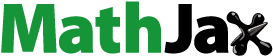
ABSTRACT
Background: Simulation modeling facilitates the estimation of long-term health and economic outcomes to inform healthcare decision-making.
Objective: To develop a framework to simulate progression of Parkinson’s disease (PD), capturing motor and non-motor symptoms, clinical outcomes, and associated costs over a lifetime.
Methods: A patient-level simulation was implemented accounting for individual variability and interrelated changes in common disease progression scales. Predictive equations were developed to model progression for newly diagnosed patients and were combined with additional sources to inform long-term progression. Analyses compared a hypothetical disease-modifying therapy (DMT) with a standard of care to explore the drivers of cost-effectiveness.
Results: The equations captured the dependence between the various measures, leveraging prior values and rates of change to obtain realistic predictions. The simulation was built upon several interrelated equations, validated by comparison with observed values for the Movement Disorder Society Unified PD Rating Scale (MDS-UPDRS) and UPDRS subscales over time. In a case study, disease progression rates, patient utilities, and direct non-medical costs were drivers of cost-effectiveness.
Conclusions: The developed equations supported the simulation of early PD. This model can support conducting simulations to inform internal decision-making, trial design, and strategic planning early in the development of new DMTs entering clinical trials.
Introduction
Parkinson’s disease (PD) is a progressive neurological disorder characterized by numerous motor (e.g., bradykinesia, rigidity, rest tremor, and postural instability) and non-motor symptoms (e.g., depression, cognitive impairment, and autonomic disturbances [Citation1]). There is currently no disease-modifying therapy (DMT) available for the treatment of patients with PD, but new therapies are being studied and entering clinical trials [Citation2]. It will be important to be able to model the long-term potential health and economic benefits from diagnosis to the more advanced stages of disease. Disease simulation models facilitate estimating the potential impact of treatment on long-term clinical and economic outcomes to inform healthcare decision-making.
Three scales have been widely used to measure the progression of PD: the Hoehn and Yahr (HY) scale and two versions of the Unified Parkinson’s Disease Rating Scale (UPDRS). The HY scale measures motor symptoms with scores ranging from 1 to 5 [Citation3]; two additional stages (1.5 and 2.5) were added in a modified version [Citation4]. As the understanding of PD evolved, the Movement Disorder Society (MDS) critiqued the UPDRS [Citation5] and published a revised version referred to as the MDS-UPDRS [Citation6]. The UPDRS had four parts: (1) mentation, behavior, and mood; (2) activities of daily living; (3) motor examination; and (4) complications of therapy. The MDS-UPDRS retains the four subscales, but the focus of each was revised: (1) non-motor experiences of daily living; (2) motor experiences of daily living; (3) motor examination; and (4) motor complications. Clinical trials of DMTs typically use the UPDRS or MDS-UPDRS as the primary outcome, since HY stage alone is not sufficiently sensitive to changes in disease progression, especially for recently diagnosed patients. Some disease progression models have been published for UPDRS [Citation7,Citation8], although MDS-UPDRS is now more widely used in trials.
Many health economic models have assessed the cost-effectiveness of existing treatments for PD, and a systematic literature search was conducted to identify studies evaluating the cost-effectiveness of treatments for PD and examining the modeling approach adopted [Citation9–11]. Most were Markov models that typically modeled transition between health states defined based on HY. Only two models tracked disease progression using the UPDRS and considered the non-motor aspects [Citation8,Citation12]; none used the MDS-UPDRS. Thus, the need was identified for a new model of PD to support the assessment of the health economics of DMTs for early PD, using the MDS-UPDRS in line with current clinical trial designs – recent examples include Exenatide-PD3 [Citation13], PASADENA [Citation14], PD STAT [Citation15], and SPARK [Citation16]. Furthermore, this new model addresses the unmet need for a disease simulator that considers the full spectrum of the disease progression from diagnosis, and could be used to understand the potential clinical and economic value of new DMTs for early PD. Based on the learning from Alzheimer’s disease, there is a recent uptake of Parkinson’s disease research focusing on early disease modification [Citation17], which poses unanswered health economic questions.
The objective of this study was to develop and validate a novel model to simulate the disease progression of PD from diagnosis over a lifetime horizon capturing motor and non-motor symptoms, health outcomes, and the associated costs. This model addresses the limitations of existing published models by using predictive equations that capture the intercorrelation between UPDRS subscales and incorporate baseline characteristics of individual simulated patients to model the progression of the MDS-UPDRS and UPDRS subscales over time. The simulation was developed to predict long-term clinical outcomes of new treatments, such as DMTs, in addition to the current standard of care, and can be leveraged to conduct cost-effectiveness analyses and clinical trial simulation. A case study was conducted to assess the cost-effectiveness of a DMT plus standard of care (SOC) vs. SOC alone in the UK. The case study results illustrated the main drivers of cost-effectiveness and identified important data gaps.
Methods
Model overview
The model simulates the clinical and economic outcomes of individual patients with PD, up to a lifetime horizon. An overview of the framework is provided in . The model supports comparing a new treatment (as an add-on to SOC) to the current SOC (symptomatic treatments). The simulation captures both the benefits of starting symptomatic treatments, dose adjustments, and longer-term limitations, such as increasing off-time and the associated complications of dopaminergic therapies. The model reports health and economic outcomes, including life years (LY), quality-adjusted life years (QALY), and several cost categories.
Figure 1. Model influence diagram
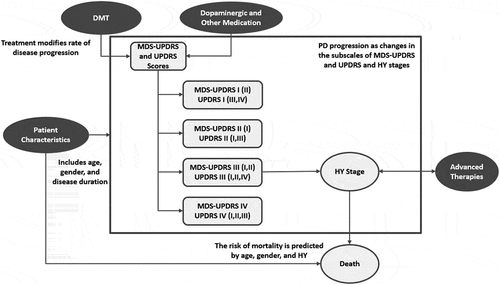
This individual patient-level simulation is implemented in Microsoft Excel® using the discretely integrated condition event (DICE) approach, which conceptualizes the decision problem in terms of the information handled by the model over time (‘conditions’) and the changes of those conditions that occur at specific points in time (‘events’) [Citation18]. The DICE approach is particularly suitable for developing event-driven simulation models and facilitates the implementation of such models in Microsoft Excel® without the need for specialized software. This simulation accounts for individual patient characteristics and represents the progression and management of PD as a combination of evolving conditions (age, MDS-UPDRS, UPDRS, HY, costs, and utilities) and events (distinct points in time where conditions change, such as treatment changes or discontinuation and death).
The model was constructed as an individual patient simulation to represent the heterogeneity observed in progression rates and capture the potential benefits of novel DMTs. Disease progression was characterized in terms of changes in MDS-UPDRS, UPDRS, and HY in the simulation, and accounts for the motor and non-motor aspects of the disease, as well as complications of therapy. Realistic simulation of progression requires consistent predictions between these various measures. The short-term benefits of symptomatic treatments, their long-term limitations, and the associated complications of therapy are captured in the underlying disease progression risk equations. Moreover, by separately tracking symptomatic and underlying disease progression, the model captures the long-term benefits of DMTs on disease progression as distinct from symptomatic improvements.
The input values and data sources are summarized in ; additional details are provided in the Supplement.
Table 1. Model inputs and sources
Table 2. Simulation base case results for a hypothetical DMT added to standard of care vs. standard of care over a lifetime horizon
Population
A target population of newly diagnosed, treatment-naïve patients at baseline was analyzed to understand the potential value of early treatment with DMT in such a population. Each simulated patient carries a profile of risk factors to predict progression, treatment changes, and mortality. These risk factors are updated over time in the simulation, according to predictive equations. Constraints can be applied to the baseline profiles selected to adapt the model to simulate a cohort of individuals aligned with a specific population. For the analyses presented, for example, the profiles were generated for a newly diagnosed treatment-naïve cohort [Citation20] by jointly sampling correlated characteristics observed in Parkinson’s Progression Markers Initiative (PPMI); the simulated cohort has a mean age of 61 years and mean time since PD diagnosis of six months (range 0 to 48 months). PPMI is a prospective cohort study initiated in 2010, enrolling 423 patients with a diagnosis of PD in the past two years and not on PD medications at baseline [Citation20]. Additional details are provided in Supplement Table S1.
Disease progression and mortality
The simulation of disease progression and mortality for newly diagnosed patients (treatment naïve at baseline) with Parkinson’s disease was based on a set of interrelated predictive equations for MDS-UPDRS, developed from analyses of longitudinal data obtained from PPMI (see Supplement for more details). Predictive equations were developed to capture both between and within patient variation in simulated outcomes. The longitudinal data from PPMI were analyzed using mixed-effect model repeated measures (MMRM) to predict change from previous values of MDS-UPDRS I (non-motor experiences of daily living), MDS-UPDRS II (motor experiences of daily living), and MDS-UPDRS III (motor examinations). This approach identified the predictors of change in subscale scores and captured the dependence between these subscales, leveraging prior values and rates of change in the subscale. Random effects of subjects were accounted for in the models by including a random intercept term. Statistical analysis to derive the MMRM model was conducted using SAS version 9.4. Initiation of PD symptomatic treatments (e.g., levodopa, dopamine agonists, or other such as monoamine oxidase-B inhibitors) were identified as predictors of MDS-UPDRS II and III scores, and the time to initiation of PD medications was based on the observed distributions of PPMI. Additional predictors in the PPMI-based models included gender, age, disease duration, time, baseline MDS-UPDRS scores, and prior MDS-UPDRS scores (Supplemental Table S2). The form of each predictive equation is a general linear model. For instance, the predictive equation for change from prior visit in MDS-UPDRS III is defined as follows:
Where represents mean change from prior visit in MDS-UPDRS III;
and
represent baseline MDS-UPDRS II and III;
and
represent MDS-UPDRS I and III at prior visit;
represents patients not receiving any PD medications; and
represents patients receiving PD medications other than dopaminergic medications.
PPMI provides up to 6 years of follow-up, with a smaller number of observations available in year 5 and 6, which has the potential to limit the long-term accuracy of predictions beyond this time frame. Thus, leveraging disease progression predictions derived from several data sources was required to accurately represent the full course of the disease – that is, there does not exist a single data source that tracks the full course of the disease, from the newly diagnosed stage of the disease to advanced PD and end of life.
In addition to the PPMI-based predictions, the simulated disease trajectories can be supplemented by a second set of predictive equations, with a switch between equations defined according to individual characteristics, such as medication status, time since baseline, or current MDS-UPDRS score. In the case study presented herein, when patients initiate symptomatic treatments, disease progression is predicted via a separate set of equations for UPDRS I–IV, developed from an analysis of NET-PD LS-1 (additional details provided in Supplement) [Citation21,Citation22]. The NET-PD LS-1 included patients receiving stable doses of dopaminergic treatment at baseline. The patient-level longitudinal dataset included 1,720 patients with five years of follow-up and was analyzed to develop equations to predict changes over time in UPDRS parts I to IV, levodopa-equivalent doses (LED), and percent off-time. LED is predicted to increase over time in the model and impacts the UPDRS I to IV scores. Predictors in the NET-PD LS-1-based UPDRS models included gender, disease duration, age, LED, time, off time, baseline UPDRS scores, prior UPDRS scores, rate of change in prior UPDRS scores, and several interactions between terms (Supplemental Table S3).
The conversions between UPDRS and MDS-UPDRS scales were based on the linear relationships published by Goetz et al. [Citation23] A UPDRS III threshold (≥27 in the case study) was used to predict the time to HY stage 3; since HY was not collected in NET-PD LS-1, the UPDRS III threshold was derived by analyzing PRECEPT [Citation24] and PostCEPT [Citation25] patient-level data (see Supplement for description of the study). PRECEPT, which enrolled patients with PD who were not on PD medications at baseline, concluded prematurely (pre-specified endpoints were not met) and was transformed into the PostCEPT study.
The progression between HY stages 3 to 5 was based on published transition probabilities; Johnson and colleagues conducted a meta-analysis of 10 studies published between 1 January 1990 and 22 April 2010 [Citation26]. These stages were further stratified by the amount of off-time experienced (percentage of waking day). Based on Lowin et al., 2017 and several published models, the advanced therapies were assumed to have an initial, one-time shift in HY stage and no subsequent benefit [Citation9,Citation27–29].
Age- and gender-specific mortality for the UK general population [Citation30] were adjusted to capture the impact of PD by applying hazard ratios (HR) by HY stage (see ). This approach aligns with other published pharmacoeconomic models [Citation26,Citation28].
Utilities
An MMRM was developed to predict EQ-5D-3L based on an analysis of data collected in NET-PD LS-1, and this predictive equation was used to assign patient utilities up to HY stage 3. Up to six years of follow-up was available in the NET-PD LS-1 dataset, with 1,741 observations at baseline and 330 observations at year 6. Gender, non-motor-, and motor-related aspects of the disease were identified as significant predictors of EQ-5D-3L utilities (see Supplement for more details). Once patients transition to HY stage 3, utilities are assigned conditional on HY stage and off-time (), following the approach used by Lowin et al. [Citation28], which estimated these values from a pooled dataset of EQ-5D-3L, derived from four PD studies (N = 2,162).
Caregiver disutilities () were obtained from Kalabina et al. [Citation31] and assigned conditional on HY stage of patients. Kalabina et al. [Citation31] reported caregiver EQ-5D utility decrements by patient HY while controlling for age and gender (derived from an analysis of patient-level data from the Adelphi Real World Parkinson’s Disease Specific Program 2012).
Costs
Costs were reported in 2020 British pounds (GBP; ) and were inflated from prior years, where necessary. UK healthcare resource profiles were derived from a survey published by Findley et al. [Citation32] reporting inpatient and outpatient care, and direct non-medical services (nursing home, residential care, sheltered housing, home help, and respite care). Unit costs were extracted for each resource and applied to derive updated average annual costs by HY stage (see ).
Pharmaceutical costs were estimated based on a weighted average of treatment distributions in the UK and LED using various sources (see Supplement for details). The treatment class was based on time-dependent probabilities observed in the PPMI study [Citation20], where many started medications within the first few years and most initiated dopaminergic therapy by year 5. Kalilani et al. [Citation33] studied treatment patterns in a retrospective cohort study using the UK Clinical Practice Research Datalink (CPRD) database (2004 to 2015; N = 7,775). The most common first-line PD medications in the CPRD database were included in the cost estimates derived per LED mg. The percent receiving advanced therapies and the associated treatment durations were derived from OBSERVE-PD, a cross-sectional study (N = 2,615; 128 movement disorder centers in 18 countries [Citation34]).
Validation analyses
To assess whether the predictive equations predict accurately when used together in a disease simulator, the predictive equations were validated in two ways. The equations developed for MDS-UPDRS and UPDRS subscales were first confirmed to predict accurately by comparing the predicted and observed scores each year post-baseline in PPMI and NET-PD LS-1, respectively; that is, observed values of the predictors were propagated through the equation and predicted outcomes were compared with the observed. The equations were then implemented in the model and the simulated outcomes confirmed to align with the observed data for three cohorts: 1) newly diagnosed treatment-naïve patients with PPMI characteristics at baseline and applying PPMI-based equations; 2) patients on PD medication and with NET-PD LS-1 characteristics at baseline and NET-PD LS-1-based equations; and 3) a cohort with the PRECEPT characteristics at baseline with NET-PD LS-1-based equations. In this way, the predicted values from one equation were used as predictors for correlated measures, and thus this assesses the joint validity of the equations when implemented in the disease simulation.
PD progression, mortality, and utilities after HY stage 3 were derived from the same sources as many PD models that have focused on more advanced stages of the disease and have been published in peer-reviewed journals[Citation28]. The model projections for this stage were compared with the source data; however, an external validation was not undertaken because of unavailability of suitable data.
Hypothetical DMT analyses
The perspective of the analyses is the UK National Health Service and includes direct medical and non-medical costs over a lifetime, and accumulated QALYs, discounted at 3.5% per year [Citation35]. The model was used to simulate newly diagnosed patients (mean time since diagnosis of six months, range 0 to 48) starting a DMT in addition to the current SOC. For the DMT, a 50% change in MDS-UPDRS progression vs. natural history was assumed; further, a 5% discontinuation rate in the first year and an annual rate of discontinuation of 2.5% in subsequent years were assumed for the patients on DMT. Patients who discontinue DMT were assumed to experience a gradual loss of treatment effect over two years – reverting to the SOC trajectories. The deterministic sensitivity analyses were conducted by systematically varying parameters from the base case one at a time. The analysis evaluated a lower and upper bound for each model parameter considered.
Results
Validation of disease progression equations
The disease progression validation results for were summarized for the aforementioned three cohorts: 1) internal validation of PPMI data (); 2) interval validation of NET-PD LS-1 data (Supplement Figure S1); and 3) external validation of NET-PD LS-1 equations using a PRECEPT-based cohort (Supplement Figure S2). The predictive equations generally performed well, as observed by comparing the simulated outcomes with the observed PPMI (MDS-UPDRS), NET-PD LS-1 and PRECEPT (UPDRS) data each year post baseline [Citation25]. PRECEPT [Citation24] enrolled patients who were not on PD medications at baseline.
Figure 2. Simulation of movement disorder society unified Parkinson’s disease rating scale scores over time for a newly diagnosed cohort: comparison of simulated with observed outcomes
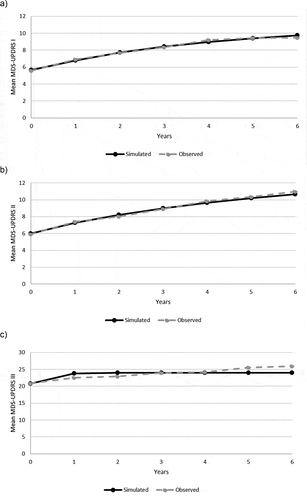
The simulated MDS-UPDRS trajectories predicted by the PPMI-based equations for the treatment-naïve cohort closely match the observed values from PPMI (). Similarly, the simulated UPDRS trajectories predicted by the LS-1-based equations for the on-treatment cohort match the observed values from LS-1 reasonably well (Supplementary Figure S1). These validation analyses illustrated how the model appropriately simulated changes in the three subscales over time for both treatment-naïve and treatment-experienced patients.
For the external validation comparing the simulated UPDRS trajectories for a simulated cohort with PRECEPT characteristics at baseline, the NET-PD LS-1 equations yielded predictions consistent with the observed averages, attesting to the generalizability of the equations outside the NET-PD LS-1 population. The validation dataset included PRECEPT participants who went on to participate in PostCEPT, and subjects who had ‘scans without evidence of dopamine deficiency’ were excluded from the data set. The mean values at two years for the simulated and observed values, respectively, were as follows: for UPDRS 1, 1.20 vs 1.14; UPDRS 2, 7.63 vs 7.78; and UPDRS 3, 21.41 vs 21.72. Additional details are provided in the Supplement Figure S2.
Economic analyses of a hypothetical DMT
Over a lifetime horizon, undiscounted LYs were estimated to be 17.8 with SOC, and DMT added to SOC yielded an increase of 0.7 LYs and 1.9 QALYs (). The discounted incremental gains were 0.3 LYs and 1.1 QALYs. Patients treated with SOC were projected to incur discounted costs of £232,619 over a lifetime – with 26% from treatment costs, and 55% from non-medical costs (including respite care and nursing home costs). As acquisition and administration costs of the hypothetical DMT were not considered, the total costs were lowered by 16%. The costs were largely offset due to a predicted reduction in hospitalizations, nursing home admissions, and at home care. Overall for this hypothetical DMT, these base-case values led to an annual economically justifiable price estimate of £6,908 to £8,206 for willingness-to pay-thresholds in the range of £20,000 to £30,000 [Citation35]. Although higher willingness-to-pay thresholds may be considered, these thresholds are generally considered appropriate by the National Institute for Health and Care Excellence (NICE). The annual economically justifiable price in other markets such as the US may be higher, as higher willingness-to-pay thresholds may also be acceptable. Deterministic sensitivity analyses identified the drivers of the results to be the efficacy assumptions, as the reduction in disease progression rate has the largest impact, patient utilities, and direct non-medical costs ().
Figure 3. Deterministic sensitivity analysis results
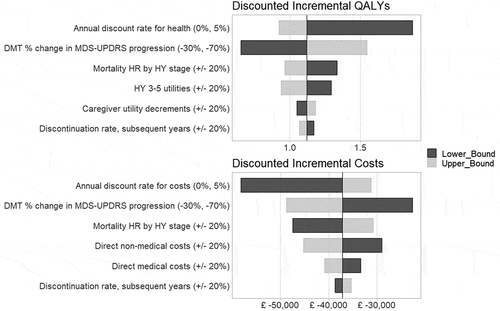
Discussion
The model developed for this study was able to predict PD progression for newly diagnosed patients, providing a new foundation for conducting simulations early in the disease and for evaluating health benefits and economic impacts. The model accounted for the influence of multiple factors on the rate of progression of motor and non-motor symptoms and captured the dependence between the various measures, leveraging prior values and rates of change to obtain realistic predictions. The predictors for the various subscales included age, duration of disease, gender, initiation of PD medications and LED, as well as off-time.
The model was used to conduct analyses to explore the potential value of a novel, hypothetical DMT for the treatment of early PD by running various aspirational efficacy scenarios. While conducting exploratory scenario analyses, the ability to vary the assumptions such as the anticipated durability of the benefits can be informative early in the development of a novel DMT; it may also help to inform internal decision-making for trial designs. As expected, the assumed reduction in disease progression rate significantly impacted the results, as well as the assumptions related to how early treatment was started, patient utilities, and direct non-medical costs. The local access to nursing home care and annual costs varied widely between countries and is expected to impact the cost-effectiveness estimates. Discounting substantially reduces the predicted impact of any DMT on health outcomes, as PD is a slowly progressing disease, and the DMT was assumed to have no benefit at initiation. When a DMT slowed the rate of progression, the benefit continued to accrue over time and the long-term benefits on quality of life were reduced by discounting. This also applies to other chronic diseases and has led to the appropriate discount rates to be discussed by health technology assessment groups, some of which have recently allowed lower rates over time [Citation36].
PD is a slowly progressing disease and no single data source was able to support developing equations to model the long-term outcomes. De novo multivariate predictive equations were developed to support modeling non-motor and motor symptoms experienced at the early stages by analyzing two data sources; the later stages (i.e., HY stages 3+) were modeled by focusing on off-time and motor symptoms using the same estimates as many other published models developed for advanced therapies.
The model was implemented and validated following the best modeling practices [Citation37,Citation38], including validation of the projections; however, some uncertainties existed due to data limitations. The established conversions between parts 2 and 3 published by MDS were used, for example, to map MDS-UPDRS to UPDRS to estimate the utilities prior to starting PD medication or HY stage 3. However, due to the nature of the changes to part 1, these mappings were not considered straightforward by the MDS researchers; nevertheless, once patients initiate medications, the UPDRS scales are modeled to ensure the appropriate predictive equations are used based on the underlying population. If future studies report MDS-UPDRS scores over time for patients with more advanced PD and treated with dopaminergic medications, additional predictive equations could be developed to inform the model. There were also some data gaps, such as studies reflecting current management practices to inform the cost estimates. More recent data for the UK and other markets would better reflect the current practices, especially as more advanced therapies were launched. The perspective of the analyses was limited to direct costs and did not consider additional societal costs such as productivity losses.
Relatively few long-term studies have been conducted using MDS-UPDRS, and the validation and refinement of the predictive equations will continue as more data become available. PPMI was therefore used to develop the functions for projecting treatment-naïve progression as well as for validation of the model. The model was extensively validated against PPMI, NET-PD LS-1, and PRECEPT by comparing the observed and simulated results. Although the NET-PD LS-1 equations appeared to be slightly overestimating UPDRS scores when compared against the observed results from NET PD LS-1, the differences were below minimal clinically important differences and these equations still validated well in the PRECEPT population. However, validation in other populations would provide a more complete understanding of the generalizability of the MDS-UPDRS-based equations.
Future research could enhance this model framework, if other datasets can be accessed that include newly diagnosed patients and MDS-UPDRS or patients with more advanced PD. The model framework was developed to conduct exploratory health economic analyses of a hypothetical DMT for the treatment of early PD. Different considerations would need to be taken into account for modeling advanced therapies, which have the potential to be disease-modifying, and are being developed to treat later stages of the disease. This framework, however, can support conducting simulations that can inform internal decision-making, trial design, and strategic planning early in the development of DMTs – particularly for those focused on early disease modification.
Authors’ roles
All authors meet the International Committee of Medical Journal Editors criteria for authorship, take responsibility for the integrity of the entirety of this work, and have given final approval to this version.
All authors contributed to the drafting of the manuscript and reviewing the presentation and interpretation of the results. The authors participated in the conceptualization of the design of the model (CC, HF, AW, and PG), acquisition of data (AW, HF, and CC), statistical analyses (IP, CF, YY, HF and CC), execution of the model design (PG, AC, HF, CC) and validation (HF, CC, PG, YY, AW and AC).
Supplemental Material
Download MS Word (165.6 KB)Acknowledgments
This research is based on datasets obtained from the Parkinson’s Progression Markers Initiative (PPMI) database (www.ppmiinfo.org/data). For up-to-date information on the study, visit www.ppmiinfo.org.
This research is based on the National Institute of Neurologic Disease and Stroke’s Archived Clinical Research data (NET-PD LS-1 Creatine in Parkinson’s Disease; Karl Kieburtz, MD, MPH [Writing Group for the NET-PDI, 2015]; PRECEPT A Randomized, Double-Blind, Placebo-Controlled Dose-Finding Study to Assess the Efficacy and Safety of CEP-1347 in Patients with Parkinson’s Disease; Ira Shoulson, MD [The Parkinson Study Group 2007]; and PostCEPT: A Longitudinal Follow-up of the PRECEPT Study Cohort; Roger M. Kurlan MD [Ravina, et al., 2009]) received from the Archived Clinical Research Dataset web site.
Supplementary material
Supplemental data for this article can be accessed here.
Additional information
Funding
References
- Rogers G, Davies D, Pink J, et al. Parkinson’s disease: summary of updated NICE guidance. BMJ. 2017;358:j1951.
- Dawson VL, Dawson TM. Promising disease-modifying therapies for Parkinson’s disease. Sci Transl Med. 2019;11:520.
- Hoehn MM, Yahr MD. Parkinsonism: onset, progression and mortality. Neurology. 1967;17(5):427–11.
- Goetz CG, Poewe W, Rascol O, et al. Movement disorder society task force report on the Hoehn and Yahr staging scale: status and recommendations. Mov Disord. 2004;19(9):1020–1028.
- Movement Disorder Society Task Force on Rating Scales for Parkinson’s D. The Unified Parkinson’s disease rating scale (UPDRS): status and recommendations. Mov Disord. 2003;18(7):738–750.
- Goetz CG, Tilley BC, Shaftman SR, et al. Movement Disorder Society-sponsored revision of the Unified Parkinson’s Disease Rating Scale (MDS-UPDRS): scale presentation and clinimetric testing results. Mov Disord. 2008;23(15):2129–2170.
- Venuto CS, Potter NB, Dorsey ER, et al. A review of disease progression models of Parkinson’s disease and applications in clinical trials. Mov Disord. 2016;31(7):947–956.
- Fundament T, Eldridge PR, Green AL, et al. Deep brain stimulation for Parkinson’s disease with early motor complications: a UK cost-effectiveness analysis. PLoS One. 2016;11(7):e0159340.
- Dams J, Bornschein B, Reese JP, et al. Modelling the cost effectiveness of treatments for Parkinson’s disease: a methodological review. Pharmacoeconomics. 2011;29(12):1025–1049.
- Folse H, Chandler C, Alvarez P, et al. Model-based economic evaluations of treatments for Parkinson’s disease: a systematic literature review. Paper presented at: International Society for Pharmacoeconomics and Outcomes Europe; Barcelona, Spain; 2018.
- Jorgensen J, Servos S, Kefalas P. The potential price and access implications of the cost-utility and budget impact methodologies applied by NICE in England and ICER in the US for a novel gene therapy in Parkinson’s disease. J Mark Access Health Policy. 2018;6(1):1500419.
- Hoerger TJ, Bala MV, Rowland C, et al. Cost effectiveness of pramipexole in Parkinson’s disease in the US. Pharmacoeconomics. 1998;14(5):541–557.
- ClinicalTrials.gov. Exenatide once weekly over 2 years as a potential disease modifying treatment for Parkinson’s disease (exenatide-PD3). [ cited 2021 Apr 2]. Available from: https://clinicaltrials.gov/ct2/show/NCT04232969
- ClinicalTrials.gov. A study to evaluate the efficacy of prasinezumab (RO7046015/PRX002) in participants with early Parkinson’s disease (PASADENA). [ cited 2021 Apr 2]. Available from: https://clinicaltrials.gov/ct2/show/NCT03100149
- Carroll CB, Webb D, Stevens KN, et al. Simvastatin as a neuroprotective treatment for Parkinson’s disease (PD STAT): protocol for a double-blind, randomised, placebo-controlled futility study. BMJ Open. 2019;9(10):e029740.
- ClinicalTrials.gov. Evaluating the safety, pharmacokinetics, and pharmacodynamics of BIIB054 in participants with Parkinson’s disease (SPARK). [ cited 2021 Apr 2]. Available from: https://clinicaltrials.gov/ct2/show/NCT03318523
- Smedinga M, Darweesh SKL, Bloem BR, et al. Towards early disease modification of Parkinson’s disease: a review of lessons learned in the Alzheimer field. J Neurol. 2021;268(2):724–733.
- Caro JJ. Discretely integrated condition event (DICE) simulation for pharmacoeconomics. Pharmacoeconomics. 2016;34(7):665–672.
- Kaltenboeck A, Johnson SJ, Davis MR, et al. Direct costs and survival of medicare beneficiaries with early and advanced Parkinson’s disease. Parkinsonism Relat Disord. 2012;18(4):321–326.
- Parkinson’s Progression Markers Initiative. Home page. [ cited 2018 Jan]. Available from: www.ppmi-info.org
- Writing Group for the NINDS Exploratory Trials in Parkinson Disease Investigators, Kieburtz K, Tilley BC, et al. Effect of creatine monohydrate on clinical progression in patients with Parkinson's disease: a randomized clinical trial. JAMA. 2015;313(6):584–593.
- National Institute of Neurological Disorders and Stroke. Archived clinical research data (NET-PD LS-1 creatine in Parkinson’s disease; Karl Kieburtz, MD, MPH). [ cited 2021 Jan]. Available from: https://www.ninds.nih.gov/Current-Research/Research-Funded-NINDS/Clinical-Research/Archived-Clinical-Research-Datasets
- Goetz CG, Stebbins GT, Tilley BC. Calibration of unified Parkinson’s disease rating scale scores to movement disorder society-unified Parkinson’s disease rating scale scores. Mov Disord. 2012;27(10):1239–1242.
- Parkinson Study Group PI. Mixed lineage kinase inhibitor CEP-1347 fails to delay disability in early Parkinson disease. Neurology. 2007;69(15):1480–1490.
- Ravina B, Tanner C, Dieuliis D, et al. A longitudinal program for biomarker development in Parkinson’s disease: a feasibility study. Mov Disord. 2009;24(14):2081–2090.
- Johnson SJ, Diener MD, Kaltenboeck A, et al. An economic model of Parkinson’s disease: implications for slowing progression in the USA. Mov Disord. 2013;28(3):319–326.
- Eggington S, Valldeoriola F, Chaudhuri KR, et al. The cost-effectiveness of deep brain stimulation in combination with best medical therapy, versus best medical therapy alone, in advanced Parkinson’s disease. J Neurol. 2014;261(1):106–116.
- Lowin J, Sail K, Baj R, et al. The cost-effectiveness of levodopa/carbidopa intestinal gel compared to standard care in advanced Parkinson’s disease. J Med Econ. 2017;20(11):1207–1215.
- Walter E, Odin P. Cost-effectiveness of continuous subcutaneous apomorphine in the treatment of Parkinson’s disease in the UK and Germany. J Med Econ. 2015;18(2):155–165.
- Office for National Statistics. National life tables, UK: 2016 to 2018. Fit parametric distributions to reported survival data.
- Kalabina S, Belsey J, Pivonka D, et al. Cost-utility analysis of levodopa carbidopa intestinal gel (Duodopa) in the treatment of advanced Parkinson’s disease in patients in Scotland and Wales. J Med Econ. 2019;22(3):215–225.
- Findley LJ, Wood E, Lowin J, et al. The economic burden of advanced Parkinson’s disease: an analysis of a UK patient dataset. J Med Econ. 2011;14(1):130–139.
- Kalilani L, Friesen D, Boudiaf N, et al. The characteristics and treatment patterns of patients with Parkinson’s disease in the USA and UK: a retrospective cohort study. PLoS One. 2019;14(11):e0225723.
- Fasano A, Fung VSC, Lopiano L, et al. Characterizing advanced Parkinson’s disease: OBSERVE-PD observational study results of 2615 patients. BMC Neurol. 2019;19(1):50.
- National Institute for Health and Care Excellence (NICE). Guide to the methods of technology appraisal 2013. Published 2013 [cited 2021 Jan]. Available from: https://www.nice.org.uk/process/pmg9/chapter/foreword
- National Institute for Health and Care Excellence (NICE). Reviewing our methods for health technology evaluation: consultation. [ cited 2021 Feb 1]. Available from: https://www.nice.org.uk/about/what-we-do/our-programmes/nice-guidance/chte-methods-consultation
- Caro JJ, Briggs AH, Siebert U, et al. Force I-SMGRPT. modeling good research practices–overview: a report of the ISPOR-SMDM modeling good research practices task force-1. Med Decis Making. 2012;32(5):667–677.
- Roberts M, Russell LB, Paltiel AD, et al. Conceptualizing a model: a report of the ISPOR-SMDM modeling good research practices task force-2. Med Decis Making. 2012;32(5):678–689.