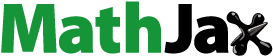
Abstract
Due to global advancement of technology in forest operations, utilization of advanced machineries such as grapple skidder (GS) in timber harvesting has been increasing in the last decades. However, in order to understand their contribution in sustainable harvesting operations, it is important to understand their performance under different operating environment. Therefore, this study aimed to quantify productivity and cost of mechanised skidding operations at Sao Hill Forest plantation (SHFP). Six variables; diameter at a breast height (dbh), tree height, skidding distance, slope, costs, and cycle time (determined using detailed continuous time study) were collected in 120 GS observations.GS productivity and costs were estimated using productive machine hour (PMH) and delays inclusion approach. Regression models were developed using a generalized linear model (GLM) approach. GS productivity under PMH was 2.6% higher than the one including delay time, while skidding costs was 2.1% higher in the approach including delays. This study revealed significant variations (p-value <0.05) in productivity and cost on various terrain classes. At 0 m – 50 m distance, an average delays free GS productivity was 85.5 m3/h, with costs amounting to 1.7 USD/m3. On the distance exceeding 150 m, productivity dropped to 20.1 m3/h, and costs increased to 12.7 USD/m3. Likewise, in 0.0% - 10.0% slope range, average delays free GS productivity and costs was 100 m3/h and 1.5 USD/m3 respectively, while at 20.1% - 30.0% slope range, productivity dropped to 32.6 m3/h and costs raised to 3.9 USD/m3. Skidding distance, slope, and volume per trip were robust predictors of the GS productivity and costs, yielding pseudo-R2 values of 58.1% and 64.3%, respectively. Therefore, this study developed statistical models useful for predicting GS productivity and costs, however, their applications are recommended to be within the ranges of the variables used to develop the models.
Introduction
Forest sector particularly plantation forests form a significant natural resource that is renewable in both economic and environmental sense (Spinelli et al., Citation2020). Therefore, proper management through careful and sustainable utilization in connection with climate change are very essential (Hartsch et al. Citation2021) and are highly encouraged to ensure sustainability to subsequent generations (Visser and Stampfer, Citation2003). This can be achieved by ensuring all key operations including timber harvesting are efficiently planned and managed (Parajuli et al., Citation2020).
To ensure proper planning and management of these operations particularly for timber harvesting so as to meet expected timber quality and demand (Jonsson et al., Citation2023), it is essential to consider various factors which limits their productivity and cost. Among others include; species type, age, stand density, timber size, bunching strategy, learning curve of the crew (Varch et al. Citation2021), site conditions (slope, soil, obstacles), available machine types, harvesting prescriptions, intended final products (Akay, Citation1998; Behjou, Citation2018; Jiroušek et al., Citation2007; Kluender and Stokes, Citation1996; Mizaras et al., Citation2008; Parajuli et al., Citation2020), training and motivation level to forest workers (Šporčić et al., Citation2023).
With the advancement of technology (i.e., due to widespread availability of information technologies (IT) (Llorente et al. 2023; Spinelli et al. Citation2019), increase in timber demand driven by urbanization and population growth, increase in safety level (Obi and Visser Citation2020), as well as intentions for costs reduction (Varch et al. Citation2021), logging operations have gradually shifted toward full mechanization (Mizaras et al., Citation2008). This transition has been facilitated by the introduction of highly efficient machines such as farm tractors, feller bunchers, and skidders (Bavaghar et al., Citation2010; Borz et al., Citation2015; Gölci et al., Citation2018; Orlovský et al., Citation2020). These machines are known for their significant production capacity and cost-effectiveness when properly planned. This had driven various researchers across the world to see the importance of analysing productivity and costs of these machines based on real-world scenarios to enhance its proper utilization to its fully capacity. Various techniques, including gross time study, detailed time study (Borz et al., Citation2013) and regression analysis such as Ordinary Least Square (OLS) have been widely applied in global research (Akay et al., Citation2004a) to estimate time consumption, productivity (Jonsson et al. Citation2023) and determine the impact of environmental, stand, economic, and human factors on machinery productivity and costs (Orlovský et al., Citation2020; Proto et al., Citation2018). For instance, studies by Ghaffariyan (Citation2020a), Kulak et al. (Citation2017), Sabo and Poršinsky (Citation2005), and Stoilov et al. (Citation2021) applied multiple linear regression analysis on predicting GS time consumption, productivity and costs. Under all reviewed studies, the variables; number of logs, slope, skidding distance, bunching distance and volume per turn were observed to be significant (p-value < 0.05). Moreover, study by Borz et al. (Citation2013) applied Stepwise backward regression techniques which normally involved combining all possible independent variables in the model at the beginning, followed by redundancy of insignificant variables at the end in order to bring highly efficient predictive models.
Although OLS have gained popularity in the field of forest management, its applications is limited by a number of assumptions (Liski et al., Citation2020), consequently, log and back transformation have always been used to account for the assumptions related with linearity and normality. In light of these limitations, a generalized linear model (GLM) has emerged as a valuable extension to the regression model (Zhang et al., Citation2020). Unlike traditional methods, the GLM can estimate machinery productivity more flexibly without requiring data transformation. It offers a better fit to the original data, handles collinear variables, and facilitates formulation of highly precise models for accurate estimations of the machinery performance in the field of forest operations (Orlovský et al., Citation2020).
Despite their predictive usefulness, models for estimating productivity and costs in mechanized timber harvesting operations are limited particularly for the studies conducted in the Sub-Saharan Africa, including Tanzania. Furthermore, productivity and cost of timber harvesting machineries are mostly influenced by environmental factors, stand characteristics, and machine-specific attributes which are highly contingent upon the specific locality and the machine itself. Consequently, there is a pressing need for further research to quantify and develop predictive models that can provide most accurately localized information for future predictions.
In Tanzania mechanized timber harvesting operations begin since early 2000s through integration of various harvesting systems such as; short wood, tree length and whole tree system using various machines such as; feller buncher, cable skidder, GS, farm tractor and three wheeled loaders. Various companies, including Mufindi Paper Mill (MPM), Green Resources Company Ltd. (GRL), and Tanzania Wattle Company (TANWAT) which are situated in the southern highlands, own a majority of these machines (Mauya et al., Citation2011). Despite of their known production capability, accurate machinery productivity and costs estimations still remain challenging due to largeness of SHFP, its diverse terrain and stand attributes. Therefore, this study addresses this knowledge gap by (i) quantify the productivity and costs of GS using the whole tree harvesting system, (ii) develop models that encompass GS time consumption, productivity, and costs at SHFP (iii) predicting GS productivity and costs across different operating terrain conditions at SHFP using the developed models. It is anticipated that the results from this study will serve as a valuable guide (Akay et al., Citation2004b; Spinelli et al., Citation2002) to logging managers, contractors, and forest owners (Miyajima et al., 2021) in accurately estimating and efficiently utilizing harvesting machinery in diverse locations (Ackerman et al., Citation2018; Borz et al., Citation2015; Gilanipoor et al., Citation2012).
Materials and methods
Study area description
This study was conducted at SHFP located in the Southern highland of Tanzania, Iringa region, Mufindi District. The plantation lies between 8° 18′ S to 8° 33′ S latitudes and 35° 06′ E to 35° 20′ E longitudes ().
The plantation is about 18km from Mafinga town with estimated total area of 135,903 ha. Due to its largeness, currently the plantation is administratively segmented into four blocks/divisions namely: Irundi, Ihefu, Ihalimba and Mgololo (MNRT, Citation2018). Each division is broken into compartments differentiated by species, planting date and site class. Generally, the plantation is composed of exotic softwood tree species of Pinus patula, Pinus elliottii, Pinus caribeae, and Cupressus lusitanica, together with hard wood species of Eucalyptus maidenii, Eucalyptus saligna and Eucalyptus camaldulensis. Also, the plantation comprises small patches of natural vegetation characterized by the mosaic of open grassland with scattered indigenous tree species of Erythrina abyssinica, Parinari curatellifolia, Apodytes dimidiata and Albizia petersiana.
Topography and Climate
The plantation is on rolling terrain interacting with some low hills and wide flat-bottomed valleys with an altitude ranging from 1,700m to 2,000 m above sea level. The soils of the area are acidic deep soil with a pH range from 4.4 to 5.4. The climate is characterized by unimodal rainfall pattern starting from November to April and a dry season from May to late October. The mean annual rainfall is 1,300 mm with a range of 725 mm to 1,400 mm. The temperatures are fairly cool, with the minimum monthly temperature range between 10 °C to 18 °C while the maximum range is between 23 °C to 28 °C (MNRT, Citation2018).
The study focused on mechanized timber skidding operations using the whole tree system. Harvesting operations in the study area involves clear-cutting, utilizing both semi-mechanized and mechanized approaches. Mechanized operations employ machinery like feller bunchers, farm tractors with wire rope winches, cable and grapple skidders. Semi-mechanized operations primarily rely on chainsaws and farm tractors. The main harvesting systems employed are cut-to-length, tree-length, and whole tree systems.
Sampling procedures
The study employed two phase sampling. On the first phase, forest compartment for the study was obtained with the aid of SHFP management plan in order to avoid destruction of immature forest stands. Moreover, since our study focused on ground skidding operation, terrain variables were considered as one of the factors that influence the performance of the GS. Therefore, forest compartment named ILASA 3/9 with heterogeneous terrain was selected with the aid of digital elevation model (DEM) with 30m x 30m resolution downloaded from https://earthexplorer.usgs.gov/. A total of 30 square plots of 15 m x 15 m were randomly distributed throughout the compartment to capture all ranges of terrain (). On second phase, 120 GS work cycle with respective work elements was determined using the formula by Murphy, (Citation2005) as indicated in Equationequation 1(1)
(1) . Whereby, 10 pilot time study was conducted, giving an average cycle time (i.e., mean WCT) of 6.873 minutes, Variance cycle time (i.e., Var WCT) of 0.071 minutes and 0.95 precision level (E) was used.
Where; n = number of work cycles to be studied, t = Student’s t-value, Var (WCT) = Variance of the work cycle time, E = Level of precision desired, = Mean work cycle time (minutes).
Machinery description
The machinery used under this study was CAT 525 grapple skidder, purchased by MPM in 2018. It is American manufactured caterpillar brand with the following characteristics available in www.ritchiespecs.com as presented in .
Table 1. GS descriptions
Data collection
GS cycle time (minutes), individual tree variables (dbh and height), terrain variables (skidding distance and slope) and costs variables were collected. The details for each collected variable are described below.
GS cycle time
GS productive time (PMH) which refers to the time spent by the skidder to perform a given task at the workplace (Magagnotti et al., Citation2013) was recorded through detailed continuous time study technique using stopwatch. The GS cycle time was segmented into five work elements namely: travel empty (TE), positioning (PS), grappling (GL), travel loaded (TL) and unloading (UNL). Also, necessary delays (inevitable interruption due to the nature of the work and the environment), as well as unnecessary delays (the wastage of time which can be eliminated by improving supervision and training to workers) (Mauya Citation2022), were recorded on each work element of the GS once they occurred. Time recording started at the beginning of a work cycle and the stopwatch was paused at the end of the cycle. Elapsed time was read directly from the stopwatch and recorded on the prepared field forms.
Tree variables
Before the beginning of skidding operations, all standing trees within 15m x 15m square plots were labelled by numbers. Thereafter, dbh and tree height for each tree were measured using calliper and vertex hypsometer respectively. Since skidding operation was performed using whole tree harvesting system (i.e., with branches and tops intact to the main trunk), the volume of each tree was estimated using the allometric single tree equation (EquationEquation 2(2)
(2) ) by Malimbwi et al., (Citation2016). The model applied is compatible with Pinus patula grown in the study area.
(2)
(2)
Terrain variables
Skidding distance (m) and slope (%) were the two-terrain variables recorded during skidding operation. Skidding distance from the stump site to the landing was measured using measuring tape, while slope was measured using vertex hypsometer.
GS costs variables
GS operational (i.e., fixed and variable) costs information was collected by interviewing logging supervisor and procurements personnel from Mufindi Paper Mill. Simply, fixed (standing or capital) costs are the ones that need to be recovered by machine irrespective of the amount of work a machine does or the revenue it earns and are associated only with owning the machine. Normally includes: depreciation, interest, insurance, and taxes (Ackerman et al., Citation2014). On the other hand, variable (running) costs are the ones incurred when the machine is working, travelling empty, or when the engine is running (Bjorheden and Thompson, Citation1995). They normally vary directly with the level of output produced by the machine. It includes costs for labour, fuel, lubricants, machine maintenance and tires. (Ackerman et al., Citation2014; Nwokoye and Ilechukwu, Citation2018).
Data analysis
GS productivity (m3/h) was computed using two approaches; with inclusion and exclusion of necessary delays i.e., Equationequations 3(3)
(3) and Equation5
(5)
(5) respectively.
(3)
(3)
Where: PD is the GS productivity (m3/h) per work cycle (including necessary delay), Tvol is skidded tree volume (m3) per work cycle, 60 is the number of minutes per a given workplace hour and T is total GS productive time (minutes) per work cycle, F is the proportion of productive time.
(4)
(4)
Where D is Delay time expressed as a percentage of workplace time in minutes.
On the other hand, GS productivity without delay time P(PMH) was computed using the formula adapted in the studies by Mauya (Citation2022), and Miyajima et al., (Citation2021a) EquationEquation 5(5)
(5) .
(5)
(5)
Where: P is the GS productivity (m3/h), 60 is the conversion factor for converting minutes to hours and PMH is the productive machine hour in minutes.
Lastly, paired t-test was performed to test if there is a significant difference between GS productivity with delay and without delay time.
All GS cost components (EquationEquations 6(6)
(6) to Equation16
(16)
(16) ) were computed based on the formulas described by Sessions, (Citation2007). The machine (i.e., GS) under study was CAT brand, with registration number T.809 DNB, having 175 horse power and it was purchased in the year 2018.The machinery delivered costs was 304,117.4 USD and has saved over a period of six years up to the present study. Furthermore, the machinery annual tax was amounting to 24,472.3 USD/year, with zero interest rate since the machine was purchased cash. The average working time was 3506 h/year, with the labour (operator’s) costs amounting to 0.56 USD/h.
Fixed costs
Depreciation
(6)
(6)
Insurance
(7)
(7)
Interest (i)
(8)
(8)
Taxes (Tc)
(9)
(9)
Variable costs
Labor costs (Lc)
(10)
(10) Where; f = Fringe benefits expressed as % of direct labor costs (i.e., 0.5% in this case).
Fuel cost (Fc)
(11)
(11) Where: GHP = Gross engine horsepower (i.e., 175 HP in this case), CL = Fuel price per liter (i.e., 1.236 USD/liter in this case), X = 0.12 for diesel and 0.175 for the gasoline engine.
Lubricants costs (Oil and grease)
(12)
(12) Where; GHP = Gross engine horsepower (i.e., 175 HP in this case), and X = 0.20 for tractors, skidders, front end loaders and trucks, X = 0.30 for feller bunchers and knuckle boom loaders, X = 0.50 for processors, harvesters and forwarders.
Maintenance costs (Mc)
(13)
(13)
Tires costs (Ty)
(14)
(14) Where; CST = Price of a set of the replaced tires (i.e., 2,606.7 USD in this case).
Hourly skidding costs (USD/h)
Statistical analysis
Model development
Previous studies (e.g. Conrad et al., Citation2013, Long, Citation2003, Wang et al., Citation2004, Citation2005) were used as the baseline for developing GS time consumption, productivity and costs models for this study. The normality of the data set was tested using Shapiro wilk test whereby variables with a p-value less than 0.05 were considered to be not normally distributed (Yap and Sim, Citation2011; Korkmaz et al., Citation2014). Modelling was performed using a GLM approach (EquationEquation 17(17)
(17) ) in R-statistical software since the GLMs provide greater flexibility in analysing data even those related to non-normal distributions (Ravindra et al., Citation2019). Due to the nature of the data being continuous, the Gaussian family was employed to ensure error terms are equally distributed throughout the given data set. Predictor variables for each model were further examined using variance inflation factor (VIF) to test for multicollinearity. Variables with VIF>5 were excluded from the model, indicating a sign of multicollinearity (Shabani et al., Citation2021).
(17)
(17)
Where: g(µi) = ni is a smooth and invertible linearizing link function g (·), which transforms the expectation of the response variable, μi = E(Yi), to the linear predictor, α is the model intercept while “β1 xi1 + β2 x i2 +….βk xi k” are independent variables which represent the change in the dependent variable associated with a one-unit change in each independent variable (Lindsey, Citation1998; Nelder and Wedderburn, Citation1972).
Finally, pseudo-R-squares (EquationEquation 18(18)
(18) ) and residual plots were used to assess the goodness of fit for the developed model.
(18)
(18)
Model validation
For the precision and accuracy of the developed model to be known, model validation is inevitable (James et al., Citation2013; Jimmy et al., Citation2013; Mauya, Citation2022). Based on the available data set (i.e.,120 GS observations), were segmented into ten-folds randomly. One subset was held out for checking the model performance while the model is trained on the remaining subsets (James et al., Citation2013). Then residual root mean square error (RMSEr) as an indicator for assessing model quality was determined using its predicted values (EquationEquations 19(19)
(19) and 20).
(19)
(19)
(20)
(20)
Where: the sum of all observations from i = 1 to i = n, yi and ŷ denote observed and predicted variables for both time consumption, productivity and unit skidding costs in a given ith observation respectively.
y̅ denotes the mean for observed time consumption, productivity and units skidding costs for all GS cycles, n is the total number of observations in the dataset.
Results
GS summary statistics
A total of 120 GS work cycles were observed for the entire study. The effective GS workplace time (PMH) ranged from 0.593 minutes at a minimum skidding distance of 14.2 m and slope of 0.4% to 11.878 minutes at a maximum skidding distance of 220 m and slope of 26.0%. The average GS time was 4.519 minutes under PMH approach and 4.625 minutes when including necessary delays. The most GS time-consuming work element was travel loaded (TL) which consumed a substantial part of total skidding time for about 47.9%, while the least work element was unloading (). The summary for all variables is presented in .
Figure 3. GS time distribution for each work element at a mean distance of 59.2 m and slope of 13.5%.

Table 2. Skidding summary statistics
GS productivity and costs
GS productivity for both productive machine hour (PMH) and when including necessary delays, were 67.5 m3/h and 64.1 m3/h respectively. Individual cost items and unit skidding costs for both approaches are presented in . Furthermore, the performed paired t-test indicated a significant difference between productivity and costs while using the effective time (PMH) and when including necessary delays (p-value < 0.05).
Table 3. GS operational costs
GS time consumption, productivity and costs models
Time consumption models
The explanatory (independent) and response (dependent) variables vary between models and their number ranged from one to three. The parameter estimates for all models except unloading were significantly different from zero (p <0.05) and the VIF values were <5 indicating an acceptable level of multicollinearity. For the case of time consumption, model for predicting machine productive hours (PMH) and individual work elements i.e., travel empty, positioning, grappling, travel loaded and unloading were developed (). Pseudo-R-square value which explains the goodness of fit of the model varied among productive work elements. The highest pseudo-R-squared was observed in the total PMH model (81.5%), while the least pseudo-R-squared was observed in unloading (UNL) work element (9.4%). The performance of each model was further assessed through residual plots ().
Table 4. GS time consumption model for individual work elements and total productive time.
Cross-validation results showed that the RMSEr value for the total productive time model was relatively smaller as compared to individual work elements. However, the scatter plots for positioning and unloading time had shown a behaviour of over-prediction since some of the scatter points deviate far from the average value ().
Productivity and costs models
For both GS productivity and unit skidding costs, the variables skidding distance (m) and slope (%) appeared to be good predictors for estimating machine productivity and costs. The pseudo-R-squared for productivity and costs models (EquationEquations 21(21)
(21) and Equation22
(22)
(22) ) were 58.1% and 64.3% respectively indicating a good fit for both models. The residual plots for both models are normally distributed, with fewer highly deviated scatter points, which are normally caused by the few observations with higher values ().
(21)
(21)
(22)
(22)
The cross-validation results indicated that RMSEr value for the GS productivity and unit skidding cots models were 49.3% and 50.4% respectively. Furthermore, RMSEr were observed to vary across terrain classes. Both productivity and costs models showed lowest RMSEr value at a distance and slope class of 0.00-50.0 m and 0.00 – 10.0% respectively, while the highest RMSEr value was observed at the highest distance and slope class (). This implies that skidding distance and slope were the main predictors for the GS productivity and costs ().
Figure 7. a. Error bars show the performance of productivity and cost models on various distances.
b. Error bars show the performance of productivity and cost models on various slopes.

Table 5. Performance of productivity and cost models on various distances and slope classes.
Discussion
This study aimed to quantify and develop predictive models for the GS time consumption, productivity, and cost using the whole tree harvesting system. The objective was to establish baseline information to accurately estimate and plan mechanized timber harvesting in Tanzania plantation forests and other geographical areas which bare similar environmental and stand characteristics. Regression and correlation analyses were employed to evaluate the influence of machine, environmental, and stand characteristics on GS performance.
The results revealed that the average PMH for the GS was 4.519 and 4.625 minutes, inclusive of necessary delays. The study also identified travel loaded (TL) as the most time-consuming work element, with an average duration of 2.165 minutes, followed by travel empty (TE) i.e., 1.308 minutes. Conversely, unloading time was found to be the least time-consuming element, lasting for only 0.084 minutes. Previous studies by Orlovský et al. (Citation2020) and Borz et al. (Citation2015) also highlighted travel loaded as the mostly time-consuming work element for the GS. The GS time in our study varies with the one reported by Mauya et al. (Citation2011) who conducted similar study in the same forest plantation using John Deere 648G (i.e., 4.8 minutes). By comparing similar aspects which was assessed in both studies, such variations are probably due to the type of machine used, terrain condition and operator experience. This can be justified by higher delay time in the study by Mauya et al. (Citation2011) which took 20.4% of the entire GS productive time mainly due to presence of obstacles in the skidding trails and poor grappling of logs, compared with the one reported in our study (which took only 2.3% of the GS productive time).
The average GS productivity and costs under PHM approach (i.e., 67.5 m3/h and 3.363 USD/m3 respectively) was 2.6% higher than the one including delays, while costs under PMH was 2.1% lower than the one including delays. This was further justified by the results from the paired t-test which indicated that there was statistically significant differences (p-value < 0.05) of productivity and costs between the two approaches. This implies that, through ensuring effective supervision, we can minimise operational delays, improving GS productivity by 2.6% and reduce the skidding operational costs by 2.1%. The GS productivity and costs values under this study fall within the range reported in other studies. For example, Ghaffariyan (Citation2020a) and Klepac and Mitchell (Citation2016) reviewed skidder productivity in coniferous forest plantations and reported a productivity range of 9.3 m3/h to 78 m3/hr. Dodson et al. (Citation2006) reported an average GS productivity of 88.7 m3/h when using the whole tree system in Central Oregon for Juniperus occidentalis forest stands. Adebayo et al. (Citation2007) reported an average productivity of 58.3 m3/h for Cat D-518 GS in northern Idaho, with an average skidding distance of 130 m and slope ranging from 3% to 34%. These studies revealed that average skidded volume per trip, extraction distance, and slope are major factors influencing GS productivity and costs. For instance, Ghaffariyan (Citation2020b) reported lower GS productivity on steep slopes (25% to 40%), ranging from 20 m3/h to 48 m3/h. Additionally, Dodson et al. (Citation2006) reported a unit skidding cost of 1.52 USD/m3 using the conventional timber harvesting system. Furthermore, Akay et al. (Citation2004) reported an average productivity and costs of 19.2 m3/h and 15.35 USD/m3 using CAT 525 GS in Turkish forests when harvesting cedars, pines and firs tree species. The average load volume per turn was 0.68 m3 and the mean slope was 31%. Kulak et al. (Citation2017) reported the GS productivity range of 8 m3/h to 14 m3/h in Pinus sylvestris, southern Poland using John Deere 548G-III in a skidding distance ranged between 124 m and 246 m, and the load volume per turn ranged from 1.86 m3 to 2.95 m3. Moreover, study by Ngulube, (Citation2012) reported an average GS productivity of 21.5 m3/h when using CAT 525C grapple skidder and whole tree harvesting system. The reported terrain slope under this study varied between 5% and 25%, with an average of 11.5%, while the average load volume and skidding distance was 1.45 m3 and 134 m respectively. However, most of these studies were conducted in the geographical regions outside Tanzania and therefore, comparison was aimed on getting the global overview trend of GS productivity and costs. But to our understanding this study is one among the few studies conducted in the plantation forests of Tanzania and the first study to explore the productivity and costs of GS when skidding using whole tree harvesting system, and predicting GS performance using GLM approach.
To enhance applications of GS in the areas with similar terrain and stand characteristics, statistical models for predicting GS time consumption, productivity and costs were developed. The results showed that, all selected predictor variables for the GS time consumption, productivity, and costs predictions were significant (p-value < 0.05), except for unloading (UNL).
The performance of the time consumption models for the different GS work elements was assessed using pseudo-R-square and RMSEr value. The highest pseudo-R-square value (i.e., 81.5%) was observed in the GS total productive time, while the lowest pseudo-R-square value (i.e., 9.4%) was observed in unloading (UNL). The residual plot for unloading showed a funnel pattern, likely due to fewer observations having higher deviation from the average value. A higher pseudo-R-square (i.e., 81.5%) indicated a better fit of the predictor variables in the model, meaning that selected variables; skidding distance, slope, and average tree dbh per trip were the good predictors of the GS skidding time. This is in line with the previous studies by Hiesl (Citation2013), Kopseak et al. (Citation2021), Ngulube et al. (Citation2014), and Vitorelo (Citation2011) which also highlighted skidding distance, slope, and load volume as the main predictors of GS cycle time (p-value < 0.05). For the productivity and costs models, the pseudo-R-square values were 58.1% and 64.3%, respectively, falling within the range reported in other studies e.g., (Visser and Stampfer Citation2003) reported a GS productivity model with an R-square value of 62%, significantly impacted by skidding distance and volume per load (p-value < 0.05). To further explore the performance of the models, K-fold cross validation was used to test the reliability of the models. The results revealed that prediction accuracy of the model decreased as the terrain variables (i.e., slope and distance) increase (). Higher GS productivity and costs prediction accuracy (i.e., RMSEr = 18.8% and RMSEr = 17.1%) were observed in a distance and slope class of 0.0 – 50 m and 0.0 – 10.0% respectively, while lower prediction accuracy was found at a higher distance and slope class (p-value < 0.05). Moreover, slight variation in RMSEr value was observed in all slope classes as compared to higher variation among distance classes. That is due to lower variation (Çalişkan, Citation2019) in the range of the measured filed slope variable (i.e., 13 ± 7.9%) compared to field measured distance (i.e., 59 ± 36 m). Moreover, model’s prediction accuracy in this study may be influenced by other factors which were beyond the scope of this study, including modelling techniques. For instance, study by Munis et al. (Citation2023) showed that other machine learning techniques such as Random Forest has higher prediction accuracy (i.e., RMSE = 18.37 ± 13.10) compared to Linear regression model (i.e., RMSE = 28.82 ± 12.88) when modelling forwarder performance in Brazilian Pinus and Eucalyptus planted forests.
Generally, the findings of this study generated a baseline information in operationalisation of the GS in Tanzania forest plantation, of which such information (i.e., skidding using whole tree harvesting system) was not present before. Moreover, further studies on heterogeneous landscape with more terrain variation are recommended to explore its influence on GS performance. Also, other modelling techniques such as machine learning are encouraged to assess their prediction accuracy on GS performance. Lastly, this study was conducted during the dry season. Hence, these models will be appropriate once machine work on the area bearing similar spatial and temporal characteristics. Further studies of the GS performance on wet condition are also recommended.
Conclusion
The study examined the productivity and costs associated with GS in two different approaches: using PMH and including delay time. The developed models for predicting GS time consumption, productivity, and costs using whole tree conventional timber harvesting system, indicated that variables; distance, slope, average tree dbh, and volume per trip were the significant predictors of the GS performance. These findings provide valuable guidelines to planners for an effective resources and facilities allocation, enabling the fulfilment of timber demand within targeted timelines and maximizing profit. Moreover, the study's findings have broader implications to forest plantations with similar environmental and stand characteristics. The insights gained can contribute to better planning of future harvesting operations, ensuring improved efficiency and productivity of the GS. Further studies are encouraged to explore potentials of other modelling techniques on GS time consumption, productivity and costs predictions.
Author Contributions
Conceptualization: Gilberth Prosper Temba, Ernest William Mauya, George Ansigar Migunga.
Data analysis: Gilberth Prosper Temba.
Methodology: Gilberth Prosper Temba, Ernest William Mauya.
Supervision: Ernest William Mauya, George Ansigar Migunga.
Writing – original draft: Gilberth Prosper Temba.
Writing – review and editing: Gilberth Prosper Temba, Ernest William Mauya, George Ansigar Migunga.
Acknowledgments
We acknowledge the project titled “Higher Education for Economic Transformation (HEET)”, under Sokoine University of Agriculture and Tanzania Forest Fund (TaFF), for providing financial support during data collection.
Additional information
Funding
References
- Ackerman, P., Belbo, H., Eliasson, L., de Jong, A., Lazdins, A., & Lyons, J. (2014). The Cost model for calculation of forest operations costs. International Journal of Forest Engineering, 25(1), 75–81. doi: 10.1080/14942119.2014.903711.
- Ackerman, P., Martin, C., Brewer, J., & Ackerman, S. (2018). Effect of slope on productivity and cost of Eucalyptus pulpwood harvesting using single-grip purpose-built and excavator-based harvesters. International Journal of Forest Engineering, 29(2), 74–82. doi: 10.1080/14942119.2018.1431491.
- Adebayo, A. B., Han, H.-S., & Leonard Johnson. (2007). Productivity and costs of Cut-to-length and whole tree harvesting in a mixed-conifer stand. Forest Products Journal, 57(6), 11.
- Akay, A. E. (1998). Estimating machine rates and production for selected forest harvesting machines operating in the western united states and determining the most economical machine combinations under representative conditions in Turkey. Oregon State University.
- Akay, A. E., Erdas, O., & Sessions, J. (2004). Determining Productivity of Mechanized Hravesting Machines. Journal of Applied Sciences, 4(1), 5. doi: 10.3923/jas.2004.100.105.
- Bavaghar, M. P., Sobhani, H., Feghhi, J., Darvishsefat, A. A., & Marvi Mohajer, M. R. (2010). Comprehensive Productivity Models for Tracked and Wheeled Skidders in the Hyrcanian Forests of Iran. Research Journal of Forestry, 4(2), 8. doi: 10.3923/rjf.2010.65.71.
- Behjou, F. K. (2018). Shape of skidder productivity function for ground based skidding system in Caspian forests. Forestry Research and Engineering: International Journal, 2(1), 19–22. doi: 10.15406/freij.2018.02.00020.
- Bjorheden, R., & Thompson, M. a. (1995). An international nomenclature for forest work study. IUFRO 1995, 20th World Congress; S3:04 Subject Area, Misc. Rep., 190–215. http://www.treesearch.fs.fed.us/pubs/15500 access date: july 16, 2008
- Borz, S. A., Dinulicǎ, F., Bîrda, M., Ignea, G., Ciobanu, V. D., & Popa, B. (2013). Time consumption and productivity of skidding Silver fir (Abies alba Mill.) round wood in reduced accessibility conditions: A case study in windthrow salvage logging form Romanian Carpathians. Annals of Forest Research, 56(2), 363–375.
- Borz, S. A., Ignea, G., Popa, B., Iordache, E., & Spârchez, G. (2015). Estimating time consumption and productivity of roundwood skidding in group shelterwood system – a case study in a broadleaved mixed stand located in reduced accessibility conditions. Croatian Journal of Forest Engineering, 36(1), 137–146.
- Çalişkan, E. (2019). Application of artificial neural networks and particle swarm optimization for timber extraction with cable crane. Applied Ecology and Environmental Research, 17(2), 2339–2355. doi: 10.15666/aeer/1702_23392355.
- Conrad, J. L., Bolding, M. C., Aust, W. M., Smith, R. L., & Horcher, A. (2013). Harvesting productivity and costs when utilizing energywood from pine plantations of the southern Coastal Plain USA. Biomass and Bioenergy, 52, 85–95. doi: 10.1016/j.biombioe.2013.02.038.
- Dodson, E. M., Deboodt, T., & Hudspeth, G. (2006). Production, cost, and soil compaction estimates for two western juniper extraction systems. Western Journal of Applied Forestry, 21(4), 185–194. doi: 10.1093/wjaf/21.4.185.
- Ghaffariyan, M. R. (2020a). General productivity predicting model for skidder working in eucalypt plantations. European Journal of Forest Engineering, 6(1), 1–6. doi: 10.33904/ejfe.618344.
- Ghaffariyan, M. R. (2020b). Reviewing productivity studies of skidders working in coniferous forests and plantations. Silva Balcanica, 21(2), 83–98. doi: 10.3897/silvabalcanica.21.e56071.
- Gilanipoor, N., Najafi, A., & Alvaezin, S. M. H. (2012). Productivity and cost of farm tractor skidding. Journal of Forest Science, 58(1), 21–26. doi: 10.17221/4804-JFS.
- Gölci, S., Böyöksakall, H., Ta, N., & Akay, A. E. (2018). Productivity Analysis of Timber Skidding Operation with Farm Tractor. European Journal of Forest Engineering, 4(1), 26–32. doi: 10.33904/ejfe.428397.
- Hartsch, F., Kemmerer, J., Labelle, E. R., Jaeger, D., & Wagner, T. (2021). Integration of harvester production data in German wood supply chains: Legal, social and economic requirements. Forests, 12(4), 1–16. doi: 10.3390/f12040460.
- Hiesl, P. (2013). Productivity Standards for Whole-Tree and Cut- To-Length Harvesting Systems in Maine. 167.
- James, G., Witten, D., Tibshirani, R., & Hastie, T. (2013). An Introduction to Statistical Learning with Applications in R (Springer. (ed.)). Springer.
- Jimmy, G., Seiler, R., & Maeder, U. (2013). Development and Validation of Energy Expenditure Prediction Models Based on GT3X Accelerometer Data in 5- to 9-Year-Old Children. Journal of Physical Activity and Healt, 10, 1057–1067. doi: 10.1123/jpah.10.7.1057.
- Jiroušek, R., Klvač, R., & Skoupý, A. (2007). Productivity and costs of the mechanised cut-to-length wood harvesting system in clear-felling operations. Journal of Forest Science, 53(10), 476–482. doi: 10.17221/2088-JFS.
- Jonsson, R., Rönnqvist, M., Flisberg, P., Jönsson, P., & Lindroos, O. (2023). Comparison of modeling approaches for evaluation of machine fleets in central Sweden forest operations. International Journal of Forest Engineering, 34(1), 42–53. doi: 10.1080/14942119.2022.2102346.
- Klepac, J., & Mitchell, D. (2016). Comparison of Four Harvesting Systems in a Loblolly Pine Plantation. Professional Agricultural Workers Journal, 4(1), 14. http://tuspubs.tuskegee.edu/pawj/vol4/iss1/9
- Kluender, R. A., & Stokes, B. J. (1996). Felling and Skidding Productivity and Harvesting Cost in Souttiet Pine Forest. Joint Conference Canadian Woodlands Forum: Canadian Pulp and Paper Association and International Union of Forest Research Organizations, 3423, 6.
- Kopseak, H., Šušnjar, M., Bačić, M., Šporčić, M., & Pandur, Z. (2021). Skidders fuel consumption in two different working regions and types of forest management. Forests, 12(5). doi: 10.3390/f12050547.
- Korkmaz, S., Goksuluk, D., & Zararsiz, G. (2014). MVN: An R package for assessing multivariate normality. R Journal, 6(2), 151–162. doi: 10.32614/RJ-2014-031.
- Kulak, D., Stańczykiewicz, A., & Szewczyk, G. (2017). Productivity and time consumption of timber extraction with a grapple skidder in selected pine stands. Croatian Journal of Forest Engineering, 38(1), 55–63.
- Lindsey, J. K. (1998). Applying Generalized Linear Models. In Technometrics (Vol. 40, Issue 2). Springer. doi: 10.2307/1270654.
- Liski, E., Jounela, P., Korpunen, H., Sosa, A., Lindroos, O., & Jylhä, P. (2020). Modeling the productivity of mechanized CTL harvesting with statistical machine learning methods. International Journal of Forest Engineering, 31(3), 253–262. doi: 10.1080/14942119.2020.1820750.
- Long, C. R. (2003). Production and cost analysis of two harvesting systems in central Appalachia,West Virginia University. https://researchrepository.wvu.edu/etd/1327/
- Magagnotti, N., Kanzian, C., Schulmeyer, F., & Spinelli, R. (2013). A new guide for work studies in forestry. International Journal of Forest Engineering, 24(3), 249–253. doi: 10.1080/14942119.2013.856613.
- Malimbwi, R. E., Mugasha, W. A., & Mauya, E. (2016). Pinus Patula Yield Tables for Sao Hill Forest Plantations, Tanzania. Department of Forest Mensuration and Management.Sokoine University of Agriculture, Morogoro.
- Mauya, E. W. (2022). Production Rates of Mechanized Tree Felling Operations at Sao-Hill Forest Plantation, Tanzania. Tanzania Journal of Forestry and Nature Conservation, Vol 91(No. 1), 45–57.
- Mauya, E. W., Kweka, A. E., Migunga, G. A., & Silayo, D. A. (2011). Productivity and cost analysis of grapple skidder at Sao Hill Forest Plantations, Tanzania. Tanzania Journal of Forestry and Nature Conservation, 81(1), 10–19.
- Miyajima, R. H., Fenner, P. T., Batistela, G. C., & Simões, D. (2021a). Effect of feller-buncher model, slope class and cutting area on the productivity and costs of whole tree harvesting in Brazilian eucalyptus stands. Juornal of Forests, 12(8), 14. doi: 10.3390/f12081092.
- Miyajima, R. H., Fenner, P. T., Batistela, G. C., & Simões, D. (2021b). Effect of feller-buncher model, slope class and cutting area on the productivity and costs of whole tree harvesting in Brazilian eucalyptus stands. Forests, 12(8). doi: 10.3390/f12081092.
- Mizaras, S., Sadauskiene, L., & Mizaraite, D. (2008). Productivity of harvesting machines and costs of mechanized wood harvesting: Lithuanian case study. Baltic Forestry, 14(2), 155–162.
- MNRT (Ministry of Natural Resource and Tourism). (2018). Sao Hill Division 1 (Irundi) Forest Plantation management plan (2018/19-2022/23) (Vol. 1). Tanzania Forest Services, Ministry of Natural Resources and Tourism, Dodoma, Tanzania. 108pp.
- Munis, R. A., Almeida, R. O., Camargo, D. A., Barbosa, R., Wojciechowski, J., & Sim, D. (2023). Tactical Forwarder Planning : A Data-Driven Approach for Timber Forwarding. Forests, 14(1782), 11. doi: 10.3390/f14091782.
- Murphy, G. E. (2005). Determining sample size for harvesting cost estimation. New Zealand Journal of Forestry Science, 35(2/3), 166–169.
- Nelder, A. J. A., & Wedderburn, R. W. M. (1972). Generalized Linear Models. Journal of the Royal Statistical Society, 135(3), 370–384. doi: 10.2307/2344614.
- Ngulube, E., Brink, M., & Chirwa, P. W. (2014). Productivity and cost analysis of semi-mechanised and mechanised systems on the Viphya forest plantations in Malawi. Southern Forests, 76(4), 195–200. doi: 10.2989/20702620.2014.938294.
- Ngulube, E. S. (2012). Prediction of timber harvesting productivity for semi-mechanised systems in Viphya forest plantations, Malawi. MSc. Dissertation, University of Pretoria-South Africa. https://repository.up.ac.za/handle/2263/30939
- Nwokoye, E. S., & Ilechukwu, N. (2018). Theory Of Costs. Principles of Economics 1, August, 144–184. https://www.researchgate.net/publication/326841533
- Obi, O. F., & Visser, R. (2020). Productivity measurement of New Zealand forest harvesting sector using the DEA-Malmquist index. International Journal of Forest Engineering, 31(3), 224–232. doi: 10.1080/14942119.2020.1770566.
- Orlovský, L., Messingerová, V., & Danihelová, Z. (2020). Analysis of the time efficiency of skidding technology based on the skidders. Central European Forestry Journal, 66(3), 177–187. doi: 10.2478/forj-2020-0016.
- Parajuli, M., Hiesl, P., Smidt, M., & Mitchell, D. (2020). Factors influencing productivity and cost in the whole-tree harvesting system. Clemson Cooperative Extension, Land-Grant Press by Clemson Extension, 1079, 1–8.
- Proto, A. R., Macrì, G., Visser, R., Harrill, H., Russo, D., & Zimbalatti, G. (2018). Factors affecting forwarder productivity. European Journal of Forest Research, 137(2), 143–151. doi: 10.1007/s10342-017-1088-6.
- Ravindra, K., Rattan, P., Mor, S., & Aggarwal, A. N. (2019). Generalized additive models: Building evidence of air pollution, climate change and human health. Environment International, 132(March), 104987. doi: 10.1016/j.envint.2019.104987.
- Sabo, A., & Poršinsky, T. (2005). Skidding of fir roundwood by Timberjack 240C from selective forests of Gorski Kotar. Croatian Journal of Forest Engineering, 26(1), 13–27.
- Sessions, J. (2007). Forest Road Operations in the tropics (H. D. Dr. Dieter Czeschlik (ed.)). Springer-Verlag is a part of Springer Science + Business Media.
- Shabani, S., Jaafari, A., & Bettinger, P. (2021). Spatial modeling of forest stand susceptibility to logging operations. Environmental Impact Assessment Review, 89(May), 106601. doi: 10.1016/j.eiar.2021.106601.
- Spinelli, R., Eliasson, L., & Han, H. S. (2020). A Critical Review of Comminution Technology and Operational Logistics of Wood Chips. Current Forestry Reports, 6(3), 210–219. doi: 10.1007/s40725-020-00120-9.
- Spinelli, R., Hartsough, B. R., Owende, P. M. O., & Ward, S. M. (2002). Productivity and Cost of Mechanized Whole-Tree Harvesting of Fast-Growing Eucalypt Stands. International Journal of Forest Engineering, 13(2), 49–60. doi: 10.1080/14942119.2002.10702462.
- Spinelli, R., Visser, R., & Han, H. S. (2019). A decade of forest engineering: Achievements and future directions. Forests, 10(9), 1–12. doi: 10.3390/f10090724.
- Šporčić, M., Landekić, M., Šušnjar, M., Pandur, Z., Bačić, M., & Mijoč, D. (2023). Deliberations of Forestry Workers on Current Challenges and Future Perspectives on Their Profession—A Case Study from Bosnia and Herzegovina. Forests, 14(4), 18. doi: 10.3390/f14040817.
- Stoilov, S., Angelov, G., Aladzhov, S., & Nichev, P. (2021). Productivity models and costs of combined skidder - Harvester in coniferous forests. Forestry Ideas, 27(1), 169–181.
- Varch, T., Erber, G., Spinelli, R., Magagnotti, N., & Stampfer, K. (2021). Productivity, fuel consumption and cost in whole tree cable yarding: conventional diesel carriage versus electrical energy-recuperating carriage. International Journal of Forest Engineering, 32(S1), 20–30. doi: 10.1080/14942119.2020.1848178.
- Visser, R., & Stampfer, K. (2003). Tree-length system evaluation of second thinning in a loblolly pine plantation. Southern Journal of Applied Forestry, 27(2), 77–82. doi: 10.1093/sjaf/27.2.77.
- Vitorelo, B. (2011). Cost and Productivity of Two Mechanical Fire Hazard Reduction methods: mastication and thinning. Faculty of Humboldt State University.
- Wang, J., LeDoux, C. B., & Li, Y. (2005). Simulating Cut-to-Length Harvesting Operations in Appalachian Hardwoods. International Journal of Forest Engineering, 16(2), 11–27. doi: 10.1080/14942119.2005.10702510.
- Wang, J., Long, C., McNeel, J., & Baumgras, J. (2004). Productivity and cost of manual felling and cable skidding in central Appalachian hardwood forests. Forest Products Journal, 54(12), 45–51.
- Yap, B. W., & Sim, C. H. (2011). Journal of Statistical Computation and Comparisons of various types of normality tests. Journal of Statistical Computation and Simulation, 81(12), 37–41. doi: 10.1080/00949655.2010.52016.
- Zhang, X., Wang, J., Vance, J., Wang, Y., Wu, J., & Hartley, D. (2020). Data Analytics for Enhancement of Forest and Biomass Supply Chain Management. In Forest Engineering. Springer.