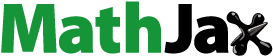
ABSTRACT
Crohn’s disease (CD) is a chronic inflammatory bowel disease associated with psychological distress and intestinal microbial changes. Here, we examined whether a 3-month period of Cognitive Behavioral and Mindfulness with Daily Exercise (COBMINDEX) intervention, which improves the wellbeing and inflammatory state of CD patients, may also affect their gut microbiome. Gut microbiota, circulating inflammatory markers and hormones were analyzed in 24 CD patients before (T1) and after 3 months of COBMINDEX (T2), and in 25 age- and sex-matched wait-list control patients at the corresponding time-points. Microbiota analysis examined relative taxonomical abundance, alpha and beta diversity, and microbiome correlations with inflammatory and psychological parameters. At T1, CD patients exhibited a characteristic microbial profile mainly constituted of Proteobacteria (17.71%), Firmicutes (65.56%), Actinobacteria (8.46%) and Bacteroidetes (6.24%). Baseline bacterial abundances showed significant correlations with psychological markers of distress and with IFN. Following COBMINDEX, no significant changes in alpha and beta diversity were observed between both study groups, though a trend change in beta diversity was noted. Significant changes occurred in the abundance of phyla, families and genera only among the COBMINDEX group. Furthermore, abundance of phyla, families and genera that were altered following COBMNIDEX, significantly correlated with levels of cytokines and psychological parameters. Our results demonstrated that a short-term intervention of COBMINDEX was associated with changes in microbial indices, some of which are linked to psychological manifestations and systemic inflammation in CD patients. Psychological interventions to reduce chronic stress, such as COBMINDEX, appear to be beneficial in mitigating the pathobiology of CD patients, and may thus provide a useful adjunct to pharmacological therapy.
Introduction
Crohn’s disease (CD), a common form of inflammatory bowel disease (IBD), is a chronic disease characterized by progressive and destructive inflammation of the gastrointestinal tract, the distal small bowel and the large intestine.Citation1–4 While the etiology of CD is unknown, its causation is considered to be multifactorial, including immune system imbalance, an altered intestinal microbiome, a genetic predisposition and environmental factors.Citation4,Citation5
Previous studies have demonstrated the dysregulated immune system in CD, where disruption of the regulatory cytokine network in the bowel wall is associated with the development and progression of CD.Citation5–7 According to these studies, microbial dysbiosis together with a defective gut barrier induce a local immune response, resulting in a pro-inflammatory cytokine loop that overrides anti-inflammatory signals and causes chronic intestinal inflammation.Citation8 Inflammatory responses which evolve in CD include pro-inflammatory cytokine (e.g., IL-6, IL-18 and TNFα) releaseCitation9 and an enhanced T helper 17 (Th17) cell response.Citation10–12 Cytokines play a key role in the pathogenesis of CD, causing not only gut inflammation but also systemic inflammation which may lead to various extraintestinal manifestations including dysregulated immunity, increased risk for age-related diseases and psychiatric disorders.Citation13,Citation14 Regulating the abnormal cytokine pattern seen in CD is the basis for the various biological treatments now in use in CD patients.Citation15,Citation16
The gastrointestinal (GI) microbiome has been linked to many diseases, including IBD, where it is frequently proposed as one of the key components affected throughout the disease course.Citation17,Citation18 Disease-dependent biodiversity and imbalanced bacterial composition have been associated with CD, where certain commensal bacteria are depleted and the microbial community is less diverse.Citation19,Citation20 Decreased representation of several taxa of the Firmicutes phylum, and increased representation of the Gamma-proteobacteria including multiple genera considered potentially pathogenic (e.g., Escherichia, Salmonella, Yersinia, Helicobacter, Vibrio) were described.Citation20 Thus, characterizing the compositional and functional changes in the GI microbiota may lead to specific microbial manipulation as a therapeutic target for CD.Citation21
CD patients typically experience negative psychological symptoms,Citation22 which may be associated with the onset and progression of IBD.Citation22 Psychological distress can be harmful, due to its impact on inflammation via pathways involving the sympathetic nervous system and the hypothalamic-pituitary-adrenal (HPA) axis.Citation23,Citation24 The main product of the HPA pathway, cortisol, is secreted in a pulsatile pattern. The pattern of release and the levels of circulating cortisol markedly change in response to environmental and psychological stressorsCitation13,Citation25 and are linked with a variety of mental health disorders.Citation13,Citation26 The possibility that psychosocial interventions may boost immunity and improve immune-related health outcomes has been established in several studies demonstrating that immune system components are influenced by environmental, social, neurocognitive, and behavioral factors.Citation27–30 Various treatments are available in clinical practice for reducing negative psychological symptoms (distress, anxiety, and depression) in IBD patients.Citation22,Citation28,Citation29 We recently reported a randomized parallel-group physician-blinded trial of cognitive behavioral and mindfulness intervention with daily exercise (COBMINDEX) in adults with mild-to-moderate CD.Citation31 This study showed that patients taught COBMINDEX had reduced psychological symptoms compared with the control wait-list group,Citation31 and an attenuated disease-associated inflammatory process.Citation14
Following our recent report of the significant associations between inflammatory markers and psychological manifestations of CD patients,Citation14 in the present study we aimed to determine whether COBMINDEX impacts the gut microbial diversity in accordance with inflammation in patients with CD by examining: 1) the gut microbiota profile of CD patients at baseline and its associations with psychological symptoms and inflammatory markers of disease activity, 2) the impact of COBMINDEX on microbial diversity and whether it associates with changes in psychological symptoms and inflammatory markers.
Results
Enrolled Crohn’s disease patients exhibit a characteristic microbial profile which correlates with psychological symptoms and circulating inflammatory markers
To characterize the gut microbiota profile of CD patients in our cohort, we analyzed stool samples from 49 CD patients at baseline T1 (cohort described in Methods and ). As expected, we found no significant differences in phyla () and genera () abundances between the two groups: COBMINDEX (intervention) and wait-list (nonintervention) groups. Notably, the four major phyla, which constitute >98% of the gut microbiome,Citation32 are highlighted in our cohort: Firmicutes with a mean abundance of 65.56% (SD = 26.12%, 81% in literature),Citation17,Citation32 Proteobacteria with a mean abundance of 17.71% (SD = 23.55%; 5.13% in literature),Citation17,Citation32 Actinobacteria with a mean abundance of 8.46% (SD = 13.75%, 3.14% in literature)Citation17,Citation32 and Bacteroidetes with a mean abundance of 6.24% (SD = 9.05%, 9.25% in literature)Citation17,Citation32 (Table S1).
Figure 1. Phyla and genera comparison between wait-list and COBMINDEX at T1 demonstrates no significant differences.

Table 1. Demographic characteristics at baseline of Crohn’s disease patients undergoing laboratory studies.
We then sought to determine whether the distinct microbial abundances observed in CD patients are coupled with inflammatory and clinical markers of the disease and/or with psychological manifestations of the disease. As shown in (interactive network) and Table S2, we observed significant correlations between phyla, family and genera abundances and psychological parameters of CD, with no differences between COBMINDEX and wait-list groups (adjusted to age and sex). Notably, most of these significant correlations were between genera and families belonging to Firmicutes, known to be decreased in CD, with the main psychological markers of distress (SIBDQ, PSS4, GSI, see Methods section and Figure S1). Among these findings: Lachnospiraceae correlated negatively with PSS4 (r = −0.37, p = .01), Subdoligranulum correlated positively with SIBDQ (r = 0.35, p = .02) and negatively with PSS4 (r = −0.351, p = .01), and Enterococcus correlated positively with GSI (r = 0.38, p = .01). Furthermore, Deferribacteraceae correlated negatively with the pro-inflammatory cytokine INF- (r = −0.28, p = .04). These findings at T1 suggest baseline microbial-psychological-inflammatory relationships among CD patients.
Figure 2. Correlations at T1 demonstrate significant ties between microbial abundance and psychological parameters.

COBMINDEX treatment leads to changes in microbial abundance and diversity among Crohn’s disease patients
Taxonomic abundance
COBMINDEX was practiced by CD patients for a period of 3 months between T1 and T2; the microbial profile in stool samples was then compared between the two timepoints. Changes in taxonomic abundances among CD patients in both groups, COBMINDEX and wait-list, revealed significant changes in phyla, families and genera only among COBMINDEX (, Table S3). For example, an increase in the abundance of the phyla Deferribacteres (LDA = 3.51 p = .038) (), a decrease in the abundance of the families Streptococcaceae (LDA = 3.63 p = .038) and Coriobacteriaceae (LDA = 3.17 p = .043) (), a decreased abundance of genera Lachnospiraceae (LDA = 3.08 p = .019), and an increased abundance of Mucispirilum (LDA = 3.43 p = .038) () occurred only in the COBMINDEX group. These significant alterations, involving changes in inflammatory-related bacteria such as Streptococcaceae and Deferribacteres (correlated with INF at T1), hint at possible improvements in the gut microbiome following COBMINDEX treatment.
Figure 3. Significant changes in microbial abundance among COBMINDEX and wait-list CD patients.

Alpha and beta diversity
The changes observed between T1 and T2 timepoints following COBMINDEX prompt us to determine whether more global differences in microbiome diversity in the gut can be attributed to COBMINDEX. We found no significant differences in alpha diversity for wait-list and COBMINDEX groups between T1 and T2 time points (Shannon and Faith’s phylogenetic diversity) ( and Table S4). In addition, there were no significant differences in beta diversity between T1 and T2 in both wait-list and COBMINDEX groups (, Table S5). Of note, although there was no difference between the experimental groups at T1 (), a trend change was observed for the wait-list group at T2 (weighted unifrac index, p = .104, ), suggesting that further worsening occurred in their gut microbiome as compared with the COBMINDEX group.
Figure 4. Alpha diversity did not differ in COBMINDEX and wait-list groups over time.

Figure 5. Beta diversity in COBMINDEX and wait-list CD patients over time.

Microbial changes following COBMINDEX correlate with psychological and inflammatory markers of CD
Since COBMINDEX affected the taxonomic abundance of the gut microbiome, we pursued to analyze whether the microbial changes at T2 are accompanied by changes in inflammatory markers and/or psychological parameters of wellbeing (scores listed in Methods and illustrated in Fig. S1) which we recently observed.Citation31 (interactive networks) and Table S6A-B highlight the significant correlations between the abundance of microbial phyla, families and genera, with specific inflammatory and psychological parameters among both study groups. Interestingly, abundances of families and genus belonging to Deferribacteres and Firmicutes, which changed following COBMINDEX (), showed correlations with inflammatory markers and/or psychological parameters of distress (, Table S6A). For example, Mucispirillum correlated positively with GSI (r = 0.43, p = .04) and IL-6 (r = 0.44, p = .04), Streptococcaceae, a marker of CD relapse that decreased post COBMINDEX, correlated positively with CRP (r = 0.43, p = .04), and Lachnospiraceae ND3007 correlated positively with PSS4 (r = 0.49, p = .02). All these significant relationships allude to a possible role of Deferribacteres and Firmicutes in modulating the inflammatory-psychological manifestations of the disease. Notably, when zooming into significant correlations at T2 among wait-list (, Table S6B), Firmicutes correlated negatively with HBI (r=−.047, p = .01) and its genus Lachnospiraceae UCG-004, which showed a significantly decreased abundance among COBMINDEX, correlated negatively with GSI (r = −0.49, p = .01) and PSS4 (r = −0.50, p = .01). Together, these correlations demonstrate an opposite trend among wait-list compared to COBMINDEX and may imply that the effect it has on psychological parameters of distress is at least in part reflected in their gut microbiome.
Figure 6. Differential correlations between microbial, psychological, and inflammatory markers in COBMINDEX vs wait-list CD patients.

Discussion
The present study evaluated stool and serum samples of CD patients before and after COBMINDEX as compared with wait-list control CD patients. Our results demonstrate a characteristic microbial profile of CD patients consisting of four main phyla. Baseline bacterial abundances showed significant correlations with psychological markers of distress and with IFN. Following COBMINDEX, significant taxonomical alterations were revealed among the COBMINDEX group in the abundances of phyla, family, and genera. These abundance changes were accompanied by distinct correlations between psychological parameters and inflammatory markers in COBMINDEX and wait-list controls. Finally, we identified new microbial populations that, based on their associations with inflammatory and/or psychological markers following COBMINDEX, should be further investigated in the context of CD pathobiology.
Previous studies by our group revealed that COBMINDEX improves the quality of life (QoL) of CD patients and that the psychological improvement is accompanied by inflammatory and hormonal changes.Citation14,Citation31 These findings allowed us to determine whether better management of psychological distress, at least in part, recovers the microbial changes associated with CD severity. We demonstrated that COBMINDEX is indeed associated with changes in microbial abundance among a variety of phyla, families and genera. The decreased abundances of Coriobacteriaceae and Streptococcaceae appear to highlight the positive effect of COBMINDEX, as these bacteria are known to increase in CD.Citation33,Citation34 Also, a lineage of Lachnospiraceae, known to be associated with severe CD,Citation35,Citation36 was decreased in COBMINDEX. While the microbial composition did not change among the groups over time, our results did show a trend change in the beta-diversity index among wait-list, but not among COBMINDEX. The very mild differences in the overall microbial composition observed in our cohort, could be best explained by the human microbiome resilience,Citation37 the individual’s heterogeneity,Citation38 and/or the relatively small sample size, which together with a short treatment time of only 3-months, may mask a more abundant collective group difference. Given these limitations, our findings suggest that COBMINDEX partially recovers the bacterial composition in patients with CD, acting as a key component which can modify the disease process.
Although microbiome alterations in CD may contribute to stress manifestations of the disease, association of psychological markers with microbial signatures was incompletely revealed. We show that both at T1 and T2 time points, families and genera belonging to Firmicutes correlated with psychological markers of distress. Subdoligranulum, found by Mondot S et al., to be imbalanced among CD patients,Citation39 had a positive correlation with the QoL measure SIBDQ and a negative correlation with the psychological stress measure PSS4—both measures were significantly improved post COBMINDEX.Citation31 In addition, Lachnospiraceae, the most abundant Firmicutes family which is known to be increased in patients with severe CD,Citation32,Citation35 correlated positively with PSS4. Notably, Lachnospiraceae was found overabundant in CD patients and suggested to contribute to psychological comorbidities via dysregulated bile acids.Citation36,Citation40 According to Min Yang.et al., gut dysbiosis leads to disturbed bile acid transformation, impairing the gut barrier and immunity in IBD patients.Citation41 Recent research explores the potential role of bile acids as a bridge between the gut microbiome and the brain, suggesting a pathway that could influence the patient’s mental well-being.Citation42,Citation43 The decreased abundance of bacteria belonging to Lachnospiraceae family in the COBMINDEX group at T2 and their positive correlation with PSS4 and GSI scores highlight Lachnospiraceae as an important therapeutic target that should be further explored particularly in the context of impaired bile acid metabolism and psychological distress which contribute to CD pathology.
Our previous research uncovered significant associations between psychological markers of distress with inflammatory and clinical markers of CD.Citation14 Accordingly, we sought to find whether microbial–inflammatory relationships explain some of these associations. At T1, Deferribacteres, known to have only a small number of genus associated with IBD pathogenesis, showed inverse correlation with IFN, and positive correlation with IL-6, two cytokines which can impact the inflammatory and psychological manifestation of CD.Citation44,Citation45 Moreover, Streptococcaceae, recently found as a microbial marker of CD relapse,Citation34 and was in decreased abundance post COBMINDEX, correlated at T2 with CRP – a clinical hallmark of disease progression.Citation46 These findings suggest an interesting tie between CD-related bacteria, pro-inflammatory cytokines, and psychological manifestations among CD patients, which should be further elucidated toward identification of disease biomarkers and mechanisms.
We acknowledge several limitations in our study. Firstly, the limitations of cohort size: a larger cohort examined over a longer period with multiple samples of stool, serum, saliva, and/or hair, may better demonstrate the changes in the microbial diversity and abundance, and their associations with inflammatory responses following COBMINDEX in CD patients. Secondly, the use of LEfSe analysis restricted our ability to test interactions between timepoints and groups directly. Moreover, diet is recognized as a risk factor for the development of CD and attributes a pathogenic role to the intestinal dysbiosis inducing an aberrant mucosal immune response.Citation47 Therefore, although in our study we did not specify dietary requirements, future research should incorporate dietary modifications to test whether they will enhance the positive effect COBMINDEX had on microbiome.Citation48,Citation49 Lastly, future studies may utilize whole-genome metagenomics to explore strain-level characteristics of the gut microbiome in this context.
Conclusions
Baseline bacterial abundances of CD patients showed significant correlations with psychological markers of distress and with IFN. The significant impact of COBMINDEX on psychological symptoms also accompanied alterations in abundances of gut microbiome and systemic inflammation. COBMINDEX may thus be a potential adjunct to the standard of care, that can significantly impact the pathobiology of CD and may underpin a more personalized treatment approach for this patient group.
Methods
Cohort
CD patients were recruited from July 2018─July 2020 by advertising at participating hospitals (Soroka Medical Center at Beer Sheva, and Rabin Medical Center at Petah Tikva, Israel) and social media; the study was completed in November 2020. There were no study-related untoward effects. Patients aged 18–75 years with Harvey-Bradshaw Index (HBI) of disease activity in the range 5–16 were eligible. Clinical social workers performed initial screening, and gastroenterologists enrolled patients according to inclusion/exclusion criteria.Citation31 Exclusion criteria included: age below 18 or older than 75 years of age, no diagnosis of CD, <1 year of follow-up since diagnosis, change of diagnosis in study period, new medication started in past 3 months, surgery in past 6 months, planned elective surgery, acute surgery during study, pregnancy, planned pregnancy in study period, present/past psychiatric disease/medication, irritable bowel syndrome, not fluent in Hebrew. Irritable bowel syndrome was excluded on clinical grounds (according to Rome 4 criteria).Citation50 Patients were then randomized to COBMINDEX or wait-list controls; study physicians and laboratory workers were blinded to patient allocation. Randomization was carried out using the cluster random sampling method,Citation31 with proportionate allocation strategy where the fractions were defined by their sex, to COBMINDEX (taught by clinical social workers per standardized protocol on Skype™ over 3 months) or wait-list (control group), as detailed in our recent publication.Citation51 The study was carried out between the time points designated as baseline (T1), and study completion after 3 months (T2). Physicians remained blinded to randomization throughout the study. The nature of the study precluded patient participation in protocol-design. All patients remained on medical follow-up, and participation in the study did not affect their treatment. Patients were instructed not to alter their diets or activities in the study period due to its research proven effects on microbiome.Citation49 The percentage of smokers in COBMINDEX and wait-list groups was similar. A detailed description of the COBMINDEX protocol can be found in our previously published articles.Citation14,Citation31,Citation51
The cohort of patients in the clinical trial was as follows: COBMINDEX 55, wait-list 61; from these patients, we selected a sub-cohort of patients based on who was able to provide both stool and blood samples at time points T1 and T2 for laboratory studies. The sub-cohort included 49 individuals, in two groups: 1) COBMINDEX (n = 24, mean age 33.9 years, 72% females), and 2) wait-list (n = 25, mean age 32.6 years, 56% females) (). COBMINDEX and wait-list patients in the laboratory sub-cohort were similar in disease activity and treatments at recruitment (). Among the COBMINDEX group, one patient took an antibiotic (Ciprofloxacin – 500 mg) for the duration of 14 days during the enlistment process to the study, 21 days prior to beginning the COBMINDEX therapy, therefore we did not consider it as an exclusion criterion. As we previously published COBMINDEX had a significant effect on the wellbeing of CD patients.Citation31 The response to COBMINDEX was sorted according to a “Responsiveness Score” detailed in our latest study presenting the effects of COBMINDEX on brain-immune axis regulation.Citation14 The patients in the current study, a subgroup of the cohort, are representatives of the responders in the cohort with an average responsiveness score among the COMBINDEX group of 1.26 (std 0.44) compared with −0.07 (std 0.44) among wait-list. Biological samples were provided at T1 and T2 time points. All the samples and data analyzed in this study were collected prior to the onset of the COVID-19 pandemic.
Trial registration
Ministry of Health, Israel.
https://my.health.gov.il/CliniTrials/Pages/MOH_2020–02-24_008721.aspx
ClinicalTrials.gov Identifier: NCT05085925.
Medical and psychological data
At baseline (T1) and after 3 months (T2) we assessed the patients using the following measuresCitation31 shown in Figure S1:
Harvey-Bradshaw Index (HBI)
This questionnaire evaluates disease activity in five questions pertaining to the past day’s symptoms of well-being. Responses are summed to provide HBI. A HBI ≤ 4 indicates disease remission, 5–7 mild disease, 8–16 moderate disease, >16 severe disease.
Short Inflammatory Bowel Disease Questionnaire (SIBDQ)
This disease-specific quality of life (QoL) questionnaire relates to the past 2 weeks’ symptoms, general feeling and mood in ten items graded on a 7-point Likert scale (1 = all the time, 7 = never). A higher score indicates better QoL.
MOS 12-Item Short Form Survey Instrument (SF-12)
This generic QoL measure has 12 items assessing physical functioning and general health. Scores are summed to yield physical health (SF-12PH) and mental health (SF-12MH) composites, with range 0–100. A higher score indicates better QoL.
Brief Symptom Inventory (BSI)
This instrumentCitation52 measures psychological symptoms in the past month. Its 53 questions assess nine dimensions (depression, somatization, obsession-compulsive, interpersonal sensitivity, anxiety, hostility, phobic anxiety, paranoid ideation, psychoticism) on a 0–4 Likert scale (0 = not affected, 4 = extremely affected). A higher score implies more severe symptoms. The BSI yields a score for each dimension and a summary score called the Global Severity Index (GSI), all with range 0–4.
Perceived Stress Scale (PSS-4)
Self-reported questionnaire that measures a person’s evaluation of stressful situations in the previous 1 month of his or her life. The instrument contains 14 statements which measure how unpredictable and overloaded respondents feel their lives are. Respondents rate how often they experience stressful situations on a 5-point Likert scale ranging from ‘never’ (0) to ‘very often’ (4). Higher scores are correlated to more stress.
Biological data collection
Biological samples (peripheral blood) for measuring inflammation and hormones were drawn from patients at baseline (T1) and after 3 months (T2). Blood samples were taken during morning hours (08:00–13:00) and serum was isolated from Vacuette tube 8 ml with gel (Greiner, Kremsmünster, Austria) and stored in aliquots at −80°C until analysis. The profiling of cytokines/chemokines in serum samples was performed with the CytoFLEX instrument (Beckman Coulter, Brea, CA, USA) using the LEGENDplex™ (BioLegend, San Diego, CA, USA) Human Inflammation Panel 1 (with assay coefficients of variation) [13-plex: IL-1β (13.2%), IFNα2 (23.9%), IFNγ (12.6%), TNF-α (11.1%), MCP-1/CCL2 (8.3%), IL-6 (20.5%), IL-8 (CXCL8–11%), IL-10 (9.1%), IL-12p70 (11.5%), IL-17A (21.4%), IL-18 (7.6%), IL-23 (9.3%), and IL-33 (19.4%)] according to the manufacturer’s instructions. Data were analyzed with the LEGENDplex™ Data Analysis Software Version 8.0. The validity and reliability of the high-sensitivity multiplex assays have been tested in previous studies, overall demonstrating that circulating serum and plasma concentrations of some cytokines correlated accurately with ELISA results.Citation53,Citation54 The multiplex assays were found reliable, particularly in the context of smaller studies performed in a small scale and/or when all samples have been collected and can be run at a single time and place.Citation54
Stool sample collection and analysis
DNA from swabs was extracted with the DNeasy PowerSoil kit without modifications (Qiagen, Hilden, Germany). The V4 region of 16S rRNA was amplified using 515F and 806 R primers and processed on a MiSeq instrument per Illumina’s 16S protocol (using a Miseq V2–500 cycle kit to generate 2 × 250 paired-end reads) (Illumina, San Diego, CA, USA).Citation55
Bioinformatics analyses
Raw Sequence Preparation: Raw sequence data were imported to the QIIME2 package (v2019.10).Citation46 Raw sequence reads underwent primer removal (16S primers from EMP [earth microbiome project], specifically 515F and 807 R for the V4) and DADA2 QC filtering and chimera sequence removal (plugin: dada2 denoise-paired),Citation56 with final read counts for 104 analyzed samples ranging from 8467 to 39,647 (average of 14,951).
Sequence data Analysis: Representative sequences (output from the DADA2 pipeline) were aligned using the phylogeny plugin (phylogeny align-to-tree-mafft-fasttree)Citation57,Citation58 producing a rooted tree, and assigned taxonomic features using the feature-classifier plugin (feature-classifier classify-sklearn)Citation59 with the SILVA DBCitation60 (v132 at 99%), trained on the 515F and 807 R (V4) subregion.Citation61
The amplicon sequence variants (ASVs) feature table, rooted tree, taxonomic assignments and metadata were imported into R (v4)Citation62 using the package file2meco (v0.5.0)Citation63 for further analyses with the R package microeco (v0.14.1).Citation64 The samples were then rarefied at a sampling depth of 8000 for downstream analyses.
Microbiome and statistical analyses
For T1 samples (n = 49), a comparison of relative abundance of phyla, families and genera between COBMINDEX and wait-list samples was performed using the LefSe methodCitation65 (with FDR adjustment), with p-values < .05 considered significant. Beta diversity (using the indices Bray-Curtis, weighted and unweighted unifrac) was measured between COBMINDEX and wait-list samples, with non-metric multidimensional scaling (NDMS) ordination plots and group significance determined by PERMANOVA test (with 999 permutations and FDR adjustment), with p-values <.05 considered significant. Differential taxa abundance (using the LefSe method at the family taxonomic level) was also measured between COBMINDEX and wait-list samples. Finally, for T1 (n = 49) and T2 (n = 48) samples (separately), a correlation analysis (Spearman’s correlation) between microbial abundances from the ASVs feature table at the phylum, family and genus taxonomic levels, with inflammatory and psychological markers, was conducted using the cal_cor function from the R package microeco with p-value <.05 considered significant.Citation66
For the COBMINDEX (n = 23 patients, 46 samples) and wait-list (n = 23 patients, 46 samples) groups, samples were analyzed over time (T1 vs. T2) for changes in relative abundance at the phylum, family and genus taxonomic levels using the LefSe method,Citation67 where variations were considered significant when the linear discriminant analysis (LDA) score was greater than 3 and/or the p-value from the LefSe test was <0.05. Due to outliers, 1 COBMINDEX and 2 wait-list patients were taken out. Changes over time in alpha diversity (using the indices Shannon, Simpson and phylogenetic diversity [PD]) were tested using the paired T-test (with FDR adjustment), with p-values < .05 considered significant. Changes over time in beta diversity (using the indices Bray-Curtis, weighted and unweighted unifrac) were analyzed using non-metric multidimensional scaling (NDMS) ordination plots and group significance was determined by PERMANOVA test (with 999 permutations and FDR adjustment), with p-values <.05 considered significant.
Co-occurrence networks were generated based on correlation scores. Network visualization and the positioning of the nodes were calculated according to the force directed Fruchterman & Reingold algorithm used for calculating layouts of simple undirected graphs.Citation68 The algorithm was implemented using nx.draw function via the ‘pos’ parameter in the ‘NetworkX’ python package v1.11. Node degree was calculated using the nx.degree function. Visualization was generated using ‘Plotly’ python package v4.9.0.
Ethical Considerations
The institutional review boards of Soroka Medical Center (Beer Sheva, Israel) and Rabin Medical Center (Petah Tikva, Israel) approved the trial. Participants were given a detailed written and oral description of the research project and provided informed consent.
Supplemental Material
Download JPEG Image (179.1 KB)supp tables 140324 clean.docx
Download MS Word (33.5 KB)Acknowledgments
The study was supported by The Leona M. and Harry B. Helmsley Charitable Trust and the Ministry of Science and Technology of Israel (MOST, 3-14356; to A.M.). We acknowledge Ekaterina Vinogradov who participated in serum samples preparation and Rotem Bartov who helped with analysis and graphic illustrations. We acknowledge the non-IIRN gastroenterologists: Eyal Hirsch, MD, Timna Naftali, MD, Anat Nevo, MD, Naim Abu-Freha, MD, Arik Segal, MD, David Yardeni, MD for their assistance in patient recruitment.
Disclosure statement
No potential conflict of interest was reported by the author(s).
Data availability statement
The data supporting this study’s findings of this study are available on request from the corresponding author [A.M]. The data are not publicly available due to their containing information that could compromise the privacy of research participants.
Supplementary material
Supplemental data for this article can be accessed online at https://doi.org/10.1080/19490976.2024.2337269
Correction Statement
This article has been corrected with minor changes. These changes do not impact the academic content of the article.
Additional information
Funding
References
- Roda G, Chien Ng S, Kotze PG, Argollo M, Panaccione R, Spinelli A, Kaser A, Peyrin-Biroulet L, Danese S. Crohn’s disease. Nat Rev Dis Primers. 2020 Apr 04;6(1):22. doi:10.1038/s41572-020-0156-2.
- Fatahi DA, Al Asmari AS, Bukhari GA, Alshamrani HA, Hunaydi KA, Sharahili AM, Alharshan TK, Alherz AA. Crohn’s disease: Pathophysiology and management. Egypt J Hosp Med. 2018;70(11):2004–15. doi:10.12816/0044858.
- Gajendran M, Loganathan P, Catinella AP, Hashash JG. A comprehensive review and update on Crohn’s disease. Dis Mon. 2018;64(2):20–57. doi:10.1016/j.disamonth.2017.07.001.
- Petagna L, Antonelli A, Ganini C, Bellato V, Campanelli M, Divizia A, Efrati C, Franceschilli M, Guida AM, Ingallinella S. et al. Pathophysiology of Crohn’s disease inflammation and recurrence. Biol Direct. 2020;15(1):1–10. doi:10.1186/s13062-020-00280-5.
- Leppkes M, Neurath M. Cytokines in inflammatory bowel diseases–Update 2020. Pharmacol Res. 2020;158:104835. doi:10.1016/j.phrs.2020.104835.
- Bevivino G, Monteleone G. Advances in understanding the role of cytokines in inflammatory bowel disease. Expert Rev Gastroenterol Hepatol. 2018;12(9):907–915. doi:10.1080/17474124.2018.1503053.
- Neurath MF. Cytokines in inflammatory bowel disease. Nat Rev Immunol. 2014;14(5):329–342. doi:10.1038/nri3661.
- Neurath MF. Targeting immune cell circuits and trafficking in inflammatory bowel disease. Nat Immunol. 2019;20(8):970–979. doi:10.1038/s41590-019-0415-0.
- Huang Y, Chen Z. Inflammatory bowel disease related innate immunity and adaptive immunity. Am J Transl Res. 2016 Jul 12;8(6):2490–2497.
- Velikova TV, Miteva L, Stanilov N, Spassova Z, Stanilova SA. Interleukin-6 compared to the other Th17/Treg related cytokines in inflammatory bowel disease and colorectal cancer. World J Gastroenterol. 2020;26(16):1912. doi:10.3748/wjg.v26.i16.1912.
- Kuwabara T, Ishikawa F, Kondo M, Kakiuchi T. The role of IL-17 and related cytokines in inflammatory autoimmune diseases. Mediators Inflamm. 2017;2017:1–11. doi:10.1155/2017/3908061.
- Hueber W. A human anti-IL-17A monoclonal antibody, for moderate to severe Crohn’s disease: unexpected results of a randomised, double-blind placebo-controlled trial. Gut. 2012;61(12):1693–1700. doi:10.1136/gutjnl-2011-301668.
- Makris AP, Karianaki M, Tsamis KI, Paschou SA. The role of the gut-brain axis in depression: Endocrine, neural, and immune pathways. Hormones. 2021;20(1):1–12. doi:10.1007/s42000-020-00236-4.
- Nemirovsky A, Ilan K, Lerner L, Cohen-Lavi L, Schwartz D, Goren G, Sergienko R, Greenberg D, Slonim-Nevo V, Sarid O. et al. Brain-immune axis regulation is responsive to cognitive behavioral therapy and mindfulness intervention: Observations from a randomized controlled trial in patients with Crohn’s disease. Brain Behav Immun-Health. 2021;19:100407. doi:10.1016/j.bbih.2021.100407.
- Schoultz IK, Keita ÅV. Cellular and molecular therapeutic targets in inflammatory bowel disease—focusing on intestinal barrier function. Cells. 2019;8(2):193. doi:10.3390/cells8020193.
- Ballegeer M, Van Looveren K, Timmermans S, Eggermont M, Vandevyver S, Thery F, Dendoncker K, Souffriau J, Vandewalle J, Van Wyngene L. et al. Glucocorticoid receptor dimers control intestinal STAT1 and TNF-induced inflammation in mice. J Clin Invest. 2018 May 11;128(8):3265–3279. doi:10.1172/jci96636.
- Aldars-García L, Chaparro M, Gisbert JP. Systematic review: The gut microbiome and its potential clinical application in inflammatory bowel disease. Microorganisms. 2021 May 06;9(5):977. doi:10.3390/microorganisms9050977.
- Øyri SF, Műzes G, Sipos F. Dysbiotic gut microbiome: A key element of Crohn’s disease. Comp Immunol Microbiol Infect Dis. 2015;43:36–49. doi:10.1016/j.cimid.2015.10.005.
- Schreiner P, Rossel JB, Biedermann L, Valko PO, Baumann CR, Greuter T, Scharl M, Vavricka SR, Pittet V, Juillerat P. et al. Fatigue in inflammatory bowel disease and its impact on daily activities. Aliment Pharmacol Ther. 2021;53(1):138–149. doi:10.1111/apt.16145.
- Wright EK, Kamm MA, Teo SM, Inouye M, Wagner J, Kirkwood CD. Recent advances in characterizing the gastrointestinal microbiome in Crohnʼs disease. Inflamm Bowel Dis. 2015;21:1219–1228. doi:10.1097/MIB.0000000000000382.
- Gevers D, Kugathasan S, Knights D, Kostic AD, Knight R, Xavier RJ. A microbiome foundation for the study of Crohn’s disease. Cell Host & Microbe. 2017;21(3):301–304. doi:10.1016/j.chom.2017.02.012.
- Wynne B, McHugh L, Gao W, Keegan D, Byrne K, Rowan C, Hartery K, Kirschbaum C, Doherty G, Cullen G. et al. Acceptance and commitment therapy reduces psychological stress in patients with inflammatory bowel diseases. Gastroenterology. 2019;156(4):935–945.e1. doi:10.1053/j.gastro.2018.11.030.
- F.S. D. Enhancing versus suppressive effects of stress on immune function: Implications for immunoprotection and immunopathology. Neuroimmunomodulation. 2009;16(5):300–317. doi:10.1159/000216188.
- Reed RG, Raison CL. Stress and the immune system. Environ Influences Immune Syst. 2016;97–126. Springer.
- Spencer RLD, Deak T. A users guide to HPA axis research. Physiol Behav. 2017;178:43–65. doi:10.1016/j.physbeh.2016.11.014.
- Sylvia KE, Demas GE. A gut feeling: microbiome-brain-immune interactions modulate social and affective behaviors. Horm Behav. 2018;99:41–49. doi:10.1016/j.yhbeh.2018.02.001.
- Shields GS, Spahr CM, Slavich GM. Psychosocial interventions and immune system function: a systematic review and meta-analysis of randomized clinical trials. JAMA Psychiarty. 2020;77(10):1031. doi:10.1001/jamapsychiatry.2020.0431.
- Gracie DJ, Irvine AJ, Sood R, Mikocka-Walus A, Hamlin PJ, Ford AC. Effect of psychological therapy on disease activity, psychological comorbidity, and quality of life in inflammatory bowel disease: a systematic review and meta-analysis. Lancet Gastroenterol Hepatol. 2017;2:189–199. doi:10.1016/S2468-1253(16)30206-0.
- Mikocka-Walus A, Bampton P, Hetzel D, Hughes P, Esterman A, Andrews JM. Cognitive-behavioural therapy for inflammatory bowel disease: 24-month data from a randomised controlled trial. Int J Behav Med. 2017;24(1):127–135. doi:10.1007/s12529-016-9580-9.
- Mikocka-Walus A, Bampton P, Hetzel D, Hughes P, Esterman A, Andrews JM. Cognitive-behavioural therapy has no effect on disease activity but improves quality of life in subgroups of patients with inflammatory bowel disease: a pilot randomised controlled trial. BMC Gastroenterol. 2015;15(1):54. doi:10.1186/s12876-015-0278-2.
- Goren G, Schwartz D, Friger M, Banai H, Sergienko R, Regev S, Abu-Kaf H, Greenberg D, Nemirovsky A, Ilan K. et al. Randomized controlled trial of cognitive-behavioral and mindfulness-based stress reduction on the quality of life of patients with Crohn disease. Inflamm Bowel Dis. 2021 Apr 14;2021. doi:10.1093/ibd/izab083
- Alam MT, Amos GCA, Murphy ARJ, Murch S, Wellington EMH, Arasaradnam RP. Microbial imbalance in inflammatory bowel disease patients at different taxonomic levels. Gut Pathog. 2020;12(1):1. doi:10.1186/s13099-019-0341-6.
- Zhang L, Liu F, Xue J, Lee SA, Liu L, Riordan SM. Bacterial species associated with human inflammatory bowel disease and their pathogenic mechanisms. Front Microbiol. 2022;13:801892. doi:10.3389/fmicb.2022.801892.
- Buffet-Bataillon S, Bouguen G, Fleury F, Cattoir V, Le Cunff Y. Gut microbiota analysis for prediction of clinical relapse in Crohn’s disease. Sci Rep. 2022;12(1):19929. doi:10.1038/s41598-022-23757-x.
- Horwat P, Kopeć S, Garczyk A, Kaliciak I, Staręga Z, Drogowski K, Mardas M, Stelmach-Mardas M. Influence of enteral nutrition on gut microbiota composition in patients with Crohn’s disease: a systematic review. Nutrients. 2020;12(9):2551. doi:10.3390/nu12092551.
- Feng L, Zhou N, Li Z, Fu D, Guo Y, Gao X, Liu X. Co-occurrence of gut microbiota dysbiosis and bile acid metabolism alteration is associated with psychological disorders in Crohn’s disease. Faseb J. 2022;36(1):e22100. doi:10.1096/fj.202101088RRR.
- Relman DA. The human microbiome: ecosystem resilience and health. Nutr Rev. 2012;70:S2–S9. doi:10.1111/j.1753-4887.2012.00489.x.
- Khan I, Ullah N, Zha L, Bai Y, Khan A, Zhao T, Che T, Zhang C. Alteration of gut microbiota in inflammatory bowel disease (IBD): Cause or Consequence? IBD treatment targeting the gut microbiome. Pathogens. 2019;8(3):126. doi:10.3390/pathogens8030126.
- Mondot S, Kang S, Furet JP, Aguirre de Carcer D, McSweeney C, Morrison M, Marteau P, Doré J, Leclerc M. Highlighting new phylogenetic specificities of Crohnʼs disease microbiota. Inflamm Bowel Dis. 2010;17(1):185–192. doi:10.1002/ibd.21436.
- Humbel F, Rieder JH, Franc Y, Juillerat P, Scharl M, Misselwitz B, Schreiner P, Begré S, Rogler G, von Känel R. et al. Association of alterations in intestinal microbiota with impaired psychological function in patients with inflammatory bowel diseases in remission. Clin Gastroenterol Hepatol. 2020;18(9):2019–2029.e11. doi:10.1016/j.cgh.2019.09.022.
- Yang M, Gu Y, Li L, Liu T, Song X, Sun Y, Cao X, Wang B, Jiang K, Cao H. et al. Bile acid–gut microbiota axis in inflammatory bowel disease: from bench to bedside. Nutrients. 2021;13(9):3143. doi:10.3390/nu13093143.
- Monteiro-Cardoso VF, Corlianò M, Singaraja RR. Bile acids: a communication channel in the gut-brain axis. Neuromolecular Med. 2021;23(1):99–117. doi:10.1007/s12017-020-08625-z.
- Ni Dhonnabhain R, Xiao Q, O’Malley D. Aberrant gut-to-brain signaling in irritable bowel syndrome - the role of bile acids. Front Endocrinol. 2021;12:745190. doi:10.3389/fendo.2021.745190.
- Tang Y, Zhao L, Lei N, Chen P, Zhang Y. Crohn’s disease patients with depression exhibit alterations in monocyte/macrophage phenotype and increased proinflammatory cytokine production. Dig Dis. 2020;38(3):211–221. doi:10.1159/000501122.
- Eriguchi Y, Nakamura K, Yokoi Y, Sugimoto R, Takahashi S, Hashimoto D, Teshima T, Ayabe T, Selsted ME, Ouellette AJ. et al. Essential role of IFNγ in T cell–associated intestinal inflammation. JCI Insight. 2018;3(18):e121886. doi:10.1172/jci.insight.121886.
- Bolyen E, Rideout JR, Dillon MR, Bokulich NA, Abnet CC, Al-Ghalith GA, Alexander H, Alm EJ, Arumugam M, Asnicar F. et al. Reproducible, interactive, scalable and extensible microbiome data science using QIIME 2. Nat Biotechnol. 2019;37(8):852–857. doi:10.1038/s41587-019-0209-9.
- Mentella MC, Scaldaferri F, Pizzoferrato M, Gasbarrini A, Miggiano GAD. Nutrition, IBD and gut microbiota: A review. Nutrients. 2020 Apr 03;12(4):944. doi:10.3390/nu12040944.
- Yanai H, Levine A, Hirsch A. et al. The Crohn’s disease exclusion diet for induction and maintenance of remission in adults with mild-to-moderate Crohn’s disease (CDED-AD): an open-label, pilot, randomised trial. Lancet Gastroenterol Hepatol. 2022;7:49–59. doi:10.1016/S2468-1253(21)00299-5.
- Godny L, Reshef L, Sharar Fischler T, Elial-Fatal S, Pfeffer-Gik T, Raykhel B, Rabinowitz K, Levi-Barda A, Perets TT, Barkan R. et al. Increasing adherence to the Mediterranean diet and lifestyle is associated with reduced fecal calprotectin and intra-individual changes in microbial composition of healthy subjects. Gut Microbes. 2022;14(1):2120749. doi:10.1080/19490976.2022.2120749.
- Oka P, Parr H, Barberio B. et al. Global prevalence of irritable bowel syndrome according to Rome III or IV criteria: a systematic review and meta-analysis. Lancet Gastroenterol Hepatol. 2020;5:908–917. doi:10.1016/S2468-1253(20)30217-X.
- Regev S, Schwartz D, Sarid O, Goren G, Slonim‐Nevo V, Friger M, Sergienko R, Greenberg D, Monsonego A, Nemirovsky A. et al. Randomised clinical trial: Psychological intervention improves work productivity and daily activity by reducing abdominal pain and fatigue in Crohn’s disease. Aliment Pharmacol Ther. 2023;57(8):861–871. doi:10.1111/apt.17399.
- Al’absi M. Stress and addiction: When a robust stress response indicates resiliency. Psychosom Med. 2018;80(1):2. doi:10.1097/PSY.0000000000000520.
- Richens JL, Urbanowicz RA, Metcalf R, Corne J, O’Shea P, Fairclough L. Quantitative validation and comparison of multiplex cytokine kits. J Biomol Screen. 2010;15(5):562–568. doi:10.1177/1087057110362099.
- Breen EC, Reynolds SM, Cox C, Jacobson LP, Magpantay L, Mulder CB, Dibben O, Margolick JB, Bream JH, Sambrano E. et al. Multisite comparison of high-sensitivity multiplex cytokine assays. Clin Vaccine Immunol. 2011;18(8):1229–1242. doi:10.1128/CVI.05032-11.
- Caporaso JG, Lauber CL, Walters WA, Berg-Lyons D, Huntley J, Fierer N, Owens SM, Betley J, Fraser L, Bauer M. et al. Ultra-high-throughput microbial community analysis on the Illumina HiSeq and MiSeq platforms. Isme J. 2012;6(8):1621–1624. doi:10.1038/ismej.2012.8.
- Callahan BJ, McMurdie PJ, Rosen MJ, Han AW, Johnson AJA, Holmes SP. DADA2: High-resolution sample inference from Illumina amplicon data. Nat Methods. 2016;13(7):581–583. doi:10.1038/nmeth.3869.
- Price MN, Dehal PS, Arkin AP, Poon AFY. FastTree 2 – Approximately maximum-likelihood trees for large alignments. PloS One. 2010;5(3):e9490. doi:10.1371/journal.pone.0009490.
- Katoh K, Standley DM. MAFFT Multiple sequence alignment software version 7: improvements in performance and usability. Mol Biol Evol. 2013;30(4):772–780. doi:10.1093/molbev/mst010.
- Pedregosa F, Varoquaux G, Gramfort A, Michel V, Thirion B, Grisel O, Blondel M, Prettenhofer P, Weiss R, Dubourg V. et al. Scikit-learn: Machine learning in Python. J Mach Learn Res. 2011;12:2825–2830.
- Quast C, Pruesse E, Yilmaz P, Gerken J, Schweer T, Yarza P, Peplies J, Glöckner FO. The SILVA ribosomal RNA gene database project: improved data processing and web-based tools. Nucleic Acids Res. 2012;41(D1):D590–D596. doi:10.1093/nar/gks1219.
- Bokulich NA, Kaehler BD, Rideout JR. et al. Optimizing taxonomic classification of marker-gene amplicon sequences with QIIME 2’s q2-feature-classifier plugin. Microbiome. 2018;6:90. doi:10.1186/s40168-018-0470-z.
- https://www.R-project.org/.
- Liu C, Li X, Mansoldo FRP, An J, Kou Y, Zhang X, Wang J, Zeng J, Vermelho AB, Yao M. et al. Microbial habitat specificity largely affects microbial co-occurrence patterns and functional profiles in wetland soils. Geoderma. 2022;418:115866. doi:10.1016/j.geoderma.2022.115866.
- Liu C, Cui Y, Li X, Yao M. Microeco: an R package for data mining in microbial community ecology. FEMS Microbiol Ecol. 2020;97(2). doi:10.1093/femsec/fiaa255.
- Segata N, Izard J, Waldron L, Gevers D, Miropolsky L, Garrett WS, Huttenhower C. Metagenomic biomarker discovery and explanation. Genome Biol. 2011;12(6):R60. doi:10.1186/gb-2011-12-6-r60.
- Lahti L, Salonen A, Kekkonen RA, Salojärvi J, Jalanka-Tuovinen J, Palva A, Orešič M, de Vos W. Associations between the human intestinal microbiota, Lactobacillus rhamnosus GG and serum lipids indicated by integrated analysis of high-throughput profiling data. PeerJ. 2013;1:e32. doi:10.7717/peerj.32.
- Villanueva RAM, Chen ZJ. ggplot2: Elegant graphics for data analysis (2nd ed.). Meas: Interdiscip Res Perspect. 2019;17(3):160–167. doi:10.1080/15366367.2019.1565254.
- Pavlopoulos GA, Secrier M, Moschopoulos CN, Soldatos TG, Kossida S, Aerts J, Schneider R, Bagos PG. Using graph theory to analyze biological networks. BioData Min. 2011;4(1):1–27. doi:10.1186/1756-0381-4-10.