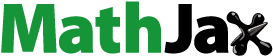
Abstract
The study area, affected by war and siege, necessitates a study of its current ecological status. The objective of this study was to assess the present forest structure, species diversity, and Total Biomass Carbon Stock (TBCS) in relation to environmental variables. The findings shown 42 woody plant species, with 23 in the highland and 41 in the midland. No significant difference in species abundance is observed between the highland and midland areas. However, there are significant differences in the average diameter and height of trees between the altitudinal classes. Additionally, diversity indices shown significant difference, with the midland shown higher values than the highland. TBCS of the forest, the highland and midland areas were 21.02, 15.6, and 22.92 tons/ha, respectively. Moreover, TBCS shown significant contributions from tree diameter and height (p < 0.05) whereas species diversity, richness, and abundance do not shown a significant influence. This research further assesses factors influencing carbon stock, identifying annual mean temperature, soil organic content, temperature seasonality, and altitude as key contributors. Dominant species are limited, with around 50% classified as rare. Hence, our study suggests that the implementation of effective forest management practices aimed at enhancing biodiversity and ecosystem services.
1. Introduction
Managing and conserving forest carbon is identified as a cost-effective decision for mitigating climate change, contributing additional advantages such as biodiversity conservation and the regulation of the hydrological cycle, along with various other forest ecosystem services (Canadell and Raupach, Citation2008; DeFries et al., Citation2010). Climate change causes threat to forest ecosystem services and biodiversity loss (Thom et al. Citation2019; Seidl et al., Citation2014). The widespread impact of rising temperatures, alterations in precipitation patterns, and various disturbances such as droughts and storms is already significantly changing the dynamics of vegetation (McDowel et al., 2020).
Forest structure, composition, and diversity have ecological roles and shows strong correlations with environmental and anthropogenic factors (Gairola et al., Citation2008; Ahmad et al., Citation2011). Forest structure, composition and the diversity are crucial elements in maintaining forest biodiversity and establishing sustainable forest management practices (Gutiérrez and Huth, Citation2012). Those are used in the study of the global carbon budget and serving as significant carbon pools (Canadell et al., Citation2007). Assessing the structure and composition of forests is crucial, as it plays a vital role in the development of adaptive strategies. These strategies are essential for ensuring the sustained provision of ecosystem services in forests and contributing to the conservation of biodiversity and the mitigation of climate change (Kremen and Merenlender, Citation2018; Messier et al., Citation2019). The most effective outcomes for conserving forests are linked to management approaches that combine conservation efforts with community involvement and law enforcement (Ahmad et al., Citation2022).
Forests, recognized as the largest carbon sink among terrestrial ecosystems, are essential contributors to the global carbon cycle (Coulston et al., Citation2015). With concerns increasing about climate change, it is crucial to monitor the amount of carbon in the terrestrial (Böttcher et al., Citation2008). Concerns regarding climate changes have forced the international community to monitor terrestrial carbon (Ahmad et al., Citation2018). The guidelines provided by the Intergovernmental Panel on Climate Change (IPCC) for measuring national greenhouse gas emissions strictly mandate monitoring carbon pools (Böttcher et al., Citation2008). Similarly, the United Nations collaborative program on reducing emissions from deforestation and forest degradation on combating climate change also requires the regional and periodic estimation of carbon in the forest ecosystem (Le Quere et al., 2009) for developing management and conservation plans (Billings et al., 2008). The UN Framework Convention on Climate Change (UNFCCC) also identifies that, reducing emissions by controlling deforestation and forest degradation is a cost-effective approach for mitigating climate change (DeFries et al. in 2010).
Carbon stock impacted by various biotic and abiotic factors (Mazarrasa et al., Citation2017), is crucial to understand for explaining inter-habitat variability and estimating carbon levels. It is also affected by structural diversity (Noulekoun et al. 2021). The study of species diversity and richness along elevation gradients is crucial (Molina-Vanegas et al., Citation2020; Willig & Presley Citation2016). The diversity shows significant variation in correlation with altitude changes (Wani et al., 2020). Plant diversification in tropical mountains is influenced by both geographic isolation and environmental heterogeneity. However, there is a lack of scientific information on these issues, which is critical for developing appropriate conservation measures and promoting the sustainable utilization of forests. Moreover, the study area has experienced deforestation due to war and a complete siege, emphasizing the urgency of assessing its current status.
Previous studies in the Hugumbrda Grat-Kahsu forest, like Kidane et al. (Citation2016), recommend conducting future research on forest composition and carbon stock in relation to environmental variables influence. Among these environmental variables, altitude plays a crucial role in shaping plant species diversity, floristic composition, and carbon stock. Altitude influences species distribution, composition, and diversity by shaping the local climate (Gaston, Citation2000). While there have been scientific studies on carbon stock in Ethiopian forest ecosystems, the specific impact of altitude on carbon stock in the dry Afromontane forests of northern Ethiopia is still limited in existing research (Muluneh and Worku, Citation2022). Therefore, this study aimed to investigate the relationship between altitude, species diversity, and Total Biomass Carbon Stock (TBCS). The research hypotheses were, altitudinal variation plays a significant role in driving species diversity, composition and structure, altitude is a crucial factor influencing TBCS, species diversity and abundance have an impact on TBCS and, various environmental factors affect TBCS in the forest.
2. Material and Methods
2.1 Study area description
The study was conducted in the Hugumbrda Grat-Kahsu National Forest protected area located in the southern zone of Tigray state, Ethiopia. The study area is located within a defined latitude range of 12° 25' - 12° 45'N and longitude 39° 23'-39° 45' E. The forest is found in an area with altitudes ranging from 1501 to 3683 m.a.s.l. The forest includes both midland and highland area, with the midland (1500-2500 m.a.s.l) covering a larger portion, the highland area (above 2500 m.a.s.l) constitutes the remaining portion. The average annual precipitation ranges from 653 to 818.6 mm, while the average maximum temperature ranges within 21.4 to 30 °C and the average minimum temperature ranges from 9.4 to 14.8 °C (Abrha et al., Citation2023). The forest is classified as a dry evergreen Afromontane Forest (Kidane et al., Citation2018). The area is characterized by rough and hilly landscape. The predominant soil types are Vertisol, leptosoil, and cambosols (Abrha et al., Citation2023).
The forest area consists of plantation, natural forests, bushes, shrubs, agricultural, and settlement areas (Kidane et al., Citation2018) and lake (Gebru et al., Citation2019). Within and around the forest boundary, there are growing human settlement, estimated at 26,889 households, with 5,496 households located fully within the forest area and the remaining 21,393 households residing in the peripheral areas in 2010 (Woldemichael et al., Citation2010). The local agricultural activity is dominated by mixed farming systems. The main food crops cultivated include Zea mays, Sorghum bicolor, Triticum durum, Eragrostis tef, Hordeum vulgare, Pisum sativum, and Cicer arietinum. In addition, high-value tree crops such as Mangifera indica, Persea americana, Carica papaya and Malus domestica are produced. The major livestock species reared in the area include cattle, sheep, goats, donkey, horse, mule, and camels (Gebru et al., Citation2019).
2.2 Input data
The forest inventory was intended following transect sampling approach, implementing a random systematic distribution of circular plots using a proportional allocation method (). A total of 37 transects were established, with a horizontal spacing of 250 meters and a vertical distance of 100 meters between plots. Within these transects, 188 representative circular plots were set up, each with a radius of 11.28 meters, to gather forest measurement data. The circular plot, as indicated in , had an area of 400 m2. Circular plots were used in this study, facilitating the identification of the center of the GPS point for establishing a permanent reference for future research. About 188 plots were utilized in this study to enhance the precision and reliability of the sampling process. The elevation of each species within each plot were taken using GPS with an accuracy of less than 3 meters. For each tree within the plot, both the height and diameter were measured. The diameters of woody plant species were assessed using a caliper at two different heights: at breast height (1.3m) and at stump height (30cm). To determine the heights of these plant species, a meter tape was used for shorter trees, while a clinometer was employed for taller ones. Only woody plant species taller than 1 meter were included in the measurements and for analysis.
The woody plant species found at each plot were identified with the help of the local community, published and unpublished literatures such as the flora of Ethiopia and Eritrea (Hedberg et al., Citation1995; Edwards et al., Citation1997; Edwards et al., Citation2000), and useful trees and shrubs for Ethiopia (Bekele, Citation2007; Woldemichael et al., Citation2010). The study evaluated tree diameter, tree height, woody species diversity, woody plant species composition, and TBCS in highland and midland altitudinal classes. There were 55 and 133 sample plots laid down in highland and midland, respectively, indicating that the size of the forest in highland areas was smaller compared to midland areas. It was conducted on the area coverage of altitudinal classes. The number of samples was determined using a combination of observational size of the forest and random sampling methods to ensure that the forest area was adequately represented in the sample and to minimize the effects of bias.
The research methodology involved obtaining bioclimatic variables from the WorldClim database at a 1 km resolution from WorldClim version 2.1 (Fick and Hijmans, Citation2017). These bioclimatic variables were derived from monthly temperature and rainfall values to enhance their biological relevance. In addition to climate data, soil data at a depth of 15-30 cm were acquired from the ISRIC (International Soil Resources Information Center) "SoilGrids" global soil Information system. The extraction of soil and climate data based on the tree locations was facilitated using Arc GIS. The utilization of both WorldClim and SoilGrids databases in with GIS tools provided a robust foundation for analyzing the bioclimatic and soil factors influencing the forest structure and species diversity.
2.3 Data analysis
2.3.1 Forest composition and structure
In accordance with the modified Braun-Blanquet scale established by van der Maarel (Citation1979), species cover values were categorized into different classes, each corresponding to specific cover percentages. These classes involve cover percentages of less than 5%, 25%, 50%, 75%, and greater than 75%, represented as rare, few, moderate, high, and dominant, respectively. These percentage-based classes serve as crucial indicators for assessing the presence and status of species within a given area. Within the forest, abundance, frequency, and stand density of trees were estimated. The evaluation of species contribution was achieved through the calculation of the Importance Value Index (IVI). The IVI was derived by summing the relative density, relative dominance, and relative frequency of a woody plant species, as expressed in Eq. 1-6.
(Eq. 1)
(Eq. 1)
(Eq. 2)
(Eq. 2)
(Eq. 3)
(Eq. 3)
(Eq. 4)
(Eq. 4)
(Eq. 5)
(Eq. 5)
(Eq. 6)
(Eq. 6)
2.3.2 Species diversity indices
The Shannon and Simpson diversity indices were used to determine species diversity. The Shannon Diversity Index ranges from 0 to 1, where 1 indicates complete evenness (Konopiński, Citation2020). The higher the index, the more diverse the species are in the habitat (Beisel and Moreteau, Citation1997). The Shannon index describes the relative evenness or equitability of species, while Simpson’s index gives weight to dominant species (Whittaker Citation1972). Simpson Diversity Index is a measure of diversity that takes into account the number of species present, as well as the relative abundance of each species (Gregorius and Gillet, Citation2008). Those indices were estimated using PAleontological Statistics (PAST 4.3) software.
2.3.3 Carbon stock
General allometric equations, established from a large dataset of trees (Jalkanen et al., Citation2005), demonstrate a significant statistical relationship with destructively measured biomass amounts. An essential advantage of these equations is their base on a wide range of tree diameters (Brown, Citation1997). Their application is vital for estimating biomass and carbon stock in terrestrial ecosystems without tree cutting and deforestation. In estimating Below-Ground Biomass (BGB), which encompasses all live root biomass, this study adopted the range of 20-26% of Above-Ground Biomass (AGB) based on the findings of Santantonio et al. (1997). For the specific calculations in this research, a value of 25% of AGB was selected to estimate BGB. The determination of carbon stock applied it as 50% of the Total Biomass (TB) (Pearson et al., 2005, Brown et al., Citation2005).
Wood Density (WD) data from the global wood density database were used to estimate AGB. In cases where a specific species was not found in the global wood density database, a default value of 0.6 g/cm3 was applied, based the recommendation by Brown et al. (Citation2005). The calculation of AGB applied by a general allometric equation developed by Chave et al. (Citation2014), chosen for its usefulness across various climatic conditions and different vegetation types. This model, was selected for its broad applicability, incorporates key biomass predictor variables such as Height (H), Diameter (D) and WD (Eq. 7).
(Eq. 7)
(Eq. 7)
In this study, specific tree allometric equations were also used in addition to the general allometric equation. Allometric equations are mathematical models specifically developed to estimate the AGB of individual tree species. Recognizing the unique characteristics and growth patterns of different tree species, we apply species-site-specific equations (Eq. 8-13).
(Eq. 8)
(Eq. 8)
(Eq. 9)
(Eq. 9)
(Eq. 10)
(Eq. 10)
(Eq. 11)
(Eq. 11)
(Eq. 12)
(Eq. 12)
(Eq. 13)
(Eq. 13)
The analysis of the contribution of environmental variables to TBCS in this study used the Random Forest (RF) algorithm for variable selection. RF, introduced by Breiman (Citation2001), is a widely recognized and highly efficient algorithm. It has gained popularity across various applications due to its robustness, non-parametric nature, and insensitivity to data skew (Biau et al., Citation2008). RF regression techniques, specifically, have been extensively utilized in previous studies for mapping carbon stocks (Srinivasarao et al., 2014). As a result, RF was applied to identify the impact of environmental variables on TBCS.
This analysis combined a total of 28 variables, including 19 climatic predictive variables, 7 soil predictor variables, elevation, and carbon stock (refer to for details). To assess model accuracy of the model, 80% of the data was utilized for training, and the remaining 20% was reserved for testing purposes. Environmental predictor values were extracted from each point location, converted to numerical values using Arc GIS, and subsequently included in the statistical analysis using the algorithm.
Table 1: Environmental variables.
2.4 Statistical Analysis
A normality test was conducted to assess the distribution of variables, including tree height, species diversity (Simpson and Shannon indices), species richness, DBH, abundance and TBCS. The results indicated that none of the variables followed a normal distribution, as evidenced by a p-value (α = 0.05). To further examine the relationships within the dataset, non-parametric statistical tests were employed. The regression test was used to evaluate the impact of species diversity, richness, abundance, tree height and DBH on TBCS, while the Mann-Whitney test was applied to assess the influence of altitude on tree height and DBH. Additionally, the Mann-Whitney test was applied to compare the effects of altitude on TBCS, species richness, diversity, and species abundance. Descriptive statistics, specifically mean estimates, were then used. To assess the influence of environmental variables on TBCS, the study used the Machine Learning technique known as Random Forest (RF). The study thus used a combination of normality tests, non-parametric tests, RF, descriptive statistics to analyze and interpret the data.
3. Results and discussions
3.1 Forest composition and structure
The study identified a total of 42 woody plant species across 188 plots, with 23 species observed in the highland and 41 species in the midland. In the forest, the estimated abundance of woody species was 906 stems per hectare. Six species, Juniperus procera, Carissa edulis, Cadia purpurea, Maytenus undata, Dodonaea viscosa subsp. Angustifolia, and Acacia abyssinica were identified as abundant. Among them, Juniperus procera emerged as the most abundant species, constituting 21.27% of the total. The study further shown that the top three important species, based on the Importance Value Index (IVI), were Juniperus procera, Acacia abyssinica, and Carissa edulis, collectively representing 44% of the total IVI. These three species also accounted for 45.2% of the relative abundance, as detailed in .
Table 2: Importance value index of each woody plant species.
However, Olinia rochetiana, Podocarpus falcatus, Dovyalis abyssinica, Pterolobium stellatum, Grewia tembensis, Clerodendrum myricoides, Clutia abyssinica, Ficus sur, Balanites aegyptiaca, Cordia africana, Ziziphus mucronata, Acokanthera schimperi and Discopodium penninervium were rare species with abundance of 0.85%. The species in the area shown different abundance levels based on the cover classes defined by the modified Braun-Blanquet scale. Specifically, 50% of the species are classified as rare, 14.6% as few, 22% as moderate, 4.9% as high, and 9.8% as dominant. Species most susceptible to loss are those with small numbers (Gayton, Citation2008). The loss of tree species has a negative impact on biodiversity, and structural complexity (Mansourian et al., Citation2005). The extinction of a single tree species can alter an ecosystem and rapid decline of ecosystem functions (Henry et al., Citation2017).
Identification of both rare and dominant tree species within a forest is essential for ecological assessments and effective conservation and management strategies. Rare tree species contribute significantly to biodiversity. Understanding the roles and distribution patterns of both dominant and rare species is crucial for implementing sustainable forest management practices and aiding decision-making in climate change mitigation. This is particularly relevant in the context of forest deforestation, rising global temperatures, and the need for biodiversity conservation (Basile, Citation2022: Tang et al., Citation2023). Conservation efforts are enhanced by prioritizing rare species, which may be more susceptible to threats, while recognizing dominant species provides insights into the economic value of the forest and its potential contributions to various industries and biodiversity. Additionally, both rare and dominant species serve as indicators of environmental change, aiding in climate change monitoring. The identification of these species is fundamental to habitat assessment, ecological research, and planning for habitat restoration and forest regeneration. In essence, recognizing both rare and dominant tree species within a forest is an integral step toward preserving biodiversity, sustaining ecosystem services, and ensuring the long-term health and resilience of the forest area.
This study did not find a significant difference in species abundance between the two altitudinal classes (p > 0.05, 0.546). Specifically, the abundance of species in the highland, midland, and the entire forest was recorded as 35.39, 36.58, and 36.24, respectively. The study observed significant differences in mean tree diameter and height among the highland, midland, and the entire forest. The mean tree diameter was 9.24 cm in the highland, 8.07 cm in the midland, and 8.41 cm in the entire forest. Additionally, the mean height varied, with the entire forest having a mean height of 3.92m, 3.87m in the highland, and 3.89m in the midland. These differences were found to be statistically significant, at a p-value of less than 0.05. The variations in diameter and height across altitudinal classes suggest that altitude influences the growth characteristics of trees in the studied area.
The results of this study are consistent with previous research conducted in the study area, particularly in Hugumbrda Grat-Kahsu forest (Aynekulu, Citation2011). Acacia abyssinica was also identified as the dominant species in the highlands of Tigray, as stated by Gidey et al. (Citation2013). Other dominant species in the dry Afromontane forests include Olea europaea, Juniperus procera, Acacia abyssinica, Cadia purpurea, Dodonaea viscosa subsp. angustifolia, Carissa edulis, and others (Kidane, 2015). Moreover, various studies conducted in the central and Northern highlands (Mengistu et al., Citation2005), southern lowlands (Angassa and Oba, Citation2010), and North-western (Mebrat et al., Citation2014) regions of Ethiopia have demonstrated the effectiveness of exclosures in improving species composition, diversity, and density.
Similar with this study, altitude highly influences the ecosystem structure, composition, and biomass by altering different factors of the environment, including precipitation, temperature, slope, aspect, soil properties, etc. (Alves et al., Citation2010). It have a significant influence on plant species distribution, composition, and structure across different ecosystems (Grytnes and Beaman, Citation2006). It is an important environmental factor that affects the presence, growth, and diversity of plant species (Zhao and Fang, Citation2006). Forests at lower elevations are characterized by greater diversity and a richer variety of plant species compared to higher-altitude forests. Additionally, there is an uneven pattern in the distribution of vegetation biomass and carbon content along the altitudinal gradient (Ali et al., Citation2022).
3.2 Species diversity
The forest shown a Simpson diversity index of 0.897 and a Shannon diversity index of 2.655. In comparison, the highland and midland shown Simpson diversity indices of 0.8413 and 0.9053, and Shannon diversity indices of 2.223 and 2.726, respectively. Both Shannon and Simpson index values were higher in the midland compared to the highland. The entire forest, highland, and midland areas had species richness values of 5.43, 4.24, and 5.9 per plot, respectively. The lower species richness in the forest area is attributed to the presence of a few dominant species, as well as the study's focus on trees with a height exceeding 1 meter. The study identified a total of 42 woody plant species, with 23 species observed in the highland and 41 species in the midland. This indicates that the midland forest had a greater diversity of woody plant species in comparison. The findings shown the significant influence of altitude on both diversity and richness.
Similar results were reported in exclosures of Northern Ethiopia (Mengistu et al., Citation2005; Birhane et al., Citation2007), aligning with the broader understanding that altitude contributes significantly to species richness and diversity (Kreft and Jetz, Citation2007). In mountainous areas, a decrease in species diversity with increasing altitude is often observed due to a decrease in land area per bioclimatic belt (Körner, 2007). High elevation communities will be strongly impacted by a changing climate, with the drivers of this biotic change being the direct effect of climate change through multiple interacting climate variables and indirectly climate-mediated species interactions. Both of these mechanisms depend on the fine-scale topographic gradients that universally characterize these mountain systems (Seastedt and Oldfather, Citation2021). Galván-Cisneros et al. (Citation2023) found an environmental filtering effect with increasing altitude causing phylogenetic clustering, decreased phylogenetic diversity, and decreased species richness. The decreasing phylogenetic distances between closest relatives are congruent with neo-endemics, suggesting recent plant diversification in high altitudes of tropical mountains, possibly driven by geographic isolation and environmental heterogeneity.
3.3 Carbon stock
The findings shown that the top four predictors for carbon stock were bio1, soil organic matter, bio4, and altitude, which collectively accounted for 22.8% of the influence (). These variables and their corresponding importance values were assessed, including bio1 (12.325), bio2 (4.617), bio3 (4.512), bio4 (6.980), bio5 (5.652), bio6 (3.597), bio7 (5.907), bio8 (5.815), bio9 (4.346), bio10 (2.663), bio11 (4.647), bio12 (5.227), bio13 (5.596), bio14 (2.357), bio15 (4.807), bio16 (4.993), bio17 (4.927), bio18 (3.852), bio19 (5.934), altitude (6.581), cation (6.259), clay (5.167), bulk (5.842), sand (5.154), silt (4.193), pH (6.012), and soil organic matter (so) (7.293). These importance values indicate the relative significance of each variable in terms of mean increase error and provide insights into their impact on carbon stock of the forest.
The forest carbon stock measured at 21.02 tons per hectare, with the highland and midland shown carbon stocks of 15.6 and 22.92 tons per hectare, respectively. This difference indicates that the highland forest has a lower carbon stock than the midland, indicating significance influence of altitude on carbon stock (p < 0.05). Additionally, it is important to highlight that tree height and DBH show a significant impact on TBCS with a p-value below 0.05, whereas species diversity, richness, and abundance do not demonstrate a significant influence (p > 0.05).
This study suggests that large-sized trees play a crucial role as predictors of TBCS. These findings align with previous research indicating that large-sized trees are linked to higher AGB stocks in tropical forests, reflecting their capacity to store substantial amounts of biomass per tree (Slik et al., Citation2013). Similarly, the positive influence of basal area, tree density, tree diameter, species richness, and species diversity on carbon storage in ecosystems has been observed in other studies, such as the work by Kaushal and Baishya (Citation2021). However, in subtropical forests, climate and large-sized trees, but not diversity, drive above-ground biomass (Bordin et al., Citation2021). These findings collectively contribute to a deeper understanding of the factors influencing carbon stock and storage in forest ecosystems.
Several studies conducted in other parts of Ethiopia and other tropical regions have observed a decreasing trend of biomass carbon stock with increasing elevation (Tesfaye and Negash Citation2018; Simegn et al. Citation2014). The mean total biomass carbon stock in the study's forest estimated within the range of studies conducted in other areas, such as the dry Afromontane forest of Northern Ethiopia (Mokria et al., Citation2015), Sub-Saharan Africa tropical dry forest (17-72 t/ha) (Gibbs et al., Citation2007).
The carbon storing capacity of forests is influenced by a complex interaction of growth conditions, species structure, and various disturbance factors (Ali et al., Citation2019). Tree biomass and carbon levels within a forest are highly dependent on factors such as tree type, forest structure, tree diameter, tree age, precipitation, stand condition, and various topographic and edaphic variables (Ullah et al., Citation2020). Functional diversity traits, including DBH, tree height, and wood density, have been reported to have a stronger correlation with Ethiopian woodland AGB than species diversity components (Sintayehu et al., Citation2020). The study suggests that both functional traits, particularly maximum DBH, and species richness traits significantly correlate with biomass, indicating that forests have the potential to accommodate more species. Management strategies can focus on enhancing the biodiversity of forest stands, simultaneously enhance carbon stocks (Kaushal and Baishya, Citation2021).
In other studies, it has been reported that species diversity and, more particularly, structural diversity positively influence AGB (Mensah et al., Citation2023). Forest Above-Ground Carbon (AGC) storage has been found to vary among different forest types (Jia et al., Citation2022), and climate has been identified as a factor influencing tree species diversity and AGC patterns in semi-arid tree savannas (Mensah et al., Citation2023). These findings collectively show the complicated nature of factors influencing carbon storage in the forest and the importance of biodiversity and forest structure.
3.4 Implication of the study
This study has significant implications for ecology, biodiversity conservation, and forest management. Understanding different patterns in species abundance, TBCS and distribution shows the necessity for adaptive forest management practices, particularly considering variations between highland and midland areas. Altitude is a key factor influencing forest structural characteristics and carbon storage capacity, guiding the development of management strategies. This study indicates the crucial role of forests at varying altitudinal classes in carbon sequestration, aligning with global climate change mitigation goals. Continuous monitoring and research on forest species composition, diversity, TBCS and abundance are essential for identifying adaptive management decisions.
3.5 Limitations and challenges
The study faced limitations due to the impacts of war and complete siege, resulting in restricted access to the whole study area. War and complete siege-affected areas presented difficulties in acquiring updated documents and conducting field surveys, including soil data collection. There was deforestation due to the war and siege. The presence of military bombs in the war-torn area added to the challenge of excavating soil for soil experiment. In response to this constraint, the study utilizes satellite data, ISRIC for soil analysis.
4. Conclusions and future works
The study identified a variety of woody plant species, with observations in both highland and midland areas. Species diversity was higher in the midland, suggesting a greater number of woody plant varieties there. The results underscore the significance impact of altitude on species diversity. The three most significant species, determined by the Importance Value Index (IVI), were Juniperus procera, Acacia abyssinica, and Carissa edulis, collectively representing a substantial portion of the total IVI and relative abundance. Significant differences in mean tree diameter and height were observed between the highland and midland, indicating that altitude affects the growth characteristics of trees in the study area. The highland forest shown a lower carbon stock compared to the midland, highlighting the influence of altitude on carbon storage. It is crucial to emphasize that tree height and DBH significantly impact TBCS, whereas species diversity, richness, and abundance do not show a significant influence.
Hence, future research efforts should prioritize understanding the temporal dynamics of the identified woody plant species, their abundance, and carbon stock. Exploring microclimatic factors, including soil moisture, is essential for a comprehensive understanding of their impact on plant growth and diversity across different altitudinal zones. In addition, it is important to raise awareness among local communities, policymakers, and stakeholders about the significant influence of altitude and other factors on both plant diversity and carbon stock. This awareness is key to informing sustainable land use practices and policies, encouraging a more ecologically responsible approach for forest ecosystem service management. Establishing a long-term carbon stock monitoring program should be a priority, allowing for continuous tracking of changes over time and providing essential data for assessing the ecosystem role in climate change mitigation. Predicting the distribution of the rare and dominant species is also important for species conservation and domestication.
Ethics declarations
Availability of data and material: Data will be made available on request
Conflict of interest: The authors declare no conflict of interest
Author’s contribution
H.A. Data collection, conception and design, writing, data analysis, revising, final approval of the version.
S.D. Conception, writing, data analysis, revising, proofreading
V.O. Data analysis, interpretation of the findings, proofreading.
E.B. Data analysis, writing, proofreading
A.M. Data analysis, proof reading and revising
Acknowledgement
We acknowledge to Dr. Florent Noulèkoun for his constructive comments during the development of this paper.
Additional information
Funding
References
- Abrha, H., Dodiomon, S., Ongoma, V., Hagos, H. and Birhane, E., 2023. Spatio-temporal prediction of climate and wildfire in Hugumbrda Grat-Kahsu forest, Tigray: priority for early warning. Geomatics, Natural Hazards and Risk, 14(1), p.2250517. doi: 10.1080/19475705.2023.2250517.
- Ahmad, A., Ahmad, S., Nabi, G., Liu, Q.J., Islam, N. and Luan, X., 2022. Trends in deforestation as a response to management regimes and policy intervention in the Hindu Kush Himalaya of Pakistan. Frontiers in Environmental Science, 10, p.810806. doi: 10.3389/fenvs.2022.810806.
- Ahmad, A., Liu, Q.-J., Nizami, S. M., Mannan, A., and Saeed, S. (2018). Carbon Emission from Deforestation, forest Degradation and wood Harvest in the Temperate Region of Hindukush Himalaya, Pakistan between 1994 and 2016. Land Use Policy 78, 781–790. doi: 10.1016/j.landusepol.2018.07.009.
- Ahmad, I.F.T.I.K.H.A.R., Ahmad, M.S.A., Hussain, M.U.M.T.A.Z., Ashraf, M.U.H.A.M.M.A.D. and Ashraf, M.Y., 2011. Spatio-temporal variations in soil characteristics and nutrient availability of an open scrub type rangeland in the sub-mountainous Himalayan tract of Pakistan. Pak. J. Bot, 43(1), pp.565–571. https://www.pakbs.org/pjbot/PDFs/43(1)/PJB43(1)565.pdf
- Ali, F., Khan, N., Abd_Allah, E.F. and Ahmad, A., 2022. Species diversity, growing stock variables and carbon mitigation potential in the phytocoenosis of Monotheca buxifolia forests along altitudinal gradient across Pakistan. Applied Sciences, 12(3), p.1292. doi: 10.3390/app12031292.
- Ali, F., Khan, N., Ahmad, A. and Khan, A.A., 2019. Structure and biomass carbon of Olea ferruginea forests in the foot hills of Malakand division, Hindukush range mountains of Pakistan. Acta Ecologica Sinica, 39(4), pp.261–266. doi: 10.1016/j.chnaes.2019.05.011.
- Alves, L.F., Vieira, S.A., Scaranello, M.A., Camargo, P.B., Santos, F.A., Joly, C.A. and Martinelli, L.A., 2010. Forest structure and live aboveground biomass variation along an elevational gradient of tropical Atlantic moist forest (Brazil). Forest ecology and management, 260(5), pp.679–691. doi: 10.1016/j.foreco.2010.05.023.
- Angassa, A., Oba, G., Treydte, A.C. and Weladji, R.B., 2010. Role of traditional enclosures on the diversity of herbaceous vegetation in a semi-arid rangeland, southern Ethiopia. Livestock Research for Rural Development, 22(9).
- Assefa, G., 2009. Evaluation models for estimation of carbon sequestrated by Cupressues lustanica plantation at Wondo Genet, Ethiopia. M.Sc. Thesis. Hawassa University, WGCF&NR, Ethiopia.
- Aynekulu, E., Denich, M., Tsegaye, D., Aerts, R., Neuwirth, B. and Boehmer, H.J., 2011. Dieback affects forest structure in a dry Afromontane forest in northern Ethiopia. Journal of Arid Environments, 75(5), pp.499–503. doi: 10.1016/j.jaridenv.2010.12.013.
- Basile, M., 2022. Rare species disproportionally contribute to functional diversity in managed forests. Scientific Reports, 12(1), p.5897. doi: 10.1038/s41598-022-09624-9.
- Beisel, J.N. and Moreteau, J.C., 1997. A simple formula for calculating the lower limit of Shannon's diversity index. Ecological Modelling, 99(2-3), pp.289–292. doi: 10.1016/S0304-3800(97)01954-6.
- Bekele-Tesemma, A. and Tengnäs, B., 2007. Useful trees and shrubs of Ethiopia: identification, propagation, and management for 17 agroclimatic zones (p. 552). Nirobi: RELMA in ICRAF Project, World Agroforestry Centre, Eastern Africa Region.
- Biau, G., Devroye, L. and Lugosi, G., 2008. Consistency of random forests and other averaging classifiers. Journal of Machine Learning Research, 9(9).
- Birhane, E., Teketay, D. and Barklund, P., 2007. Enclosures to enhance woody species diversity in the dry lands of eastern Tigray, Ethiopia. East African Journal of Sciences, 1(2), pp.136–147. doi: 10.4314/eajsci.v1i2.40352.
- Bordin, K.M., Esquivel-Muelbert, A., Bergamin, R.S., Klipel, J., Picolotto, R.C., Frangipani, M.A., Zanini, K.J., Cianciaruso, M.V., Jarenkow, J.A., Jurinitz, C.F. and Molz, M., 2021. Climate and large-sized trees, but not diversity, drive above-ground biomass in subtropical forests. Forest Ecology and Management, 490, p.119126. doi: 10.1016/j.foreco.2021.119126.
- Böttcher, H., Kurz, W.A. and Freibauer, A., 2008. Accounting of forest carbon sinks and sources under a future climate protocol—factoring out past disturbance and management effects on age–class structure. Environmental Science & Policy, 11(8), pp.669–686. doi: 10.1016/j.envsci.2008.08.005.
- Breiman, L., 2001. Random forests. Machine learning, 45, pp.5–32. doi: 10.1023/A:1010933404324.
- Brown, S., 1997. Estimating biomass and biomass change of tropical forests: a primer. For the Food and Agriculture Organization of the United Nations. Rome, 1997. FAO Forestry Paper-134. ISBN 92-5-103955-0.
- Brown, S., Pearson, T., Slaymaker, D., Ambagis, S., Moore, N., Novelo, D. and Sabido, W., 2005. Creating a virtual tropical forest from three‐dimensional aerial imagery to estimate carbon stocks. Ecological applications, 15(3), pp.1083–1095. doi: 10.1890/04-0829.
- Canadell, J.G. and Raupach, M.R., 2008. Managing forests for climate change mitigation. science, 320(5882), pp.1456–1457. doi: 10.1126/science.1155458.
- Canadell, J.G., Le Quéré, C., Raupach, M.R., Field, C.B., Buitenhuis, E.T., Ciais, P., Conway, T.J., Gillett, N.P., Houghton, R.A. and Marland, G., 2007. Contributions to accelerating atmospheric CO2 growth from economic activity, carbon intensity, and efficiency of natural sinks. Proceedings of the national academy of sciences, 104(47), pp.18866–18870. doi: 10.1073/pnas.0702737104.
- Chave, J., Réjou‐Méchain, M., Búrquez, A., Chidumayo, E., Colgan, M.S., Delitti, W.B., Duque, A., Eid, T., Fearnside, P.M., Goodman, R.C. and Henry, M., 2014. Improved allometric models to estimate the aboveground biomass of tropical trees. Global change biology, 20(10), pp.3177–3190. doi: 10.1111/gcb.12629.
- Coulston, J.W., Wear, D.N. and Vose, J.M., 2015. Complex forest dynamics indicate potential for slowing carbon accumulation in the southeastern United States. Scientific reports, 5(1), p.8002. doi: 10.1038/srep08002.
- DeFries, R.S., Rudel, T., Uriarte, M. and Hansen, M., 2010. Deforestation driven by urban population growth and agricultural trade in the twenty-first century. Nature Geoscience, 3(3), pp.178–181. doi: 10.1038/ngeo756.
- Dharmesh G. Jaiswal, Chirag N. Patel, Hitesh A. Solanki and Himanshu A. Pandya (2018). Allometric model to determine carbon stock from DBH of major tree species in Mansa Range, Gandhinagar Forest Division, Gujarat, India. International Journal of Research in Advent Technology, 6 (6), 2321–9637.
- Edwards, S., Demissew, S. and Hedberg, I., 1997. Flora of Ethiopia and Eritrea: Hydrocharitaceae to Arecaceae. The National Herbarium, Addis Ababa University.
- Edwards, S., Mesfin, T., Sebsebe, D. and Hedberg, I., 2000. Flora of Ethiopia and Eritrea, vol. 2 (1). The National Herbarium, Addis Ababa University, Addis Ababa and Department of Systematic Botany, Uppsala University, Uppsala.
- Fick, S.E. and Hijmans, R.J. (2017) WorldClim 2: New 1-Km Spatial Resolution Climate Surfaces for Global Land Areas. International Journal of Climatology, 37, 4302–4315. doi: 10.1002/joc.5086.
- Gairola, S., Rawal, R.S. and Todaria, N.P., 2008. Forest vegetation patterns along an altitudinal gradient in sub-alpine zone of west Himalaya, India. African Journal of Plant Science, 2(6), pp.42–48. http://www.academicjournals.org/AJPS
- Galván-Cisneros, C.M., Villa, P.M., Coelho, A.J., Campos, P.V. and Meira-Neto, J.A., 2023. Altitude as environmental filtering influencing phylogenetic diversity and species richness of plants in tropical mountains. Journal of Mountain Science, 20(2), pp.285–298. doi: 10.1016/j.envexpbot.2019.10385310.1007/s11629-022-7687-9.
- Gaston, K.J., 2000. Global patterns in biodiversity. Nature, 405(6783), pp.220–227. doi: 10.1038/35012228.
- Gayton, D.V., 2008. Impacts of climate change on British Columbia's biodiversity: a literature review. Journal of Ecosystems and Management. doi: 10.22230/jem.2008v9n2a393.
- Gebru, B.M., Lee, W.K., Khamzina, A., Lee, S.G. and Negash, E., 2019. Hydrological response of dry afromontane forest to changes in land use and land cover in northern Ethiopia. Remote sensing, 11(16), p.1905. doi: 10.3390/rs11161905.
- Gereslassie, T., Workineh, A., Takele, G., Adem, M. and Berhe, L., 2019. Total volume and aboveground biomass models for Juniperus procera plantation in Wondo Genet, Southern Ethiopia. Open Journal of Forestry, 9(02), p.89. doi: 10.4236/ojf.2019.92004.
- Gibbs, H.K., Brown, S., Niles, J.O. and Foley, J.A., 2007. Monitoring and estimating tropical forest carbon stocks: making REDD a reality. Environmental research letters, 2(4), p.045023. doi: 10.1088/1748-9326/2/4/045023.
- Gidey, K., Eshete, G., Barklund, P., Aertsen, W., Muys, B., 2013. Wood biomass functions for Acacia abyssinica trees and shrubs and implications for provision of ecosystem services in a community managed exclosure in Tigray, Ethiopia. Journal of Arid Environments, 94, pp.80–86. doi: 10.1088/1748-9326/2/4/045023.
- Gregorius, H.R. and Gillet, E.M., 2008. Generalized Simpson-diversity. Ecological Modelling, 211(1-2), pp.90–96. doi: 10.1016/j.ecolmodel.2007.08.026.
- Grytnes, J.A. and Beaman, J.H., 2006. Elevational species richness patterns for vascular plants on Mount Kinabalu, Borneo. Journal of biogeography, 33(10), pp.1838–1849. doi: 10.1111/j.1365-2699.2006.01554.x.
- Gutiérrez, A.G. and Huth, A., 2012. Successional stages of primary temperate rainforests of Chiloé Island, Chile. Perspectives in Plant Ecology, Evolution and Systematics, 14(4), pp.243–256. doi: 10.1016/j.ppees.2012.01.004.
- Hailu, Z., 2002. Ecological impact evaluation of Eucalyptus plantations in comparison with agricultural and grazing land-use types in the Highlands of Ethiopia. Vienna University of Agricultural Sciences, Vienna.
- Hedberg, I., Edwards, S., Sileshi, N. (Eds.), 1995. Flora of Ethiopia and Eritrea, Vol. 4 (1). The National Herbarium Addis Abeba University, Addis Abeba and Department of Systematic Botany, Uppsala University, Uppsala
- Henry, R.C., Palmer, S.C., Watts, K., Mitchell, R.J., Atkinson, N. and Travis, J.M., 2017. Tree loss impacts on ecological connectivity: Developing models for assessment. Ecological Informatics, 42, pp.90–99. doi: 10.1016/j.ecoinf.2017.10.010.
- Jalkanen, A., Mäkipää, R., Ståhl, G., Lehtonen, A. and Petersson, H., 2005. Estimation of the biomass stock of trees in Sweden: comparison of biomass equations and age-dependent biomass expansion factors. Annals of Forest Science, 62(8), pp.845–851. doi: 10.1051/forest:2005075.
- Jia, B., Guo, W., He, J., Sun, M., Chai, L., Liu, J. and Wang, X., 2022. Topography, diversity, and forest structure attributes drive aboveground carbon storage in different forest types in Northeast China. Forests, 13(3), p.455. doi: 10.3390/f13030455.
- Kaushal, S. and Baishya, R., 2021. Stand structure and species diversity regulate biomass carbon stock under major Central Himalayan forest types of India. Ecological Processes, 10, pp.1-18. doi: 10.1186/s13717-021-00283-8.
- Kidane, L., Nemomissa, S. & Bekele, T. (2018). Human-forest interfaces in hugumburda-gratkhassu national forest priority area, north-eastern Ethiopia. Journal of Ethnobiology and Ethnomedicine, 14(1), 1–12. doi: 10.1186/s13002-018-0218-7.
- Kidane, L., Nemomissa, S. and Woldu, Z., 2016. The effects of disturbance on the population structure and regeneration potential of five dominant woody species–in Hugumburda‐Gratkhassu National Forest Priority Area, North‐eastern Ethiopia. African journal of ecology, 54(1), pp.20–28. doi: 10.1111/aje.12254.
- Konopiński, M.K., 2020. Shannon diversity index: a call to replace the original Shannon’s formula with unbiased estimator in the population genetics studies. PeerJ, 8, p.e9391. doi: 10.7717/peerj.9391.
- Körner, C., 2000. Why are there global gradients in species richness? Mountains might hold the answer. Trends in Ecology & Evolution, 15(12), pp.513–514. doi: 10.1016/S0169-5347(00)02004-8.
- Kreft, H. and Jetz, W., 2007. Global patterns and determinants of vascular plant diversity. Proceedings of the National Academy of Sciences, 104(14), pp.5925–5930. doi: 10.1073/pnas.060836110.
- Kremen, C. and Merenlender, A.M., 2018. Landscapes that work for biodiversity and people. Science, 362(6412), p.eaau6020. doi: 10.1126/science.aau602.
- Le Quéré, C., Raupach, M.R., Canadell, J.G., Marland, G., Bopp, L., Ciais, P., Conway, T.J., Doney, S.C., Feely, R.A., Foster, P. and Friedlingstein, P., 2009. Trends in the sources and sinks of carbon dioxide. Nature geoscience, 2(12), pp.831–836. doi: 10.1038/ngeo689.
- Mansourian, S., Vallauri, D., Dudley, N. and Dudley, N., 2005. Impact of forest loss and degradation on biodiversity. Forest Restoration in Landscapes: Beyond Planting Trees, pp.17–21.
- Mazarrasa, I., Marbà, N., Garcia‐Orellana, J., Masqué, P., Arias‐Ortiz, A. and Duarte, C.M., 2017. Effect of environmental factors (wave exposure and depth) and anthropogenic pressure in the C sink capacity of Posidonia oceanica meadows. Limnology and Oceanography, 62(4), pp.1436–1450. doi: 10.1002/lno.10510.
- McDowell, N.G., Allen, C.D., Anderson-Teixeira, K., Aukema, B.H., Bond-Lamberty, B., Chini, L., Clark, J.S., Dietze, M., Grossiord, C., Hanbury-Brown, A. and Hurtt, G.C., 2020. Pervasive shifts in forest dynamics in a changing world. Science, 368(6494), p.eaaz9463. doi: 10.1126/science.aaz94.
- Mebrat, W., Molla, E. and Gashaw, T., 2014. A comparative study of woody plant species diversity at Adey Amba enclosed forest and nearby open site in West Belessa district, northwestern Ethiopia. J. Biol. Agricult. Healthc, 4, p.15.
- Mengistu, T., Teketay, D., Hulten, H. and Yemshaw, Y., 2005. The role of enclosures in the recovery of woody vegetation in degraded dryland hillsides of central and northern Ethiopia. Journal of arid environments, 60(2), pp.259–281. doi: 10.1016/j.jaridenv.2004.03.014.
- Mensah, S., Noulèkoun, F., Dimobe, K., Seifert, T. and Glèlè Kakaï, R., 2023. Climate and soil effects on tree species diversity and aboveground carbon patterns in semi-arid tree savannas. Scientific Reports, 13(1), p.11509. doi: 10.1038/s41598-023-38225-3.
- Mensah, S., Noulèkoun, F., Salako, V.K., Lokossou, C.S., Akouété, P., Seifert, T. and Glèlè Kakaï, R., 2023. Structural and taxonomic diversity predict above‐ground biomass better than functional measures of maximum height in mixed‐species forests. Applied Vegetation Science, 26(2), p.e12732. doi: 10.1111/avsc.12732.
- Messier, C., Bauhus, J., Doyon, F., Maure, F., Sousa-Silva, R., Nolet, P., Mina, M., Aquilué, N., Fortin, M.J. and Puettmann, K., 2019. The functional complex network approach to foster forest resilience to global changes. Forest Ecosystems, 6(1), pp.1–16. doi: 10.1186/s40663-019-0166-2.
- Mokria, M., Gebrekirstos, A., Aynekulu, E. and Braeuning, A., 2015. Tree dieback affects climate change mitigation potential of a dry afromontane forest in northern Ethiopia. Forest Ecology and Management, 344, pp.73–83. doi: 10.1016/j.foreco.2015.02.008.
- Molina-Venegas, R., Fischer, M. and Hemp, A., 2020. Plant evolutionary assembly along elevational belts at Mt. Kilimanjaro: Using phylogenetics to asses biodiversity threats under climate change. Environmental and Experimental Botany, 170, p.103853. doi: 10.1016/j.envexpbot.2019.103853.
- Muluneh, M.G. and Worku, B.B., 2022. Carbon storages and sequestration potentials in remnant forests of different patch sizes in northern Ethiopia: an implication for climate change mitigation. Agriculture & Food Security, 11(1), pp.1–38. doi: 10.1186/s40066-022-00395-0.
- Noulèkoun, F., Birhane, E., Mensah, S., Kassa, H., Berhe, A., Gebremichael, Z.M., Adem, N.M., Seyoum, Y., Mengistu, T., Lemma, B. and Hagazi, N., 2021. Structural diversity consistently mediates species richness effects on aboveground carbon along altitudinal gradients in northern Ethiopian grazing exclosures. Science of the Total Environment, 776, p.145838. doi: 10.1016/j.scitotenv.2021.145838.
- Seastedt, T.R. and Oldfather, M.F., 2021. Climate change, ecosystem processes and biological diversity responses in high elevation communities. Climate, 9(5), p.87. doi: 10.3390/cli9050087.
- Seidl, R., Schelhaas, M.J., Rammer, W. and Verkerk, P.J., 2014. Increasing forest disturbances in Europe and their impact on carbon storage. Nature climate change, 4(9), pp.806–810. doi: 10.1038/nclimate2318.
- Simegn, T.Y., Soromessa, T. and Bayable, E., 2014. Forest carbon stocks in lowland area of Simien Mountains National Park: implication for climate change mitigation. Science, Technology and Arts Research Journal, 3(3), pp.29–36. doi: 10.4314/star.v3i3.5.
- Simpson EH (1949). Measurement of diversity. Nature 163, pp.688:688 doi: 10.1038/163688a0.
- Sintayehu, D.W., Belayneh, A. and Dechassa, N., 2020. Aboveground carbon stock is related to land cover and woody species diversity in tropical ecosystems of Eastern Ethiopia. Ecological Processes, 9(1), pp.1–10. doi: 10.1186/s13717-020-00237-6.
- Slik, J.F., Paoli, G., McGuire, K., Amaral, I., Barroso, J., Bastian, M., Blanc, L., Bongers, F., Boundja, P., Clark, C. and Collins, M., 2013. Large trees drive forest aboveground biomass variation in moist lowland forests across the tropics. Global ecology and biogeography, 22(12), pp.1261–1271. doi: 10.1111/geb.12092.
- Solomon, N., Birhane, E., Tadesse, T., Treydte, A.C. and Meles, K., 2017. Carbon stocks and sequestration potential of dry forests under community management in Tigray, Ethiopia. Ecological processes, 6(1), pp.1–11. doi: 10.1186/s13717-017-0088-2.
- Srinivasarao, C., Lal, R., Kundu, S. and Thakur, P.B., 2015. Conservation agriculture and soil carbon sequestration. Conservation agriculture, pp.479–524. doi: 10.1007/978-3-319-11620-4_19.
- Tang, R., Li, S., Lang, X., Huang, X. and Su, J., 2023. Rare species contribute greater to ecosystem multifunctionality in a subtropical forest than common species due to their functional diversity. Forest Ecology and Management, 538, p.120981. doi: 10.1016/j.foreco.2023.120981.
- Tesfaye, M. and Negash, M., 2018. Combretum-Terminalia vegetation accumulates more carbon stocks in the soil than the biomass along the elevation ranges of dryland ecosystem in Southern Ethiopia. Journal of arid environments, 155, pp.59–64. doi: 10.1016/j.jaridenv.2018.02.004.
- Thom, D., Golivets, M., Edling, L., Meigs, G.W., Gourevitch, J.D., Sonter, L.J., Galford, G.L. and Keeton, W.S., 2019. The climate sensitivity of carbon, timber, and species richness covaries with forest age in boreal–temperate North America. Global Change Biology, 25(7), pp.2446–2458. doi: 10.1111/gcb.14656.
- Ullah, S., Khan, N., Ali, F., Badshah, L., Ali, A. and Muhammad, M., 2020. An ecological assessment of Justicia adhatoda L. Malakand Division, Hindukush range of Pakistan. Bioscience Research, 17, pp.1082–1094.
- Van der Maarel, E., 1979. Transformation of cover-abundance values in phytosociology and its effects on community similarity. Vegetatio, 39, pp.97–114. doi: 10.1007/BF00052021.
- Wani, Z.A., Khan, S., Bhat, J.A., Malik, A.H., Alyas, T., Pant, S., Siddiqui, S., Moustafa, M. and Ahmad, A.E., 2022. Pattern of β-diversity and plant species richness along vertical gradient in Northwest Himalaya, India. Biology, 11(7), p.1064. doi: 10.3390/biology11071064.
- Whittaker, R.H., 1972. Evolution and measurement of species diversity. Taxon, 21(2-3), pp.213–251. doi: 10.2307/1218190.
- Willig, M.R. and Presley, S.J., 2016. Biodiversity and metacommunity structure of animals along altitudinal gradients in tropical montane forests. Journal of Tropical Ecology, 32(5), pp.421–436. doi: 10.1017/S0266467415000589.
- Woldemichael, L.K., Bekele, T. and Nemomissa, S., 2010. Vegetation composition in Hugumbirda-Gratkhassu national forest priority area, South Tigray. Momona Ethiopian Journal of Science, 2(2), pp.27–48. doi: 10.4314/mejs.v2i2.57673.
- Zewdie, M., Olsson, M. and Verwijst, T., 2009. Above-ground biomass production and allometric relations of Eucalyptus globulus Labill. coppice plantations along a chronosequence in the central highlands of Ethiopia. Biomass and bioenergy, 33(3), pp.421–428. doi: 10.1016/j.biombioe.2008.08.007.
- Zhao, S. and Fang, J., 2006. Patterns of species richness for vascular plants in China's nature reserves. Diversity and Distributions, 12(4), pp.364–372. doi: 10.1111/j.1366-9516.2006.00232.x.