S9), we fitted new models including breastfeeding as a covariate. The effect remained significant, and the effect size unchanged, suggesting that SSC has an effect on microbiota composition independent of its effect on breastfeeding duration. In the PP analyses, the effect size increased slightly, but the standard error also increased because of the lower sample size, leading to a non-significant effect (p = .058). Note also that the result was sensitive to the choice of another distance metric. When using Bray Curtis similarity, the main effect was no longer significant (p = .377) while the interaction term suggested that SSC may have only had an effect on the microbiota at 5 weeks (p = .069). Using Bray Curtis similarity, SSC only showed a significant effect in the PP analyses when fitting a model to all samples (p = .035).
Genus level abundances
Because recent benchmark studies have shown that differential abundance analysis results can depend heavily on the specific method that is applied,Citation57,Citation58 we used several methods to evaluate the robustness of findings across methods. While we focus on the results of MaAsLin2 in this section, indicates whether a taxon was identified by one or more methods (denoted as M (MaAsLin2), L (LinDA) or A (ANCOMBC), respectively). MaAsLin2 detected a lower relative abundance of Faecalibacterium, Eubacterium hallii, and Rothia and higher abundance of Flavonifractor, Lacticaseibacillus, Bacteroides and Megasphaera in the SSC group compared to the CAU group. The top six genera in stand out, as they were identified as drivers of differences between groups in at least two methods. From the heatmap, we can further infer that some genera were only differentially abundant in either early or late infancy. Note that ANCOMBC, the most conservative method, did not detect any genera that were differentially abundant.
Figure 5. Heatmap of clr-transformed bacterial abundances that were either differentially abundant between treatment (SSC) and control (CAU) or important for the microbiota age model. The letter behind each genus indicates whether it was important for the microbiota age model (R, see corresponding section) or identified by any of the differential abundance analysis methods (M = Maaslin2, L = LinDA). The relative abundance values have been scaled to zero mean and unit variance in order to highlight differences in the variation relative to the mean level within each taxonomic group. The color scale has been limited to the interval [−1, 1].
![Figure 5. Heatmap of clr-transformed bacterial abundances that were either differentially abundant between treatment (SSC) and control (CAU) or important for the microbiota age model. The letter behind each genus indicates whether it was important for the microbiota age model (R, see corresponding section) or identified by any of the differential abundance analysis methods (M = Maaslin2, L = LinDA). The relative abundance values have been scaled to zero mean and unit variance in order to highlight differences in the variation relative to the mean level within each taxonomic group. The color scale has been limited to the interval [−1, 1].](/cms/asset/b085272c-6a56-413e-8f2c-bb1cf4f0d0e9/kgmi_a_2295403_f0005_oc.jpg)
Microbiota volatility
Microbiota volatility is defined as the intra-individual change in microbiota composition over time and was calculated as described by Bastiaanssen et al.Citation59 We fitted Bayesian robust linear models to the volatility scores in early infancy (2–5 weeks) and from early to late infancy (5–52 weeks) (). Volatility was lower in the SSC group in early infancy than in the CAU group ( = −0.31, 95% HDI [−0.62;0],
= 0.95), rejecting the null hypothesis that SSC has no influence on microbiota volatility. This effect remained unchanged after including breastfeeding in the regression model, suggesting that SSC affects microbiota volatility in early infancy, independent of its effect on breastfeeding. Note that in the PP analyses for early infancy, the effect size increased slightly, whereas the highest density interval widened with a smaller sample size(
= −0.4, 95% HDI [−0.88;0.09],
= 0.92). While average volatility was also lower in the SSC group when looking at the distances between the 5 and 52 weeks samples, the effect did not pass the decision criterion (
= −0.16, 95% HDI [−0.47;0.15],
= 0.80) to reject the null hypothesis. Breastfeeding (
= −0.13, 95% HDI [−0.22;-0.03],
= 0.99) and gestational age (
= −0.25, 95% HDI [−0.46;-0.04],
= 0.99) were negatively related to volatility (breastfeeding only for volatility between 5 and 52 weeks).
Microbiota age
Microbiota age was estimated as described by Subramanian et al. by training a Random Forest model using samples from two other Dutch longitudinal studies (BIBO, BINGO) in the age range of 7–561 days ().Citation60 The Random Forest model performance was comparable to that of Subramanian et al.Citation60 as 64% of variance in age could be explained by the microbiota. We evaluated the model fit and significance by computing the correlation between predictions and actual ages using SKIPPY samples (r = 0.862, p < 0.001). Besides illustrating the results of the differential abundance analysis, shows the most important features of the RF model (top 15 with non-zero abundance in the SKIPPY samples; denoted by R next to the taxon name in ). To predict microbiota age, Faecalibacterium was the most informative next to other genera that are known to either dominate early infancy due to breastfeeding (Staphylococcus and Bifidobacterium) or to only occur with the cessation of breastfeeding and introduction of solid food as dominance of Bifidobacterium disappears. Note that we have lower coverage of the ages around 1 year in BIBO and BINGO compared to the coverage of ages in early infancy (), resulting in higher uncertainty in the estimation of the microbiota age reference compared to the time period early in infancy. Nevertheless, we could compare the microbiota ages of the treatment and control groups in the SKIPPY cohort. depicts the microbiota age scores at each time point between the treatment groups in the SKIPPY samples. The samples obtained at one year show relatively low microbiota age, indicating that the SKIPPY cohort had lower microbiota ages than the BIBO and BINGO cohorts. The Bayesian robust regression models indicated that treatment was associated with a lower microbiota age with an average decrease of 25.65 days ( = −25.65, 95% HDI [−50.37;-1.31],
= 0.98) at one year of age. After adding breastfeeding, the effect size decreased (
= −19.32, 95% HDI [−47.90;8.01],
= 0.90)) while breastfeeding was associated with lower microbiota age, as expected (
= −7.27, 95% HDI [−13.94;-0.62],
= 0.98). These results indicate that the SSC intervention influenced microbiota development with visible effects at 1 year of age. Breastfeeding duration may have partially mediated this effect. An exploratory dose-response analysis within only the treatment group indicated that there was no stronger effect on microbiota age when subjects provided more hours of SSC. Thus, infants in the treatment group were probably mostly above the minimal number of hours needed to produce the observed effect on microbiota age (Figure S7).
Gut brain module abundances
We estimated 44 metabolic pathways related to gut-brain communication (gut brain modules).Citation61 These pathways include, for example, serotonin degradation or histamine production. Using MaAsLin2 (), we found that nitric oxide degradation was lower (FDR = 0.166), while butyrate synthesis I (FDR = 0.169) and acetate synthesis III (FDR = 0.148) were higher in the SSC group compared to the CAU group (Figure S8).
Discussion
We hypothesized that a skin-to-skin intervention (SSC) applied in the first weeks of life in full-term infants would influence features of the gut microbiota via several potential mechanisms. These include physical transmission,Citation55 prolonged breastfeedingCitation40 and by having a stress-reducing, stabilizing effect on the developing infant.Citation17–19 The microbiota features that were investigated included alpha-diversity, beta-diversity, genus level abundances, volatility, microbiota age and abundances of selected predicted functional pathways (gut brain modules; GBMs). The results provide evidence that a postpartum skin-to-skin intervention may influence gut microbiota development.
Lower microbiota volatility in the SSC group
Infants that received the SSC intervention significantly differed in their overall microbiota composition and had lower microbiota volatility in early infancy. Volatility has been found to be higher in stressed mice, in humans reporting higher experienced stress during exam periods, and in patients with inflammatory bowel disease.Citation56,Citation62 In addition, a related metric indicated higher volatility (lower stability) in infants with colic.Citation63 We furthermore observed that higher gestational age was associated with lower volatility in both time windows, possibly indicating that infants who are further in their physical development for their chronological age have more stable gut microbiota.
Lower microbiota age in the SSC group
Infants in the SSC group had a lower microbiota age at one year of age compared with the control group. Our results further indicated that breastfeeding may have partially mediated this effect of SSC on microbiota age, which is in line with previous research showing that breastfeeding duration is negatively associated with microbiota maturation.Citation13,Citation64 However, after controlling for months of exclusive breastfeeding or alternatively, age at weaning, SSC infants still had, on average, 19 or 15 days, respectively, lower microbiota age. In addition to breastfeeding, lower microbiota age has been associated with exposure to antibiotics, delivery via cesarean section,Citation65 and asthma.Citation64 We found that siblings and educational level were positively associated with microbiota age at one year. The former is in line with previous research showing that infants with older siblings show faster maturation of the microbiotaCitation66 and specifically earlier colonization with Faecalibacterium prausnitzii.Citation67 Colonization with Faecalibacterium prausnitzii is a characteristic of microbiota maturationCitation68 and this taxon was the most important feature in our microbiota age model.
It is important to note that environmental factors appear to alter the dynamics of microbiota maturation rather than the speed of maturation. For example, formula-fed infants or infants born via cesarean section showed initially higher microbiota ages (in the first 6–12 months) before they started to show lower or similar microbiota ages compared to breastfed or vaginally born infants at later developmental stages.Citation65 A reverse dynamic was observed for antibiotic exposure with initially lower and later temporarily higher microbiota age compared to individuals not exposed to antibiotics.Citation65 The diverse associations with microbiota age raise the question of which specific aspects of microbiota maturation at which specific ages may be beneficial or possibly unfavorable for healthy development.
To investigate this further, it would be desirable for future research to utilize a common microbiota age model trained on large samples for the respective geographic region. Combined with sampling for a longer time span (e.g., the first three years), it may be possible to describe different trajectories of microbiota maturation that may be related to environmental variables, and in longitudinal follow-ups, to health outcomes in later life. Finally, while increasing alpha diversity is a characteristic of microbiota maturation, we did not observe any differences in alpha diversity between the groups. Our observation that siblings was associated with lower alpha diversity and with higher microbiota age might seem contrary, but note that having one or more siblings was only negatively associated with alpha diversity in early infancy and not at 1 year of age, in line with findings in another Dutch cohortCitation12 as well as with those of other studies.Citation69–72
Differential abundance of individual genera between groups
Differential abundance analysis highlighted several genera (Faecalibacterium, Megasphaera, Bacteroides, Flavonifractor, Rothia and Eubacterium hallii) as well as predicted functional pathways (nitric oxide degradation, butyrate synthesis I, and acetate synthesis III) as differentially abundant between the groups. Faecalibacterium prausnitzii is well known as a butyrate producer, and because of that, and its negative relationship with inflammatory bowel diseases, it is generally considered a health-promoting species.Citation67,Citation73 Laursen et al.Citation67 found that the prevalence was between approximately 55% and 80% at 9–10 months of age and increased to almost 100% at 16 months of age in three separate infant populations. The abundance of Faecalibacterium increased substantially after 1 year of age, but no longer from 3 to 5 years of age.Citation67,Citation68 In our study sample, the prevalence of Faecalibacterium at 12 months of age was 86.8% for the CAU and 68.0% for the SSC group. This finding, combined with the previously discussed findings of the microbiota age model, indicates a slower speed of microbiota maturation in the SSC group along a normal trajectory of microbiota development until the first year of life. This is supported by the fact that while the microbiota of infants typically matures along similar trajectories, differences in speed between individuals have been documented.Citation68
In contrast to Faecalibacterium, the abundance of Bacteroides was higher in the SSC group. Higher abundance at 1 year of age has previously been associated with enhanced neurodevelopment.Citation74 Megasphaera was more abundant in the SSC group. This genus includes butyrate- and propionate-producing strainsCitation75 and has been negatively associated with diarrheal cryptosporidiosisCitation76 and maternal stress.Citation77 However, the relationship with maternal stress was only present at 6 weeks and 3 months of age and reversed at 6 months of age. Flavonifractor, also higher in the SSC group, has been negatively associated with the development of asthma later in lifeCitation78 and was able to strongly suppress Th2 immune responses in mice.Citation79 Furthermore, Flavonifractor plautii was negatively associated with maternal stress in samples obtained at 6 weeks, 3 months, and 6 months.Citation77 Rothia, with a slightly lower relative abundance in the SSC group, has been reported to be increased in formula-fed infants in two studies (one study found the opposite)Citation80 and to be negatively associated with childhood asthma.Citation81 Lastly, Eubacterium hallii, reclassified as Anaerobutyricum soehngenii, Citation82 was lower in the SSC group. Anaerobutyricum soehngenii can produce butyrate by utilizing the byproducts of other early colonizers that metabolize human milk oligosaccharides.Citation83,Citation84 It has been positively associated with infant colicCitation85 and is under investigation for its benefits in relation to glucose metabolism.Citation86
Summary and conclusion
We found evidence that SSC affected microbiota composition in early infancy (2 and 5 weeks) and development in early and late infancy, as measured by volatility and microbiota age. Differential abundance and microbiota age analyses highlighted individual genera that differed between the groups. We proposed three mechanisms of action through which SSC may affect the microbiota. First, the transmission route appeared least relevant to the observed effects. Skin bacteria such as Staphylococcus were not differentially abundant between the groups. Also, the groups did not yet differ in the number of exclusively breastfed infants at five weeks (80.2% vs. 78.4%). Differences in breastfeeding were observed only at later ages. Thus, we can assume that physical contact, which occurs naturally through maternal caregiving and breastfeeding, was sufficient to transmit bacteria that were also transmitted through increased direct physical contact by the SSC intervention. Furthermore, previous research has demonstrated that most skin bacteria obtained from the mother are mainly detectable shortly after delivery but not at later time pointsCitation55 as they do not colonize the gut. Second, our results suggested that breastfeeding may be one of the mechanisms by which the SSC intervention altered microbiota maturation long after the intervention, at one year of age. Third, considering prior evidenceCitation56 and the lack of differences in breastfeeding in early infancy, we hypothesize that the effect of SSC on volatility in early infancy may be due to its de-stressing effect.Citation17–19 Future longitudinal studies would need to measure stress in addition to the measures we collected in order to investigate the proposed mechanism of SSC influencing microbiota volatility by acting as a stress buffer.
This preregistered study is the first RCT to assess the effects of SSC on the developing gut microbiota. Strengths include a randomized controlled design with blind recruitment and a low dropout rate throughout the intervention phase. In addition, microbiota and relevant covariates were sampled at several time points and included a follow-up microbiota sample long after the intervention was finalized. This allowed us to estimate the effect of SSC on microbiota development and explore one possible mechanism. Furthermore, we investigated a broad set of features of the microbiota and took measures to ensure the robustness of the results. Our analyses revealed that it is important to look beyond alpha diversity, beta diversity, and differential abundance analysis and include features that reflect microbiota development (volatility and microbiota age). Our study has some limitations. Since we did not measure all (time-varying) variables that influence protocol adherence, we may not exclude potential confounding bias in the per-protocol analysis estimates.Citation87,Citation88 Note however, this limitation is not relevant for our intention-to-treat analysis, which is regarded as the preferred analytic approach for RCTs. Finally, the generalizability of the study is limited, given the relatively homogeneous sample with mainly families of highly educated mothers.
In conclusion, we provide evidence that a postpartum skin-to-skin intervention in full-term infants may influence gut microbiota composition and volatility in early infancy, as well as microbiota age (for chronological age) in late infancy. The effects on microbiota age may have been partially mediated by SSC prolonging breastfeeding duration. It is highly desirable to replicate these findings to validate their robustness and establish their generalizability. Future studies would benefit by extending microbiota sampling beyond the first year of life to investigate the effect of SSC on microbiota maturation at later time points. Including variables that measure stress would allow for the investigation of whether the intervention has an effect on microbiota volatility by reducing stress.
Methods
Study design
This RCT included two parallel groups: an intervention group and a passive control.Citation38 Ethics approval was granted by the ethics committee of the Social Science Faculty of Radboud University (ECSW2015–2311–358). This study was registered in the Netherlands Trial Register (NTR5697). It followed CONSORT guidelines and the protocol was published.Citation38
Participants
Expectant mothers from the Nijmegen region were recruited between April 2016 and September 2017. Recruitment was performed with the help of a database of pregnant mothers who expressed interest in participating in scientific research, as well as via promotions at pregnancy clubs, baby fairs, and baby shops. Prenatal characteristics were examined during the last trimester of pregnancy, using an eligibility survey. Expectant women were eligible to participate when they were at least 18 years old, had good physical and mental health, had a singleton pregnancy, were not using drugs during pregnancy, and had sufficient understanding of the Dutch language. After the birth of their child, mothers were excluded if their child was born before 37 weeks, had congenital anomalies, a birth weight less than 2500 g, and/or a 5-minutes Apgar score of < 7.
Procedure
Prenatal
Detailed study information was provided to eligible women during a home visit between weeks 34–36 of gestation. After informed consent was obtained, only mothers allocated to the SSC group were encouraged to engage in at least one uninterrupted daily hour of SSC, starting immediately after birth until including the fifth postnatal week (Dutch mothers are entitled to 10 to 12 weeks of paid leave after birth). Detailed written and oral instructions about the SSC protocol, optimal SSC position and safety were provided. CAU mothers received no additional information, and all mothers were encouraged to contact the principal investigator when experiencing any problems during the study by phone, text message or e-mail. Besides SSC, both conditions underwent the same procedures.
Postnatal
All mothers reported daily information on SSC, holding (clothed physical contact), and no-contact in 15-min intervals every 2-3 h in a logbook throughout the 5-week intervention period. All mothers were contacted weekly by telephone (postnatal day 5 and 13) or text-message/e-mail (postnatal day 21 and 28) to remind mothers to complete the logbook, ask for questions/comments and, for SSC mothers, to discuss SSC obstacles. When their child was two weeks, five weeks and one year of age, parents were instructed to collect a fecal sample. The feces were collected directly from the first diaper that day, with the help of a provided plastic scoop. Parents were instructed to avoid any contact with surfaces or humans and put two or three scoops into a sterilized plastic tube. In addition to that, a stool questionnaire was completed, providing information on date of collection and infant health. After being temporarily stored in the home freezer (−20 degrees Celsius), the samples were collected during the home visits at 5 weeks and 1 year and then stored at −80 degrees Celsius. During the same home visits, questionnaires were obtained with information on covariates, such as gestational age, birth mode and feeding patterns.
DNA extraction and processing to microbiota features
The samples were transported to the Laboratory of Microbiology at Wageningen University and stored at −80°C until they were processed for DNA extraction as published previouslyCitation89 and described in the following: DNA extraction was carried out with the Maxwell 16 TOTAL RNA system (Promega, Wisconsin, USA) in conjunction with Stool Transport and Recovery Buffer (STAR; Roche Diagnostics Corporation, Indianapolis, IN). The V4 region of the 16S ribosomal RNA (rRNA) gene was amplified in duplication, generating amplicons of approximately 290bp. PCR reactions comprised 0.5 µl Phusion Green Hot Start II High-Fidelity DNA polymerase (Thermo Scientific, US) at 2 U/µl, 1 µl of 10um barcoded primers 515F-n(5’-GTGYCAGCMGCCGCGGTAA-3’) and 806 R-n(5’- GGACTACNVGGGTWTCTAAT-3’), 10 µl 5×Phusion Green HF Buffer (Thermo Scientific, US), 1 µl of 10 mM dNTPs mix (Promega Corporation, US), 36.5 µl Nuclease-free water, and 1 µl of 20ng/µl DNA template. PCR involved an initial denaturation period of 30s at 98°C, followed by 25 cycles of denaturation (98°C, 10 s), annealing (50°C, 10 s), and extension (72°C, 10 s), concluding with a final elongation step (72°C, 7 min). PCR products were validated through gel electrophoresis and purified using the HighPrep® PCR kit (MagBio Genomics, Alphen aan den Rijn, Netherlands). DNA concentration was assessed using a fluorometer (DS-11; DeNovix) with the Qubit® dsDNA BR Assay Kit (Life Technologies, Leusden, Netherlands). Barcoded samples belonging to the same library (200 ng) were combined. Each library incorporated 69 unique barcode tags, with 2 specifically designed for artificial control communities representing human gut microbiota. The mixture underwent purification again to achieve a final volume of 40 µl using the HighPrep® PCR kit. Sequencing was performed on the Illumina platform at Eurofins Genomics in Germany. Data were pre-processed from raw genetic sequences to amplicon sequence variant (ASV) tables using the NGTax2 pipeline (version 2.1.74) with the SILVA database (version 138.1). The sequence data, together with the metadata, were stored in a TreeSummerizedExperiment (TreeSE) containerCitation90 for further analysis. To analyze whether the gut microbiota differed between the SSC and CAU groups, the different features of the infant gut microbiome were evaluated. For alpha diversity, we calculated Shannon, inverse Simpson, Faith, and Chao1 indices. For beta diversity, we calculated the Aitchison distance and Bray – Curtis similarity. For volatility, we calculated the Aitchison distance sequentially between intra-individual samples.Citation56 The microbiota age was determined as described by Subramanian et al.Citation60 and we controlled for age in the regression models. We used samples from other longitudinal studies (BIBO and BINGO) to train the Random Forest model. Functional pathways, specifically the gut-brain-module (GBM), were calculated as described by Bastiaanssen et al.Citation59
Statistical analysis
In the following section, we briefly describe the statistical analysis. For a more detailed description see the preregistration (https://doi.org/10.17605/OSF.IO/S45MU). All analyses were performed in R (version 4.2.1)Citation91 and the code was published (https://doi.org/10.5281/zenodo.8155205). Missing covariates and outcome variables were imputed using predictive mean matching (m = 50)Citation92 with the package mice in R.Citation93 Deviations of results from complete case analyses were to be reported. For each feature of the gut microbiota (alpha diversity, beta diversity, genus level abundance, volatility, microbiota age, and GBM), we performed intention-to-treat (ITT) and per-protocol (PP) analyses. We used Bayesian robust linear models to regress alpha diversity, volatility, and microbiota age on SSC and the covariates. These models were fit using the brms packageCitation94 with default priors and a Student’s t distribution for the response variable. For differential abundance analysis (genus level and GBMs), we utilized ANCOMBC,Citation95 LinDA,Citation96 and MaAsLin2.Citation97 Combining the three methods ensures robustness of the presented findings. Furthermore, we applied a false-discovery rate (FDR) of FDR < 0.2 to control false discoveries while also limiting false negatives. The adonis2 function from the vegan package was used for beta diversity analyses.Citation98 We accounted for non-independence by specifying random intercepts in models that included repeated samples of an individual. Different alpha diversity indices were calculated using the R package mia.Citation99
Covariates
In the ITT analyses, we can assume that randomization prevented confounding of the average causal effect estimate (Figure S9). Therefore, we only added covariates that may improve the precision of our estimate of interest in a data-driven manner.Citation100 Possible mediators of the effect of SSC, such as breastfeeding, had to be excluded to estimate the total effect of SSC.Citation100 Breastfeeding was only added to determine the magnitude of the direct effect of SSC on the microbiota once an effect of SSC was detected. If the direct effect is smaller than the total effect upon inclusion, while SSC is positively related to breastfeeding and breastfeeding is related to the microbiota, this would suggest a mediating role of breastfeeding under the assumed directed acyclic graph (Figure S9).Citation100 For the Bayesian models, we used leave-one-out cross-validationCitation101 to evaluate whether any of the following variables improved model fit per microbiome feature: C-section, birth weight, siblings (yes/no), sex, Apgar (at 5 minutes), gestational age at birth, and education level. To determine whether SSC had an effect across all samples and over time, we modeled SSC in interaction with age across the models that included the samples obtained at 2 and 5 weeks. When modeling the samples at 52 weeks, we omitted the interaction term. For the PP analyses, we did not use the data-driven approach because confounding of the total effect estimate is possible, and we needed to make stronger assumptions.Citation87,Citation88 Measured variables that may influence whether an individual actually received the treatment (irrespective of assigned treatment) would need to be included to avoid confounding. Here, we included the following additional covariates in the models: birth weight, gestational age at birth, education level, cesarean section, and sex. Sensitivity analyses were performed using complete case analyses, and all covariates were excluded from the ITT analyses. The results did not differ meaningfully unless reported otherwise.
Author contributions
The authors have made the following contributions. Henrik Eckermann: Original Draft Preparation, Data Analysis, Review & Editing; Jennifer Meijer: Original Draft Preparation, Data Analysis, Review & Editing; Kelly Cooijmans: Conceptualization, Review & Editing; Leo Lahti: Supervision, Review & Editing; Carolina de Weerth: Conceptualization, Review, & Editing, Supervision.
Supplemental Material
Download PDF (553.8 KB)Disclosure statement
No potential conflict of interest was reported by the author(s).
Data availability statement
The research data are part of an ongoing study but can be requested from C de Weerth ([email protected]) for scientific purposes.
Supplemental material
Supplemental data for this article can be accessed online at https://doi.org/10.1080/19490976.2023.2295403
Additional information
Funding
References
- Cerqueira FM, Photenhauer AL, Pollet RM, Brown HA, Koropatkin NM. Starch digestion by gut bacteria: crowdsourcing for carbs. Trends Microbiol. 2020;28(2):95–18. doi:10.1016/j.tim.2019.09.004.
- Gensollen T, Iyer SS, Kasper DL, Blumberg RS. How colonization by microbiota in early life shapes the immune system. Science. 2016;352:539–544.
- Zhang Y-J, Li S, Gan R-Y, Zhou T, Xu D-P, Li H-B. Impacts of gut bacteria on human health and diseases. Int J Mol Sci. 2015;16(12):7493–7519. doi:10.3390/ijms16047493.
- Cryan JF, O’Riordan KJ, Cowan CSM, Sandhu KV, Bastiaanssen TFS, Boehme M, Codagnone MG, Cussotto S, Fulling C, Golubeva AV, et al. The microbiota-gut-brain axis. Physiol Rev. 2019;99(4):1877–2013. doi:10.1152/physrev.00018.2018.
- Cryan JF, Dinan TG. Mind-altering microorganisms: the impact of the gut microbiota on brain and behaviour. Nat Rev Neurosci. 2012;13:701–712.
- Claesson MJ, Jeffery IB, Conde S, Power SE, O’Connor EM, Cusack S, Harris HMB, Coakley M, Lakshminarayanan B, O’Sullivan O, et al. Gut microbiota composition correlates with diet and health in the elderly. Nature. 2012;488:178–184.
- Sekirov I, Russell SL, Antunes LCM, Finlay BB. Gut microbiota in health and disease. Physiol Rev. 2010;90(3):859–904. doi:10.1152/physrev.00045.2009.
- Milani C, Duranti S, Bottacini F, Casey E, Turroni F, Mahony J, Belzer C, Delgado Palacio S, Arboleya Montes S, Mancabelli L, et al. The first microbial colonizers of the human gut: composition, activities, and health implications of the infant gut microbiota. Microbiol Mol Biol R. 2017;81:e00036–17.
- Van Daele E, Knol J, Belzer C. Microbial transmission from mother to child: improving infant intestinal microbiota development by identifying the obstacles. Crit Rev Microbiol. 2019;45(5–6):613–648. doi:10.1080/1040841X.2019.1680601.
- Yang L, Sakandar HA, Sun Z, Zhang H. Recent advances of intestinal microbiota transmission from mother to infant. J Funct Foods. 2021;87:104719.
- Valles-Colomer M, Blanco-Míguez A, Manghi P, Asnicar F, Dubois L, Golzato D, Armanini F, Cumbo F, Huang KD, Manara S, et al. The person-to-person transmission landscape of the gut and oral microbiomes. Nature. 2023;614(7946):125–135. doi:10.1038/s41586-022-05620-1.
- Hermes GDA, Eckermann HA, de Vos WM, de Weerth C. Does entry to center-based childcare affect gut microbial colonization in young infants? Sci Rep. 2020;10:10235.
- Bäckhed F, Roswall J, Peng Y, Feng Q, Jia H, Kovatcheva-Datchary P, Li Y, Xia Y, Xie H, Zhong H, et al. Dynamics and stabilization of the human gut microbiome during the first year of life. Cell Host & Microbe. 2015;17(5):690–703. doi:10.1016/j.chom.2015.04.004.
- Ou Y, Belzer C, Smidt H, de Weerth C. Development of the gut microbiota in healthy children in the first ten years of life: associations with internalizing and externalizing behavior. Gut Microbes. 2022;14:2038853.
- Korpela K, Zijlmans MAC, Kuitunen M, Kukkonen K, Savilahti E, Salonen A, De Weerth C, De Vos WM. Childhood BMI in relation to microbiota in infancy and lifetime antibiotic use. Microbiome. 2017;5(1):26. doi:10.1186/s40168-017-0245-y.
- WHO. Kangaroo mother care: a practical guide. Geneva: World Health Organization; 2003.
- Beijers R, Cillessen L, Zijlmans MAC. An experimental study on mother-infant skin-to-skin contact in full-terms. Infant Behav Dev. 2016;43:58–65.
- Cristóbal Cañadas D, Parrón Carreño T, Sánchez Borja C, Bonillo Perales A. Benefits of Kangaroo mother care on the physiological stress parameters of preterm infants and mothers in neonatal intensive care. Int J Envir Res & Pub Health. 2022;19(12):7183. doi:10.3390/ijerph19127183.
- Ionio C, Ciuffo G, Landoni M. Parent and stress Regulation: a systematic Review of the literature. Int J Envir Res & Pub Health. 2021;18:4695.
- Bergman N, Linley L, Fawcus S. Randomized controlled trial of skin-to-skin contact from birth versus conventional incubator for physiological stabilization in 1200- to 2199-gram newborns. Acta Paediatr. 2004;93(6):779–785. doi:10.1111/j.1651-2227.2004.tb03018.x.
- Conde-Agudelo A, Díaz-Rossello JL. Kangaroo mother care to reduce morbidity and mortality in low birthweight infants. Cochrane Database Syst Rev. 2016;2017.
- Feldman R, Rosenthal Z, Eidelman AI. Maternal-preterm skin-to-skin contact enhances child physiologic organization and cognitive control across the first 10 years of life. Biol Psychiatry. 2014;75(1):56–64. doi:10.1016/j.biopsych.2013.08.012.
- Meder U, Tarjanyi E, Kovacs K, Szakmar E, Cseko AJ, Hazay T, Belteki G, Szabo M, Jermendy A. Cerebral oxygenation in preterm infants during maternal singing combined with skin-to-skin care. Pediatr Res. 2021;90(4):809–814. doi:10.1038/s41390-020-01235-2.
- WHO Immediate KMC Study Group. Immediate “Kangaroo mother care” and survival of infants with low birth weight. N Engl J Med. 2021;384(21):2028–2038. doi:10.1056/NEJMoa2026486.
- de Alencar AEMA, Arraes LC, de Albuquerque EC, Alves JGB. Effect of Kangaroo mother care on postpartum depression. J Trop Pediatr. 2007;55(1):36–38. doi:10.1093/tropej/fmn083.
- Dombrowski MAS, Anderson GC, Santori C, Burkhammer M. Kangaroo (skin-to-skin) care with a postpartum Woman who Felt Depressed. MCN: Am J Maternal/Child Nurs. 2001;26(4):214–216. doi:10.1097/00005721-200107000-00012.
- Feldman R, Eidelman AI, Sirota L, Weller A. Comparison of skin-to-skin (Kangaroo) and traditional care: parenting outcomes and preterm infant development. Pediatrics. 2002;110(1):16–26. doi:10.1542/peds.110.1.16.
- Sinha B, Sommerfelt H, Ashorn P, Mazumder S, Taneja S, More D, Bahl R, Bhandari N. Effect of community-initiated Kangaroo mother care on postpartum depressive symptoms and stress among mothers of low-birth-weight infants: a randomized clinical trial. JAMA Netw Open. 2021;4(4):e216040. doi:10.1001/jamanetworkopen.2021.6040.
- Moore ER, Bergman N, Anderson GC, Medley N. Early skin-to-skin contact for mothers and their healthy newborn infants. Cochrane Database Syst Rev. 2016;2016(11):2016. doi:10.1002/14651858.CD003519.pub4.
- Feldman R, Eidelman AI. Skin-to-skin contact (Kangaroo care) accelerates autonomic and neurobehavioural maturation in preterm infants. Dev Med Child Neurol. 2003;45(04). doi:10.1017/S0012162203000525.
- Föhe K, Kropf S, Avenarius S. Skin-to-skin contact improves gas exchange in premature infants. J Perinatol. 2000;20(5):311–315. doi:10.1038/sj.jp.7200378.
- Charpak N, Tessier R, Ruiz JG, Hernandez JT, Uriza F, Villegas J, Nadeau L, Mercier C, Maheu F, Marin J, et al. Twenty-year follow-up of Kangaroo mother care versus traditional care. Pediatrics. 2017;139(1):e20162063. doi:10.1542/peds.2016-2063.
- Ropars S, Tessier R, Charpak N, Uriza LF. The long-term effects of the Kangaroo mother care intervention on cognitive functioning: results from a longitudinal study. Dev Neuropsychol. 2018;43(1):82–91. doi:10.1080/87565641.2017.1422507.
- Mehler K, Hucklenbruch-Rother E, Trautmann-Villalba P, Becker I, Roth B, Kribs A. Delivery room skin-to-skin contact for preterm infants—A randomized clinical trial. Acta Paediatr. 2020;109(3):518–526. doi:10.1111/apa.14975.
- Walters MW, Boggs KM, Ludington-Hoe S, Price KM, Morrison B. Kangaroo Care at Birth for Full Term Infants: A Pilot Study. MCN: Am J Maternal/Child Nurs. 2007;32(6):375–381. doi:10.1097/01.NMC.0000298134.39785.6c.
- Bigelow A, Power M, MacLellan-Peters J, Alex M, McDonald C. Effect of mother/infant skin-to-skin contact on postpartum depressive symptoms and maternal physiological stress. J Obstet, Gynecol Neonatal Nurs. 2012;41(3):369–382. doi:10.1111/j.1552-6909.2012.01350.x.
- Bigelow AE, Power M. Mother–infant skin-to-skin contact: short- and long-term effects for mothers and their children born full-term. Front Psychol. 2020;11:1921. doi:10.3389/fpsyg.2020.01921.
- Cooijmans KHM, Beijers R, Rovers AC, de Weerth C. Effectiveness of skin-to-skin contact versus care-as-usual in mothers and their full-term infants: study protocol for a parallel-group randomized controlled trial. BMC Pediatr. 2017;17(1):154. doi:10.1186/s12887-017-0906-9.
- Cooijmans KHM, Beijers R, Brett BE, de Weerth C. Daily mother-infant skin-to-skin contact and maternal mental health and postpartum healing: a randomized controlled trial. Sci Rep. 2022;12(1):10225. doi:10.1038/s41598-022-14148-3.
- Cooijmans KHM, Beijers R, Brett BE, Weerth C. Daily skin-to-skin contact in full-term infants and breastfeeding: secondary outcomes from a randomized controlled trial. Matern Child Nutr. 2022;18(1):18. doi:10.1111/mcn.13241.
- Cooijmans KHM, Beijers R, De Weerth C. Daily skin-to-skin contact and crying and sleeping in healthy full-term infants: a randomized controlled trial. Dev Psychol. 2022;58(9):1629–1638. doi:10.1037/dev0001392.
- Rheinheimer N, Beijers R, Bruinhof N, Cooijmans KHM, de Weerth C. Effects of daily full-term infant skin-to-skin contact on behavior and cognition at age three – secondary outcomes of a randomized controlled trial. J Child Psychol Psychiatry. 2022;64(1):pp.136–144. doi:10.1111/jcpp.13679.
- Rheinheimer N, Beijers R, Cooijmans KHM, Brett BE, de Weerth C. Effects of skin-to-skin contact on full-term infants’ stress reactivity and quality of mother–infant interactions. Dev Psychobiol. 2022;64(7):64. doi:10.1002/dev.22308.
- Browne PD, Aparicio M, Alba C, Hechler C, Beijers R, Rodríguez JM, Fernández L, de Weerth C. Human milk microbiome and maternal postnatal psychosocial distress. Front Microbiol. 2019;10:2333. doi:10.3389/fmicb.2019.02333.
- Ziomkiewicz A, Babiszewska M, Apanasewicz A, Piosek M, Wychowaniec P, Cierniak A, Barbarska O, Szołtysik M, Danel D, Wichary S. Psychosocial stress and cortisol stress reactivity predict breast milk composition. Sci Rep. 2021;11(1):11576. doi:10.1038/s41598-021-90980-3.
- van den Elsen LWJ, Garssen J, Burcelin R, Verhasselt V. Shaping the gut microbiota by breastfeeding: The Gateway to Allergy prevention? Front Pediatr. 2019;7:47. doi:10.3389/fped.2019.00047.
- Bailey MT, Dowd SE, Galley JD, Hufnagle AR, Allen RG, Lyte M. Exposure to a social stressor alters the structure of the intestinal microbiota: implications for stressor-induced immunomodulation. Brain Behav Immun. 2011;25(3):397–407. doi:10.1016/j.bbi.2010.10.023.
- O’Mahony SM, Clarke G, Dinan TG, Cryan JF. Early-life adversity and brain development: is the microbiome a missing piece of the puzzle? Neuroscience. 2017;342:37–54. doi:10.1016/j.neuroscience.2015.09.068.
- de Weerth C. Do bacteria shape our development? Crosstalk between intestinal microbiota and HPA axis. Neurosci Biobehav Rev. 2017;83:458–471. doi:10.1016/j.neubiorev.2017.09.016.
- Galley JD, Nelson MC, Yu Z, Dowd SE, Walter J, Kumar PS, Lyte M, Bailey MT. Exposure to a social stressor disrupts the community structure of the colonic mucosa-associated microbiota. BMC Microbiol. 2014;14(1):189. doi:10.1186/1471-2180-14-189.
- Xu M, Wang C, Krolick KN, Shi H, Zhu J. Difference in post-stress recovery of the gut microbiome and its altered metabolism after chronic adolescent stress in rats. Sci Rep. 2020;10(1):3950. doi:10.1038/s41598-020-60862-1.
- Dill-McFarland KA, Tang Z-Z, Kemis JH, Kerby RL, Chen G, Palloni A, Sorenson T, Rey FE, Herd P. Close social relationships correlate with human gut microbiota composition. Sci Rep. 2019;9(1):703. doi:10.1038/s41598-018-37298-9.
- Drell T, Štšepetova J, Simm J, Rull K, Aleksejeva A, Antson A, Tillmann V, Metsis M, Sepp E, Salumets A, et al. The influence of different maternal microbial communities on the development of infant gut and oral microbiota. Sci Rep. 2017;7(1):9940. doi:10.1038/s41598-017-09278-y.
- Browne HP, Shao Y, Lawley TD. Mother-infant transmission of human microbiota. Curr Opin Microbiol. 2022;69:102173. doi:10.1016/j.mib.2022.102173.
- Ferretti P, Pasolli E, Tett A, Asnicar F, Gorfer V, Fedi S, Armanini F, Truong DT, Manara S, Zolfo M, et al. Mother-to-infant microbial transmission from different body sites shapes the developing infant gut microbiome. Cell Host & Microbe. 2018;24(1):133–145.e5. doi:10.1016/j.chom.2018.06.005.
- Bastiaanssen T, Gururajan A, van de Wouw M, Moloney GM, Ritz N, Long-Smith CM, Wiley NC, Murphy AB, Lyte JM, Fouhy F, et al. Volatility as a concept to understand the impact of stress on the microbiome. Psychoneuroendocrinology. 2021;124:105047. doi:10.1016/j.psyneuen.2020.105047.
- Cappellato M, Baruzzo G, Di Camillo B, Coelho LP. Investigating differential abundance methods in microbiome data: a benchmark study. PLoS Comput Biol. 2022;18(9):e1010467. doi:10.1371/journal.pcbi.1010467.
- Nearing JT, Douglas GM, Hayes MG, MacDonald J, Desai DK, Allward N, Jones CMA, Wright RJ, Dhanani AS, Comeau AM, et al. Microbiome differential abundance methods produce different results across 38 datasets. Nat Commun. 2022;13(1):342. doi:10.1038/s41467-022-28034-z.
- Bastiaanssen TFS, Quinn TP, Loughman A Treating bugs as features: a compositional guide to the statistical analysis of the microbiome-gut-brain axis [internet]. 2022 [accessed 2022 Sep 6]. https://arxiv.org/abs/2207.12475.
- Subramanian S, Huq S, Yatsunenko T, Haque R, Mahfuz M, Alam MA, Benezra A, DeStefano J, Meier MF, Muegge BD, et al. Persistent gut microbiota immaturity in malnourished Bangladeshi children. Nature. 2014;510(7505):417–421. doi:10.1038/nature13421.
- Valles-Colomer M, Falony G, Darzi Y, Tigchelaar EF, Wang J, Tito RY, Schiweck C, Kurilshikov A, Joossens M, Wijmenga C, et al. The neuroactive potential of the human gut microbiota in quality of life and depression. Nat microbiol. 2019;4:623–632.
- Clooney AG, Eckenberger J, Laserna-Mendieta E, Sexton KA, Bernstein MT, Vagianos K, Sargent M, Ryan FJ, Moran C, Sheehan D, et al. Ranking microbiome variance in inflammatory bowel disease: A large longitudinal intercontinental study. Gut. 2021;70(3):499–510. doi:10.1136/gutjnl-2020-321106.
- de Weerth C, Fuentes S, Puylaert P, de Vos WM. Intestinal microbiota of infants with colic: development and specific signatures. PEDIATRICS. 2013;131:e550–8.
- Depner M, Taft DH, Kirjavainen PV, Kalanetra KM, Karvonen AM, Peschel S, Schmausser-Hechfellner E, Roduit C, Frei R, Lauener R, et al. Maturation of the gut microbiome during the first year of life contributes to the protective farm effect on childhood asthma. Nat Med. 2020;26(11):1766–1775. doi:10.1038/s41591-020-1095-x.
- Bokulich NA, Chung J, Battaglia T, Henderson N, Jay M, Li HD, Lieber A, Wu F, Perez-Perez GI, Chen Y, et al. Antibiotics, birth mode, and diet shape microbiome maturation during early life. Sci Transl Med. 2016;8(343). doi:10.1126/scitranslmed.aad7121.
- Baniel A, Petrullo L, Mercer A, Reitsema L, Sams S, Beehner JC, Bergman TJ, Snyder-Mackler N, Lu A. Maternal effects on early-life gut microbiota maturation in a wild nonhuman primate. Curr Biol. 2022;32:4508–4520.e6.
- Laursen MF, Laursen RP, Larnkjær A, Mølgaard C, Michaelsen KF, Frøkiær H, Bahl MI, Licht TR, Suen G. Faecalibacterium gut colonization is Accelerated by presence of older siblings. mSphere. 2017;2(6):e00448–17. doi:10.1128/mSphere.00448-17.
- Roswall J, Olsson LM, Kovatcheva-Datchary P, Nilsson S, Tremaroli V, Simon M-C, Kiilerich P, Akrami R, Krämer M, Uhlén M, et al. Developmental trajectory of the healthy human gut microbiota during the first 5 years of life. Cell Host & Microbe. 2021;29:765–776.e3.
- Adlerberth I, Strachan DP, Matricardi PM, Ahrné S, Orfei L, Åberg N, Perkin MR, Tripodi S, Hesselmar B, Saalman R, et al. Gut microbiota and development of atopic eczema in 3 European birth cohorts. J Allergy Clin Immunol. 2007;120(2):343–350. doi:10.1016/j.jaci.2007.05.018.
- Azad MB, Konya T, Maughan H, Guttman DS, Field CJ, Sears MR, Becker AB, Scott JA, Kozyrskyj AL. CHILD study investigators. Infant gut microbiota and the hygiene hypothesis of allergic disease: impact of household pets and siblings on microbiota composition and diversity. Allergy, Asthma & Clinical Immunol. 2013;9:15.
- Christensen ED, Hjelmsø MH, Thorsen J, Shah S, Redgwell T, Poulsen CE, Trivedi U, Russel J, Gupta S, Chawes BL, et al. The developing airway and gut microbiota in early life is influenced by age of older siblings. Microbiome. 2022;10(1):106. doi:10.1186/s40168-022-01305-z.
- Laursen MF, Zachariassen G, Bahl MI, Bergström A, Høst A, Michaelsen KF, Licht TR. Having older siblings is associated with gut microbiota development during early childhood. BMC Microbiol. 2015;15:154.
- Miquel S, Martín R, Rossi O, Bermúdez-Humarán L, Chatel J, Sokol H, Thomas M, Wells J, Langella P. Faecalibacterium prausnitzii and human intestinal health. Curr Opin Microbiol. 2013;16(3):255–261. doi:10.1016/j.mib.2013.06.003.
- Tamana SK, Tun HM, Konya T, Chari RS, Field CJ, Guttman DS, Becker AB, Moraes TJ, Turvey SE, Subbarao P, et al. Bacteroides-dominant gut microbiome of late infancy is associated with enhanced neurodevelopment. Gut Microbes. 2021;13(1):1930875. doi:10.1080/19490976.2021.1930875.
- Duncan SH, Louis P, Flint HJ. Cultivable bacterial diversity from the human colon. Lett Appl Microbiol. 2007;44(4):343–350. doi:10.1111/j.1472-765X.2007.02129.x.
- Carey MA, Medlock GL, Alam M, Kabir M, Uddin MJ, Nayak U, Papin J, Faruque ASG, Haque R, Petri WA, et al. Megasphaera in the stool microbiota is negatively associated with diarrheal cryptosporidiosis. Clin Infect Dis. 2021;73(6):e1242–51. doi:10.1093/cid/ciab207.
- Dutton CL, Maisha FM, Quinn EB, Morales KL, Moore JM, Mulligan CJ. Maternal psychosocial stress is associated with reduced diversity in the early infant gut microbiome. Microorganisms. 2023;11(4):975. doi:10.3390/microorganisms11040975.
- Stokholm J, Blaser MJ, Thorsen J, Rasmussen MA, Waage J, Vinding RK, Schoos A-M, Kunøe A, Fink NR, Chawes BL, et al. Publisher correction: maturation of the gut microbiome and risk of asthma in childhood. Nat Commun. 2018;9(1). doi:10.1038/s41467-018-03150-x.
- Ogita T, Yamamoto Y, Mikami A, Shigemori S, Sato T, Shimosato T. Oral administration of Flavonifractor plautii strongly suppresses Th2 immune responses in mice. Front Immunol. 2020;11:379. doi:10.3389/fimmu.2020.00379.
- Wang Z, Neupane A, Vo R, White J, Wang X, Marzano SYL. Comparing gut microbiome in mothers’ own breast milk- and formula-fed moderate-late preterm infants. Front Microbiol. 2019;11. doi:10.3389/fmicb.2020.00891.
- Arrieta M-C, Stiemsma LT, Dimitriu PA, Thorson L, Russell S, Yurist-Doutsch S, Kuzeljevic B, Gold MJ, Britton HM, Lefebvre DL, et al. Early infancy microbial and metabolic alterations affect risk of childhood asthma. Sci Transl Med. 2015;7(307). doi:10.1126/scitranslmed.aab2271.
- Shetty SA, Zuffa S, Bui TPN, Aalvink S, Smidt H, De Vos WM. Reclassification of Eubacterium hallii as Anaerobutyricum hallii gen. Nov., comb. Nov., and description of Anaerobutyricum soehngenii sp. Nov., a butyrate and propionate-producing bacterium from infant faeces. Int J Syst Evol Microbiol. 2018;68(12):3741–3746. doi:10.1099/ijsem.0.003041.
- Chia LW. Cross-feeding interactions of gut symbionts driven by human milk oligosaccharidesand mucins. 2018.
- Dedon LR, Hilliard MA, Rani A, Daza-Merchan ZT, Story G, Briere C-E, Sela DA. Fucosylated human milk oligosaccharides drive structure-specific syntrophy between Bifidobacterium infantis and Eubacterium hallii within a modeled infant gut microbiome. Mol Nutr Food Res. 2023;67(11):2200851. doi:10.1002/mnfr.202200851.
- Pham VT, Lacroix C, Braegger CP, Chassard C. Lactate-utilizing community is associated with gut microbiota dysbiosis in colicky infants. Sci Rep. 2017;7(1):11176. doi:10.1038/s41598-017-11509-1.
- Gilijamse PW, Hartstra AV, Levin E, Wortelboer K, Serlie MJ, Ackermans MT, Herrema H, Nederveen AJ, Imangaliyev S, Aalvink S, et al. Treatment with Anaerobutyricum soehngenii: a pilot study of safety and dose–response effects on glucose metabolism in human subjects with metabolic syndrome. npj Biofilm Microbio. 2020;6(1):16. doi:10.1038/s41522-020-0127-0.
- Hernán M, Hernández-Díaz S, Robins J. Randomized trials analyzed as observational studies. Ann Intern Med. 2013. doi:10.7326/0003-4819-159-8-201310150-00709.
- Hernán M, Hernández-Díaz S. Beyond the intention-to-treat in comparative effectiveness research. Clin Trials. 2012;9(1):48–55. doi:10.1177/1740774511420743.
- Ramiro-Garcia J, Hermes GDA, Giatsis C, Sipkema D, Zoetendal EG, Schaap PJ, Smidt H. NG-Tax, a highly accurate and validated pipeline for analysis of 16S rRNA amplicons from complex biomes. F1000Research. 2018;5:1791. doi:10.12688/f1000research.9227.2.
- Huang R, Soneson C, Ernst FGM, Rue-Albrecht KC, Yu G, Hicks SC, Robinson MD. TreeSummarizedExperiment: a S4 class for data with hierarchical structure. F1000Research. 2021;9:1246. doi:10.12688/f1000research.26669.2.
- R Core Team. R: a language and environment for statistical computing [internet]. Vienna, Austria: R Foundation for Statistical Computing; 2022. https://www.R-project.org/.
- Kleinke K. Multiple imputation under violated distributional assumptions: a systematic evaluation of the assumed robustness of predictive Mean matching. J Educ Behav Stat. 2017;42(4):371–404. doi:10.3102/1076998616687084.
- Van Buuren S, Groothuis-Oudshoorn K. Mice: multivariate imputation by chained equations in R. J Stat Soft. 2011;45(3). doi:10.18637/jss.v045.i03.
- Bürkner P-C. Advanced Bayesian multilevel modeling with the R package brms. R J. 2018;10(1):18. doi:10.32614/RJ-2018-017.
- Lin H, Peddada SD. Analysis of compositions of microbiomes with bias correction. Nat Commun. 2020;11(1):3514. doi:10.1038/s41467-020-17041-7.
- Zhou H, He K, Chen J, Zhang X. LinDA: Linear models for differential abundance analysis of microbiome compositional data. Genome Biol. 2022;23(1):95. doi:10.1186/s13059-022-02655-5.
- Mallick H, Rahnavard A, McIver LJ, Ma S, Zhang Y, Nguyen LH, Tickle TL, Weingart G, Ren B, Schwager EH, et al. Multivariable association discovery in population-scale meta-omics studies. PLoS Comput Biol. 2021;17(11):e1009442. doi:10.1371/journal.pcbi.1009442.
- Oksanen J, Simpson GL, Blanchet FG, Kindt R, Legendre P, Minchin PR, O’Hara RB, Solymos P, Stevens MHH, Szoecs E, et al. Vegan: Community ecology package [Internet]. 2022. https://CRAN.R-project.org/package=vegan.
- Ernst FGM, Shetty SA, Borman T, Lahti L. Mia: Microbiome analysis [Internet]. 2022. https://github.com/microbiome/mia.
- Cinelli C, Forney A, Pearl J. A crash course in good and bad controls. SSRN Electron J. 2020. doi:10.2139/ssrn.3689437.
- Vehtari A, Gelman A, Gabry J. Practical Bayesian model evaluation using leave-one-out cross-validation and WAIC. Stat Comput. 2017;27(5):1413–1432. doi:10.1007/s11222-016-9696-4.