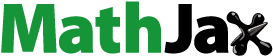
ABSTRACT
Dietary omega-3 polyunsaturated fatty acids (n-3 PUFAs) and the gut microbiome affect each other. We investigated the impact of supplementation with Buglossoides arvensis oil (BO), rich in stearidonic acid (SDA), on the human gut microbiome. Employing the Mucosal Simulator of the Human Intestinal Microbial Ecosystem (M-SHIME), we simulated the ileal and ascending colon microbiomes of four donors. Our results reveal two distinct microbiota clusters influenced by BO, exhibiting shared and contrasting shifts. Notably, Bacteroides and Clostridia abundance underwent similar changes in both clusters, accompanied by increased propionate production in the colon. However, in the ileum, cluster 2 displayed a higher metabolic activity in terms of BO-induced propionate levels. Accordingly, a triad of bacterial members involved in propionate production through the succinate pathway, namely Bacteroides, Parabacteroides, and Phascolarctobacterium, was identified particularly in this cluster, which also showed a surge of second-generation probiotics, such as Akkermansia, in the colon. Finally, we describe for the first time the capability of gut bacteria to produce N-acyl-ethanolamines, and particularly the SDA-derived N-stearidonoyl-ethanolamine, following BO supplementation, which also stimulated the production of another bioactive endocannabinoid-like molecule, commendamide, in both cases with variations across individuals. Spearman correlations enabled the identification of bacterial genera potentially involved in endocannabinoid-like molecule production, such as, in agreement with previous reports, Bacteroides in the case of commendamide. This study suggests that the potential health benefits on the human microbiome of certain dietary oils may be amenable to stratified nutrition strategies and extend beyond n-3 PUFAs to include microbiota-derived endocannabinoid-like mediators.
Introduction
The popularity of plant sources of omega-3 polyunsaturated fatty acids (n-3 PUFAs) including nuts and seed oils rich in alpha-linolenic acid (18:3 n-3, ALA) and stearidonic acid (18:4 n-3, SDA), has increased in recent years for several reasons. First, attractivity – they are a sustainable and ethical alternative to conventional fish oils that are rich in eicosapentaneoic acid (20:5 n-3, EPA) and docosahexanoic acid (22:6 n-3, DHA);Citation1–3 second, biotransformation – SDA, unlike -linolenic acid, is readily converted in humans into EPA,Citation4 a long chain PUFAs widely recognized for its multifaceted health benefitsCitation5–9 and third, health properties – SDA displays emerging anti-inflammatory, cardioprotective and neuroprotective properties, which position it as a potential alternative to marine source-derived EPA and DHA.Citation10–15 The seed oil from Buglossoides arvensis (BO) is the richest known natural source of SDA (~21% compared to echium oil ~ 13%) and provides the highest n-3 to n-6 ratio plant-based fatty acids (4:1).Citation16,Citation17 Despite this remarkable fatty acid profile, the specific health effects of BO have been poorly studied.
Within the palette of mechanisms potentially explaining the beneficial actions of dietary PUFAs, the pivotal role of the gut microbiome in mediating a wide range of health effects and maintaining body homeostasis has garnered significant attention.Citation18,Citation19 PUFAs can act as prebiotics,Citation20 enhancing beneficial host functions by influencing gut bacterial composition and metabolic activity.Citation19,Citation21 Conversely, gut bacteria can affect PUFAs metabolism,Citation22 suggesting that the health advantages associated with dietary oils extend beyond just PUFAs as such. Indeed, PUFAs are metabolized by the host to a plethora of metabolites, including oxylipins, prostanoids, leukotrienes, and products of non-oxidative metabolism, such as endocannabinoids and their polyunsaturated congeners.Citation23 These latter metabolites, including N-acyl ethanolamines (NAEs), and in particular the arachidonic acid-derived endocannabinoid anandamide,Citation23,Citation24 can be catabolized by gut bacteria.Citation25 Additionally, bacteria produce their own endocannabinoid-like metabolites, which however are not usually obtained from the processing of n-3 PUFAs, as in the case of commendamide and its congeners.Citation26,Citation27 Altogether, these metabolites can influence various host physiological processes, including energy processing, inflammation, and mood regulation.Citation28,Citation29 Notably, there is a surprising lack of research on the direct effects of SDA-rich oils, such as BO, on the gut microbiome. Only one study has shown that Ahiflower oilTM, the trademark of BO, when encapsulated with a blend of probiotic strains, enhances the viability of these strains under simulated physicochemical conditions mimicking the upper gastrointestinal tract in a TIM-1 model.Citation30 However, there is currently no evidence for the capability of gut bacteria to produce NAEs, underscoring the need for further investigations in these areas.
It is becoming increasingly apparent that nutritional studies need to consider inter-individual responses to dietary components, which may represent the basis to develop stratified nutritional strategies, now recognized as a crucial requirement for the optimization and sustainability of new therapeutic tools. Among the myriad of factors justifying the need for personalized nutrition, the inherent variability in gut bacteria composition among individuals is the main one.Citation31,Citation32
Current methods investigating the interactions between the human gut microbiome and dietary supplements are often limited to animal studies, which may be of little translatable value to humans.Citation33 As an alternative to identify more clinically translatable and host-independent mechanisms, multicompartmental in vitro fermentation systems, such as the Mucosal Simulator of the Human Intestinal Microbial Ecosystem (M-SHIME®), help elucidating the mechanisms of the effects of dietary components on gut microorganisms, whilst simulating their spatial distribution within the gut.Citation34 Here, we used the M-SHIME® to mimic the ileum, a feature uniquely reproduced in this set-up, and ascending colon lumen and mucosal microbiome ecosystems of four donors. This ecological framework enables the study, in an individual- and time-dependent manner, of the compositional, metabolic (in terms of short chain fatty acids; SCFAs) and targeted lipidomics responses of gut microbiota regional signatures to BO.
Results
The impact of Buglossoides oil on the gut microbiome depends on initial donor bacterial microbiota composition and its gut ecosystem signature
We used fecal samples from four different donors (Supp Table S1), cultured in duplicate, to dynamically assess the impact of 14 days of daily supplementation with n-3 FA-rich BO on the shaping of gut bacterial microbiota communities within the M-SHIME ileum and ascending colon lumen (L_IL and L_AC, respectively) and mucus (M_IL and M_AC, respectively) niches (Supp Fig S1a). The general impact of the oil on the bacterial community structure was assessed by 16S rRNA gene amplicon sequencing data at different time points during control (CTRL) and BO (Supp Fig S1b).
The primary objective was to systematically assess the variables that could potentially influence bacterial microbiota variance (). We first utilized distance-based redundancy analysis (dbRDA) to visualize the overall separation of samples based on the condition CTRL vs BO (). Taking into consideration all 261 samples collected from the SHIME from different donors, gut ecosystems, and timepoints, the 14-day BO supplementation contributed significantly to the modest grouping of samples at the genus level (2%, p < .001), as confirmed by distance-based redundancy analysis dbDRA (). In , we visualized separation based on individual donors. Not surprisingly, the distinctiveness of individual donor bacterial microbiota composition contributed considerably more to the bacterial microbiota variance than the daily supplementation of BO (12.3%, p < .001) (). Indeed, when looking at the initial percentage of shared amplicon sequence variants (ASVs) between donors in the fecal inoculum, prior to SHIME fermentation, only a core set of bacteria representing less than 10% of all ASVs were conserved between all four donors (, Supp Fig S2). The percentage of shared ASVs was increased when pairwise comparisons of fecal samples were performed, ranging from 20% to 40% (). Donors B, C and D initially shared significantly more identical ASVs than donor A (p < .001). Then, during the SHIME, the cultivation of fecal bacterial microbiota with the same nutritional medium/dietary supplementation for 21 days increased the pairwise ASVs sharing rate for donors A and B (to over 40%) and donors C and D, predominantly in the luminal gut geography, i.e., L_IL (). It creates therefore a consistent environment conducive the growth of certain bacterial species across multiple donors. Remarkably, the additional 14 days of fermentation in the presence of BO tended to slightly increase the pairwise sharing of ASVs between donors A and B in L_IL, (68% under BO vs 60% under CTRL period, p < .05), in M_IL (45% under BO vs. 22% under CTRL period, p < .01), and in M_AC (54% under BO vs 21% under CTRL period, p < .01) () for donors A and C. Globally, the donor variance explained about 12.5% of the variation between microbiomes (p < .001). , these results underscore the significance of accounting for gut ecosystem niches, which explained 22% of the variance (p < .001), in subsequent analysis.
Figure 1. Overall impact of Buglossoides oil on gut bacterial communities. (a-c) comparison of bacterial beta diversity index using partial distance-based redundancy analysis (dbRDA) based on the Bray-Curtis distance metric. Ellipses represent 95% confidence intervals. Supplementation condition (a), and donor (c) were set as explanatory variables (in blue). (b) Recapitulates the percentage of bacterial variance according to the condition and donor variables of the study. *** indicates p < .001 significance of the observed group separation, as assessed with a Permutational Multivariate analysis of variance (PERMANOVA) using distance matrixes. (d) Percentage of shared ASVs between all and specific donor pairs in the fecal inoculum and gut geographies reproduced in the SHIME, i.e., L_IL, M_IL, L_AC and M_AC under CTRL vs. BO. Statistically significant differences between CTRL period and BO are denoted with p < .05 (*), p < .01 (**), and p < .001 (***), as determined by T-test with Bonferroni correction. Control; CTRL, Buglossoides oil; BO.

Figure 2. Temporal and individual responses of α -, β-diversity and mucus engraftment rate following buglossoides oil supplementation. (a) Percentage of bacterial microbiota variance according to the key variables of the study, including the condition CTRL vs BO; time; donor; and gut niches. (b) Comparison of bacterial beta diversity index using partial distance-based redundancy analysis (dbRDA) based on the Bray-Curtis distance metric. Time evolution (days) across fermentation was set as explanatory variables (in blue). *** indicates p < .001 significance of the observed group separation, as assessed with a PERMANOVA using distance matrixes. (c) Percentage of bacterial microbiota variance elicited by BO across the different gut geographies of the study. (d) Shannon alpha diversity displayed across gut geographies. (e) Chao1 richness index only shown for the gut region in which significant differences were observed. (f) ASVs engraftment rate in mucus is displayed for all donors together, each donor and period of the different gut geographies. Statistically significant differences between CTRL and BO of Shannon, Chao1 indexes, and mucus engraftment are denoted with p < .05 (*), p < .01 (**), p < .001 (***), and p < .001 (****), as determined by T-test with Bonferroni correction. (g) Average relative composition of the 22 most abundant genera between the four different gut geographies according to the donor and fermentation period.

The time of the fermentation was another variable to consider, as it explained 7.6% of the bacterial microbiota variation (p < .001), (). Interestingly, the dbRDA revealed a significant relationship between the variation in bacterial microbiota composition and the timing of BO supplementation, as evidenced by a progressive, day-dependent divergence in samples obtained during BO, compared to those collected under CTRL (). Therefore, for the following analysis, the samples collected during the 14-day BO were split into two periods: days 8–14 (BO1, i.e., first week BO) and days 15–21 (BO2, i.e., second week BO), (). The lack of significant variance over time during CTRL confirmed that the four bacterial microbiota were stable prior to the start of BO (1.2%, p = .64) (Supp Fig S3).
Buglossoides oil progressively reduces overall bacterial microbiota diversity but promotes mucus colonization in a donor-dependent fashion
Following the 14 days (BO1 and BO2), bacterial microbiota β-diversity was significantly altered in the luminal regions: L_IL and L_AC (p < .001; ). The Shannon α-diversity index was decreased in response to BO supplementation and was generally more significant in BO2, when all the donors are averaged except for the M_AC, while only a tendency emerged when this parameter was individualized due to the lower statistical power (, Supp Fig S4). Although BO did not significantly affect the Shannon α-diversity and β-diversity in the M_AC (), the BO2 period resulted in a significant increase in ASVs richness in M_AC but only in a trend increase when the donors are considered individually (Chao1, p < .01, , Supp Fig S5). This finding was supported by the remarkable increase in ASVs engraftment in mucus samples from donors C and D during BO2 (p < .001, ). Compilation of the relative abundance of the top-22 genera for each donor, within each gut ecosystem and between conditions, periods, highlighted the diversity of the individual bacterial signatures (). The full timeline of bacterial composition per replicate is provided at the genus level (Supp Fig S6, 7).
Identification of cluster bacterial microbiota
We opted to use clustering (Supp Fig S8, Supp Table S3), to simplify the analysis and interpretation of results, despite the small sample size of four donors to investigate response variability to BO supplementation. This approach allowed us to draw conclusions based on groups rather than individual’s data, with a more comprehensive understanding of the patterns and trends within the data, as well as increasing statistical power. We categorized the four donors into two clusters of microbiota: cluster 1 (C1) and cluster 2 (C2) (). This clustering also aligns with previous observations: (i) donors A-B and C-D had higher levels of shared ASVs () and, (ii) dominant genera in common (, Supp Fig S6, 7). After DESeq2 analysis, heatmaps were generated at the genus level to visually display the bacterial signatures (expressed as normalized counts), subsequent clustering analysis clearly separated the CRTL and BO periods and individual C1 (individual A and B) and C2 (individual C and D) from each other, in IL () and AC (), as described below. As a final confirmation that the clusters displayed independent signatures, when looking at the bacterial taxa co-occurring together, we identified that there is no co-occurrence shared between inter-cluster (C1-C2) for a given gut region/niche ().
Figure 3. Donor clustering identifies key taxa dissimilarity between donors in each gut region. (a) Cluster dissimilarity representation using PCA in the AC during BO. (b-c) heatmaps display the DESeq normalised counts of genera that are significantly shifted between CTRL and BO using a Wald test with p < .05. Hierarchical clustering of the donors, based on Pearson distance, is represented for the luminal (b) IL and (c) AC regions. (d) Upset plot visually depicts the intersection of sets, showcasing the number of bacterial co-occurences shared among the different microbial regions (L/M, IL and AC) and/or donor clusters (C1 and C2). “Mix” represents inter-class co-occurences (e.g., one bacterium belonging from Clostridia, and the other from Bacilli), while the remaining colors are intra-class co-occurences, indicating bacterial associations within the same class (e.g., two bacteria belonging from Clostridia). This graphical depiction offers insights into the complex relationships and patterns of bacterial co-occurrences across different microbial regions and clusters, facilitating a deeper understanding of the microbial community structure and interactions.

Buglossoides oil causes both similar and contrasting changes in the luminal bacterial microbiota of the two clusters
Both luminal bacterial microbiota clusters showed both common and specific changes in response to BO (). First, the DESeq2 analysis and Wald test for statistics, helped elucidating core and significant changes of luminal bacterial microbiota that were representative of the two clusters, although changes were more often significant in C2 than C1 (). Notably, dominant taxa belonging mostly to the Clostridia class i.e., Blautia, Coprococcus, Dorea, Lachnospiraceae ND3007, and Ruminococcus torques, were depleted with BO (p < .05), as were the Actinomycetia Bifidobacterium (p < .01), the Coriobacteriia Collinsella (p < .05) in the L_IL and L_AC regions; and the Bacilli Enterococcus in the L_AC (p < .05). In contrast, BO significantly stimulated only one Clostridia genus, Lachnoclostridium, in the L_IL and L_AC (p < .0001, for C2), and increased the Bacteroidia genera Bacteroides, Parabacteroides (p < .001, for C2), the Negativicutes genera Acidaminococcus (p < .05), and the Gammaproteobacteria genus Escherichia-Shigella, with a trend for Veillonella (p = .061) in L_IL (p < .05) ().
Figure 4. Luminal co-abundance response clusters in response to Buglossoides oil. Selection of genera in normalized log transformed counts that are significantly modulated by BO similarly (a) or dissimilarly (b) between clusters, as assessed by DESeq analysis. Statistical differences between CTRL and BO across gut regions were determined using a wald test with p < .05 (*), p < .01 (**), p < .001 (***), and p < .001 (****). Colored labels indicate the class of the respective genera.

Moreover, the DESeq2 analysis also uncovered distinct changes in the abundance of less dominant genera in response to BO, which varied depending on the type of cluster (). For instance, C1 showed specific depletion in the Clostridia class, including Eubacterium fissicatena in L_IL (p < .001), Anaerostipes, and Intestinibacter in the L_IL and L_AC regions (p < .05), whereas C2, which initially had the Clostridia Ruminococcus gnavus and Subdoligranulum in the L_IL and L_AC (p < .05), and Candidatus Soleaferrea and Faecalibacterium in the AC (p < .01), exhibited a significant decrease in the relative abundance of these classes (, Supp Fig S9). These findings highlight the distinct responses of different Clostridia taxa to BO based on their respective clusters C1 and C2. Among other taxa, the Verrucomicrobiae Akkermansia muciniphila (ASV_107) was initially present (CTRL) at a low level in the L_AC of C2 and, remarkably, was significantly enriched after BO compared to C1 (p < .05) (, Supp Fig S9, Table S4). The same observation was made for the Negativicutes class with a significant stimulation of Phascolarctobacterium, Anaeroglobus (p < .01) and the Erysipellotrichia Erysipelotrichaceae in the L_IL and L_AC of C2 (p < .05), which were mostly absent or non-significantly stimulated in C1 (, Supp Fig S9). Interestingly, rare but contrasting results were observed for the same taxa in C1 and C2. For example, the Gammaproteobacteria Klebsiella was significantly stimulated by BO supplementation in the L_AC of C2 (p < .001), while remaining unchanged in C1. On the other hand, Clostridium sensu stricto 1 tended to increase with BO supplementation in the L_IL of C1 but showed a significant decrease in C2 (p < .05) (, Supp Fig S9).
Buglossoides oil selectively modulates the mucus-associated bacterial microbiota with pronounced differences between clusters
First, a strong discrepancy between the mucus-associated bacterial microbiota compositions was observed in the two clusters (). In addition, the DESeq analysis displayed a more modest modulation of the mucus-associated bacterial relative abundances in response to BO () than luminal ones (). When looking at the relative abundance of the taxa that were shifted by BO, most of the taxa were the non-dominant mucus flora, representing less than 10% of total abundance (). For example, Ligilactobacillus a genus only present in C2 M_IL and M_AC, was gradually but non-significantly decreased during BO supplementation, and so was Agathobacter due to large intra-cluster variability (), while Pseudomonas, present in the M_IL of C1 and C2, was significantly increased at the end of BO2 only in C1. Additional contrasting results consisted of a gradual and significant increase of Dialister and Blautia with BO in the M_AC of C1, that instead were gradually depleted in C2 (, Supp Fig S10). Among the taxa representing less than 1% of the total bacterial microbiota abundance, Sellimonas intestinalis (ASV_228) was significantly increased in the M_IL and M_AC of C1 only (, Supp Fig S10, Supp Table S4), while Akkermansia muciniphila (ASV_107), like in the lumen, was significantly increased by BO in the M_AC () in C2 only (p < .05) (, Supp Fig S10). By contrast, the significant decrease of Erysipelatoclostridium with BO was seen in both clusters in both the M_IL and M_AC, (p < .05).
Figure 5. Buglossoides oil impacts non-dominant taxa in mucus bacterial microbiota with marked dissimilarity among clusters. (a-b) volcano plots indicating the genera differentially enriched (a) between clusters under BO and (b) between CTRL and BO in the overall mucus gut geographies. Statistical differences were determined using a wald test. The log transformed adjusted p-value is displayed on the y-axis and the α = 0.05 significance level is indicated by a dashed line. (c-d) selection of genera that represent less than (c) 10% and (d) 1% of the total abundance, with contrasting modulation between clusters.

Cluster 2 exhibits an enhanced metabolic response following Buglossoides oil supplementation, hallmarked by an increase of propionate
During fermentation, acid and base delivery were recorded. A gradual rise in acid delivery was observed in the L_AC (BO2 vs CTRL, p < .0001) (), associated with a significant decrease in total SCFA levels from 52 ± 5.6 mM to 44 ± 3.8 mM toward the end of the 14 days BO supplementation in both clusters (BO2, p < .0001) (). Such changes in the levels of total SCFA reflect, in fact, a gradual shift of the main and minor SCFA ratios () through a significant decrease of acetate in the L_IL (BO2, p < .001) and L_AC (BO2, p < .05) of C2 solely. In contrast, the propionate was approximately doubled in both the L_IL and L_AC regions, again for C2 (BO2, p < .001and in L_AC only for C1 (BO2, p < .01). Cluster 2 L_AC exhibited a noteworthy shift in the propionate/butyrate ratio (BO2, p < .05), with the reversal of the dominance of butyrate production observed during CTRL (). No change of butyrate was observed in L_IL in either cluster. Furthermore, minor SCFA production was a clear marker of the inter-cluster variability, with C2 having more diverse minor SCFAs produced and being the only one capable of producing caproate in both the L_IL and L_AC (). Caproate, however, was not affected by BO. Opposite trends were seen for valerate in response to BO. Valerate was significantly increased in both the L_IL and L_AC of C2 (BO2, p < .001), whereas it was significantly decreased in the L_AC of C1 (BO2, p < .01). Finally, we did not detect changes in the levels of branched chain fatty acids in response to BO, possibly due to inter-individual variability ().
Figure 6. SCFA alterations associated with Buglossoides oil. (a) Daily acid and base delivery (mL) to the SHIME during fermentation. (b) Mean concentration of total SCFAs were calculated for each cluster. (c-d) area graphs of mean ratios (%) of (c) major and (d) minor SCFAs. Statistically significant differences between CTRL and BO periods are denoted for p < .05 (*), p < .01 (**), p < .001 (***), and p < .001 (****) as determined by Pairwise Wilcoxon rank sum test with Bonferroni correction.

Spearman correlations under BO () showed that the observed increase of Bacteroidetes, including species with potential probiotic properties in C2 (e.g., B. xylanisolvens, B. ovatus), and Parabacteroides distasonis (ASVs_48, 64, 66, 119, 174) (Supp Table S4), concomitantly with the growth of Phascolarctobacterium faecium (ASV_34) are positively associated with the increased propionate production in L_IL of C2 (r = 0.75, p < .0001). We also observed that these three genera strongly co-occur (r = 0.93, p < .0001) (). Since Bacteroides and Parabacteroides are known succinate producers,Citation35–37 and Phascolarctobacterium is known to be a succinate consumer,Citation35 our results suggest that this latter species used succinate produced by Bacteroides/Parabacteroides species as a precursor for propionate production, explaining therefore the increased propionate under BO specifically in the C2 L_IL (). Finally, the strong decrease in Bifidobacterium (ASVs_13, 38, 226) as well as of most of the Clostridia class of bacteria in both clusters (), was significantly associated with the observed decrease in acetate and butyrate production, as previously reported in the literatureCitation38 ().
Figure 7. Associations between SCFAs, bacterial genera, and co-occurrence patterns under Buglossoides oil supplementation. (a) Spearman correlograms depicting significant associations (p < .05) with FDR corrections, between SCFAs and bacterial genera with a cutoff of r ˃ 0.6 or < −0.6. (b) Co-occurrence network of the ileum of cluster 2 microbiomes, generated with Cytoscape. A visibility cutoff of r > 0.7 was applied. Red circle highlights three genera known in the literature to be associated with the succinate pathway.

Buglossoides oil elicits the biosynthesis of commendamide and the unprecedented bio-transformation by gut bacteria of an n-3 PUFA into the corresponding bioactive NAE
After introducing daily BO, an SDA-rich oil (), into the Stomach_Si, we measured the fate of the fatty acid-derived lipids, including free fatty acids and their corresponding monoacylglycerols and NAEs (Supp Fig S11). With the help of mathematical modeling to assess whether there is a production, accumulation, or degradation of the lipids in the M-SHIME system (see discussion), the analysis revealed an active breakdown of SDA in the gut regions, particularly in L_AC (). The concentration of SDA consistently fell below the modeled area. Strikingly, a substantial and significant production of SDEA over time was observed in L_IL (p < .05), and in L_AC, with a very significant production for C2 (p < .001), surpassing the modeling area (). Barely detectable amounts of SDEA originated from the oil per se was confirmed (), whereas the compound was not detected in the nutritional medium and enzymatic digestive cocktail (Supp Fig S12). This result represents the first direct evidence suggesting that gut bacteria can convert a dietary n-3 PUFA, i.e., SDA, into its corresponding NAE, i.e., SDEA, a bioactive endocannabinoid-like mediator. A Spearman correlation analysis was conducted in the colon of all donors (), revealing significant associations between SDEA production and specific bacterial genera (p < .05). Butyricicoccus, Campylobacter and Phascolarctobacterium exhibited the strongest positive association with SDEA. Interestingly, these taxa are prevailing within C2 (), where we observed the highest capability to produce SDEA (). Our method was not designed to measure the ethanolamide of ALA, the most abundant fatty acid component of BO, but could detect in the L_AC section from one or two donors, respectively, the above-control formation of LEA and PEA, i.e., respectively the ethanolamides of linoleic and palmitic acid, two other abundant components of the oil (, Suppl. Fig. S11).
Figure 8. Buglossoides oil elicits the cluster-dependent production of SDEA by bacteria. (a) Percentage of lipid content in an BO capsule. (b) Amounts of SDA in nmol total per gut region for each donor. The area depicted in blue represents the mathematical modeling of SDA concentration. Lines below the blue area are indicative of consumption of SDA by bacteria. (c) Amounts of SDEA in nmol total per gut region for each donor. The area depicted in blue represents the mathematical modeling of SDEA concentration. Lines over the blue area are indicative of production of SDEA by bacteria. Statistically significant differences between the mathematical modeling and SDEA concentrations are denoted with p < .05 (*), and p < .001 (***), as determined by the T-test with Bonferroni correction. (d) Spearman’s correlation analysis between SDEA amounts and the relative abundance of bacterial genera found in the proximal colon of all clusters. Only significant associations (p < .05) with FDR corrections are shown.

Next, since we observed a remarkable and significant stimulation of Bacteroides during BO in both clusters (), we quantified the levels of a well-documented Bacteroides-derived endocannabinoid-like mediator, commendamide. No production of commendamide was detected in the Stomach-Si (), where there was no bacteria. Conversely, in the L_IL and L_AC, there was a modest baseline of commendamide production during CTRL. However, this production saw a notable increase following BO (). Commendamide levels remained significantly elevated in the L_IL BO1 of C1 (p < .05), and we observed a noticeable trend also for C2. Finally, we identified strong correlations between several Bacteroides species with commendamide (). B. dorei (ASV_6), B. ovatus (ASV_61), and B. distasonis (ASV_65) were the most positively associated with commendamide increase. Interestingly, those three species are more abundant in C2, and stimulated by BO ().
Figure 9. Commendamide production by gut bacteria is stimulated by Buglossoides oil. (a) Stratification of the relative abundance of Bacteroides species between IL and AC lumen across periods for each microbial cluster. (b) Concentration of commendamide in nmol total across gut regions, and periods for each microbial cluster. Statistically significant differences between CTRL and BO are denoted with p < .05 (*), as determined by the T-test with Bonferroni correction. (c) Targeted Spearman’s correlation between commendamide and all the Bacteroides species found in the ileum of all clusters. Only significant associations (p < .05) with FDR corrections are shown.

Discussion
Diet shapes gut microbiota, making dietary modulation a key avenue toward a healthy gut microbiome.Citation39 Traditionally, research on diets and dietary supplements within the microbiome field has focused on unimodal perspectives, examining alpha- and beta-diversity modulation of the gut microbiota. Here, we illustrate the dynamic and bimodal interactions between an SDA-rich omega-3 oil – obtained from Buglossoides arvensis seeds – and the gut bacteriome. Our findings illustrate that this dietary supplement not only exhibits prebiotic-like effects,Citation40,Citation41 but also fosters the remarkable and previously understudied capability of gut microbiota to bio-transform dietary fatty acids into bioactive endocannabinoid-like mediators, which have pleiotropic physiological effects.Citation41,Citation42 This mutual metabolic crosstalk between commensal bacteria and PUFAs is further complicated by the inherent uniqueness of the gut microbiota and the consequent inter-individual variation in its composition and response,Citation43 recently witnessed also by the ever-increasing efforts within the emerging fields of nutri-microbiomics and nutri-metabolomicsCitation44 to unravel individual functional responses to diet.
In our study, we interrogated inter-individual variations in the microbiota and comprehended specific microbial-driven metabolic responses. Although we explored a small cohort of four donors with unique and distinct fecal microbiota, our dynamic longitudinal sampling, along with mimicking the luminal and mucus geographies of the ileum and ascending colon,Citation45 allowed us to discern two distinct donor clusters, based on both the initial composition, and substantial differential modulation of such microbiota by a 14-day BO supplementation ex vivo across various simulated gut ecosystems.
First, we observed a common microbial modulation in response to BO. The most striking outcome was the gradual reduction in the normalized abundance of several Clostridia members, including Blautia, Coprococcus, Dorea, and Lachnospiraceaeae in both L_IL and L_AC. It is worth noting that similar findings have been reported with other omega-3 interventions.Citation19,Citation46 Exploring the metabolic footprint of these microbes and their nutrient source preferences behind these microbial shifts will provide valuable insights into understanding how gut bacteria respond to dietary energy sources. The use of culturomics approaches, for instance, may help unravel the underlying mechanisms driving these observed changes in the microbiota composition.
Secondly, interesting microbial cluster-specific changes emerged with BO supplementation. In Clostridia, certain genera exhibited distinct responses in one cluster but not the other. For example, Anaerostipes, initially enriched in C1, was notably depleted following BO, while this genus was absent in C2. A key additional signature for C2 was a gradual enrichment of known beneficial bacteria for health, namely Akkermansia muciniphilaCitation19 and Phascolarctobacterium faecium,Citation47 which were not detected in C1. While we indeed observed substantial differences in the microbial compositions of the two clusters, a shared trait emerged concerning SCFAs, the most studied gut bacterial metabolites, renowned for their wide-ranging impact on various physiological processes.Citation48 Both clusters exhibited an overall increase in L_AC propionate and a reduction in acetate. However, some differences emerged in L_IL, where only C2 displayed a significant rise in propionate levels. Our correlation and co-occurrence analyses unveiled an intriguing triad network of bacterial taxa potentially involved in propionate production, suggesting that this SCFA may arise from an augmented production of succinate by Bacteroides/Parabacteroides, and its conversion into propionate by Phascolarctobacterium.Citation35
Thirdly, although there was a depletion in some species known as traditional probiotics in both clusters, i.e., Bifidobacterium bifidum (ASV_38), Bifidobacterium longum (ASV_226), or no significant stimulation, as with Lactobacillus, we identified an improved and significant capacity in C2 to enhance the relative abundance of some species suggested to act as potential 2nd generation probiotics.Citation49 It is the case of the Bacteroidia class, which prevailed specifically in C2: Bacteroides xylanisolvensCitation50 ASV_84 (lumen), 122 and 154 (mucus), and Bacteroides ovatusCitation51 ASV_58, 130 in lumen/mucus, were all increased, as was Akkermansia muciniphilaCitation52 (ASV_107) in the L_AC and M_AC.
Forth, we observed that alterations in the mucus-attached microbiota were relatively subtle, with only a few taxa showing significant changes, which varied notably between the clusters. It is worth noting that in a prior study utilizing the M-SHIME system with EPA- and DHA-rich oil, a much more pronounced modification of the mucus-associated niche was reported, though there, as here, to a lesser extent than in the lumen.Citation19 This divergence in results is interesting, particularly when considering the concept of prebiotic properties, defined as substances selectively utilized by host microorganisms to confer health benefits.Citation40 As per this definition, it is anticipated that the impact on the gut bacteriome would be specific for different n-3 PUFAs, rather than affecting the entire microbial ecosystem similarly. In this context, our findings suggest a more targeted and selective influence of BO, aligning with the criteria accepted to define a food supplement as a prebiotic.
Finally, and perhaps the most important finding of this study, we demonstrated the capacity of gut microbiota to serve as a bio-factory to produce host-targeting endocannabinoid-like molecules. In the context of multi-compartmented gut systems, a key challenge in interpreting metabolomics data is represented by the need of distinguishing between actual metabolite production and potential accumulation based on long hydraulic retention times or repeated transfer between bioreactors, and dilution levels. Our team recently developed a mathematical modeling approach based on a set of ordinary differential equations, which enhances, for the first time, our capacity to decipher the fate of metabolic compounds originating from dietary supplements in complex systems such as the M-SHIME.Citation53 By applying this method here, we could make sure the observed effects of BO on the SHIME metabolome were not biased by the above confounding factors. Indeed, we found that the oil unmasks the capability of microorganisms from L_IL and, more effectively, the L_AC to generate host-cell targeting endocannabinoid-like molecules, and specifically SDEA, from its most abundant fatty acids, SDA. While non-endocannabinoid N-acylethanolamines (NAEs) such as SDEA, by definition, do not strongly bind to cannabinoid receptors, they do activate other targets, such as peroxisome proliferator-activated receptor α (PPARα), a nuclear receptor playing an important role in the regulation of hepatic lipogenesis, and accordingly reduce lipid accumulation in hepatocytes (Flamand, Silvestri and Di Marzo, paper in preparation). These are two effects often reported in vivo for long chain n-3 PUFAs biosynthesized in mammals from SDA, i.e., EPA and DHA.Citation54 Here, we suggest that dietary SDA may influence host lipid metabolism also through its role as an SDEA precursor, and that such property can be exerted also through the intermediacy of the gut microbiome, thus opening new avenues in the possible therapeutic exploitation of this n-3 PUFA and its dietary sources. Recent research has shown that elevated levels of NAEs derived from arachidonic, oleic, linoleic, and palmitic acid are present in the stool of patients with inflammatory bowel disease, and that they increase the growth of Proteobacteria at the expense of Bacteroidetes in vitro.Citation25 However, while demonstrating that gut bacteria can affect the efflux and metabolism of NAEs in culture, these authors did not report that these microorganisms can produce these molecules. Thus, our study is the first to describe the actual formation of NAEs by gut microbiota. Further investigations are now needed to determine whether SDEA, like other NAEs25, also affects bacterial growth on top of the aforementioned beneficial effect in hepatocytes.
It is noteworthy that SDEA formation in the M-SHIME differed among the four donors. Accordingly, SDEA levels were positively associated with genera such as Butyricicoccus, Campylobacter and Phascolarctobacterium that were most abundant in donors with the highest capability of producing SDEA. Future studies will be needed to investigate if these genera include species capable of expressing the enzymes necessary for the conversion of PUFAs into the corresponding NAEs, such as N-acyltransferases and fatty acid amidases working in reverse. Using Indeed, according to the InterPro database,Citation55 Anaeroglobus geminatus, a species found in C1 was identified with the potential for a UDP-3-O-[3-hydroxymyristoyl] glucosamine N-acyltransferase LpxD activity, while some Bacillota, potentially Phascolartobacterium, abundant in C2, possess a Glycine N-acyltransferase. However, analyses at the strain level are necessary to gain mechanistic insights in PUFAs conversion into NAEs by gut microbiota. These will clarify why our M-SHIME microbiota preferred to produce the ethanolamide of SDA vs those of other fatty acid components of the oil. Furthermore, it will be important to understand whether SDEA is produced by bacteria, or other members of the gut microbiome, such as fungi.Citation56
SDA transformation into SDEA was not the only mechanism through which BO stimulated the production of endocannabinoid-like molecules by the human microbiome in the M-SHIME, since we also observed the increased formation of commendamide, whose fatty acid precursor is not detectable in the oil. Various lipids, including glycine lipids, plasmalogens, glycerophospholipids and sphingolipids, have been identified in members of the Bacteroidota phylum.Citation57,Citation58 Commendamide (N-acyl-3-hydroxy-palmitoyl glycine) is structurally similar to long-chain N-acyl-amides and activates G-protein – coupled receptors (GPCRs), including GPCR G2A/GPR132, involved in various cellular responses.Citation26,Citation59 Our observation of a significant enrichment of Bacteroides in both clusters following BO led us to investigate the production of this metabolite, which was indeed increased in L_AC and, particularly, L_IL. Accordingly, our Spearman’s correlation analysis uncovered a strong positive correlation between commendamide and multiple Bacteroides species, i.e., B. vulgatus and B. dorei, two species known to natively biosynthesize this compound,Citation26 as well as B. distasonis, which has never been reported to produce it.
In conclusion, this study shows that the potential health benefits of n-3 PUFA-containing oils on the human bacteriome encompass individual-dependent effects on both its taxonomic composition and metabolic properties and extend beyond PUFAs to endocannabinoid-like mediators. While this paper does not delve into the bioactivity of these metabolites Citation26,Citation59 , it is currently under investigation in pre-clinical and clinical models of metabolic disorders in our laboratory. We anticipate that the human ex vivo data presented here will pave the way to future dietary intervention studies exploring potential therapeutic effects of SDA-containing oils. Our ex vivo data in four donors are clearly not directly translatable to mechanisms occurring in vivo in larger populations, but should be viewed as: 1) evidence on novel potential mechanisms through which dietary oils can affect gut microbiota and their metabolome, and 2) proof of concept requiring deeper explorations of crucial individual variations in microbiota and lipid metabolism in response to such dietary interventions, through the enrollment of larger cohorts of donors and the study of their gut microbiota using high throughput fermentation systems. This will lead to identify specific populations that may experience the benefits of this fatty acid or Buglossoides arvensis oil, either directly or via the formation of SDEA and other endocannabinoid-like metabolites. This concept aligns with the paradigm of precision nutrition, aiming to stratify supplementation strategies based on the enterotype and gut metabotype of each participant.Citation60
Methods
Gut fermentation system
The M-SHIME® (Prodigest, Gent, Belgium), was run simultaneously with two anaerobic computer-controlled TWIN-M-SHIME units in semi-continuous mode.Citation34 In this study, a SHIME unit comprised a series of pH-controlled vessels, including a vessel for the stomach/proximal small intestine, which mimicked successively the gastric acid digestion of a standardized nutritional medium followed by the delivery of pancreatic/bile juices. The next vessels simulated the transit and bacterial microbiota composition of an ileum and an ascending colon. To capture inter-individual variability in bacterial microbiota composition, lipid metabolism, and response to the vegetal oil supplement, fecal bacterial microbiotas collected from four healthy adults (ranging from 25 to 48 years old), without antibiotic history were tested in duplicate (n = 8) (Supp Table S1, ). Consent for fecal donation was obtained under registration number 2022–382/17-11-2022 (Laval University, Québec, Canada). Fecal material collection and inoculum preparation respect the well-established natural procedure.Citation61 In addition, the procedure for establishing an ileal microbial community at low biomass concentration has been described elsewhere.Citation19 Outer mucus-associated bacterial microbiotas of the ileum and ascending colon were mimicked through the incorporation of microcosms (AnoxKaldnes K1 carrier, Lund, Sweden) coated with type II porcine MUC2 gel-forming mucin (Carl Roth, Karlsruhe, Germany) prepared with agar. General functioning of the system, mucin carrier replacement and media composition have been presented in Roussel et al.Citation19,Citation62
Buglossoides oil addition in the SHIME
The TWIN-M-SHIME® fermentation was performed for 35 days including a 14-day stabilization period, a 7-day control diet followed by a 14-day n-3 BO supplementation. This non-GMO AhiflowerTM seed oil (Natures Crops International, Kensington, Canada) consisted in the supplementation of 1200 mg oil (2 capsules)/day/donor in the SHIME stomach, containing up to 21% of SDA (Supp Table S2). Both periods, CTRL and BO were supplemented with an emulsifier containing 0.38% (w/v) lecithin (Sigma-Aldrich, St. Louis, US) to help solubilizing the oil (). All vessels were protected from light source to prevent photo-oxidation of the oil.
Sample collection and storage
SHIME suspensions from the different gut vessels were sampled every 3 days, centrifuged at 4°C, 18 000 × g for 8 min for subsequent SCFA (supernatant) and DNA (pellet) analysis, and stored at −20°C. Outer mucus-associated bacterial microbiota samples from microcosms were obtained every 2–3 days.Citation62 Aliquots of 125 mg mucus from the ileum vessel, and 250 mg from the colon vessel were taken with a mini sampler spoon (Bel ArtTM SciencewareTM, Thermo Fisher Scientific, Waltham, US) and stored at −20°C prior to DNA extraction. Samples for targeted lipidomic were taken every 3 days and stored at −80°C ().
Bacterial DNA extraction from feces and SHIME samples
Samples preparation was performed as previously described.Citation63 Briefly, 500 μL of fecal inoculum suspension or SHIME effluents were stained with 1.25 μL of propidium monoazide (PMA, 50 μM) (Biotium, Fremont, Canada) to inactivate dead bacterial DNA. DNA was pelleted (8 min, 18 000 × g, 4°C), and extracted according to Geirnaert et al.Citation64 In the mucus microcosm samples, an additional step was performed to increase DNA yield as previously described.Citation19 DNA extracts were eluted in 1X TE buffer (Tris and EDTA). The quality of DNA was analyzed by gel electrophoresis (1.2% w/v agarose) (Life Technologies, Madrid, Spain). Concentrations were measured by Qubit (Thermo Fisher Scientific, Waltham, US) and the DNA were stored at −20°C, until 16S rRNA gene library preparation.
16S rRNA gene amplicon sequencing
Library preparation and sequencing were performed as described in detail previously,Citation19 using a subset of 4 fecal inoculum samples, 134 luminal samples and 127 mucus samples from the ileum and ascending colon vessels, and 3 blank buffer negative controls. Briefly, the QIAseq 16S Region Panel protocol in conjunction with the QIAseq 16S/ITS 384-Index I kit (Qiagen, Hilden, Germany) were used for amplification and indexing of the V3-V4 region of the 16S rRNA gene (341F-805 R) for all DNA samples. Sequencing was performed with pooled samples, diluted to a final concentration of 10 pM using the MiSeq 600 cycles Reagent Kit V3 (Illumina, San Diego, US) by an Illumina MiSeq System (Illumina, San Diego, US).
Gut microbial community analysis
Demultiplexed raw data files covering all the samples were imported into R studio environment (version 4.2.2, R Core Team). ASVs were inferred using the DADA2 R package version 1.26.0, applying the recommended workflow.Citation65 Briefly, sequence reads were first filtered and trimmed with the following parameters: truncQ = 2, truncLen=c(255,220), maxEE=c.2,2 Filtered reads were denoised using the DADA2 algorithm, which infers the sequencing errors. The reads were merged if they overlapped precisely, and an ASVs table was constructed, recording the number of times each ASV was observed in each sample. Default parameters were used to estimate error rates using learnErrors, and chimeras were removed using removeBimeraDenova (method = “consensus”). ASVs were assigned taxonomy using the most recent SILVA taxonomic database (SILVA SSURef 138.1 NR, March 2021) as a reference dataset. Unassigned taxa and singletons were removed. ASV sequences non-assigned at species level with SILVA database were retrieved using the RDP sequence match taxonomy version 18 with shared 7-mers score (S_ab score) over 0,96. To deal with differences in sampling depth, the data were rescaled to proportions for further analysis.
Amplicon data statistics and reproducibility
All statistical analyses were performed in R 4.2.2 (R Core Team, 2022) with full factorial (biological and technical) replication. Only 4 samples from the 268 selected samples were excluded from the bacterial microbiota analysis due to low read counts, including 2 blank buffer negative controls. If data were normally distributed (evaluated by visual inspection and D’Agostino – Pearson test), parametric statistical tests were used (T-test), whereas non-parametric tests were used with non-normally distributed data.
The overall data were graphically visualized using ggplot2 3.4.1 and ggrepel 0.9.3, and means statistics were compared using ggpubr 0.6.0, unless otherwise indicated. All formal hypothesis tests were conducted on the 5% significance level.
The α-diversity (Shannon, Chao1 indexes), and β-diversity were determined using the “vegan” R package 2.6–4.Citation66 To test the influence of a set of variables contributing to the bacterial microbiota dissimilarity (condition, donor, time, and gut niche), constrained ordination technique was used using the capscale function. The generated Bray-Curtis dissimilarity matrix retrieved from the “vegdist” function was used to perform a distance-based redundancy analysis (db-RDA) at the genus level.Citation66 Interpretation of the results was preceded by a permutation test of the RDA results to confirm that a linear relationship exists between the response data and the exploratory variables. The constrained fraction of the variance explained by the exploratory variables was adjusted by applying Ezekiel’s formula. The significance of the observed group separation was assessed with a Permutational Multivariate Analysis of Variance (PERMANOVA) using distance matrixes (vegan 2.6–4).Citation66 Prior to this formal hypothesis testing, the assumption of similar multivariate dispersions was evaluated. The results of the db-RDA were visualized in a type 2 scaling correlation triplot.
To determine the enriched and depleted genera abundance between periods in different gut regions and donors/clusters, the DESeq2 package 1.38.3 was applied.Citation67,Citation68 Rawdata counts were normalized using the function DESeq2:counts and converted into log2 fold change. The factors periods, gut regions, and donors/clusters were used in the design of LRT formula. The function lfcShrink type “apeglm” was used to reduce false positives. Statistical differences were determined using a Wald Test. P-values were adjusted for multiple testing using the Benjamini-Hochberg procedure. Significant differences were visualized in a volcanoplot, showing the -log10(adjusted p-value) as a function of the shrunken log2 Fold Change.Citation69
Assessing ASVs sharing and mucus engraftment rates
The ASVs count data table was converted to presence – absence binary data to calculate the ASVs sharing rate (SR) between donors per gut region, as adapted from Laniro et al:Citation70
The mucus engraftment rate (MER) per gut regions was calculated as
Bacterial microbiota clustering method
Donors’ bacterial microbiota clustering was performed at the genus level. Several methods including K-means algorithm (package ClusterSim 0.50–1), hierarchical clustering with Pearson distance were used (package factoextra 1.0.7). To further determine the reliability of the generated clusters, silhouette coefficient was calculated (package ClusterSim 0.50–1), as well as the optimal number of clusters using the Calinski-Harabasz (CH) index (package fpc 2.2–10).Citation71 Final data were visualized using ComplexHeatmap package 2.14.0.
Microbial co-occurrence analysis
Inter-genus correlations were examined using Spearman correlations with p-adjusted FDR for robust control of false discovery rates (package corrplot 0.92). Networks were built using Cytoscape 3.9.1, focusing solely on significant relationships with an absolute Spearman coefficient exceeding 0.7. Additionally, to summarize some specific information related on number of taxa shared between conditions, we used scalable alternative to Venn diagram with informative UpSetR graphs (package UpSetR 1.4.0), considering exclusively positive and significant correlations with Spearman coefficients greater than 0.7.
SCFA profiling using gas chromatography and correlations
SHIME effluents from ileum and colon vessels (125 μL) were centrifuged at 18 000 × g for 8 minutes at 4°C. SCFAs were extracted with diethyl ether and analyzed through a gas chromatograph equipment coupled to a flame ionization detector as previously described.Citation72 SCFA concentration was expressed in mM. SCFA ratios of the major (acetate, propionate, and butyrate), and the minor (branched SCFAs, and the medium CFAs valerate and caproate) SCFAs were determined. Spearman correlations were used to examine the relationships between bacterial genera and SCFA, and visualized with heatmaps (package corrplot 0.92), highlighting significant associations with p-values FDR adjusted.
Endocannabinoid-like molecule profiling using HPLC-MS/MS
The analysis of MAGs and NAEs was done by mixing 500 µL samples with 500 µL Tris buffer (50 mM, pH 7) then processed as described before.Citation73 Samples were then analyzed using the analytical method described before.Citation74 For the analysis of commendamide, 25 µL samples were mixed with 475 µL Tris containing 2 ng commendamide-d2. Samples then were extracted and analyzed by LC-MS/MS using a linear gradient of 40% solvent A (water +1 mM ammonium acetate + 0.05% acetic acid) and solvent B (acetonitrile/water 95/5 + 1 mM ammonium acetate + 0.05% acetic acid) over 5 minutes. Spearman correlations were used to examine the relationships between bacterial genera and lipids mediators as described on top.
Mathematical modeling of lipid mediator production in the M-SHIME
To assess whether the concentration changes of the different lipid mediators were solely due to the fluid dynamics (accumulation/dilution due to the transfers during each meal) or also to the gut microbial metabolism, we calculated the concentration of the different lipid mediators at each timepoints, as described thoroughly elsewhere. In brief, we modeled the concentration changes due to the fluid dynamics of the different lipid mediators in the proximal colon with a set of ordinary differential equations.Citation53 The maximal concentration measured in the duodenum was used in the model.
Authors’ contributions
C.R., C.S., and V.D. had full access to all the data in the study and accept the responsibility for the integrity of the data and the accuracy of the data analyses. C.R., C.S., and V.D. designed the study. C.S. and V.D. supervised the work. C.R., and P.G. conducted the in vitro fermentations and quality control. C.R. conducted the molecular-based experiments, and metagenomics sequencing. N.F. and J.L.L. conducted the lipidomics analysis. J.L.L. conducted the mathematical modeling. O.A. and N.F. developed and employed the commendamide analytical method. R.V. conducted the chemical synthesis of commendamide and its analogues in deuterated and non-deuterated forms. C.R. and M.S. performed the bioinformatics and data analysis. C.R. interpreted the overall data and wrote the manuscript first draft. C.R., C.S., V.D., N.F., F.R. and J.L.L. revised the manuscript.
Availability of data and materials
All data generated or analyzed during this study are included in this published article (and its supplementary information files). All 16S rRNA gene amplicon sequencing data were deposited in the Sequence Read Archive (SRA) under the BioProject PRJNA836582.
Ethics approval
Consent for fecal donation was obtained under registration number 2022–382/17-11-2022 (Laval University, Québec, Canada).
Ahiflower_Supp_Data_clean.docx
Download MS Word (7.9 MB)Acknowledgments
The authors are grateful to INAF analytical and metabolomic platforms and would like to acknowledge Pier-Luc Plante for injecting the lipid compounds, and Roxanne Nolet and Perrine Feutry for their assistance in SCFAs measurements. We are also grateful to Elizabeth Dumais for providing an updated procedure of the lipid extraction and calibration. Finally, we thank Joseph Lupien-Meilleur for his help in creating the figure of the study design.
Disclosure statement
No potential conflict of interest was reported by the author(s).
Supplementary material
Supplemental data for this article can be accessed online at https://doi.org/10.1080/19490976.2024.2335879
Additional information
Funding
References
- Santos HO, Price JC, Bueno AA. Beyond fish oil supplementation: the effects of alternative plant sources of omega-3 polyunsaturated fatty acids upon lipid indexes and cardiometabolic biomarkers—an overview. Nutrients. 2020;12(10):3159. doi:10.3390/nu12103159.
- Naylor RL, Hardy RW, Buschmann AH, Bush SR, Cao L, Klinger DH, Little DC, Lubchenco J, Shumway SE, Troell M. et al. A 20-year retrospective review of global aquaculture. Nature. 2021;591(7851):551–23. doi:10.1038/s41586-021-03308-6.
- Lane KE, Wilson M, Hellon TG, Davies IG. Bioavailability and conversion of plant based sources of omega-3 fatty acids – a scoping review to update supplementation options for vegetarians and vegans. Crit Rev Food Sci. 2022;62(18):4982–4997. doi:10.1080/10408398.2021.1880364.
- Coupland K. Stearidonic acid: a plant produced omega‐3 PUFA and a potential alternative for marine oil fatty acids. Lipid Technol. 2008;20(7):152–154. doi:10.1002/lite.200800045.
- Liao J, Xiong Q, Yin Y, Ling Z, Chen S. The effects of fish oil on cardiovascular diseases: systematical evaluation and recent advance. Front Cardiovasc Med. 2022;8:802306. doi:10.3389/fcvm.2021.802306.
- Kerdiles O, Layé S, Calon F. Omega-3 polyunsaturated fatty acids and brain health: preclinical evidence for the prevention of neurodegenerative diseases. Trends Food Sci Tech. 2017;69:203–213. doi:10.1016/j.tifs.2017.09.003.
- Liao Y, Xie B, Zhang H, He Q, Guo L, Subramanieapillai M, Fan B, Lu C, McIntyre RS. Efficacy of omega-3 PUFAs in depression: a meta-analysis. Transl Psychiat. 2019;9(1):190. doi:10.1038/s41398-019-0515-5.
- Komal F, Khan MK, Imran M, Ahmad MH, Anwar H, Ashfaq UA, Ahmad N, Masroor A, Ahmad RS, Nadeem M. et al. Impact of different omega-3 fatty acid sources on lipid, hormonal, blood glucose, weight gain and histopathological damages profile in PCOS rat model. J Transl Med. 2020;18(1):349. doi:10.1186/s12967-020-02519-1.
- Freitas RDS, Campos MM. Protective effects of omega-3 fatty acids in cancer-related complications. Nutrients. 2019;11(5):945. doi:10.3390/nu11050945.
- Li Y, Lai W, Zheng C, Babu JR, Xue C, Ai Q, Huggins KW. Neuroprotective effect of stearidonic acid on amyloid β-induced neurotoxicity in rat hippocampal cells. Antioxidants. 2022;11(12):2357. doi:10.3390/antiox11122357.
- Lefort N, LeBlanc R, Surette ME. Dietary Buglossoides arvensis oil increases circulating n-3 polyunsaturated fatty acids in a dose-dependent manner and Enhances Lipopolysaccharide-Stimulated Whole Blood Interleukin-10—A randomized placebo-controlled trial. Nutrients. 2017;9(3):261. doi:10.3390/nu9030261.
- Whelan J, Gouffon J, Zhao Y. Effects of dietary stearidonic acid on biomarkers of lipid Metabolism4. J Nutrition. 2012;142(3):630S–634S. doi:10.3945/jn.111.149138.
- Prasad P, Anjali P, Sreedhar RV. Plant-based stearidonic acid as sustainable source of omega-3 fatty acid with functional outcomes on human health. Crit Rev Food Sci. 2021;61(10):1725–1737. doi:10.1080/10408398.2020.1765137.
- Greupner T, Koch E, Kutzner L, Hahn A, Schebb NH, Schuchardt JP. Single-dose SDA-Rich echium oil increases plasma EPA, DPAn3, and DHA concentrations. Nutrients. 2019;11(10):2346. doi:10.3390/nu11102346.
- Walker CG, Jebb SA, Calder PC. Stearidonic acid as a supplemental source of ω-3 polyunsaturated fatty acids to enhance status for improved human health. Nutrition. 2013;29(2):363–369. doi:10.1016/j.nut.2012.06.003.
- Cumberford G, Hebard A. Ahiflower oil: a novel non‐GM plant‐based omega‐3+6 source. Lipid Technol. 2015;27(9):207–210. doi:10.1002/lite.201500044.
- Myers A, Cumberford G. Ahiflower Oil-The Rising GLA Alternative to evening primrose for women & vegans. Integr Medicine Encinitas Calif. 2021;20:30–33.
- Fu Y, Wang Y, Gao H, Li D, Jiang R, Ge L, Tong C, Xu K. Associations among dietary omega-3 polyunsaturated fatty acids, the gut microbiota, and intestinal immunity. Mediat Inflamm. 2021;2021:1–11. doi:10.1155/2021/8879227.
- Roussel C, Guebara SAB, Plante PL, Desjardins Y, Marzo VD, Silvestri C. Short-term supplementation with ω-3 polyunsaturated fatty acids modulates primarily mucolytic species from the gut luminal mucin niche in a human fermentation system. Gut Microbes. 2022;14(1):2120344. doi:10.1080/19490976.2022.2120344.
- Brown EM, Clardy J, Xavier RJ. Gut microbiome lipid metabolism and its impact on host physiology. Cell Host Microbe. 2023;31(2):173–186. doi:10.1016/j.chom.2023.01.009.
- Menni C, Zierer J, Pallister T, Jackson MA, Long T, Mohney RP, Steves CJ, Spector TD, Valdes AM. Omega-3 fatty acids correlate with gut microbiome diversity and production of N-carbamylglutamate in middle aged and elderly women. Sci Rep-Uk. 2017;7(1):11079. doi:10.1038/s41598-017-10382-2.
- Druart C, Bindels LB, Schmaltz R, Neyrinck AM, Cani PD, Walter J, Ramer‐Tait AE, Delzenne NM. Ability of the gut microbiota to produce PUFA‐derived bacterial metabolites: proof of concept in germ‐free versus conventionalized mice. Mol Nutr Food Res. 2015;59(8):1603–1613. doi:10.1002/mnfr.201500014.
- Silvestri C, Marzo VD. The gut microbiome–endocannabinoidome axis: a new way of controlling metabolism, inflammation, and behavior. Function. 2023;4(2):zqad003. doi:10.1093/function/zqad003.
- Tagliamonte S, Gill CIR, Pourshahidi LK, Slevin MM, Price RK, Ferracane R, Lawther R, O’Connor G, Vitaglione P. Endocannabinoids, endocannabinoid-like molecules and their precursors in human small intestinal lumen and plasma: does diet affect them? Eur J Nutr. 2021;60(4):2203–2215. doi:10.1007/s00394-020-02398-8.
- Fornelos N, Franzosa EA, Bishai J, Annand JW, Oka A, Lloyd-Price J, Arthur TD, Garner A, Avila-Pacheco J, Haiser HJ. et al. Growth effects of N-acylethanolamines on gut bacteria reflect altered bacterial abundances in inflammatory bowel disease. Nat Microbiol. 2020;5(3):486–497. doi:10.1038/s41564-019-0655-7.
- Cohen LJ, Kang HS, Chu J, Huang YH, Gordon EA, Reddy BVB, Ternei MA, Craig JW, Brady SF. Functional metagenomic discovery of bacterial effectors in the human microbiome and isolation of commendamide, a GPCR G2A/132 agonist. Proc Natl Acad Sci. 2015;112(35):E4825–34. doi:10.1073/pnas.1508737112.
- Lynch A, Crowley E, Casey E, Cano R, Shanahan R, McGlacken G, Marchesi JR, Clarke DJ. The Bacteroidales produce an N-acylated derivative of glycine with both cholesterol-solubilising and hemolytic activity. Sci Rep-Uk. 2017;7(1):13270. doi:10.1038/s41598-017-13774-6.
- Sihag J, Marzo VD. (Wh)olistic (E)ndocannabinoidome-microbiome-axis modulation through (N)utrition (WHEN) to Curb Obesity and related disorders. Lipids Heal Dis. 2022;21(1):9. doi:10.1186/s12944-021-01609-3.
- Cani PD, Plovier H, Hul MV, Geurts L, Delzenne NM, Druart C, Everard A. Endocannabinoids — at the crossroads between the gut microbiota and host metabolism. Nat Rev Endocrinol. 2016;12(3):133–143. doi:10.1038/nrendo.2015.211.
- Venema K, Verhoeven J, Beckman C, Keller D. Survival of a probiotic-containing product using capsule-within-capsule technology in an in vitro model of the stomach and small intestine (TIM-1). Benef Microbes. 2020;11(4):403–409. doi:10.3920/BM2019.0209.
- Vandeputte D. Personalized nutrition through the gut microbiota: Current insights and future perspectives. Nutr Rev. 2020;78(Supplement_3):66–74. doi:10.1093/nutrit/nuaa098.
- Kolodziejczyk AA, Zheng D, Elinav E. Diet–microbiota interactions and personalized nutrition. Nat Rev Microbiol. 2019;17(12):742–753. doi:10.1038/s41579-019-0256-8.
- Park JC, Im SH. Of men in mice: the development and application of a humanized gnotobiotic mouse model for microbiome therapeutics. Exp Mol Med. 2020;52(9):1383–1396. doi:10.1038/s12276-020-0473-2.
- de Wiele TV, den Abbeele PV, Ossieur W, Possemiers S, Marzorati M. The Impact of Food Bioactives on health, in vitro and ex vivo models. 2015;305–317.
- Ikeyama N, Murakami T, Toyoda A, Mori H, Iino T, Ohkuma M, Sakamoto M. Microbial interaction between the succinate‐utilizing bacterium phascolarctobacterium faecium and the gut commensal bacteroides thetaiotaomicron. Microbiologyopen. 2020;9(10):e1111. doi:10.1002/mbo3.1111.
- Wang K, Liao M, Zhou N, Bao L, Ma K, Zheng Z, Wang Y, Liu C, Wang W, Wang J. et al. Parabacteroides distasonis alleviates obesity and metabolic dysfunctions via production of succinate and secondary bile acids. Cell Rep. 2019;26(1):222–235.e5. doi:10.1016/j.celrep.2018.12.028.
- Fernández-Veledo S, Vendrell J. Gut microbiota-derived succinate: friend or foe in human metabolic diseases? Rev Endocr Metabolic Disord. 2019;20(4):439–447. doi:10.1007/s11154-019-09513-z.
- Tsukuda N, Yahagi K, Hara T, Watanabe Y, Matsumoto H, Mori H, Higashi K, Tsuji H, Matsumoto S, Kurokawa K. et al. Key bacterial taxa and metabolic pathways affecting gut short-chain fatty acid profiles in early life. ISME J. 2021;15(9):2574–2590. doi:10.1038/s41396-021-00937-7.
- David LA, Maurice CF, Carmody RN, Gootenberg DB, Button JE, Wolfe BE, Ling AV, Devlin AS, Varma Y, Fischbach MA. et al. Diet rapidly and reproducibly alters the human gut microbiome. Nature. 2014;505(7484):559–563. doi:10.1038/nature12820.
- Gibson GR, Hutkins R, Sanders ME, Prescott SL, Reimer RA, Salminen SJ, Scott K, Stanton C, Swanson KS, Cani PD. et al. Expert consensus document: the International Scientific Association for Probiotics and prebiotics (ISAPP) consensus statement on the definition and scope of prebiotics. Nat Rev Gastroenterol Hepatol. 2017;14(8):491–502. doi:10.1038/nrgastro.2017.75.
- Marzo VD. New approaches and challenges to targeting the endocannabinoid system. Nat Rev Drug Discov. 2018;17(9):623–639. doi:10.1038/nrd.2018.115.
- de Vos WM, Tilg H, Hul MV, Cani PD. Gut microbiome and health: mechanistic insights. Gut. 2022;71(5):1020–1032. doi:10.1136/gutjnl-2021-326789.
- Lozupone CA, Stombaugh JI, Gordon JI, Jansson JK, Knight R. Diversity, stability and resilience of the human gut microbiota. Nature. 2012;489(7415):220–230. doi:10.1038/nature11550.
- Hotea I, Sirbu C, Plotuna AM, Tîrziu E, Badea C, Berbecea A, Dragomirescu M, Radulov I. Integrating (nutri-)metabolomics into the one health tendency—the key for personalized medicine advancement. Metabolites. 2023;13(7):800. doi:10.3390/metabo13070800.
- McCallum G, Tropini C. The gut microbiota and its biogeography. Nat Rev Microbiol. 2023;22(2):105–118. doi:10.1038/s41579-023-00969-0.
- Lim RRX, Park MA, Wong LH, Haldar S, Lim KJ, Nagarajan N, Henry CJ, Jiang YR, Moskvin OV. Gut microbiome responses to dietary intervention with hypocholesterolemic vegetable oils. npj Biofilms Microbiomes. 2022;8(1):24. doi:10.1038/s41522-022-00287-y.
- Li W, Li L, Yang F, Hu Q, Xiong D. Correlation between gut bacteria phascolarctobacterium and exogenous metabolite α-linolenic acid in T2DM: a case-control study. Ann Transl Med. 2022;10(19):1056–0. doi:10.21037/atm-22-3967.
- Chambers ES, Viardot A, Psichas A, Morrison DJ, Murphy KG, Zac-Varghese SEK, MacDougall K, Preston T, Tedford C, Finlayson GS. et al. Effects of targeted delivery of propionate to the human colon on appetite regulation, body weight maintenance and adiposity in overweight adults. Gut. 2015;64(11):1744. doi:10.1136/gutjnl-2014-307913.
- O’Toole PW, Marchesi JR, Hill C. Next-generation probiotics: the spectrum from probiotics to live biotherapeutics. Nat Microbiol. 2017;2(5):17057. doi:10.1038/nmicrobiol.2017.57.
- Brodmann T, Endo A, Gueimonde M, Vinderola G, Kneifel W, de Vos WM, Salminen S, Gómez-Gallego C. Safety of novel microbes for human consumption: practical examples of assessment in the European Union. Front Microbiol. 2017;8:1725. doi:10.3389/fmicb.2017.01725.
- Horvath TD, Ihekweazu FD, Haidacher SJ, Ruan W, Engevik KA, Fultz R, Hoch KM, Luna RA, Oezguen N, Spinler JK. et al. Bacteroides ovatus colonization influences the abundance of intestinal short chain fatty acids and neurotransmitters. iScience. 2022;25(5):104158. doi:10.1016/j.isci.2022.104158.
- Cani PD, Depommier C, Derrien M, Everard A, de Vos WM. Akkermansia muciniphila: paradigm for next-generation beneficial microorganisms. Nat Rev Gastroenterol Hepatol. 2022;19(10):625–637. doi:10.1038/s41575-022-00631-9.
- Lessard-Lord J, Lupien-Meilleur J, Roussel C, Gosselin-Cliche B, Silvestri C, Marzo VD, Roy D, Rousseau E, Desjardins Y. Mathematical modeling of fluid dynamics in in vitro gut fermentation systems: a new tool to improve the interpretation of microbial metabolism. FASEB J. 2023;38(2). doi:10.1096/fj.202301739RR.
- de Vogel-van den Bosch HM, Bünger M, de Groot PJ, Bosch-Vermeulen H, Hooiveld GJ, Müller M. Pparalpha-mediated effects of dietary lipids on intestinal barrier gene expression. BMC Genom. 2008;9(1):231. doi:10.1186/1471-2164-9-231.
- Paysan-Lafosse T, Blum M, Chuguransky S, Grego T, Pinto BL, Salazar GA, Bileschi M, Bork P, Bridge A, Colwell L. et al. InterPro in 2022. Nucleic Acids Res. 2022;51(D1):D418–27. doi:10.1093/nar/gkac993.
- Merkel O, Schmid PC, Paltauf F, Schmid HHO. Presence and potential signaling function of N-acylethanolamines and their phospholipid precursors in the yeast Saccharomyces cerevisiae. Biochim Biophys Acta (BBA) - Mol Cell Biol Lipids. 2005;1734(3):215–219. doi:10.1016/j.bbalip.2005.03.004.
- Ryan E, Joyce SA, Clarke DJ. Membrane lipids from gut microbiome-associated bacteria as structural and signalling molecules. Microbiology. 2023;169(3):micro001315. doi:10.1099/mic.0.001315.
- Ryan E, Pastor BG, Gethings LA, Clarke DJ, Joyce SA. Lipidomic Analysis Reveals Differences in Bacteroides Species driven largely by Plasmalogens, glycerophosphoinositols and certain sphingolipids. Metabolites. 2023;13(3):360. doi:10.3390/metabo13030360.
- Cohen LJ, Esterhazy D, Kim SH, Lemetre C, Aguilar RR, Gordon EA, Pickard AJ, Cross JR, Emiliano AB, Han SM. et al. Commensal bacteria make GPCR ligands that mimic human signalling molecules. Nature. 2017;549(7670):48–53. doi:10.1038/nature23874.
- Costea PI, Hildebrand F, Arumugam M, Bäckhed F, Blaser MJ, Bushman FD, de Vos WM, Ehrlich S, Fraser CM, Hattori M. et al. Enterotypes in the landscape of gut microbial community composition. Nat Microbiol. 2018;3(1):8–16. doi:10.1038/s41564-017-0072-8.
- Pérez-Burillo S, Molino S, Navajas-Porras B, Valverde-Moya ÁJ, Hinojosa-Nogueira D, López-Maldonado A, Pastoriza S, Rufián-Henares JÁ. An in vitro batch fermentation protocol for studying the contribution of food to gut microbiota composition and functionality. Nat Protoc. 2021;16(7):3186–3209. doi:10.1038/s41596-021-00537-x.
- den Abbeele PV, Roos S, Eeckhaut V, MacKenzie DA, Derde M, Verstraete W, Marzorati M, Possemiers S, Vanhoecke B, Van Immerseel, F. et al. Incorporating a mucosal environment in a dynamic gut model results in a more representative colonization by lactobacilli. Microb Biotechnol. 2012;5(1):106–115. doi:10.1111/j.1751-7915.2011.00308.x.
- Roussel C, Galia W, Leriche F, Chalancon S, Denis S, de Wiele TV, Blanquet-Diot S. Comparison of conventional plating, PMA-qPCR, and flow cytometry for the determination of viable enterotoxigenic Escherichia coli along a gastrointestinal in vitro model. Appl Microbiol Biotechnol. 2018;102(22):9793–9802. doi:10.1007/s00253-018-9380-z.
- Geirnaert A, Wang J, Tinck M, Steyaert A, den AP, Eeckhaut V, Vilchez-Vargas R, Falony G, Laukens D, De Vos M. et al. Interindividual differences in response to treatment with butyrate-producing Butyricicoccus pullicaecorum 25–3T studied in an in vitro gut model. FEMS Microbiol Ecol. 2015;91(6):fiv054. doi:10.1093/femsec/fiv054.
- Callahan BJ, McMurdie PJ, Rosen MJ, Han AW, Johnson AJA, Holmes SP. DADA2: high-resolution sample inference from Illumina amplicon data. Nat Methods. 2016;13(7):581–583. doi:10.1038/nmeth.3869.
- (PDF) Vegan: Community Ecology Package. R package version 2.0-2 [internet]. [ accessed 2023 Apr 13]. https://www.researchgate.net/publication/282247686_Vegan_Community_Ecology_Package_R_package_version_20-2.
- McMurdie PJ, Holmes S, McHardy AC. Waste not, want not: why rarefying microbiome data is inadmissible. PLoS Comput Biol. 2014;10(4):e1003531. doi:10.1371/journal.pcbi.1003531.
- Love MI, Huber W, Anders S. Moderated estimation of fold change and dispersion for RNA-seq data with DESeq2. Genome Biol. 2014;15(12):550. doi:10.1186/s13059-014-0550-8.
- Paepe KD, Verspreet J, Verbeke K, Raes J, Courtin CM, de WT. Introducing insoluble wheat bran as a gut microbiota niche in an in vitro dynamic gut model stimulates propionate and butyrate production and induces colon region specific shifts in the luminal and mucosal microbial community. Environ Microbiol. 2018;20(9):3406–3426. doi:10.1111/1462-2920.14381.
- Ianiro G, Punčochář M, Karcher N, Porcari S, Armanini F, Asnicar F, Beghini F, Blanco-Míguez A, Cumbo F, Manghi P. et al. Variability of strain engraftment and predictability of microbiome composition after fecal microbiota transplantation across different diseases. Nat Med. 2022;28(9):1913–1923. doi:10.1038/s41591-022-01964-3.
- Arumugam M, Raes J, Pelletier E, Paslier DL, Yamada T, Mende DR, Fernandes GR, Tap J, Bruls T, Batto J-M. et al. Enterotypes of the human gut microbiome. Nature. 2011;473(7346):174–180. doi:10.1038/nature09944.
- Roussel C, Chabaud S, Lessard-Lord J, Cattero V, Pellerin FA, Feutry P, Bochard V, Bolduc S, Desjardins Y. UPEC colonic-virulence and urovirulence are blunted by proanthocyanidins-rich cranberry extract microbial metabolites in a gut Model and a 3D tissue-engineered urothelium. Microbiol Spectr. 2022;10(5):e02432–21. doi:10.1128/spectrum.02432-21.
- Manca C, Boubertakh B, Leblanc N, Deschênes T, Lacroix S, Martin C, Houde A, Veilleux A, Flamand N, Muccioli GG. et al. Germ-free mice exhibit profound gut microbiota-dependent alterations of intestinal endocannabinoidome signaling. J Lipid Res. 2020;61(1):70–85. doi:10.1194/jlr.RA119000424.
- Everard A, Plovier H, Rastelli M, Hul MV, de Wouters d’Oplinter A, Geurts L, Druart C, Robine S, Delzenne NM, Muccioli GG. et al. Intestinal epithelial N-acylphosphatidylethanolamine phospholipase D links dietary fat to metabolic adaptations in obesity and steatosis. Nat Commun. 2019;10(1):457. doi:10.1038/s41467-018-08051-7.