ABSTRACT
The biopsychosocial model is among the most influential frameworks for human-centered health improvement but has faced significant criticism– both conceptual and pragmatic. This paper extends and fundamentally re-structures the biopsychosocial model by combining it with sociotechnical systems theory. The resulting biopsychosociotechnical model addresses key critiques of the biopsychosocial model, providing a more “practical theory” for human-centered health improvement. It depicts the determinants of health as complex adaptive system of systems; includes the the artificial world (technology); and provides a roadmap for systems improvement by: differentiating between “health status” and “health and needs assessment”, [promoting problem framing]; explaining health as an emergent property of the biopsychosociotechnical context [imposing a systems orientation]; focusing on “interventions” vs. “treatments” to modify the biopsychosociotechnical determinants of health, [expanding the solution space]; calling for a participatory design process [supporting systems awareness and goal-orientation]; and including intervention management to support the full lifecycle of health improvement.
1. Introduction
This article presents the biopsychosociotechnical model, which extends the biopsychosocial model by combining it with sociotechnical systems theory. This combined model offers a more “practical theory” for assessing determinants of health and taking action to improve them through the participatory design and management of systems-focused interventions.
The biopsychosocial model is among the most influential frameworks for person-centered care and health improvement. An early example of systems thinking in healthcare, it was introduced by George Engel as a new scientific model to address the limitations of the biomedical paradigm, which he saw as unscientifically reductionist; rooted in mind-body dualism; and neglectful of the patient as a person (G L Engel, Citation1981; George L., Citation1977).
Engel argued that health and illness cannot be fully captured by biochemical processes. Thus, “ … to be scientific, a model for medicine must include the psychosocial dimensions (personal, emotional, family, community) in addition to the biological aspect (diseases) of all patients”.(Smith, Citation2002)
As illustrates, the biopsychosocial model conceptualizes health status and the determinants of health in terms of a single nested system with many levels, with the person level as the “primary frame of reference”. (George L Engel, Citation1978) This is intended to promote a more holistic and contextualized understanding of patients and their health status.
Figure 1. The biopsychosocial model(used by permission, Engel (Citation1981)).
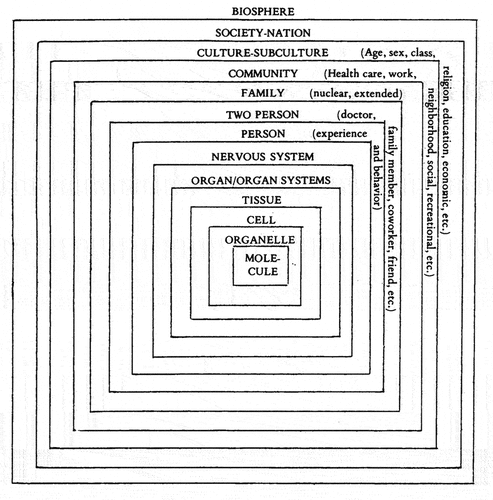
The goals of the biopsychosocial model are important. Compassionate, whole-person care delivers better results, including both “soft” (self-reported) health outcomes and “hard” measures, such as reduced all-cause mortality (Dambha-Miller et al., Citation2019; Trzeciak & Mazzarelli, Citation2019), and as an aspiration, biopsychosocial care is widely accepted.
The model is influential in literally setting the terms by which many researchers and practitioners consider the determinants of health. For instance, in the United States, it has influenced the requirements for physician residency programs (Frankel & Quill, Citation2005), is an almost universal part of pain management training for physical therapists (Hoeger & Sluka, Citation2015), and is a required competency for masters-prepared nurses (American Association of Colleges of Nursing, Citation2011). It also underpins a number of clinical approaches and validated measures (D. T. Wade & Halligan, Citation2017). However, it has not been as influential in shaping frontline practice as its proponents would desire, and critiques of the model have suggested opportunities for improvement on both conceptual and practical grounds.
The remainder of this paper will use the inductive top-down theorizing method (described in the next section) to identify and address those opportunities for improvement. First, it will explore key theoretical problems in the biopsychosocial model. Next it will describe sociotechnical systems theory a second “kernel theory” (alongside the biopsychosocial model) to build an extended mid-range theory/design process model that addresses these problems. It will then introduce the proposed solution, the biopsychosociotechnical model, and discuss how this extended and restructured approach supports the realization of the biopsychosocial model's humanistic aims.
2. Methods
Inductive top-down theorizing was used to investigate theoretical problems in the biopsychosocial model, and iteratively construct (and reconstruct) a problem frame and proposed solution, guided by conceptual representations of the biopsychosocial model in is original form and the proposed new model (Shepherd & Sutcliffe, Citation2018). Using the inductive top-down theorizing method, research literature serves as a primary data source for identifying theoretical problems. After an initial exploration of the theoretical problems of the biopsychosocial model (described below), the goal became the construction of a mid-range theory/design process model (Wynn & Clarkson, Citation2004) for health improvement interventions (Venable, Citation2006). The inductive top-down theorizing process was guided in part by the author's prior training in the fields of public health and design, and “gists” drawn from the literatures in these fields, along with the conceptual representations described above, until a new theory (the biopsychosociotechnical model) was finalized, based on two “kernel theories” (Venable, Citation2006)–the biopsychosocial model and sociotechnical systems theory.
3. Key problems in the biopsychosocial model
The analysis in this section supports an extension of the biopsychosocial model by describing problems that have been identified over the past 40+ years, and which may present opportunities for improvement. Some of the issues identified in the literature are structural, while others could be more appropriately described simply as “branding issues”. In all cases, however, these problems are raised in service of a single goal: not burying the biopsychosocial model, but revising it to more effectively implement its humanistic and person-centered goals.
4. The biopsychosocial model as a model
One of the most common critiques of the biopsychosocial model is that, despite Engel's ambitions, it is not a scientific model at all. An important theme among these critiques is that the model is too vague to be testable (Álvarez et al., Citation2012; Epstein & Borrell-Carrió, Citation2005; Farre & Rapley, Citation2017; McLaren, Citation1998; Smith et al., Citation2013) because it does not “specify variables or specific relations among the components, and does not contain the elements necessary to make specific testable hypotheses” (B. L. B. L. Wood, Citation2012). Smith, et al. (Smith et al., Citation2013) suggest this “ … non-scientific status accounts in large part for its limited penetration into mainstream medicine, especially research”.
This recurring critique (though justified) has distracted from the model's real and significant contributions. The biopsychosocial model continues to shape thinking about health as a highly influential clinical model (a guide to conceptualizing and teaching clinical practice) and scientific meta-theory (a guide to developing models and theories within a broader paradigm) (B. L. B. L. Wood, Citation2012). In addition, Smith, et al. have argued that improved approaches to patient interviewing may provide the data required to “[make] the biopsychosocial model scientific” (Smith et al., Citation2013).
5. A model without a method
Perhaps the most important critique of the biopsychosocial model, especially as a clinical model, is that it is too difficult to implement (Epstein & Borrell-Carrió, Citation2005; Weston, Citation2005). In part, this may stem from a mismatch between Engel's descriptive focus and the action-oriented needs of practitioners:
“Engel’s biopsychosocial model was a descriptive model to understand patients’ illness experiences and, in that way, assist and expand the diagnostic process. Even as his career evolved, he was only secondarily interested in treatment.” (Epstein & Borrell-Carrió, Citation2005)
The BPS model was also introduced without tools for operationalizing its insights (Farre & Rapley, Citation2017; Smith, Citation2002). In part because of this lack, the model “ … does not lend itself to the kind of pragmatic decisions that many clinical situations demand of physicians”. (Freudenreich et al., Citation2010)
Contributing to this difficulty is Engel's failure to provide realistic guidance for gathering the “right” biopsychosocial data to provide “good” biopsychosocial care (Epstein & Borrell-Carrió, Citation2005; Farre & Rapley, Citation2017; Smith et al., Citation2013). Several authors have identified the need for a pragmatic approach to implementation, countering the model's implication that all patients require a comprehensive exploration of all biopsychosocial influences at every encounter (Epstein & Borrell-Carrió, Citation2005; Frankel & Quill, Citation2005; Weston, Citation2005).
6. The structure of the model
The biopsychosocial model depicts health, and the determinants of health, as a single (and simple) nested system. It also depicts this system as static, in the sense that it represents a single moment in time and provides no process for systems improvement. This structure imposes a conceptual approach that has been criticized as both unhelpful and often incorrect (J. Sturmberg & Martin, Citation2004). Álvarez, et al. (Álvarez et al., Citation2012) label this “the key conceptual problem” with the biopsychosocial model and a significant barrier to clinical application.
Espstein & Borrel-Carrio (Epstein & Borrell-Carrió, Citation2005) describe the underlying problem with structuring the model as a single nested system:
“ … the hierarchy of natural systems is an incomplete model; in some cases, it might not be a hierarchy, and not all levels are weighted equally in all situations. Rather, the elements may be arranged in different ways depending on the problem encountered … . A diagram of this interaction and the relative importance of each element would look more like a matrix or a web rather than a linear ordering of levels. If the ‘theory’ of a biopsychosocial approach rests on verification of a linear hierarchical model, we will not find convincing evidence that that model applies to all situations.”
Contemporary literature views health and the determinants of health as complex adaptive systems whose components interact–often recursively–at multiple levels (Anderson, Citation2015; Black et al., Citation2015; Frank et al., Citation2013; Kruger & Cross, Citation2006; Petticrew et al., Citation2015; J. Sturmberg & Martin, Citation2004; Tipirneni et al., Citation2015; J. J. Ward et al., Citation2017). Perhaps an even more useful lens is that of health as a complex adaptive system of systems (CASoS):
“The definition of a CASoS goes beyond the definition of a complex system [Glass Citation2008a]. CASoS are adaptive in that the system entities or components can change their behavior, which can result in a change in system structure in response to external stimuli. Additionally, system elements exert directional or bidirectional influences on one another that can change the system structure or behavior. CASoS are also systems-of-systems in that they are comprised of individual but connected systems, each of which is irreducible, and in that the behavior and functionality of the CASoS differ from the sum of the behaviors and functionalities of the individual systems of which it is composed. In a system of systems, not only can each entity be characterized as a system with its own rules and agenda, but the interaction among the systems can cause behavioral or structural modifications within the larger, interconnected CASoS”. (Brodsky et al., Citation2011)
Thus, in the context of a biopsychosocial model of health, it would be more useful to consider the biological, psychological, and social components as separate but related complex adaptive systems, each with its own subordinate systems, “rules” and modes of interaction. This understanding is ill-served by the biopsychosocial model's depiction of “a Russian doll on a shelf” – a single, simple, and static nested system.
This understanding dovetails with an analogous argument by Neal & Neal, who advocate a networked vs. nested model for ecological systems theory (Neal & Neal, Citation2013). Applying their logic to the biopsychosocial model, one might say it depicts organelles as nested within nations and societies, “ … but it makes little sense to view the former as a subset of the latter. Instead these are two distinct systems … that influence one another. [Viewing them] as nested undermines the theoretical coherence and conceptual utility of [the model]”. (Neal & Neal, Citation2013),p.726
Perhaps the most important impact of this oversimplification is that it enabled Engel to depict only a static, descriptive model that conflates health and the determinants of health. The biopsychosocial model has nothing explicit (and little implicit) to say about how to improve health, except for the vague guidance that patients should be treated as human beings. This structural shortfall may stem from the fact that treatment was an afterthought for Engel (Epstein & Borrell-Carrió, Citation2005), as well as the early state of systems science at the time that he became aware of the field. In turn, it probably explains much of why the model is seen as un-implementable: From a process perspective, there is nothing to implement.
7. The biopsychosocial excludes the artificial
Another limitation of the biopsychosocial model relates to its scope: It ignores the artificial world. This insight was generated primarily through the iterative model development process and the integration of the biopsychosocial model with sociotechnical systems theory, rather than the literature on the biopsychosocial model, itself. However, some authors have proposed more limited extensions to the biopsychosocial model that imply a recognition of the role of the artificial in influencing health and the need to expand the model's scope to address this.
A recent paper focused on “digital health solutions” proposes a biopsychosocial-digital model, (Ahmadvand et al., Citation2018) the digital component of which includes ideas related to “digital expansion of biological self”, as well as digitally-enabled patient engagement and social networking. Other authors have suggested extensions that include aspects of the built environment and assistive technologies (D. Wade, Citation2015; WHO, Citation2002).
Engel's failure to consider technology is puzzling, because he promoted a systems-focused approach to the determinants of health and explicitly acknowledged the multi-causality of health and illness:
“ … we attempt to prevent the occurrence of the disease state by interfering with the operation of one or more factors before the necessary and sufficient conditions have been achieved. The scientific attitude can permit no restrictions as to the category of natural phenomena investigated; the scientist’s first obligation is to his data, wherever or however it may appear.” (George L Engel, Citation1960)
However, in limiting his purview to “natural phenomena”, Engel failed to live up to the very obligation laid out above. To follow the data wherever it leads, one must also consider the impact of the artificial.
Engel formulated the BPS model in opposition to the biomedical paradigm, which he argued had “acquired the status of dogma” (George L. Engel, Citation1977).
Biomedical dogma requires that all disease, including “mental” disease, be conceptualized in terms of derangement of underlying physical mechanisms. This permits only two alternatives whereby behavior and disease can be reconciled: the reductionist, which says that all behavioral phenomena of disease must be conceptualized in terms of physicochemical principles; and the exclusionist, which says that whatever is not capable of being so explained must be excluded from the category of disease (George L. Engel, Citation1977).
This polemical approach (B. L. B. L. Wood, Citation2012) may have limited the scope of Engel's vision. He appears to have considered technology only in terms of diagnostic and treatment technologies in the hands of physicians, and viewed it as an “impersonal and … mechanical” (George L. Engel, Citation1977) aspect of the biomedical paradigm. Even in later years, he limited his focus to “natural systems”, (George L. Engel, Citation1992) with seemingly no direct interest in the role of artificial systems in shaping health.
Today, the role of the artificial world in influencing health is inescapable. In part, this recognition is driven by evolving technologies (e.g., the Internet and everything connected to it), and in part by an evolving understanding of how designed artifacts shape the determinants of health (e.g., healthcare facility design arguably began coalescing as an industry and field of enquiry as recently as 1988, (Marberry, Citation2019)).
describes a few of the many ways technology (defined broadly as any designed artifact) serves as a determinant of health. While some certainly can play the depersonalizing role Engel envisioned (e.g., electronic health record systems that reduce eye contact between physicians and patients (Asan & Montague, Citation2012) or the infringement of unnecessary diagnostic testing on the clinician-patient relationship (J. P. Sturmberg & Martin, Citation2007)), there are many examples of technological interventions that actively support the biopsychosocial goal of treating patients as human beings (e.g., (Lydahl, Citation2019; Shepley & Pasha, Citation2017)).
Table 1. Examples of how technology can affect health.
More to the point, however, technology is a ubiquitous part of modern life that clearly shapes health status. Whether the impacts of technology are positive or negative, humanizing or dehumanizing, the fact remains that those impacts exist – and are largely ignored by the biopsychosocial model.
If the biopsychosocial model was “ … an emotive case for more humanity and less technology in medicine”,(McLaren, Citation1998) the realities of modern life dictate that it evolve to promote humanity in medicine, regardless of and even enabled by, technology.
8. Continued relevance of the biopsychosocial model
Despite these critiques, the biopsychosocial model remains influential as a touchstone for person-centered and humanistic approaches to health improvement–and especially healthcare training (Freudenreich et al., Citation2010). It has also played an increasingly prominent role in health research. A search of the Pubmed database finds the term “biopsychosocial” appeared in 113 articles in 1990, 274 articles in 2010, and 896 articles in 2020. The biopsychosocial model is particularly influential in certain fields such as pain (Mescouto et al., Citation2020; Miaskowski et al., Citation2020; Moseley & Butler, Citation2015)–it serves as a cornerstone of several national pain strategies (Australian and New Zealand College of Anaesthetists, Citation2010; Interagency Pain Research Coordinating Committee, Citation2016; Waddell et al., Citation2017))–and rehabilitation (Talo & Rytökoski, Citation2016; D. Wade, Citation2015; Wainwright & Low, Citation2020; WHO, Citation2002).
At a more paradigmatic level, the BPS model played a vital role in helping establish the applicability of systems theory to health and health services. This has both directly and indirectly contributed to a an ever-growing range of theoretical and practical advances with implications for the design and implementation of health improvement interventions, the design and improvement of healthcare systems, and the definition of health, itself (Bircher & Kuruvilla, Citation2014; Jeffrey Braithwaite et al., Citation2021; Brodsky et al., Citation2011; Card, Citation2017; Card & Klein, Citation2016; Trisha Greenhalgh & Papoutsi, Citation2018; Hawe et al., Citation2009; Martin & Sturmberg, Citation2009; May et al., Citation2016; Norman, Citation2009; J. P. Sturmberg & Martin, Citation2007; J. J. Sturmberg & Topolski, Citation2014; Wieman & Wieman, Citation2004). And, of course, the BPS model has played an especially important role in legitimizing modern research into the psychosocial determinants of health, helping pave the way for fields of inquiry such as the impact of adverse childhood experiences (ACEs) on health (Boullier & Blair, Citation2018; Burke et al., Citation2011; Dube et al., Citation2002; Felitti, Citation2009; Felitti et al., Citation1998; Hughes et al., Citation2017; Oh et al., Citation2018; Schilling et al., Citation2007).
The conceptual utility of a framework for synthesizing the humanistic and biomedical approaches has served as a bulwark against the BPS model's detractors (Farre & Rapley, Citation2017). And, in a more practical vein, its continuing impact has been supported by implementation strategies that aim to address at least some of the model's shortfalls, while avoiding the temptation to “throw the baby out with the bathwater” (Farre & Rapley, Citation2017).
These implementation strategies include BPS-based patient interviewing and heuristic approaches. In addition, a number of adapted/extended versions of the model have been introduced, which aim to better support the implementation of the model's goals.
8.1. Patient interviewing methods
One promising area of development is the implementation of biopsychosocial care through improved patient interviewing (Frankel & Quill, Citation2005; Huyse et al., Citation2002; Smith, Citation2002).
Smith et al. (Citation2013) have addressed some of the practical and scientific shortcomings of the biopsychosocial model by describing two structured, evidence-based approaches to conducting patient interviews: Smith's integrated patient-centered and doctor-centered interview model, which is the basis of a key textbook (Fortin et al., Citation2019), and the four habits interviewing model (Smith et al., Citation2013)). These tools enable biopsychosocial care by eliciting relevant biopsychosocial data quickly enough (within 3–5 minutes) to fit within the average clinical encounter (Smith et al., Citation2013).
Clinical trials assessing Smith's integrated patient-centered and doctor-centered interview model demonstrated improved patient outcomes. These findings suggest an approach like the biopsychosocial model can have practical impacts if supported by well-designed implementation tools (Smith et al., Citation2013).
8.2. Heuristic approaches
Another path toward implementation of the biopsychosocial model has focused on heuristic approaches, guides to thinking about clinical care in a biopsychosocial way. describes the components of three such tactics.
Table 2. Heuristic approaches for biopsychosocial care.
These heuristic approaches attempt to avoid the shortfalls of the biopsychosocial model by largely ignoring the model, itself. Instead, they aim to support the clinical implementation of what the authors see as the intent behind the model.
The four habits patient interviewing model can also be viewed through a heuristic lens, but it goes further in including step-by-step instructions for integrating of these ways of thinking into the patient interview process.
8.3. Extended models
A number of domain-specific adaptations of the BPS model have also been developed, (Lindau et al., Citation2007; D. Wade, Citation2015; D. T. Wade & Halligan, Citation2017; WHO, Citation2002; Williams et al., Citation2005; B. L. B. L. Wood et al., Citation2008) as well as a few more universal approaches such as the dynamic biopsychosocial model (Lehman et al., Citation2017), the somato-psycho-socio-semiotic model (Bennett et al., Citation2018; J. P. Sturmberg, Citation2021), and the sociopsychobio model (Haslam et al., Citation2019).
All these models address some key critiques of the biopsychosocial model–especially its formulation as a nested system–by reframing the determinants of health as dynamic and interdependent forces that influence not only health status, but also each other. Despite their focus on dynamics, however, these revised models are essentially descriptive. They support health evaluation and problem analysis, but do not include a process for designing interventions to improve health.
9. Minding the gap
The biopsychosocial model represented an important step toward a more humane and human-centered understanding of health and disease. This understanding was not trapped in amber in 1977, however, and with the insights gleaned from more than 40 years of additional research, theory, and societal change, there is important scope for updating and improving the model. As Sturmburg & Martin wrote:
Engel was visionary in so far as he understood the limitations imposed by
the – at his time accelerating – reductionist momentum of medical
research; we need to be visionary to take his induction further for the sake
of humanity and society at large. (J. Sturmberg & Martin, Citation2004)
The remainder of this article attempts to bridge the gaps identified above by extending and fundamentally re-structuring the biopsychosocial model.
10. The missing link: Sociotechnical systems theory
Sociotechnical systems theory is a complementary framework that addresses many of the shortcomings of the biopsychosocial model. Both approaches trace their origins, in part, to early developments in open systems theory (G L Engel, Citation1981; E. Trist & Murray, Citation1993), but sociotechnical systems theory also draws on the work of Kurt Lewin (especially in the areas of field theory and action research (Pasmore et al., Citation2019; E. Trist & Murray, Citation1993; E. L. E. L. Trist, Citation1976)), as well as research on autonomous work groups (E. E. Trist, Citation1981).
11. Sociotechnical systems theory
Sociotechnical systems theory, as such, first arose out of action research conducted by the Tavistock Institute to address production and labor relations issues in the British coal industry, leading to significant improvements (E. E. Trist, Citation1981; E. Trist & Murray, Citation1993). Its initial focus was on the use of self-organizing autonomous work groups. As it spread and matured, sociotechnical systems theory also came to include notions of organizational democracy and participatory design (E. Trist & Murray, Citation1993; E. L. E. L. Trist, Citation1976), as well as continuous learning (E. L. E. L. Trist, Citation1976). Modern sociotechnical systems theory has continued to evolve. In healthcare today, it is best known for contributions to systems safety (J. Braithwaite et al., Citation2009; Pascale Carayon et al., Citation2014; Runciman et al., Citation2010; P. Waterson et al., Citation2015; Wears, Citation2015) and health information technology (Gaskin et al., Citation2011; Singh & Sittig, Citation2016; Sittig & Singh, Citation2010). In both cases, it is strongly associated with systems design.
Sociotechnical systems theory rests on the central premise that work is based on a joint socio-technical system and that both the social and technical components must operate together to achieve success (E. Trist & Murray, Citation1993). This insight gave rise to the principle of joint optimization:
Where the achievement of an objective is dependent upon independent but correlative systems, then it is impossible to optimize for overall performance without seeking to jointly optimize these correlative systems.
Any attempt to optimize for one without due regard to the other will lead to suboptimal overall performance, so even if an effort is made in an industrial situation to follow the traditional pattern, i.e., to optimize the technical system and hope the social system will somehow sort itself out, then suboptimization is certain to result. This is also the case when attempting to optimize each system, but independently, ignoring interaction effects. (Emery, Citation1971) [emhasis in original]
Strictly speaking, optimization is seldom achievable in complex adaptive systems like healthcare and public health. Instead, health practitioners face “wicked problems” (Klein & Young, Citation2015; Rittel & Webber, Citation1973) that do not allow for a single best answer. Wicked problems are the classic domain of design thinking (Cross, Citation1982), and thus a good fit for the participatory, design-oriented approach of sociotechnical systems theory.
For wicked problems, the design goal is to arrive at a solution that satisfices (Cross, Citation1982) – that is, a solution that satisfies all requirements to a sufficient degree, even if it is not the optimal solution to each individual requirement. The term “joint optimization” in this context should be read as “joint satisficing”.
12. Lewinian influences
Although they began as separate streams of research, the development of sociotechnical systems theory was significantly influenced by the research of psychologist Kurt Lewin. At the root of this work is field theory (Lewin, Citation1947b), a term Lewin borrowed from physics to explain the ecological drivers of human behavior. Along with the rest of Lewin's approach to planned change (which also includes group dynamics; action research; and the three-step model of change (Burnes & Cooke, Citation2013)), it helped shape the emergence of sociotechnical systems theory.
Field theory posits that human behaviour in a social system (such as a workplace) is based on a balance of interacting forces that produces a semi-stable but dynamic equilibrium that maintains the status quo. To effect a planned change in behaviour, one must re-balance those forces in favour of the intended outcome. This context-focused approach was formalized by the proposition “behaviour B is a function of the interaction between the person p (or group) and their environment e”, (Burnes & Cooke, Citation2013), or: B= f(p,e). Lewin called for all forces impacting a system to be mapped out and assessed in terms of their impact on both the status quo and the planned change (Burnes & Cooke, Citation2013; Lewin, Citation1947b).
Today, Lewin is best known for his theory of planned change, which he developed to address problems that arose in applying field theory to social change. Specifically, he noted a tendency for systems to revert to baseline after a planned change (a phenomenon that will be familiar to anyone who works in the areas of health behaviour change or treatment adherence):
“[I]t does not suffice to define the objective of a planned change in group performance as the reaching of a different level. Permanency at the new level, or permanency for a desired period, should be included in the objective.” (Lewin, Citation1947a)
In response, Lewin theorized a three-step model of change, made up of 1) Unfreezing (destabilizing the current quasi-stationary equilibrium to enable change), 2) Moving (implementing the change), and 3) Freezing (rebalancing the quasi-stationary equilibrium to sustain the change) (Lewin, Citation1947b).
Lewin's other key contribution to what would become sociotechnical systems theory was action research, which might be best defined as “interventional social science”. Lewin described action research as “ … comparative research on the conditions and effects of various forms of social action, and research leading to social action” (Lewin & Frontiers in Group Dynamics: II, Citation1947c), which “ … proceeds in a spiral of steps each of which is composed of a circle of planning, action, and fact-finding about the results of the action”. (Lewin, Citation1946)
13. Participatory design and the democratization of work
Participatory design and the democratization of work are key themes that sociotechnical systems theory can contribute to the biopsychosocial model (E. Trist & Murray, Citation1993; P. Waterson et al., Citation2015). Though sometimes challenging (Tricia Greenhalgh et al., Citation2016), involving stakeholders in the design of interventions is an important way to gain insight into both the current and desired state of a system to be improved (Taysom & Crilly, Citation2017). This systems awareness is important for enabling joint optimization and is central to both health service design (Tricia Greenhalgh et al., Citation2016) and patient-centered care (Mastro et al., Citation2014) (i.e., the design of health improvement interventions for individual patients).
Sociotechnical systems theory traditionally promoted autonomous work groups as a way of improving both operations and worker satisfaction. In this model, workers are encouraged to manage their own team-based approach to achieving operational goals, and “The function of supervision is to manage the boundary conditions in the group's environment so that the group itself may be freed to manage its own activities”. (E. E. Trist, Citation1981)
This is relevant because–whether they are patients, caregivers, or communities–those who do most of the day-to-day work of improving health do so in a self-organized fashion. Clinicians and public health workers provide crucial support and facilitation, as well as direct care, but they do not wield managerial authority over those they help. Sociotechnical systems theory's focus on creating the conditions for frontline success in cooperation with those who actually do the work is a good fit for the field of health improvement.
14. Action-orientation and support for change
Another important contribution sociotechnical systems theory could make to the biopsychosocial model is its focus on, and support for, taking action. Treatment was an afterthought in the development of the biopsychosocial model (Epstein & Borrell-Carrió, Citation2005), and the model itself is static in nature. In contrast, action research to improve outcomes has always been central to the sociotechnical systems approach.
The Systems Engineering Initiative for Patient Safety (SEIPS) model (P Carayon et al., Citation2006; Pascale Carayon, Citation2006; Holden et al., Citation2013) () is an sociotechnical systems model for patient safety improvement. Because of this adjacency to health improvement work, it serves as an accessible example of the ways in which many sociotechnical systems models are designed to support the improvement process.
Figure 2. The SEIPS 2.0 Model, from (Holden et al., Citation2013) (used by permission).
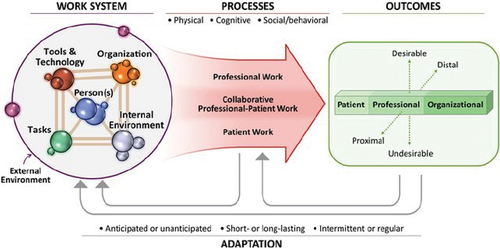
As shows, SEIPS is based on assessing contextual determinants (the work system), processes (the work, itself), and the results of that work in context (outcomes). Feedback loops illustrate adaptations (both planned and unplanned). SEIPS is used to assess the system at baseline and then after improvement interventions. It can also be used to compare alternatives for intervention design (Holden et al., Citation2013). In contrast with the biopsychosocial model's static approach, SEIPS provides its users with an explicit process for understanding and enacting change.
15. Tailoring sociotechnical systems theory for a new context
Sociotechnical systems theory offers a number of advantages that would be useful in extending the BPS model; however, some adaptation is required.
First, although democratization of work processes was an early theme of sociotechnical systems theory, the overall goals of that work (e.g., production quotas) were mostly set in a top-down fashion. Newer approaches have emerged that rely on participatory goal-setting, especially in the context of “expert” workers (P. Waterson et al., Citation2015). It will be important from both practical and ethical perspectives to extend these developments to include patients and communities, who are the experts in their own goals and lived experiences.
Second, sociotechnical systems frameworks focus primarily on formal workers and formal work. Informal health improvement work performed by patients/communities and their support networks is very different from that of clinicians or public health/social care professionals. It is influenced by different goals, barriers, resources, sociotechnical systems, and norms (Holden & Mickelson, Citation2013). As a result, the health improvement work of these populations may not align well with existing sociotechnical systems tools. For instance, current approaches often focus significantly on management-worker relations and adherence to/deviation from formal policies and procedures, neither of which exists in the same form for patients (still less for the populations served by public health professionals). The underlying approach behind these tools appears to be more broadly applicable, however, and could be adapted to better capture the dimensions of patient work (Holden et al., Citation2013; Holden & Mickelson, Citation2013).
Finally, the goal of health improvement is qualitatively different from production quotas. Thus, the determinants, interventions, and outcomes addressed by a hybrid biopsychosocial-sociotechnical systems model would differ from existing sociotechnical systems approaches, though the overall logic would be similar.
16. Results
16.1. The Biopsychosociotechnical Model
The biopsychosociotechnical model () is a practice model (B. L. B. L. Wood, Citation2012) and mid-range theory (Davidoff et al., Citation2015) for assessing determinants of health and taking action to improve them through the participatory design and management of systems-focused interventions. It is based on the following principles:
Biological, psychological, social, and technological inputs operate both as quasi-independent systems and, together, as a complex adaptive system of systems (Ames et al., Citation2011) (the biopsychosociotechnical context) that directly influences health status.
“Health is the experience of physical and psychological well-being. Good health and poor health do not occur as a dichotomy, but as a continuum. The absence of disease or disability is neither sufficient nor necessary to produce health.” (Card, Citation2017) Health status operates as a complex adaptive system and influences the biopsychosociotechnical context.
Biopsychosociotechnical context and health status are open systems whose elements interact at multiple levels. The biopsychosociotechnical determinants of health are not arranged in a single nested system (as in the biopsychosocial model), but rather as interdependent and interlocking parts of a broader “system of systems.”
In addition to its scope under the biopsychosocial model (ranging from families to nation-states), the social system in the biopsychosociotechnical model also encompasses a sociotechnical systems perspective, focusing on how stakeholders work together to improve outcomes and social influences on that work (e.g., laws, norms, standard of practice, health and social care funding, etc.).
The technological system is defined broadly to include all designed artifacts. It includes computer hardware, software, and networks, but also the built environment, assistive technology, furniture, forms, pill bottles, modes of transportation, medical equipment, etc.
Health and needs assessment should be informed by health status, patient/community goals, and the biopsychosociotechnical context.
Interventions to improve health operate by modifying the biopsychosociotechnical context. In addition to direct impact (e.g., through treatment or prevention), interventions may also include modifications to the biopsychosociotechnical context aimed at enabling adherence/sustainment (e.g., improving the usability/desirability of lifestyle changes or technologies, reducing negative side-effects, enhancing positive side-effects, enabling integration with clinical workflows, etc.).
Health improvement interventions should be designed through a participatory process wherever practicable, with the nature and level of participation driven by stakeholder needs.
Problem exploration and intervention design should pragmatically account for relevant biopsychosociotechnical determinants, with the depth of analysis determined by stakeholder needs.
Efforts to improve the biopsychosociotechnical context should aim to achieve joint optimization (or, more accurately, joint satisficing) of the biological, psychological, social, and technological determinants of health, with the goal of establishing a new “quasi-stationary equilibrium” that improves health trajectories.
Many health improvement interventions will require implementation, sustainment, and evaluation. These functions should be included as key components of intervention design, (Card et al., Citation2014) and are dynamically influenced by the biopsychosociotechnical context.
Complex adaptive systems may not behave predictably in response to interventions. In addition, neither the biopsychosociotechnical context nor health status are static, even in the absence of purposeful intervention. Longitudinal health improvement efforts may therefore require an iterative process of guided co-evolution between interventions and the biopsychosociotechnical context. (Well-resourced initiatives may also benefit from various forms of systems modeling and simulation to improve predictability (Brailsford et al., Citation2021; R. M. R. M. Wood et al., Citation2021; Richard M. R. M. Wood, Citation2020; Yaylali et al., Citation2016)).
17. Discussion
17.1. Contributions of the biopsychosociotechnical model
The biopsychosociotechnical model extends the biopsychosocial model in several important ways.
17.2. A lens for understanding context and complexity
This model depicts the biopsychosociotechnical context as a complex adaptive system of systems in which the biological, psychological, social, and technological determinants of health exert influence on one another and on health status as an outcome. This owes more to a pragmatic reinterpretation of Lewinian field theory than to Engel's single nested system.
In contrast with the exhaustive approach promoted by both Lewin and Engel, however, the biopsychosociotechnical model focuses on pragmatically identifying and addressing relevant biopsychosociotechnical determinants, with the depth of analysis determined by stakeholder needs. This reflects both the limited time and resources of health intervention designers (whether frontline clinicians or public health authorities) and calls for parsimony in the literature on complex adaptive systems of systems (Ames et al., Citation2011).
For instance, depicts a hypothetical biopsychosociotechnical analysis as part of the design process for a public health program to address critically low COVID-19 immunisation rates among an immigrant community in a large American city. The specific influences were identified through structured brainstorming using the components of the BPST context (biological, psychological, social, and technological determinants) as prompts. Interconnections between biopsychosociotechnical determinants are mapped out to facilitate joint optimization of correlated systems. Depending on the level of funding, time, and expertise available, this might serve as a starting point for system dynamics modeling or other more sophisticated analysis, or it might be the final product of the systems mapping process.
Figure 4. Hypothetical biopsychosociotechnical analysis of under-immunisation in an immigrant community.
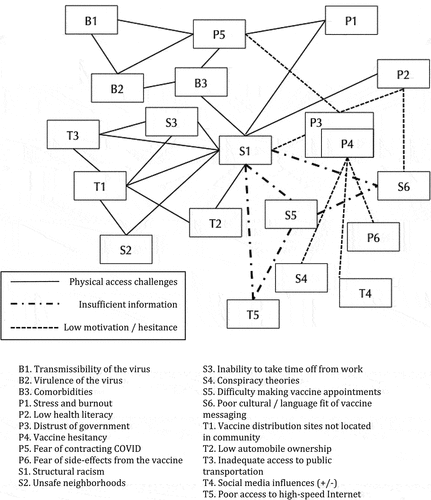
Note that the individual influences are labeled according to the prompt that elicited each as a response; many of them could be labeled with more than one BPST category. For instance, T5 (poor access to high-speed Internet) is as much a social issue of access as it is a technological issue of high-speed Internet. The relevant issue health and needs assessment is successful elicitation and analysis of these influences, not “correct” categorization.
The use of the biopsychosociotechnical model in routine clinical consultation is unlikely to warrant such a detailed systems analysis. For a 10–15-minute appointment to help a patient with obstructive sleep apnea choose his first continuous positive air pressure (CPAP) interface, a clinician might use an approach more like the hypothetical scenario presented in . This is a more heuristic use of the model with fewer details and without explicit mapping of interconnections between the biopsychosociotechnical determinants, but still uses these determinants to provide structure for the health and needs assessment.
Table 3. Potential biopsychosociotechnical considerations for selecting a CPAP interface.
18. A process for participatory systems improvement
By providing a process model for participatory systems improvement, the biopsychosociotechnical model addresses one of the most important critiques of the biopsychosocial approach–that it is difficult to implement because its static structure in fact offers no process to be implemented. Structurally, as shown in , the biopsychosociotechnical model supports a process of participatory systems improvement in two key ways. First, it disentangles the biopsychosocial model's muddling of cause (the determinants of health) and effect (health status), enabling more effective problem exploration and problem solving.
Second, the biopsychosociotechnical model goes further than both the biopsychosocial model and many sociotechnical systems models (cf. (Davis et al., Citation2014; P. E. Waterson & Jenkins, Citation2010)) in explicitly mapping out an intervention design and management process for improving outcomes. In addition to differentiating between cause and effect, this is accomplished by “showing the work” in five key components of the model, rather than glossing over the processes that take place between baseline evaluation of health status and post-intervention outcomes.
First, and perhaps most importantly, the model differentiates between health status and health and needs assessment. This clarifies that there is a problem framing step, in which the goals of a patient or community are considered alongside information about health status and the biopsychosociotechnical context to actively construct the problem(s) to be solved. The problem frame and proposed solutions may co-evolve (Crilly, Citation2021a, Citation2021b) as attempts to change the system drive new learning:
“In order to formulate a design problem to be solved, the designer must frame a problematic design situation: set its boundaries, select particular things and relations for attention, and impose on the situation a coherence that guides subsequent moves. Moreover, the work of framing is seldom done in one burst at the beginning of a design process. Designing triggers awareness of new criteria for design: problem solving triggers problem setting.” (Schön, Citation1984) p.182
Figure 5. Five key components of the BPST model that “show the work” of participatory systems improvement for health.
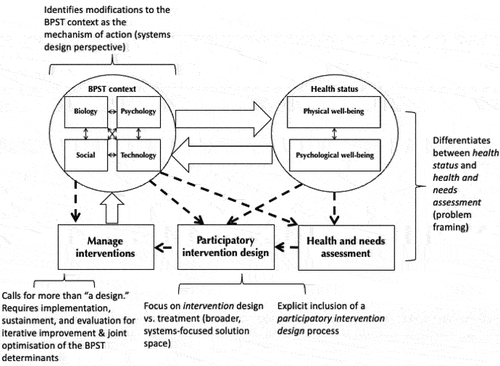
Second, echoing Lewin's B= f(p,e), the biopsychosociotechnical model proposes that the health of an individual or community is an emergent property of the complex adaptive system of systems that makes up the BPST context. Thus, health status can only be changed indirectly–through changes to the BPST context. This imposes a systems perspective, which is further reinforced by the third component: A focus on interventions as opposed to treatments. Treatments are typically limited to addressing pathologies or etiologies of disease; interventions can also aim to modify the biopsychosociotechnical context in other beneficial ways, e.g., to make treatments/prevention efforts easier or more successful, to make adherence more desirable, to reduce negative side effects for third parties, enable integration with clinical workflows, etc.
The fourth component is the explicit inclusion of a participatory intervention design process. This signifies that solutions must be constructed; they may not be ready-made and simply awaiting “selection”. Even straightforward, non-longitudinal treatments such as childhood immunization may require broader interventions if, for instance, a child's parents are vaccine-hesitant. For patients with complex chronic conditions, or communities facing chronic poverty and discrimination, this focus on design as a process becomes even more important. The model's call for participatory design supports the systems awareness and goal-orientation required for successfully improving complex adaptive systems.
Finally, by including intervention management alongside intervention design, the model clarifies that simply designing a (potentially) effective intervention is not enough. To purposefully rebalance the biopsychosociotechnical context, interventions must be implemented, sustained, and evaluated (Card et al., Citation2014). Especially for longitudinal interventions, participatory and dynamic approaches are required for ongoing joint optimization and sustained success (Chambers et al., Citation2013; Papoutsi et al., Citation2020). This also implies a goal of designing for intervention management in the participatory intervention design process, similar to the total product lifecycle model (Wynn & Clarkson, Citation2004) .
19. Expanding the scope to include technology
In contrast with the biopsychosocial model, which excludes the artificial world, the biopsychosociotechnical model includes technology as part of the biopsychosociotechnical context. In addition to the manifest benefits of simply not ignoring such a pervasive influence on health and care, this also enables the integration of approaches from sociotechnical systems theory. Taken together, these advances support better, more systems-focused health and needs assessment. They also support joint optimization in the design and management of health improvement interventions.
The inclusion of technology and sociotechnical systems perspectives also makes the biopsychosociotechnical model more relevant to adjacent fields of research and practice, such as the built environment; occupational burnout and wellbeing; digital health/healthcare technology design; digital health ethics; healthcare quality & safety (and disparities in quality & safety); dissemination and implementation science; context and complexity studies; and policy development, all of which address the interactions between technology and health.
20. A practical theory
Lewin famously said “ … there is nothing so practical as a good theory”, (quoted in (Burnes, Citation2004)). The biopsychosocial model, though insightful, has underperformed for two separate reasons: First, it does not make for very “good” theory, in that it is under-developed and provides no process for implementation. The more comprehensive and action-oriented approach of the biopsychosociotechnical model is intended to address this.
Second, however, the biopsychosocial model was introduced as a revolutionary scientific model. Many critiques focus on Engel's failure to deliver on that promise, distracting from the model's real (if under-realized) contributions to practice (B. L. Wood, Citation2012).
The biopsychosociotechnical model, in contrast, is primarily intended as a practice model and mid-range theory to inform the design and management of health improvement interventions. It may also serve as a useful lens for advancing fields that underlie or overlay healthcare and public health practice, but its fundamental purpose is to improve that practice. Implementation of the biopsychosociotechnical model should therefore be pragmatic, not dogmatic, and proportionate to the potential benefit. For instance, a person with complex chronic comorbidities would likely benefit from a greater investment in biopsychosociotechnical history-taking/participatory intervention design than an otherwise healthy child with a cold.
In considering the biopsychosociotechnical context, the focus should be on gathering relevant information to support decision-making, not drawing up an exhaustive topography of all biopsychosociotechnical influences (which would rarely be possible, in any case (Francisco Borrell-Carrió et al., Citation2015)). Hardwiring the biopsychosociotechnical model into practice through tools such as structured patient interviewing methods (Smith et al., Citation2013), shared decision-making tools (Gonzales & Riek, Citation2013), data models (Green & Klinkman, Citation2015; Ziegelstein, Citation2018), or program design tools (e.g., checklists, logic models, etc. (Petersen, et al., Citation2013; Card, Citation2013; CDC, Citationn.d.; Goeschel et al., Citation2012)) may help to enable this.
It is therefore important that relevant biopsychosociotechnical determinants be considered and addressed, but far less crucial that they be categorized “correctly;” the purpose of the biopsychosociotechnical model is to improve health intervention design and management, not to serve as an abstract taxonomy.
Finally, the human-centered view of health and healthcare envisioned in the biopsychosocial model remains under threat from re-ascendant biomedical reductionism. This is reflected in the dominance of the genetics and other biomarkers as the primary determinants of health considered in the emerging field of personalized medicine/personalized health. The biopsychosociotechnical model provides a practical approach to support the implementation of a broader scope in which personalized health addresses all the determinants of health. (Hekler et al., Citation2020; Ziegelstein, Citation2017)
21. Conclusion
The biopsychosociotechnical model extends the biopsychosocial model by integrating it with sociotechnical systems theory, addressing several key critiques of Engel's approach. It differentiates between health status and the determinants of health, provides an action-oriented change model for health improvement, accounts for and contextualizes the role of technology, and supports the management of context and complexity. Perhaps as important as any of these, it makes the biopsychosocial model more pragmatic and implementable, hopefully helping to move its person-centered insights into action.
Ethical approval
No ethical approval was required for this work, because no human subjects research was involved.
Acknowledgments
Many thanks to Dr. Kyung (Kay) Rhee for helpful comments on an earlier version of this manuscript.
Disclosure statement
No potential conflict of interest was reported by the author(s).
Additional information
Funding
References
- Trist, E., & Murray, H. Eds. (1993). The Social Engagement of Social Science, Vol. II: The Socio-Technical Perspective. Assistant Editor: Beulah Trist. University of Pennsylvania Press.
- Ahmadvand, A., Gatchel, R., Brownstein, J., & Nissen, L. (2018). The biopsychosocial-digital approach to health and disease: Call for a paradigm expansion. Journal of Medical Internet Research, 20(5), 1–7. doi:10.2196/jmir.9732
- Álvarez, A. S., Pagani, M., & Meucci, P. (2012). The Clinical Application of the Biopsychosocial Model in Mental Health. American Journal of Physical Medicine & Rehabilitation, 91(13), S173–S180. doi:10.1097/PHM.0b013e31823d54be
- American Association of Colleges of Nursing. (2011). The Essentials of Master’s Education in Nursing.
- Ames, A. L., Glass, R. J., Brown, T. J., Linebarger, J. M., Beyeler, W. E., Finley, P. D., & Moore, T. W. (2011). Complex Adaptive System of Systems (CASoS) Engineering Framework Version 1.0, SAND Report. SAND 2011-8793 (Sandia National Lab) (Issue November).
- Anderson, J. E. (2015). Complex interventions and their implications for systematic reviews: Commentary on Petticrew. International Journal of Nursing Studies, 52(7), 1209–1210. doi:10.1016/j.ijnurstu.2015.01.003
- Asan, O., & Montague, E. (2012). Physician interactions with electronic health records in primary care. Health Systems, 1(2), 96–103. doi:10.1057/hs.2012.11
- Astorga, M., Mitjans, A., Riek, L. D., & Favela, J. (2021). Design of a Social Robot to Understand Caregivers’ Perception of Disruptive Eating Behaviors by People with Dementia Research Through Design Approaches in Human-Robot Interaction 2021, March 8th 1–3 Accessed 18 January 2022 https://rtdxhri.com/papers/RtDxHRI2021_Astorga_et_al.pdf Virtual. .
- Australian and New Zealand College of Anaesthetists. (2010). National Pain Strategy. Pain Management for all Australians (Australia). www.painsummit.org.au/strategy/Strategy-NPS.pdf/view
- Banh, S., Zheng, E., Kubota, A., & Riek, L. D. (2021). A robot-based gait training system for post-stroke rehabilitation. ACM/IEEE International Conference on Human-Robot Interaction 2021, March (ACM) Virtual, 452–456. 10.1145/3434074.3447212
- Bayramzadeh, S. (2017). An Assessment of Levels of Safety in Psychiatric Units. Health Environments Research and Design Journal, 10(2), 66–80. doi:10.1177/1937586716656002
- Bennett, J. M., Rohleder, N., & Sturmberg, J. P. (2018). Biopsychosocial approach to understanding resilience: Stress habituation and where to intervene. Journal of Evaluation in Clinical Practice, 24(6), 1339–1346. doi:10.1111/jep.13052
- Bircher, J., & Kuruvilla, S. (2014). Defining health by addressing individual, social, and environmental determinants: New opportunities for health care and public health. Journal of Public Health Policy, 35(3), 363–386. doi:10.1057/jphp.2014.19
- Black, N., Burke, L., Forrest, C. B., Ravens Sieberer, U. H., Ahmed, S., Valderas, J. M., Bartlett, S. J., & Alonso, J. (2015). Patient-reported outcomes: Pathways to better health, better services, and better societies. Quality of Life Research, 25(5), 1103–1112. doi:10.1007/s11136-015-1168-3
- Borrell-Carrió, F., Estany, A., Platt, F. W., & MoralesHidalgo, V. (2015). Doctors as a knowledge and intelligence building group: Pragmatic principles underlying decision-making processes. Journal of Epidemiology and Community Health, 69(4), 303–305. doi:10.1136/jech-2014-203868
- Borrell-Carrió, F., Suchman, A. L., & Epstein, R. M. (2004). The Biopsychosocial Model 25 Years Later. Annals Of Family Medicine, 2(6), 576–582. doi:10.1370/afm.245
- Boullier, M., & Blair, M. (2018). Adverse childhood experiences. Paediatrics and Child Health (United Kingdom), 28(3), 132–137. doi:10.1016/j.paed.2017.12.008
- Brailsford, S., Bayer, S., Connell, C., George, A., Klein, J., & Lacey, P. (2021). Embedding OR modelling as decision support in health capacity planning: Insights from an evaluation. Health Systems, 1–14. doi:10.1080/20476965.2021.1983476
- Braithwaite, J., Ellis, L. A., Churruca, K., Long, J. C., Hibbert, P., & Clay-williams, R. (2021). Complexity Science as a Frame for Understanding the Management and Delivery of High Quality and Safer Care. In L. Donaldson, W. Ricciardi, S. Sheridan, and R. Tartaglia (Eds.), Textbook of Patient Safety and Clinical Risk Management. pp.375-391.
- Braithwaite, J., Runciman, W. B., & Merry, A. F. (2009). Towards safer, better healthcare: Harnessing the natural properties of complex sociotechnical systems. Quality and Safety in Health Care, 18(1), 37–41. doi:10.1136/qshc.2007.023317
- Brodsky, N., Ames, A., Glass, R., Brown, T., Finley, P., Moore, T., Linebarger, J., Zagonel, A., & Maffit, S. (2011). Application of complex adaptive systems of systems engineering to tobacco products, SAND2011-3249C. (Albuquerque, NM: Sandia National Lab).
- Burke, N. J., Hellman, J. L., Scott, B. G., Weems, C. F., & Carrion, V. G. (2011). The impact of adverse childhood experiences on an urban pediatric population. Child Abuse and Neglect, 35(6), 408–413. doi:10.1016/j.chiabu.2011.02.006
- Burnes, B. (2004). Kurt Lewin and the Planned Approach to Change: A Re-appraisal. Journal of Management Studies, 41(6), 977–1002. doi:10.1111/j.1467-6486.2004.00463.x
- Burnes, B., & Cooke, B. (2013). Kurt Lewin’s field theory: A review and re-evaluation. International Journal of Management Reviews, 15(4), 408–425. doi:10.1111/j.1467-6486.2004.00463.x.
- Carayon, P. Human factors of complex sociotechnical systems. (2006). Applied Ergonomics, 37(4), 525–535. SPEC. ISS. 10.1016/j.apergo.2006.04.011
- Carayon, P., Du, S., Brown, R., Cartmill, R., Johnson, M., & Wetterneck, T. (2017). EHR-related medication errors in two ICUs. Journal of Healthcare Risk Management, 36(3), 6–15. doi:10.1002/jhrm.21259
- Carayon, P., Schoofs Hundt, A., Karsh, B.-T., Gurses, A. P., Alvarado, C. J., Smith, M., Flatley Brennan, P., Shoofs Hundt, A., Karsh, B.-T., Gurses, A. P., Alvarado, C. J., Smith, M., & Brennan, P. F. (2006). Work system design for patient safety: The SEIPS model. Quality and Safety in Health Care, 15(Suppl. 1), i50–i58. doi:10.1136/qshc.2005.015842
- Carayon, P., Xie, A., & Kianfar, S. (2014). Human factors and ergonomics as a Patient safety practice. BMJ Quality and Safety, 23(3), 196–205. doi:10.1136/bmjqs-2013-001812
- Card, A. J. (2013). A new tool for hazard analysis and force field analysis: The Lovebug Diagram. Clinical Risk, 19(4–5), 87–92. doi:10.1177/1356262213510855
- Card, A. J. (2017). Moving Beyond the WHO Definition of Health: A New Perspective for an Aging World and the Emerging Era of Value-Based Care. World Medical and Health Policy, 9(1), 127–137. doi:10.1002/wmh3.221
- Card, A. J., & Klein, V. R. (2016). A New Frontier in Healthcare Risk Management: Working to Reduce Avoidable Patient Suffering. Journal of Healthcare Risk Management, 35(3), 31–37. doi:10.1002/jhrm.21207
- Card, A. J., & Riek, L. D. (2009). First Do No Harm : Providing safe and effective chronic disease management during a flu pandemic. Workshop on Designing With Care at the 3rd International Conference on Pervasive Computing Technologies for Healthcare (Pervasive Health 09), 2009, March 31. https://www.researchgate.net/publication/350640818_First_Do_No_Harm_Providing_safe_and_effective_chronic_disease_management_during_a_flu_pandemic_First_do_no_harm_Providing_safe_and_effective_chronic_disease_management_during_a_flu_pandemic. London, UK.
- Card, A. J., Ward, J. R., & Clarkson, P. J. (2014). Rebalancing Risk Management -Part 1: The Process for Active Risk Control (PARC). Journal of Healthcare Risk Management, 34(2), 21–30. doi:10.1002/jhrm.21155
- CDC. (n.d.). Health Planning Tools. Retrieved February 15, 2021, from https://www.cdc.gov/healthyplaces/health_planning_tools.htm
- Chambers, D. A., Glasgow, R. E., & Stange, K. C. (2013). The dynamic sustainability framework: Addressing the paradox of sustainment amid ongoing change. Implementation Science, 8(1), 1–11. doi:10.1186/1748-5908-8-117
- Choi, J. S., Betz, J., Li, L., Blake, C. R., Sung, Y. K., Contrera, K. J., & Lin, F. R. (2016). Association of Using Hearing Aids or Cochlear Implants With Changes in Depressive Symptoms in Older Adults. JAMA Otolaryngology–Head & Neck Surgery, 142(7), 652. doi:10.1001/jamaoto.2016.0700
- Crilly, N. (2021a). The Evolution of “Co-evolution” (Part I): Problem Solving, Problem Finding, and Their Interaction in Design and Other Creative Practices. She Ji: The Journal of Design, Economics, and Innovation, 7(3), 309–332. doi:10.1016/j.sheji.2021.07.003
- Crilly, N. (2021b). The Evolution of “Co-evolution” (Part II): The Biological Analogy, Different Kinds of Co-evolution, and Proposals for Conceptual Expansion. She Ji: The Journal of Design, Economics, and Innovation, 7(3), 333–355. doi:10.1016/j.sheji.2021.07.004
- Cross, N. (1982). Designerly ways of knowing. Design Studies, 3(4), 221–227. doi:10.1016/0142-694X(82)90040-0
- Dambha-Miller, H., Feldman, A. L., Kinmonth, A. L., & Griffin, S. J. (2019). Association between primary care practitioner empathy and risk of cardiovascular events and all-cause mortality among patients with type 2 diabetes: A population- based prospective cohort study. Annals of Family Medicine, 17(4), 311–318. doi:10.1370/afm.2421
- Davidoff, F., Dixon-Woods, M., Leviton, L., & Michie, S. (2015). Demystifying theory and its use in improvement. BMJ Quality & Safety, 24(3), 228–238. doi:10.1136/bmjqs-2014-003627
- Davis, M. C., Challenger, R., Jayewardene, D. N. W., & Clegg, C. W. (2014). Advancing socio-technical systems thinking: A call for bravery. Applied Ergonomics, 45(2 Part A), 171–180. doi:10.1016/j.apergo.2013.02.009
- Dube, S. R., Anda, R. F., Felitti, V. J., Edwards, V. J., & Croft, J. B. (2002). Adverse childhood experiences and personal alcohol abuse as an adult. Addictive Behaviors, 27(5), 713–725. doi:10.1016/S0306-4603(01)00204-0
- Emery, F. E. (1971). Democratization of the work place: A historical review of studies. International Studies of Management & Organization, 1(2), 181–201. doi:10.1080/00208825.1971.11656089
- Engel, G. L. (1960). A Unified Concept of Health and Disease. Perspectives in Biology and Medicine, 3(5), 459–485. doi:10.1353/pbm.1960.0020
- Engel, G. L. (1977). The need for a new medical model. Science, 196(4286), 129–136. doi:10.1126/science.847460
- Engel, G. L. (1978). The biopsychosocial model and the education of health professionals. Annals New York Academy of Sciences, 310(1), 169–181. doi:10.1111/j.1749-6632.1978.tb22070.x
- Engel, G. L. (1981). The clinical application of the biopsychosocial model. The Journal of Medicine and Philosophy, 6(2), 101–123. doi:10.1093/jmp/6.2.101
- Engel, G. L. (1981). The clinical application of the biopsychosocial model. The Journal of Medicine and Philosophy, 6, 101–123.
- Engel, G. L. (1992). How much longer must medicine’s science be bound by a seventeenth century world view? Psychotherapy and Psychosomatics, 57(1–2), 3–16. doi:10.1159/000288568
- Epstein, R. M., & Borrell-Carrió, F. (2005). The biopsychosocial model: Exploring six impossible things. Families, Systems and Health, 23(4), 426–431. doi:10.1037/1091-7527.23.4.426
- Farre, A., & Rapley, T. (2017). The New Old (and Old New) Medical Model: Four Decades Navigating the Biomedical and Psychosocial Understandings of Health and Illness. Healthcare, 5(4), 88. doi:10.3390/healthcare5040088
- Felitti, V. J. (2009). Adverse Childhood Experiences and Adult Health. Academic Pediatrics, 9(3), 131–132. doi:10.1016/j.acap.2009.03.001
- Felitti, V. J., Anda, R. F., Nordenberg, D., Williamson, D. F., Spitz, A. M., Edwards, V., Koss, M. P., & Marks, J. S. (1998). Relationship of childhood abuse and household dysfunction to many of the leading causes of death in adults: The adverse childhood experiences (ACE) study. American Journal of Preventive Medicine, 14(4), 245–258. doi:10.1016/S0749-3797(98)00017-8
- Fortin, A. H. V., Dwamena, F. C., Frankel, R. M., Lepisto, B. L., & Smith, R. C. (2019). Smith’s Patient-Centered Interviewing (4th ed.). McGraw-Hill Education.
- Frank, C., Kobesova, A., & Kolar, P. (2013). Dynamic neuromuscular stabilization & sports rehabilitation. International Journal of Sports Physical Therapy, 8(1), 62–73. http://search.ebscohost.com/login.aspx?direct=true&db=rzh&AN=104285501&site=ehost-live
- Frankel, R. M., & Quill, T. (2005). Integrating biopsychosocial and relationship-centered care into mainstream medical practice: A challenge that continues to produce positive results. Families, Systems and Health, 23(4), 413–421. doi:10.1037/1091-7527.23.4.413
- Freudenreich, O., Kontos, N., & Querques, J. (2010). The Muddles of Medicine: A Practical, Clinical Addendum to the Biopsychosocial Model. Psychosomatics, 51(5), 365–369. doi:10.1016/S0033-3182(10)70717-9
- Gaskin, G. L., Longhurst, C. A., Slayton, R., & Das, A. K. (2011). Sociotechnical Challenges of Developing an Interoperable Personal Health Record. Applied Clinical Informatics, 2(4), 406–419. doi:10.4338/ACI-2011-06-RA-0035
- Glass, R. J., Ames, A. L., Stubblefield, W. A., Conrad, S. H., Maffitt, S. L., Malczynski, L. A., Wilson, D. G., Carlson, J. J., Backus, G. A., Ehlen, M. A., Vanderveen, K. B., & Engi, D. (2008). A Roadmap for the Complex Adaptive Systems of Systems CASoS) Engineering Initiative. In Sandia National Laboratories Report.
- Goeschel, C. A., Weiss, W. M., & Pronovost, P. J. (2012). Using a logic model to design and evaluate quality and patient safety improvement programs. International Journal for Quality in Health Care, 24(4), 330–337. doi:10.1093/intqhc/mzs029
- Gonzales, M., & Riek, L. (2012). A Shared Interface to Improve Oncologist-Patient Communication. Proceedings of the 6th International Conference on Pervasive Computing Technologies for Healthcare 2012, May 21-24. San Diego, CA:IEEE. doi:10.4108/icst.pervasivehealth.2012.248711.
- Gonzales, M., & Riek, L. (2013). Co-designing Patient-centered Health Communication Tools for Cancer Care. Proceedings of the ICTs for Improving Patients Rehabilitation Research Techniques 2013, May 5-8 Venice: IEEE. doi:10.4108/icst.pervasivehealth.2013.252109.
- Graber, M. L., Siegal, D., Riah, H., Johnston, D., & Kenyon, K. (2019). Electronic Health Record-Related Events in Medical Malpractice Claims. Journal of Patient Safety, 15(2), 77–85. doi:10.1097/PTS.0000000000000240
- Green, L. A., & Klinkman, M. (2015). Perspectives in primary care: The foundational and urgent importance of a shared primary care data model. Annals of Family Medicine, 13(4), 303–311. doi:10.1370/afm.1817
- Greenhalgh, T., Jackson, C., Shaw, S., & Janamina, T. (2016). Achieving Research Impact Through Co-creation in Community-Based Health Services: Literature Review and Case Study. The Millbank Quarterly, 94(2), 392–429. doi:10.1111/1468-0009.12197
- Greenhalgh, T., & Papoutsi, C. (2018). Studying complexity in health services research: Desperately seeking an overdue paradigm shift. BMC Medicine, 16(95), 1–6. doi:10.1186/s12916-018-1089-4
- Guan, C., Bouzida, A., & Oncy-Avila, R. M., Moharana, Sanika, Riek, Laurel D. (2021). Taking an (embodied) cue from community health: Designing dementia caregiver support technology to advance health equity. Conference on Human Factors in Computing Systems - Proceedings 2021, May 8-13 (ACM)Article No.: 655 Virtual. 10.1145/3411764.3445559
- Glass, R. J., Ames, A. L., Stubblefield, W. A., Conrad, S. H., Maffitt, S. L., Malczynski, L. A., Wilson, D. G., Carlson, J. J., Backus, G. A., Ehlen, M. A., Vanderveen, K. B., & Engi, D. (2008). A Roadmap for the Complex Adaptive Systems of Systems CASoS) Engineering Initiative. In Sandia National Laboratories Report.
- Haslam, S. A., Haslam, C., Jetten, J., Cruwys, T., & Bentley, S. (2019). Group life shapes the psychology and biology of health: The case for a sociopsychobio model. Social and Personality Psychology Compass, 13(8): e12490. doi:10.1111/spc3.12490
- Hawe, P., Shiell, A., & Riley, T. (2009). Theorising interventions as events in systems. American Journal of Community Psychology, 43(3–4), 267–276. doi:10.1007/s10464-009-9229-9
- Hekler, E., Tiro, J. A., Hunter, C. M., & Nebeker, C. (2020). Precision health: The role of the social and behavioral sciences in advancing the vision. Annals of Behavioral Medicine, 54(11), 805–826. doi:10.1093/abm/kaaa018
- Herman, J. L. (2013). Gendered restrooms and minority stress: The public regulation of gender and its impact on transgender people’s lives. Journal of Public Management and Social Policy, 19(1) , 65–80. https://williamsinstitute.law.ucla.edu/wp-content/uploads/Restrooms-Minority-Stress-Jun-2013.pdf.
- Hoeger, B., . M. K., & Sluka, K. A. (2015). The current state of physical therapy pain curricula in the United States: A faculty survey. Journal of Pain, 16(2), 144–152. doi:10.1016/j.jpain.2014.11.001
- Holden, R. J., Carayon, P., Gurses, A. P., Hoonakker, P., Hundt, A. S., Ozok, A. A., & Rivera-Rodriguez, A. J. (2013). SEIPS 2.0: A human factors framework for studying and improving the work of healthcare professionals and patients. Ergonomics, 56(11), 1669–1686. doi:10.1080/00140139.2013.838643
- Holden, R. J., & Mickelson, R. S. (2013). Performance barriers among elderly chronic heart failure patients: An application of patient-engaged human factors and ergonomics. Proceedings of the Human Factors and Ergonomics Society Los Angeles, CA (Sage), 758–762.
- Hom, G. G., & Chous, A. P. (2007). The prospect of pandemic influenza: Why should the optometrist be concerned about a public health problem? Optometry, 78(12), 629–643. doi:10.1016/j.optm.2007.04.099
- Hou, S. I., Charlery, S. A. R., & Roberson, K. (2014). Systematic literature review of Internet interventions across health behaviors. Health Psychology and Behavioral Medicine, 2(1), 455–481. doi:10.1080/21642850.2014.895368
- Hughes, K., Bellis, M. A., Hardcastle, K. A., Sethi, D., Butchart, A., Mikton, C., Jones, L., & Dunne, M. P. (2017). The effect of multiple adverse childhood experiences on health: A systematic review and meta-analysis. The Lancet Public Health, 2(8), e356–e366. doi:10.1016/S2468-2667(17)30118-4
- Huyse, F. J., Lyons, J. S., Stiefel, F., Slaets, J., De Jonge, P., & Latour, C. (2002). Operationalizing the Biopsychosocial Model: The INTERMED. Psychosomatics, 42(1), 5–13. doi:10.1176/appi.psy.42.1.5
- Interagency Pain Research Coordinating Committee. (2016). National Pain Strategy: A Comprehensive Population Health-Level Strategy for Pain (Washington, D.C.: US Department of Health and Human Services, National Institutes of Health).
- Klein, J. H., & Young, T. (2015). Health care: A case of hypercomplexity? Health Systems, 4(2), 104–110. doi:10.1057/hs.2014.21
- Koonin, L. M., Hoots, B., Tsang, C. A., Leroy, Z., Farris, K., Jolly, B., Antall, P., McCabe, B., Zelis, C. B. R., Tong, I., & Harris, A. M. (2020). Trends in the Use of Telehealth During the Emergence of the COVID-19 Pandemic — United States, January–March 2020. MMWR. Morbidity and Mortality Weekly Report, 69(43), 1595–1599. doi:10.15585/mmwr.mm6943a3
- Kruger, C., & Cross, N. (2006). Solution driven versus problem driven design: Strategies and outcomes. Design Studies, 27(5), 527–548. doi:10.1016/j.destud.2006.01.001
- Kubota, A., & Riek, L. D. (2021). Behavior adaptation for robot-assisted neurorehabilitation. In ACM/IEEE International Conference on Human-Robot Interaction Virtual (Vol. 1, Issue 1). Association for Computing Machinery. 10.1145/3434074.3446359
- Kun, L. (2007). The Use of Technology to Transform the Home into a Safe-Haven Bernd Blobel, Lodewijk Bos. Medical and Care Compunetics 4, 127, Studies in Health Technology and Informatics (IOS Press), 18–27 https://ebooks.iospress.nl/volumearticle/10872.
- Lai, F. H. Y., Yan, E. W. H., Yu, K. K. Y., Tsui, W. S., Chan, D. T. H., & Yee, B. K. (2020). The Protective Impact of Telemedicine on Persons With Dementia and Their Caregivers During the COVID-19 Pandemic. American Journal of Geriatric Psychiatry, 28(11), 1175–1184. doi:10.1016/j.jagp.2020.07.019
- Lehman, B. J., David, D. M., & Gruber, J. A. (2017). Rethinking the biopsychosocial model of health: Understanding health as a dynamic system. Social and Personality Psychology Compass, 11(8), 1–17. doi:10.1111/spc3.12328
- Leung, L. (2014). Predicting Internet risks: A longitudinal panel study of gratifications-sought, Internet addiction symptoms, and social media use among children and adolescents. Health Psychology and Behavioral Medicine, 2(1), 424–439. doi:10.1080/21642850.2014.902316
- Lewin, K. (1946). Action research and minority problems: Selected papers on group dynamics. In G. W. Lewin (Ed.), Resolving Social Conflict. Harper & Row. pp. 201–229.
- Lewin, K. (1947a). ‘Frontiers in Group Dynamics’, Parts 1 & 2. Human Relations, 1(1):1: 5-41; 2: 143-153. https://journals.sagepub.com/toc/huma/1/1.
- Lewin, K. (1947b). Frontiers in Group Dynamics: Concept, Method and Reality in Social Science; Social Equilibria and Social Change. Human Relations, 1(1), 5–41. doi:10.1177/001872674700100103
- Lewin, K., & . (1947c). Frontiers in Group Dynamics: II. Channels of Group Life; Social Planning and Action Research. Human Relations, 1(2), 143–153. doi:10.1177/001872674700100201
- Lindau, S. T., Laumann, E. O., Levinson, W., & Waite, L. J. (2007). Synthesis of Scientific Disciplines in Pursuit of Health: The Interactive Biopsychosocial Model. Perspectives in Biology and Medicine, 46(3x), S74–S86. doi:10.1353/pbm.2003.0055
- Lydahl, D. (2019). Standard tools for non-standard care: The values and scripts of a person-centred assessment protocol. Health, 25(1), 103–120. doi:10.1177/1363459319851541
- Malloy, K. M., & Milling, L. S. (2010). The effectiveness of virtual reality distraction for pain reduction: A systematic review. Clinical Psychology Review, 30(8), 1011–1018. doi:10.1016/j.cpr.2010.07.001
- Marberry, S. (2019). Past is Present: 8 Historic Events That Affected Healthcare Facility Design and Construction. https://www.saramarberry.com/more-historic-events-healthcare-facility-design-and-construction/
- Martin, C., & Sturmberg, J. (2009). Complex adaptive chronic care. Journal of Evaluation in Clinical Practice, 15(3), 571–577. doi:10.1111/j.1365-2753.2008.01022.x
- Mastro, K. A., Flynn, L., & Preuster, C. (2014). Patient- and Family-Centered Care A Call to Action for New Knowledge and Innovation. Jona, 44(9), 446–451. doi:10.1097/01.NURSE.0000454963.41514.f6
- May, C. R., Johnson, M., & Finch, T. (2016). Implementation, context and complexity. Implementation Science, 11(1), 1–12. doi:10.1186/s13012-016-0506-3
- McLaren, N. (1998). A critical review of the biopsychosocial model. Australian and New Zealand Journal of Psychiatry, 32(1), 86–96. doi:10.3109/00048679809062712
- Meeks, D. W., Smith, M. W., Taylor, L., Sittig, D. F., Scott, J. M., & Singh, H. (2014). An analysis of electronic health record-related patient safety concerns. Journal of the American Medical Informatics Association, 21(6), 1053–1059. doi:10.1136/amiajnl-2013-002578
- Menon, S., Singh, H., Meyer, A. N. D., Belmont, E., & Sittig, D. F. (2014). Electronic health record–related safety concerns: A cross-sectional survey. Journal of Healthcare Risk Management, 34(1), 14–26. doi:10.1002/jhrm
- Mescouto, K., Olson, R. E., Hodges, P. W., & Setchell, J. (2020). A critical review of the biopsychosocial model of low back pain care: Time for a new approach? Disability and Rehabilitation, 1–15. doi:10.1080/09638288.2020.1851783
- Miaskowski, C., Blyth, F., Nicosia, F., Haan, M., Keefe, F., Smith, A., & Ritchie, C. (2020). A biopsychosocial model of chronic pain for older adults. Pain Medicine (United States), 21(9), 1793–1805. doi:10.1093/pm/pnz329
- Moharana, S., Panduro, A. E., Lee, H. R., & Riek, L. D. (2019). Robots for Joy, Robots for Sorrow: Community Based Robot Design for Dementia Caregivers. Proceedings of the ACM/IEEE International Conference on Human Robot Interaction Daegu, Republic of Korea (ACM).
- Moosaei, M., Das, S. K., Popa, D. O., & Riek, L. D. (2017). Using Facially Expressive Robots to Calibrate Clinical Pain Perception. Proceedings of the 2017 ACM/IEEE International Conference on Human-Robot Interaction Vienna (ACM), 32–41.
- Moosaei, M., Pourebadi, M., & Riek, L. D. (2019). Modeling and Synthesizing Idiopathic Facial Paralysis. IEEE International Conference on Automatic Face and Gesture Recognition Lille, France (IEEE).
- Moseley, G. L., & Butler, D. S. (2015). Fifteen Years of Explaining Pain: The Past, Present, and Future. Journal of Pain, 16(9), 807–813. doi:10.1016/j.jpain.2015.05.005
- Mulder, I., Schikhof, Y., Vastenburg, M., Card, A., Dunn, T., Komninos, A., McGee-Lennon, M., Santcroos, M., Tiotto, G., Van Gils, M., Klooster, V. T., Veys, A, J.-W., & Zarifi Eslami, M. (2009). Designing with care: The future of pervasive healthcare. IEEE Pervasive Computing, 8(4), 85–88. doi:10.1109/MPRV.2009.71
- Neal, J. W., & Neal, Z. P. (2013). Nested or networked? Future directions for ecological systems theory. Social Development, 22(4), 722–737. doi:10.1111/sode.12018
- Norman, C. D. (2009). Health promotion as a systems science and practice. Journal of Evaluation in Clinical Practice, 15(5), 868–872. doi:10.1111/j.1365-2753.2009.01273.x
- Oh, D. L., Jerman, P., Silvério Marques, S., Koita, K., Purewal Boparai, S. K., Burke Harris, N., & Bucci, M. (2018). Systematic review of pediatric health outcomes associated with childhood adversity. BMC Pediatrics, 18(1): 83. doi:10.1186/s12887-018-1037-7
- Papoutsi, C., Wherton, J., Shaw, S., Morrison, C., & Greenhalgh, T. (2020). Putting the social back into sociotechnical: Case studies of co-design in digital health. Journal of the American Medical Informatics Association, 28(October 2020), 284–293. doi:10.1093/jamia/ocaa197
- Pasmore, W., Winby, S., Mohrman, S. A., & Vanasse, R. (2019). Reflections: Sociotechnical Systems Design and Organization Change. Journal of Change Management, 19(2), 67–85. doi:10.1080/14697017.2018.1553761
- Petersen, Dana , Taylor, Erin F. , Peikes, Deborah . (2013). The Logic Model: The Foundation to Implement, Study, and Refine Patient-Centered Medical Home Models AHRQ Publication No. 13-0029-EF. (Rockville, MD: AHRQ).
- Petticrew, M., Anderson, L., Elder, R., Grimshaw, J., Hopkins, D., Hahn, R., Krause, L., Kristjansson, E., Mercer, S., Sipe, T., Tugwell, P., Ueffing, E., Waters, E., & Welch, V. (2015). Complex interventions and their implications for systematic reviews: A pragmatic approach. International Journal of Nursing Studies, 52(7), 1211–1216. doi:10.1016/j.ijnurstu.2015.01.004
- Riek, L. D. (2015). Robotics technology in mental health care. In D. Luxton (Ed.), Artificial Intelligence in Behavioral Health and Mental Health Care (pp. 185–203). Elsevier.
- Riek, L. D. (2017). Healthcare Robotics. Communications of the ACM, 60(11), 68–78. doi:10.1145/3127874
- Riek, L. D., & Robinson, P. (2011). Using robots to help people habituate to visible disabilities. IEEE International Conference on Rehabilitation Robotics Zurich (IEEE).
- Rittel, H., & Webber, M. (1973). Dilemmas in a General Theory of Planning. Policy Sciences, 4(2), 155–169. doi:10.1007/BF01405730
- Rodgers, R. F., Skowron, S., & Chabrol, H. (2012). Disordered Eating and Group Membership Among Members of a Pro-anorexic Online Community. European Eating Disorders Review, 20(1), 9–12. doi:10.1002/erv.1096
- Runciman, W., Baker, G., Michel, P., Dovey, S., Lilford, R., Jensen, N., Flin, R., Weeks, W., Lewalle, P., Larizgoitia, I., & Bates, D. (2010). Tracing the foundations of a conceptual framework for a patient safety ontology. Quality & Safety in Healthcare, 19(6), e56 doi:10.1136/qshc.2009.035147.
- Saddichha, S., Al-Desouki, M., Lamia, A., Linden, I. A., & Krausz, M. (2014). Online interventions for depression and anxiety – A systematic review. Health Psychology and Behavioral Medicine, 2(1), 841–881. doi:10.1080/21642850.2014.945934
- Sallis, J. F., Floyd, M. F., Rodríguez, D. A., & Saelens, B. E. (2012). Role of built environments in physical activity, obesity, and cardiovascular disease. Circulation, 125(5), 729–737. doi:10.1161/CIRCULATIONAHA.110.969022
- Schairer, C. E. (2011). Communities of prosthesis users and possibilities for personal innovation. In A. C. Carey & R. K. Scotch (Eds.), Disability and Community (Research in Social Science and Disability (Vol. 6, pp. 113–133). Emerald Group Publishing Limited.
- Schilling, E. A., Aseltine, R. H., & Gore, S. (2007). Adverse childhood experiences and mental health in young adults: A longitudinal survey. BMC Public Health, 7(1), 1–10. doi:10.1186/1471-2458-7-30
- Schön, D. A. (1984). Problems, frames and perspectives on designing. Design Studies, 5(3), 132–136. doi:10.1016/0142-694X(84)90002-4
- Shanafelt, T. D., Dyrbye, L. N., Sinsky, C., Hasan, O., Satele, D., Sloan, J., & West, C. P. (2016). Relationship Between Clerical Burden and Characteristics of the Electronic Environment With Physician Burnout and Professional Satisfaction. Mayo Clinic Proceedings, 91( 7), 836–848.
- Shepherd, D. A., & Sutcliffe, K. M. (2018). Inductive top-down theorizing: A source of new theories of organization. The Academy of Management Review, 36(2), 361–380. http://www.jstor.org/stable/41318005
- Shepley, M. M., & Pasha, S. (2017). Design Attributes for Improved Mental and Behavioral Health. Design for Mental and Behavioral Health. (Taylor & Francis). 75–97. doi:10.4324/9781315646916
- Singh, H., & Sittig, D. F. (2016). Measuring and improving patient safety through health information technology: The health IT safety framework. BMJ Quality and Safety, 25(4), 226–232. doi:10.1136/bmjqs-2015-004486
- Sittig, D. F., & Singh, H. (2010). A New Socio-technical Model for Studying Health Information Technology in Complex Adaptive Healthcare Systems. Quality & Safety in Health Care, 19(Supp 3), i68–i74. doi:10.1136/qshc.2010.042085
- Smith, R. C. (2002). The Biopsychosocial Revolution. Journal of General Internal Medicine, 17(4), 309–310. doi:10.1046/j.1525-1497.2002.20210.x
- Smith, R. C., Fortin, A. H., Dwamena, F., & Frankel, R. M. (2013). An evidence-based patient-centered method makes the biopsychosocial model scientific. Patient Education and Counseling, 91(3), 265–270. doi:10.1016/j.pec.2012.12.010
- Stergiou, G. S., & Bliziotis, I. A. (2011). Home blood pressure monitoring in the diagnosis and treatment of hypertension: A systematic review. American Journal of Hypertension, 24(2), 123–134. doi:10.1038/ajh.2010.194
- Sturmberg, J. P. (2021). Systems Design for Health System Reform. In G. S. Metcalf, K. Kijima, & H. Deguchi (Eds.), Handbook of Systems Sciences (pp. 735–764). Springer. doi:10.1007/978-981-15-0720-5
- Sturmberg, J. P., & Martin, C. M. (2004). Progressing Engel’s biopsychosocial model of health. Ann Fam Med. https://www.annfammed.org/content/2/6/576/tab-e-letters#progressing-engels-biopsychosocial-model-of-health
- Sturmberg, J. P., & Martin, C. M. (2007). The Foundations of Primary Care: Daring to be Different (1st ed.). CRC Press. doi:10.1201/9781315383361
- Sturmberg, J., & Topolski, S. (2014). For every complex problem, there is an answer that is clear, simple and wrong: And other aphorisms about medical statistical fallacies. Journal of Evaluation in Clinical Practice, 20(6), 1017–1025. doi:10.1111/jep.12156
- Subhi, Y., Bube, S. H., Rolskov Bojsen, S., Skou Thomsen, A. S., & Konge, L. (2015). Expert Involvement and Adherence to Medical Evidence in Medical Mobile Phone Apps: A Systematic Review. JMIR MHealth and UHealth, 3(3), e79. doi:10.2196/mhealth.4169
- Talo, S. A., & Rytökoski, U. M. (2016). BPS-ICF model, a tool to measure biopsychosocial functioning and disability within ICF concepts: Theory and practice updated. International Journal of Rehabilitation Research, 39(1), 1–10. doi:10.1097/MRR.0000000000000151
- Taylor, E., Card, A. J., & Piatkowski, M. (2018). Single-Occupancy Patient Rooms: A Systematic Review of the Literature Since 2006. Health Environments Research and Design Journal, 11(1), 85–100. doi:10.1177/1937586718755110
- Taysom, E., & Crilly, N. (2017). Resilience in Sociotechnical Systems: The Perspectives of Multiple Stakeholders. She Ji: The Journal of Design, Economics, and Innovation, 3(3), 165–182. doi:10.1016/j.sheji.2017.10.011 Resilience.
- Tipirneni, R., Vickery, K. D., & Ehlinger, E. P. (2015). Accountable communities for health: Moving from providing accountable care to creating health. Annals of Family Medicine, 13(4), 367–369. doi:10.1370/afm.1813
- Trist, E. L. (1976). Foreword to Autonomy at work: A sociotechnical analysis of participatory management by Gerald I. Susman. Praeger.
- Trist, E. (1981). The evolution of socio-technical systems: a conceptual framework and an action research program. (Ontario Quality of Working Life Centre 400). Occasional paper number 2.
- Trzeciak, S., & Mazzarelli, A. (2019). Compassionomics: The Revolutionary Scientific Evidence That Caring Makes a Difference. Studer Group.
- Venable, J. R. (2006). The Role of Theory and Theorising in Design Science Research. Proceedings of the 1st International Conference on Design Science in Information Systems and Technology (DESRIST) Claremont, CA (CGU), 1–18.
- Virdi, J. (2017). Prevention &Conservation: Historicizing the Stigma of Hearing Loss, 1910-1940. The Journal of Law, Medicine & Ethics, 45(4), 531–544. doi:10.1177/1073110517750587
- Wachter, R. (2015). The Digital Doctor. McGraw-Hill Education.
- Waddell, K., Moat, K., & Lavis, J. (2017). Evidence Brief: Developing a National Pain Strategy for Canada. (McMaster Health Forum). https://macsphere.mcmaster.ca/bitstream/11375/23360/1/pain-strategy-eb.pdf
- Wade, D. T., & Halligan, P. W. (2017). The biopsychosocial model of illness: A model whose time has come. Clinical Rehabilitation, 31(8), 995–1004. doi:10.1177/0269215517709890
- Wade, D. (2015). Rehabilitation - A new approach. Part two: The underlying theories. Clinical Rehabilitation, 29(12), 1145–1154. doi:10.1177/0269215515601175
- Wainwright, T. W., & Low, M. (2020). Why the biopsychosocial model needs to be the underpinning philosophy in rehabilitation pathways for patients recovering from COVID-19. Integrated Healthcare Journal, 2(1), e000043. doi:10.1136/ihj-2020-000043
- Waljee, J. F., Rogers, M. A. M., & Alderman, A. K. (2007). Decision aids and breast cancer: Do they influence choice for surgery and knowledge of treatment options? Journal of Clinical Oncology, 25(9), 1067–1073. doi:10.1200/JCO.2006.08.5472
- Walker, J., Meltsner, M., & Delbanco, T. (2015). US experience with doctors and patients sharing clinical notes. BMJ, 350(February), 1–4. doi:10.1136/bmj.g7785.
- Ward, A. M., Takahashi, O., Stevens, R., & Heneghan, C. (2012). Home measurement of blood pressure and cardiovascular disease: Systematic review and meta-analysis of prospective studies. Journal of Hypertension, 30(3), 449–456. doi:10.1097/HJH.0b013e32834e4aed
- Ward, J., Clarkson, J., Goodman-Deane, J., & Card, A. (2017). Improve. In Systems Safety Assessment (SSA) Toolkit. Cambridge Engineering Design Centre. http://www.ssatoolkit.com/ssatoolkit/improve1overview/.
- Waterson, P. E., & Jenkins, D. P. (2010). Methodological considerations in using ACCIMAPS and the risk management framework to analyse large-scale systemic failures. 5th IET International Conference on System Safety, Manchester. doi: 10.1049/cp.2010.0830
- Waterson, P., Robertson, M. M., Cooke, N. J., Militello, L., Roth, E., & Stanton, N. A. (2015). Defining the methodological challenges and opportunities for an effective science of sociotechnical systems and safety. Ergonomics, 58(4), 565–599. doi:10.1080/00140139.2015.1015622
- Wears, R. L. (2015). Improvement and evaluation. BMJ Quality and Safety, 24(2), 92–94. doi:10.1136/bmjqs-2014-003889
- Weston, W. W. (2005). Patient-Centered Medicine: A Guide to the Biopsychosocial Model. Families, Systems, & Health, 23(4), 387–392. doi:10.1037/1091-7527.23.4.387
- WHO. (2002). Towards a Common Language for Functioning, Disability and Health: ICF WHO/EIP/GPE/CAS/01.3. (Geneva: World Health Organisation). https://www.who.int/classifications/icf/training/icfbeginnersguide.pdf.
- Wieman, T. J., & Wieman, E. A. (2004). A systems approach to error prevention in medicine. Journal of Surgical Oncology, 88(3), 115–121. doi:10.1002/jso.20121
- Williams, J. K., Ph, D., & N, F. A. A. (2005). Toward a Biopsychosocial Model for 21st-Century Genetics. Family Process, 44(1): 3–24. doi:10.1111/j.1545-5300.2005.00039.x.
- Wood, B. L. (2012). Chapter 9. Biopsychosocial. In L. L’Abate (Ed.), Paradigms in Theory Construction (pp. 31–66). Springer.
- Wood, B. L., Lim, J., Miller, B. D., Cheah, P., Zwetsch, T., Ramesh, S., & Simmens, S. (2008). Testing the Biobehavioral Family Model in Pediatric Asthma: Pathways of Effect. Family Process, 47(1), 21–40. doi:10.1111/j.1545-5300.2008.00237.x
- Wood, R. M. (2020). Modelling the impact of COVID-19 on elective waiting times. Journal of Simulation, 1–9. doi:10.1080/17477778.2020.1764876
- Wood, R. M., Pratt, A. C., Murch, B. J., Powell, A. L., Booton, R. D., Thomas, D. G., Twigger, J., Diakou, E., Coleborn, S., Manning, T., Davies, C., & Turner, K. M. (2021). Establishing an SEIR-based framework for local modelling of COVID-19 infections, hospitalisations and deaths. Health Systems, 10(4), 337–347. doi:10.1080/20476965.2021.1973348
- Woodworth, B., Ferrari, F., Zosa, T. E., & Riek, L. D. (2018). Preference Learning in Assistive Robotics: Observational Repeated Inverse Reinforcement Learning. Machine Learning for Healthcare Conference, Palo Alto, CA, 85, (Proceedings of Machine Learning Research), 1–19.
- Wynn, D., & Clarkson, J. (2004). Models of Designing. In J. Clarkson, and C. Eckert (Eds.), Design Process Improvement: A Review of Current Practice, (London: Springer), (pp. 1–18).
- Yaylali, E., Ivy, J. S., Uzsoy, R., Samoff, E., Meyer, A. M., & Maillard, J. M. (2016). Modeling the effect of public health resources and alerting on the dynamics of pertussis spread. Health Systems, 5(2), 81–97. doi:10.1057/hs.2015.6
- Yusif, S., Soar, J., & Hafeez-Baig, A. (2016). Older people, assistive technologies, and the barriers to adoption: A systematic review. International Journal of Medical Informatics, 94, 112–116. doi:10.1016/j.ijmedinf.2016.07.004
- Ziegelstein, R. C. (2017). Personomics: The missing link in the evolution from precision medicine to personalized medicine. Journal of Personalized Medicine, 7(4), 11. doi:10.3390/jpm7040011
- Ziegelstein, R. C. (2018). Perspectives in Primary Care: Knowing the Patient. Annals of Family Medicine, 16(1), 4–5. doi:10.1370/afm.2169