Abstract
Objective
Receptor activator of NF-κβ ligand (RANKL) is crucial for the development of hepatic insulin resistance and poor glucose uptake; therefore, inhibiting RANKL with Denosumab could improve fasting plasma glucose (FPG) and insulin (FPI).
Methods
A systematic review was conducted to evaluate the effects of Denosumab on glycemic parameters. PubMed, SCOPUS, EBSCO, and LILACS databases were searched for studies that investigated the effect of Denosumab on FPG, glycated hemoglobin (HbA1c), FPI, and Homeostatic Model Assessment for Insulin Resistance (HOMA1-IR). The pooled standard difference in means (SDM) and 95% confidence intervals (95%CI) were calculated. The results were stratified into (1) Normal Glucose Tolerance (NGT) and (2) Impaired Glucose Tolerance (IGT).
Results
Six publications (1203 participants) were included. There was a significant association between Denosumab and FPG (SDM = −0.388, 95%CI: −0.705 to −0.070, p = .017) and with HOMA1-IR (SDM = −0.223, 95%CI: −0.388 to −0.058, p = .008), but not for HbA1c and FPI. When stratified by glucose tolerance, the association between Denosumab and FPG, HbA1c, and HOMA1-IR was present for the IGT group. Lastly, Denosumab had a time-dependent effect on HbA1c (slope = −0.037, 95%CI: −0.059 to −0.015, p < .005).
Conclusions
Denosumab significantly improved glycemic parameters. This outcome was more prominent for subjects with compromised glucose tolerance, positing that Denosumab can be used as a treatment to improve glucose metabolism for persons with pre-diabetes and diabetes.
Introduction
Normally, the receptor activator of NF-κB ligand (RANKL) stimulates the fusion of pre-osteoclasts, the attachment of osteoclasts to the bone, and the activation of osteoclasts for bone resorption [Citation1]; however, RANKL participates in other tissues with different functions. For example, in muscles, high levels of RANKL expression generate poor glucose uptake, leading to alterations in muscle metabolism [Citation2]. In the liver, RANKL can induce insulin resistance by promoting inflammation [Citation2,Citation3]. In the pancreas, RANKL produces a hyperglycemic state by decreasing insulin production and augments glucagon production [Citation4], which could lead to β-cell dysfunction [Citation3]. The principal sources of RANKL are macrophages that have infiltrated the bone, pancreas, liver, and muscles [Citation5,Citation6], which is a signal for the inflammatory NF-κB pathway, linking it to impaired glucose tolerance (IGT) [Citation7]. The inflammatory NF-κB pathway could involve red and white blood cells. As shown using the platelet-to-lymphocyte ratio, the neutrophil-to-lymphocyte ratio, and the red cell distribution width, these markers of inflammation are associated with elevated HbA1c in uncontrolled diabetic patients [Citation8–10]. Moreover, there are a few studies demonstrating that metformin, an antidiabetic drug, decreased RANKL activity and its expression in the bone and liver [Citation11,Citation12], although the molecular mechanisms involved are not fully understood. However, Saisho et al. has suggested that an anti-inflammatory effect by inhibiting the NF-κB pathway in macrophages is inferred [Citation13]. In addition, dipeptidilpeptidase-4 (DPP4), a well-known enzyme involved in glucose homeostasis, has been recognized as an osteoclast-derived protein [Citation4]. DPP4, as well as Metformin, represents a crucial link between RANKL/bone remodeling and energy metabolism [Citation4,Citation12]. Due to these reasons, it has been hypothesized that blocking RANKL signaling might improve fasting plasma glucose (FPG) concentrations [Citation3].
In the RANKL pathway, osteoprotegerin (OPG) is an inhibitor of RANKL, which enhances osteoclasts apoptosis [Citation1]. Interestingly, elevated OPG serum levels are correlated with IGT [Citation14] and that could be due to insulin levels decreasing over the years in patients with IGT [Citation15] as well as the similar roles between OPG and insulin in blocking osteoclastogenesis [Citation16]. Based on OPG’s function, Denosumab, a monoclonal antibody for human RANKL [Citation17], could improve glucose entry into the muscle through increasing insulin sensitivity [Citation2]. Furthermore, Denosumab was also proven to decrease DPP4 serum concentrations and increase glucagon-like peptide-1 (GLP-1) in subjects with IGT, demonstrating a crucial role in glucose and insulin metabolism [Citation4]. Typically, Denosumab inhibits the maturation of osteoclasts and their precursors [Citation18], as well as it is the current treatment for osteoporosis and other bone diseases. However, multiple studies, randomized controlled trials, and observational studies have assessed the effect of Denosumab on insulin sensitivity and FPG with contradictory results. In a few studies, the authors demonstrate a beneficial effect, in which Denosumab decreased FPG [Citation4,Citation19]; however, Schwartz et al. demonstrate that Denosumab clearly increased FPG [Citation20]. To address this conflict, a systematic review was conducted, aimed to evaluate the effects of Denosumab on different glycemic parameters.
Materials and methods
Search strategy
PubMed, SCOPUS, EBSCO, and LILACS databases were searched for studies that investigated the effect of Denosumab on glucose metabolism. The following keywords/terms and any of their derivations were used: “Denosumab” as well as other terms related to the treatment; “glucose” and “insulin”. The search was performed without any language restrictions for publications published until 14 October 2020. Afterward, the complied publications references were hand searched.
Inclusion and exclusion criteria
Two authors (B.T.P.S. and R.G.E.R.) determined if each study was to be included. If a disagreement occurred about a publication, a third author (L.M.P.) analyzed the publication in question. Initially, titles and abstracts were examined to determine if the publications were original research, focused on Denosumab treatment, and examined parameters of glucose metabolism [FPG; fasting plasma insulin (FPI); glycated hemoglobin (HbA1c), and insulin resistance using the Homeostatic Model Assessment for Insulin Resistance (HOMA1-IR)]. For inclusion, the studies must have met the following criteria: (1) prospective or case-control study; (2) used Denosumab as a treatment for any pathology (not just limited to osteoporosis); and (3) contained information about metabolic parameters related to glucose metabolism. Any study in which FPG was not reported was excluded. Studies were also excluded if they were: (1) a case study, review, or editorial; (2) information was used in a previous publication; (3) in vitro or animal studies; (4) failed to use Denosumab as the primary treatment; and (5) failed to measure or report parameters related to glucose metabolism or unable to determine the values from the reported data.
Bias analysis and data extraction
Two authors (B.T.P.S. and R.G.E.R.) independently assessed the quality of the studies using the Downs and Black Checklist [Citation21] for randomized controlled and non-controlled trials. The reviewers’ results were compared by an external reviewer (M.E.G.M.) and discrepancies were resolved in a consensus meeting. The following aspects of each study were evaluated: reporting, external validity, internal validity-bias, internal validity-confounding (selection bias), and power. For the analysis, the quality score ranges were given corresponding quality levels as previously reported [Citation22]: excellent (26–28); good (20–25); fair (15–19); and poor (<14) (Supplement Figure 1). The following data was collected from each study: first author's name, year of publication, geographical location, sample size, duration of the intervention, and pre- and post-intervention values for FPG, FPI, HbA1c, and HOMA1-IR. Furthermore, data that was reported as the standard error of the mean or 95% Confidence Intervals (95%CI) were converted back to standard deviation. When FPG levels were reported in mmol/L, a multiplication factor of 18.018 was used to convert to mg/dL. Likewise, when FPI levels were reported in pmol/L, a multiplication factor of 0.144 was used to convert to µIU/mL. Finally, when HbA1c was reported in the International Federation of Clinical Chemistry units (IFCC, mmol/mol), the value was converted to the National Glycohemoglobin Standardization Program (NGSP, %) by multiplying it by 0.0915 then adding 2.152 [Citation23].
Statistical analysis
All analyses were conducted using the Comprehensive MetaAnalysis Version 2 software (CMA, Englewood, NJ, USA). The Standard Difference in Means (SDM) and 95%CIs were calculated for each individual comparison. Heterogeneity was assessed using the chi2-based Q-test and the Inconsistency Index (I2) determined its degree. Depending on the results of the heterogeneity tests, either Fixed Effects (chi2-based Q-test p > .10 and I2 < 50%) or Random Effects (chi2-based Q-test p < .10 and I2 > 50%) was used to calculate the pooled SDM and 95%CI. Due to multiple time points assessed by each study, for the overall pooled effect analysis, the study’s SDM was based on calculating net change from baseline to the longest endpoint, as suggested by the Cochrane Handbook (Section 9.3.4) [Citation24]. However, for the time-dependent analysis, all possible comparisons were used. For CMA, to calculate the pooled SDM, the correlation coefficient for each study had to be known; however, this value was not reported nor could it be calculated. Therefore, for each analysis, the pooled SDM was calculated using a range of increasing correlation coefficients (0.2, 0.4, 0.6, and 0.8). The results were minimally affected by increasing the correlation coefficient (Supplement Table 1); therefore, all data shown are for a correlation coefficient of 0.6. Sensitivity analysis, removing one study and recalculating the pooled SDM and 95%CI, was conducted to verify the stability of the results. Begg's funnel plot, Begg-Mazumdar's test [Citation25], and Egger's linear regression test [Citation26] were used to assess publication bias. To assess the effect of glucose tolerance on Denosumab’s effect on glucose metabolism, the studies were stratified into two groups: (1) normal glucose tolerance (NGT) and (2) IGT, which consisted of persons with Type 2 Diabetes (T2D) and prediabetes (PT2D). p-Values <.05 (two-tailed) were considered statistically significant unless noted otherwise.
Results
Literature search and characteristics of the included studies
After removing all duplicate publications, we examined 374 publications (). Three hundred sixty-seven publications were excluded for not being original research, not focusing on human subjects, subjects did not receive Denosumab, or glucose metabolism parameters were not examined or reported. Afterward, the remaining seven publications were extensively reviewed, where one was removed for not containing sufficient data. In the end, six publications (eight studies) met the inclusion criteria and were included in this meta-analysis, which consisted of 1203 participants.
The majority of the publications were comprised of Caucasian populations: three from Italy [Citation27–29] and one from USA [Citation4]. The remaining publications were from Japan [Citation19] and the multinational FREEDOM study [Citation30]. The characteristics of the studies are summarized in . Participants in most of the studies were only females with only two studies including males. The posology was a subcutaneous injection of Denosumab (60 mg) every 6 months for every study; however, the number of doses given varied between studies. Co-supplementation with either Vitamin D and calcium occurred in five and two publications, respectively. The quality of the studies was assessed using the Downs and Black Checklist, in which all of the studies scored ≥14, suggesting minimal study bias. The baseline characteristics of glucose metabolism parameters and their follow-up data are shown in .
Table 1. Characteristics of the includes studies.
Table 2. Data used for analyses.
Treatment with denosumab improves FPG concentrations in IGT patients
For the overall analysis (1203 participants), the longest time point of each study was taken according to the Cochrane handbook [Citation24]. Using six publications (eight studies), there was significant heterogeneity (I2 = 95.6%, Chi2: p < .005); therefore, the Random Effects model was utilized. When the studies were pooled together, there was a significant association between Denosumab treatment and decreased FPG ( and Supplement Figure 2). To assess the stability of the results, one study was removed and the association was reevaluated. Only when the Napoli 2018 (PT2D patients) and the Napoli 2018 (T2D patients) studies were removed, a loss of the association resulted (SDM = −0.415, 95%CI: −0.943 to 0.113, p = .124 and SDM = −0.427, 95%CI: −0.927 to 0.073, p = .094, respectively, Supplement Figure 2). Publication bias was assessed by examining the funnel plot. The funnel plot demonstrated no significant asymmetry and the shape of the funnel plot also suggested no evidence of publication bias (Supplement Figure 2). No correlation was determined by the Begg-Mazumdar’s test (p = .32) or bias by Egger’s test (p = .43). When the cohort was stratified by glucose tolerance status (NGT vs. IGT), only the IGT group showed a significant effect (). Even though the effect for the IGT group was 4.2-fold more prominent, this was not significant (p = .263).
Table 3. The effect of denosumab treatment on glucose metabolism, stratified by glucose tolerance.
Treatment with denosumab has no effect on FPI concentrations
Using four publications (four studies), which is comprised of only NGT subjects (98 participants), there was no significant heterogeneity (I2 = 16.3%, Chi2: p = .310); therefore, the Fixed Effects model was utilized. When the studies were pooled together, insulin did not show a significant association ( and Supplement Figure 3). Removing one study did not change the association. No publication bias was observed by the Begg-Mazumdar’s test (p = .49) or by Egger’s test (p = .82).
Treatment with denosumab improves HbA1c concentrations in IGT patients
Five publications (five studies) reported HbA1c values. For the overall analysis (211 participants), there was significant heterogeneity (I2 = 63.7%, Chi2: p = .026); therefore, the Random Effects model was utilized. When the studies were pooled together, HbA1c did not show a significant association ( and Supplement Figure 4), and removing one study did not affect the results. No publication bias was determined by the Begg-Mazumdar’s test (p = .32) or by Egger’s test (p = .41). When the cohort was stratified by glucose tolerance status, only the IGT group showed a significant effect (). The IGT group’s effect was 3.2-fold higher than the NGT group and this difference was significant (p = .001).
Treatment with denosumab has no effect on insulin resistance
Five publications (five studies) assessed insulin resistance using HOMA1-IR. For the overall analysis (118 participants), there was no significant heterogeneity (I2 = 39.3%, Chi2: p = .159); therefore, the Fixed Effects model was utilized. When the studies were pooled together, lower HOMA1-IR scores were significantly associated with the Denosumab treatment ( and Supplement Figure 5); however, the association was sensitive to the Abe 2019 study (SDM = −0.160, 95%CI: −0.339 to 0.020, p = .081). No publication bias was determined by the Begg-Mazumdar’s test (p = .62) or by Egger’s test (p = .96). When the cohort was stratified by glucose tolerance status, only the IGT group demonstrated a significant effect (). Even though the IGT group’s effect was 2.6-fold greater than the NGT group, this difference was not significant (p = .078).
Time-dependent effect of denosumab treatment with respect to glucose parameters
To assess a time-dependent effect, all possible study time points were utilized. No time-dependent effect was observed between the Denosumab treatment and FPG or HOMA1-IR (). There was almost a time-dependent association between the Denosumab treatment and FPI (slope = 0.062, 95%CI: −0.007 to 0.131, p = .078, ). However, we did observe a strong association between the Denosumab treatment and HbA1c (slope = −0.037, 95%CI: −0.059 to −0.015, p < .005, ).
Figure 2. Time dependent effect of Denosumab treatment with respect to (A) Fasting plasma Glucose, (B) Fasting Plasma Insulin, (C) HbA1c, and (D) HOMA1-IR. The analysis was made with linear meta-regression using the method of moments by the comprehensive meta-analysis software V2. There was only a strong association between denosumab treatment and HbA1c (slope = −0.037, 95%CI: −0.059 to −0.015, p < .005).
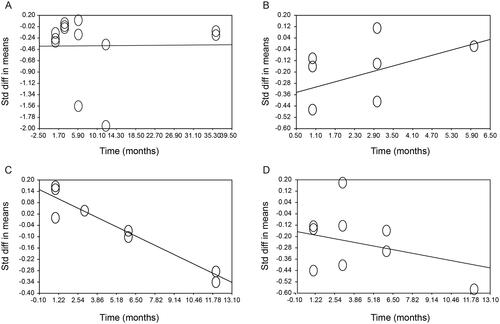
Discussion
It has been hypothesized that inhibiting RANKL can improve hepatic insulin resistance and decrease FPG. Indeed, we determined that inhibiting RANKL with Denosumab did improve glucose metabolism. However, this effect was more prominent in subjects with compromised glucose tolerance.
Even though our results did indicate a significant association between Denosumab and glucose parameters, there was significant variability in the analysis between individual studies, as demonstrated by the presence of heterogeneity. Age affects RANKL and OPG concentrations and this could affect Denosumab’s action. Chung et al. demonstrated that RANKL was positively correlated with aging and OPG was negatively correlated [Citation31]. In our analysis, all patients are at least 45 years old and similar in age. Another source for variability would be gender. Only two studies, the Abe 2019 study, and the Weivoda 2020 study had a mixed population. Healthy men have higher RANKL concentrations than healthy women and the situation is reversed with OPG [Citation32]. Even though we initially wanted an unbiased sample of studies, our literature review resulted in a mix of patients with either osteoporosis or compromised glucose tolerance. For compromised glucose tolerance, depending on the duration of diabetes, secondary comorbidities could be present but were not considered during the analysis. Similarly, for osteoporosis, bone tissue was recently demonstrated to be metabolically active; with proteins that modulate insulin secretion and insulin sensitivity, such as osteocalcin [Citation33]. In osteoporosis, osteocalcin levels are impaired [Citation34] and consequently alter insulin secretion [Citation35]. Lastly, another source of variability could be concomitant treatments that were not adjusted in the final analyses of the studies, such as aromatase inhibitors, Vitamin D, and anti-diabetic medications (ADM). The induction and increased risk of developing IGT after the use of aromatase inhibitors are well-documented but the underlying mechanisms are not fully understood [Citation36]; therefore, the conjunction of Denosumab and aromatase inhibitors is not well-studied and its effects need further evaluation.
Both Vitamin D and, undoubtedly, ADMs have a beneficial effect on glucose homeostasis and insulin sensitivity in patients with IGT [Citation37–39]. For Vitamin D, most of the included studies gave this supplement—only three studies did not indicate if the patients received Vitamin D [Citation4,Citation28]. This could be due to that Vitamin D tends to increase OPG levels [Citation40]. This addition might have resulted in a synergistic effect between Denosumab and Vitamin D. Interestingly, it is required to supplement Denosumab with Vitamin D and calcium, which are also a treatment for osteoporosis, to avoid secondary side effects, such as hypocalcemia [Citation41]. For this reason, we encourage future studies to take this into consideration as, for example, Abe et al., who started their subjects on activated Vitamin D for more than 26 weeks before the first administration of Denosumab and continue taking it during the study so the baseline parameters would not be affected [Citation19].
ADMs influence glucose metabolism parameters [Citation39], and it is known that ADMs’ effect on FPG and FPI, specifically Metformin [Citation42], Sitagliptin [Citation43], and Liraglutide [Citation44], plateaus within three months if the dose is not adjusted or combination therapy is not added [Citation45]. Here, patients were taking these medications before the baseline parameters were obtained, and, according to most of the included studies, these treatments or duration of diabetes does exceed 3 months. We speculate that the therapies did not change during the study period, which would suggest the marked improvement in glucose parameters were due to the Denosumab treatment. Nevertheless, we encourage future studies to take this into consideration and state the type of ADMs used, dose and duration of the treatment, and adjust the analysis, if necessary.
Here, we observe an effect of Denosumab improving glucose metabolism and it appears to be mainly associated with PT2D and T2D. This result was expected. PT2D and T2D results from pancreatic β-cell dysfunction [Citation46] and most AMDs focus on normalizing or stimulating β-cell homeostasis [Citation39]. OPG stimulates replication of adult human β-cells without causing dedifferentiation by modulating the CREB and the GSK3 pathways through binding RANKL [Citation47]. Denosumab’s mechanism involves mimicking a partial functional equivalent of OPG by inhibiting RANKL and likewise inducing a mitogenic effect on pancreatic β-cells. Also, in several in vitro and in vivo studies, a higher expression and concentration of RANKL have been associated with prolonged hyperglycemia [Citation7,Citation48]. Additionally, recent studies have shown that Denosumab decreases DPP4 and increases GLP-1 concentrations in IGT patients [Citation4]. NGT subjects have functioning β-cells and do not have an increased expression or concentrations of RANKL [Citation7]. This could be a reason why they may not benefit from Denosumab as much as the IGT group. This corresponds with the results from our study which demonstrated that the effect of RANKL inhibition on glucose parameters was more pronounced in the IGT group.
Osteonecrosis of the jaw is one of the major adverse effects associated with the use of Denosumab [Citation49], especially in IGT patients, which are reported to have poor oral health [Citation50]. Other adverse effects to Denosumab, independent of IGT, are flatulence, eczema, and cellulitis [Citation51]. Despite this, Denosumab is the most dominant option and cost-effectiveness drug for osteoporosis [Citation52]. With respect to IGT, since the treatment for Denosumab (one dose every six months) can cost between $608 and $1811 USD yearly ($51–151 USD monthly), which is similar to the cost for Metformin [non-generic: $35 USD (500 mg once a day) to $175 USD (850 mg three times a day)], this suggests the potential cost-effectiveness for Denosumab. Therefore, more studies are needed to analyzed if Denosumab should be a treatment option for IGT patients [Citation53].
This study has a few limitations. First, the duration of the studies was not consistent so the results could not be compared using specific time points. As seen in , a time-dependent factor is clear for HbA1c and because many studies did not present results for >3 months, it was not possible to view the extent of this effect. This result should be assessed carefully. Second, most of the studies were comprised of only female patients (two studies included males). Therefore, this result could be valid for only females. Futures studies should focus on males only or perform a gender sub-analysis. Third, due to the fact that the strength of the association is unknown, we varied the correlation coefficient to calculate the pooled SDMs. Increasing the correlation coefficient will affect the pooled SDM. Nevertheless, when we did increase the correlation coefficient (Supplement Table 1), there was no change in the overall results. We presented SDMs with the correlation coefficient of 0.6; however, the true pooled SDMs remain unidentified. Fourth, our cohort of studies included a mix of randomized clinical trials as well as prospective observational studies; therefore, we used the Downs and Black Checklist to assess the risk of bias for each study, which was minimal. A sub-analysis by study type could resolve this issue; however, due to the few studies included here, we believe the analysis could yield misleading conclusions. Furthermore, it should be acknowledged that the main aim for most of the included studies was to treat osteoporosis and not IGT. Fifth, in studies that included persons with T2D and PT2D, the patients did not discontinue using ADMs, which could be a source of bias. Nevertheless, as mentioned above, most ADMs plateaus after three months of treatment and according to most studies, the treatments’ durations did exceed three months. We speculate that this effect should be minimal; however, this should be resolved with a better-designed study and we encourage future studies to take this into consideration. Lastly, we used two different methods to input the data into CMA. With the CMA program, the data can be inputted with many different formats, which will yield the same effect size measure, however, depending on the method used, the standard error can be different. Therefore, one method can give more accurate estimates.
Conclusion
Here, our study indicates that the treatment with Denosumab resulted in a significant reduction in FPG as well as improving other glucose metabolism parameters, such as HOMA1-IR, specifically for subjects with compromised glucose tolerance. This does posit that Denosumab can be used as an adjuvant to improve glucose metabolism when considering the association between glucose and bone metabolism. We encourage future studies to further evaluate the possible advantages of Denosumab, such as cost-effectiveness and adherence, due to its twice a year application, for the treatment of hyperglycemia.
Transparency
Declaration of funding
This work was supported by grants from the Programa para el Desarrollo Profesional Docente (CA-160 FACMED) and the Vicerrectorıa de Investigacion, Benemerita Universidad Autonoma de Puebla, Mexico (GOMM-SAL18-I). The funders had no role in the study’s design, data collection or analysis, decision to publish, or preparation of the manuscript.
Declarations of financial/other relationships
No potential competing interest was reported by the authors. Peer reviewers on this manuscript have no relevant financial or other relationships to disclose.
Authors contributions
B.T.P.S.: conceptualization, methodology, formal analysis, investigation, data curation, writing–original draft, and visualization. R.G.E.R.: methodology, formal analysis, investigation, writing–original draft, and visualization. L.M.P.: conceptualization, methodology, formal analysis, and visualization. E.T.R.: resources, writing–review and editing, and supervision. R.P.F.: validation, writing–review and editing, and funding acquisition. M.E.G.M.: conceptualization, methodology, validation, writing–review and editing, visualization, supervision, project administration, and funding acquisition.
Supplemental Material
Download PDF (2.6 MB)Acknowledgements
The authors would like to express their gratitude to Mtro. Ricardo Villegas Tovar, Coordinator of Scientific Production and International Visibility, BUAP, and Dr. Catalano, for his quick response and clarification of his study.
Data availability statement
All data generated or analyzed during this study are included in this published article and its supplementary information files.
References
- Kostenuik PJ. Osteoprotegerin and RANKL regulate bone resorption, density, geometry and strength. Curr Opin Pharmacol. 2005;5(6):618–625.
- Bonnet N, Bourgoin L, Biver E, et al. RANKL inhibition improves muscle strength and insulin sensitivity and restores bone mass. J Clin Invest. 2019;129(8):3214–3223.
- Wilson C. Diabetes: Blocking RANKL signalling might prevent T2DM. Nat Rev Endocrinol. 2013;9(4):188.
- Weivoda MM, Chew CK, Monroe DG, et al. Identification of osteoclast-osteoblast coupling factors in humans reveals links between bone and energy metabolism. Nat Commun. 2020;11(1):87.
- Esser N, Legrand-Poels S, Piette J, et al. Inflammation as a link between obesity, metabolic syndrome and type 2 diabetes. Diabetes Res Clin Pract. 2014;105(2):141–150.
- Huang R, Wang X, Zhou Y, et al. RANKL-induced M1 macrophages are involved in bone formation. Bone Res. 2017; 5(1):17019.
- Kiechl S, Wittmann J, Giaccari A, et al. Blockade of receptor activator of nuclear factor-κB (RANKL) signaling improves hepatic insulin resistance and prevents development of diabetes mellitus. Nat Med. 2013;19(3):358–363.
- Atak B, Aktas G, Duman TT, et al. Diabetes control could through platelet-to-lymphocyte ratio in hemograms. Rev Assoc Med Bras. 2019;65(1):38–42.
- Duman TT, Aktas G, Atak BM, et al. Neutrophil to lymphocyte ratio as an indicative of diabetic control level in type 2 diabetes mellitus. Afr Health Sci. 2019;19(1):1602–1606.
- Bilgin S, Aktas G, Zahid Kocak M, et al. Association between novel inflammatory markers derived from hemogram indices and metabolic parameters in type 2 diabetic men. Aging Male. 2020;23(5):923–927.
- Liu L, Zhang C, Hu Y, et al. Protective effect of metformin on periapical lesions in rats by decreasing the ratio of receptor activator of nuclear factor kappa B ligand/osteoprotegerin. J Endod. 2012;38(7):943–947.
- Mai QG, Zhang ZM, Xu S, et al. Metformin stimulates osteoprotegerin and reduces RANKL expression in osteoblasts and ovariectomized rats. J Cell Biochem. 2011;112(10):2902–2909.
- Saisho Y. Metformin and inflammation: its potential beyond glucose-lowering Effect. Endocr Metab Immune Disord Drug Targets. 2015;15(3):196–205.
- Duan P, Yang M, Wei M, et al. Serum osteoprotegerin is a potential biomarker of insulin resistance in Chinese postmenopausal women with prediabetes and type 2 diabetes. Int J Endocrinol. 2017;2017:1–8.
- Cantley J, Ashcroft FQA. Insulin secretion and type 2 diabetes: why do β-cells fail?. BMC Biol. 2015;13:33.
- Jeffcoate WJ, Game F, Cavanagh PR. The role of proinflammatory cytokines in the cause of neuropathic osteoarthropathy (acute charcot foot) in diabetes. Lancet. 2005;366(9502):2058–2061.
- Dahiya N, Khadka A, Sharma AK, et al. Denosumab: a bone antiresorptive drug. Med J Armed Forces India. 2015;71(1):71–75.
- Mohsin S, Baniyas MM, AlDarmaki RS, et al. An update on therapies for the treatment of diabetes-induced osteoporosis. Expert Opin Biol Ther. 2019;19(9):937–948.
- Abe I, Ochi K, Takashi Y, et al. Effect of denosumab, a human monoclonal antibody of receptor activator of nuclear factor kappa-B ligand (RANKL), upon glycemic and metabolic parameters: effect of denosumab on glycemic parameters. Medicine. 2019;98(47):e18067.
- Schwartz AV, Schafer AL, Grey A, et al. Effects of antiresorptive therapies on glucose metabolism: results from the FIT, HORIZON-PFT, and FREEDOM trials. J Bone Miner Res. 2013;28(6):1348–1354.
- Downs SH, Black N. The feasibility of creating a checklist for the assessment of the methodological quality both of randomised and non-randomised studies of health care interventions. J Epidemiol Community Health. 1998;52(6):377–384.
- Hooper P, Jutai JW, Strong G, et al. Age-related macular degeneration and low-vision rehabilitation: a systematic review. Can J Ophthalmol. 2008;43(2):180–187.
- Sacks DB. Measurement of hemoglobin A(1c): a new twist on the path to harmony. Diabetes Care. 2012;35(12):2674–2680.
- Higgins J, Green S. Cochrane handbook for systematic reviews of interventions. Version 5.1.0 2011 [updated 2011 Mar; cited 2020 Dec 10]. Available from: https://handbook-5-1.cochrane.org/.
- Begg CB, Mazumdar M. Operating characteristics of a rank correlation test for publication bias. Biometrics. 1994;50(4):1088–1101.
- Egger M, Davey Smith G, Schneider M, et al. Bias in meta-analysis detected by a simple, graphical test. BMJ. 1997;315(7109):629–634.
- Lasco A, Morabito N, Basile G, et al. Denosumab inhibition of RANKL and insulin resistance in postmenopausal women with osteoporosis. Calcif Tissue Int. 2016;98(2):123–128.
- Passeri E, Benedini S, Costa E, et al. A single 60 mg dose of denosumab might improve hepatic insulin sensitivity in postmenopausal nondiabetic severe osteoporotic women. Int J Endocrinol. 2015;2015:352858.
- Rossini A, Frigerio S, Dozio E, et al. Effect of denosumab on glucose homeostasis in postmenopausal women with breast cancer treated with aromatase inhibitors: a pilot Study. Int J Endocrinol. 2020;2020:1809150.
- Napoli N, Pannacciulli N, Vittinghoff E, et al. Effect of denosumab on fasting glucose in women with diabetes or prediabetes from the FREEDOM trial. Diabetes Metab Res Rev. 2018;34(4):e2991.
- Chung PL, Zhou S, Eslami B, et al. Effect of age on regulation of human osteoclast differentiation. J Cell Biochem. 2014;115(8):1412–1419.
- Naumnik B, Klejna K, Koc-Żórawska E, et al. Age and gender predict OPG level and OPG/sRANKL ratio in maintenance hemodialysis patients. Adv Med Sci. 2013;58(2):382–387.
- de Paula FJ, Horowitz MC, Rosen CJ. Novel insights into the relationship between diabetes and osteoporosis. Diabetes Metab Res Rev. 2010;26(8):622–630.
- Singh S, Kumar D, Lal AK. Serum osteocalcin as a diagnostic biomarker for primary osteoporosis in Women. J Clin Diagn Res. 2015; 9(8):RC04–RC07.
- Kawai M, Devlin MJ, Rosen CJ. Fat targets for skeletal health. Nat Rev Rheumatol. 2009;5(7):365–372.
- Buch K, Gunmalm V, Andersson M, et al. Effect of chemotherapy and aromatase inhibitors in the adjuvant treatment of breast cancer on glucose and insulin metabolism–a systematic review. Cancer Med. 2019;8(1):238–245.
- Mirhosseini N, Vatanparast H, Mazidi M, et al. Vitamin D supplementation, glycemic control, and insulin resistance in prediabetics: a meta-analysis. J Endocr Soc. 2018;2(7):687–709.
- Yousefi Rad E, Djalali M, Koohdani F, et al. The effects of vitamin D supplementation on glucose control and insulin resistance in patients with diabetes type 2: a randomized clinical trial study. Iran J Public Health. 2014;43(12):1651–1656.
- Chaudhury A, Duvoor CR, Dendi VS, et al. Clinical review of antidiabetic drugs: Implications for type 2 diabetes mellitus management. Front Endocrinol. 2017;8:6.
- Baldock PA, Thomas GP, Hodge JM, et al. Vitamin D action and regulation of bone remodeling: suppression of osteoclastogenesis by the mature osteoblast. J Bone Miner Res. 2006;21(10):1618–1626.
- Nakamura Y, Suzuki T, Kamimura M, et al. Vitamin D and calcium are required at the time of denosumab administration during osteoporosis treatment. Bone Res. 2017;5(1):e17021.
- Sanchez-Rangel E, Inzucchi SE. Metformin: clinical use in type 2 diabetes. Diabetologia. 2017;60(9):1586–1593.
- Lim S, Ku EJ, Lee SY, et al. Therapeutic efficacy and safety of initial triple combination of metformin, sitagliptin, and lobeglitazone in drug-naïve patients with type 2 diabetes: initial triple study. BMJ Open Diabetes Res Care. 2020;8(1):e000807.
- Pratley R, Nauck M, Bailey T, et al. One year of liraglutide treatment offers sustained and more effective glycaemic control and weight reduction compared with sitagliptin, both in combination with metformin, in patients with type 2 diabetes: a randomised, parallel-group, open-label trial. Int J Clin Pract. 2011;65(4):397–407.
- Stein SA, Lamos EM, Davis SN. A review of the efficacy and safety of oral antidiabetic drugs. Expert Opin Drug Saf. 2013;12(2):153–175.
- Weir GC, Bonner-Weir S. Islet β cell mass in diabetes and how it relates to function, birth, and death. Ann N Y Acad Sci. 2013;1281(1):92–105.
- Kondegowda NG, Fenutria R, Pollack IR, et al. Osteoprotegerin and denosumab stimulate human beta cell proliferation through inhibition of the receptor activator of NF-κB ligand pathway. Cell Metab. 2015;22(1):77–85.
- Cunha JS, Ferreira VM, Maquigussa E, et al. Effects of high glucose and high insulin concentrations on osteoblast function in vitro. Cell Tissue Res. 2014;358(1):249–256.
- Boquete-Castro A, Gómez-Moreno G, Calvo-Guirado JL, et al. Denosumab and osteonecrosis of the jaw. A systematic analysis of events reported in clinical trials. Clin Oral Implants Res. 2016;27(3):367–375.
- Poudel P, Griffiths R, Wong VW, et al. Oral health knowledge, attitudes and care practices of people with diabetes: a systematic review. BMC Public Health. 2018;18(1):577.
- Deeks ED. Denosumab: a review in postmenopausal osteoporosis. Drugs Aging. 2018;35(2):163–173.
- Li N, Cornelissen D, Silverman S, et al. An updated systematic review of Cost-Effectiveness analyses of drugs for osteoporosis. Pharmacoeconomics. 2021;39(2):181–209.
- John M. Medicines for type 2 diabetes: a review of the research for adults. In: Comparative effectiveness review summary guides for clinicians [Internet]. Rockville (MD): Agency for Healthcare Research and Quality (US); 2007 [cited 2021 Aug 1]. Available from: https://www.ncbi.nlm.nih.gov/books/NBK63537/