ABSTRACT
Background
Special populations like people living with HIV/AIDS (PLWHA) and people with opioid use disorder (OUD) smoke tobacco cigarettes at rates three to four times greater than the general population. Patients with tobacco use disorder exhibit attentional bias (AB) for cigarette cues. Eye tracking can quantify this bias by measuring fixation time (FT) on cigarette and matched neutral cues, to calculate an AB score. Although previous studies have measured this bias in people who smoke without any other comorbid conditions, no study, to our knowledge, has measured or compared this bias in special populations.
Methods
We performed exploratory analyses on eye tracking data collected in two separate randomized clinical trials (RCTs) (NCT05049460, NCT05295953). We compared FT and cigarette-cue AB score (measured by subtracting FT on neutral cues from FT on cigarette cues) between PLWHA and people with OUD who smoke, using a visual probe task and Tobii Pro Fusion eye tracker. We used two cigarette cue types, one encompassing people smoking cigarettes and the other consisting of cigarette paraphernalia. We used two cue presentation times, 1000 and 2000 milliseconds (ms).
Results
Cues of people smoking cigarettes elicited greater AB than cues of cigarette paraphernalia across both subject groups when cues were presented for 2000 ms, but not 1000 ms. PLWHA who smoke exhibited greater AB for cues of people smoking cigarettes than cigarette paraphernalia when presented for 2000 ms compared to people with OUD who smoke.
Conclusion
We use cigarette-cue AB to quantify craving and cigarette consumption in two populations smoking at elevated rates. The addition of social cues potentiates cigarette cue AB, based on cue type and stimulus presentation time. Understanding the neurobiology of this relationship can help design novel smoking cessation treatments that target AB and prevent relapse in these populations with suboptimal response to smoking cessation treatments.
Trial registration
Clinical trials that provided the data for post hoc analyses are NCT05049460 and NCT05295953.
1. Introduction
Smoking is the leading preventable cause of death and disease in the United States (CDC, Citationn.d). Fourteen percent (14%) of adults in the United States smoke (Wang et al., Citation2018). People living with HIV/AIDS (PLWHA) smoke at nearly three to four times the rate of the general population (Lifson & Lando, Citation2012). Smoking in PLWHA is also associated with increased incidence of comorbid substance use disorders, low adherence to antiretroviral therapy (ART) medications and consequently increased mortality and poor quality of life (Pacek et al., Citation2014; Pacek & Crum, Citation2015).
One additional group of individuals with disproportionally high rates of cigarette use are individuals with opioid use disorder (OUD). Approximately 74%–94% of patients with OUD smoke (Guydish et al., Citation2011). The mechanisms for high smoking rates in individuals with OUD are unknown. Two proposed pharmacological mechanisms are cross-tolerance between nicotine and opioids (Kohut, Citation2017; Yoon et al., Citation2015) and nicotine ameliorating pain via alpha-4-beta-2 acetylcholine receptors agonism (α4β2) in individuals with OUD (Yoon et al., Citation2015). In addition, medications used to treat OUD (e.g. methadone and suboxone) are associated with increased smoking rates (Elkader et al., Citation2009; Mutschler et al., Citation2002; Nahvi et al., Citation2006; Richter et al., Citation2007).
Patients with a current or previous history of tobacco use disorder demonstrate an attentional bias (AB) for cigarette cues when exposed to cigarette and neutral cues (Engelmann et al., Citation2012; Lin et al., Citation2020; Masiero et al., Citation2019), whereas those without a history of tobacco use disorder do not. Cigarette cue-AB has also been shown to be associated with increased craving and propensity for relapse in people who smoke cigarettes (Christiansen et al., Citation2015). Eye-tracking measures can be utilized to quantify the differential allocation of attention to salient cues (e.g. cigarettes) as compared to neutral cues (Berridge, Citation2007; Field & Cox, Citation2008). Eye-tracking measures such as the visual probe task utilize fixation time (FT), measured in milliseconds towards salient cues and neutral cues as an index of AB (Christiansen et al., Citation2015; Marks et al., Citation2015). Cigarette-cue AB have been shown to be consistent over longitudinal periods of time when measured in subjects with substance use disorders (Marks et al., Citation2015). Plausible modulators of cigarette-cue AB include abstinence, the presence of withdrawal, craving for the substance of choice, sensory modality, duration of salient cue presentation, and emotional valence of cues (Field & Cox, Citation2008; Jasinska et al., Citation2014).
Previous work quantified cigarette-cue AB via eye-tracking measures with cue presentation times ranging from 200 to 2000 ms (Bradley et al., Citation2003, Citation2008; Creswell & Skrzynski, Citation2021; Marks et al., Citation2016; Mogg et al., Citation2003; Waters et al., Citation2003). All these studies compared people with and without tobacco use disorder. No previous studies have examined cigarette-cue AB in populations with higher rates of smoking such as PLWHA or people with OUD. In addition, previous studies have not compared how cigarette-cue AB differs based on cue type (people smoking cigarettes [social] versus cigarette paraphernalia [non-social]) but have suggested it as a potential area to explore (Jasinska et al., Citation2014).
The present study sought to compare in a post-hoc manner, differences in cigarette-cue AB between PLWHA who smoke tobacco cigarettes and people with OUD who smoke tobacco cigarettes. Our aims with these analyses were two-fold: (1) To examine the effect of cue types and cue presentation times on cigarette-cue AB and (2) Compare cigarette-cue AB between two populations with significantly elevated smoking rates to delineate the neurobiological bases. We used two cue types- people smoking cigarette cues and cigarette paraphernalia in separate trials. For each cue type, we used cue presentation times of 1000 milliseconds (ms) and 2000 ms. Previous studies demonstrated greater cigarette-cue AB at longer cue durations (Bradley et al., Citation2003, Citation2008).
The primary hypothesis of the present study was cigarette-cue AB towards both cue types would be significantly greater with presentation times of 2000 ms compared to 1000 ms. Given higher substance use comorbidity and disease burden in PLWHA who smoke, we also hypothesized PLWHA who smoke would show greater cigarette-cue AB than people with OUD who smoke (Pacek et al., Citation2014). Given no previous studies on cigarette-cue AB in these populations and lack of research on neurobiology underlying the elevated smoking rates, we intend these analyses to be proof of concept in utilizing cigarette-cue AB to quantify cigarette craving and consumption in such special populations with elevated smoking rates.
2. Methods
2.1. Participants
In an exploratory post-hoc manner, we analyzed cigarette-cue AB data (encompassing FT and AB scores) collected from participants in two randomized clinical trials (RCTs). These participants were recruited in two RCTs comparing effects of active versus sham transcranial magnetic stimulation (TMS) on cigarette-cue attentional bias (NCT05049460, NCT05295953). Although the RCTs differed in number of TMS sessions (one session in NCT05295953 and four sessions in NCT05049460) cigarette-cue AB data was acquired in the same manner in participants in both RCTs. The RCTs examined how TMS modulated cigarette-cue AB, and so we analyzed only baseline cigarette-cue AB (acquired before TMS) for the purpose of this manuscript.
We compared cigarette-cue AB between ten PLWHA who smoke tobacco cigarettes (1 female, 9 male) and ten participants with OUD who smoke tobacco cigarettes (6 females, 4 males). Both sets of participants came from two clinics affiliated with the University of Kentucky Medical Center.
2.2. Procedures
The study protocols that recruited participants were approved by Medical Institutional Review Board (IRB) at University of Kentucky and were registered on clinical trials.gov (NCT05049460, NCT05295953). Prior to study day, all participants completed a phone screen to determine eligibility. Once deemed eligible for the study, participants were instructed to abstain from smoking cigarettes, or using substances (such as alcohol, cocaine, methamphetamine, opioids [except those that are prescribed], and psychedelics) for two hours prior to start of experimental session.
On study day, all participants completed an IRB approved informed consent. Demographic information, medical history, and current and past use of tobacco were then assessed. Smoking abstinence was corroborated with carbon monoxide (CO) measurement using a Smokerlyzer Breath CO Monitor (Bedfont Scientific Ltd., Rochester, England). Breath alcohol level (BAL) was also recorded using a Breathalyzer (Alco-Sensor FST, St. Louis, MO, U.S.A.). Participants were required to have a CO level less than 10 ppm and a BAL of 0.000% to proceed with study procedures. Participants completed Fagerstrom Test for Nicotine Dependence (FTND) and four sets of cigarette-cue AB trials. Two sets of cigarette-cue AB trials measured cigarette-cue AB for cues encompassing people smoking cigarette versus neutral cues and two sets of cigarette-cue AB trials measured cigarette-cue AB for cigarette paraphernalia cues versus neutral cues.
2.3. Visual probe task
Attentional Bias was measured using the visual probe task (Marks et al., Citation2014). Fixation data were collected using Tobii Pro Fusion 120 Hz eye tracker (Tobii Technology, Sweden). For each AB trial, images of a cigarette (C) and a matched neutral (N) cue were presented on a laptop screen, 3 cm apart. Upon offset of the image pairs, a visual probe (X) appeared on either the left side or right side of the screen, in the same location as one of the previously presented images. Firstly, twenty trials showed images of cues encompassing people smoking cigarettes and matched neutral cues (C–N) for 1000 ms. An additional twenty trials showed the same image pairs for 2000ms. Secondly, twenty trials showed images of cues encompassing cigarette paraphernalia and matched neutral cues (C–N) for 1000 ms. An additional twenty trials showed the same image pairs for 2000 ms.
For each set of twenty cigarette-cue AB trials, twenty filler trials consisting of twenty pairs of additional neutral cue images (N-N) were intermixed to generate a total of 40 trials. Filler trials were also presented for 1000 or 2000 ms, depending on presentation times of C–N cue pairs. Intervals between C–N and N–N cue pairs were 1000 or 2000 ms, contingent on cue presentation time. Please see Supplementary material figure 1 for a visual of cue presentation.
Cigarette-cue images were taken from a validated database (Smoking Cue Database [SmoCuDa]) (Manoliu et al., Citation2021). The database divided 250 image cues related to smoking, into eighteen categories based on similarities in cue description. Each cue image in SmoCuDa was rated by 40 participants who smoked, on ratings of ‘urge to smoke’, ‘valence’ and ‘arousal’ between 1 and 100. For cues of people smoking cigarette cues, we chose the first twenty relevant images in the database, ranked by urge to smoke from highest to lowest. Cues were counterbalanced for sex, ethnicity, and affective content. For cues encompassing cigarette paraphernalia, we chose the first twenty relevant images ranked by urge to smoke from highest to lowest.
2.4. Data analytic strategy.
A trial level cigarette-cue AB score was calculated for every trial by FT on cigarette cue minus FT on neutral cue (Carlson & Fang, Citation2020; Yang et al., Citation2022). We calculated averages of trial level FT for cigarette cues, trial level FT for neutral cues, and trial level AB score for cigarette cues for each set of cues separately for 1000 ms and 2000 ms of cue presentation times in both participant groups. Any trial wherein the FT on both cigarette and neutral cue were both null was deemed an outlier and removed. As part of cleaning the data, we removed 101 outlier observations which had null values for FT on cigarette and neutral cues. This reduced total number of total trials from 1600 to 1459.
We analyzed the data in two ways: (1) Utilizing trial level FT and cigarette-cue AB scores and (2) Average FT and cigarette-cue AB scores. We did this given previously established advantages of trial level scores over average scores including but not limited to capturing temporal fluctuations and variability of attentional allocation trial by trial in addition to being less susceptible to data cleaning procedures (Carlson & Fang, Citation2020; Yang et al., Citation2022).
In the first analysis, all trial level FT for cigarette cues and respective AB scores were entered into separate linear mixed effects regression models (Judd et al., Citation2017). Outcome variables in one model was FT on cigarette cues and cigarette-cue AB score in the other model. Predictor variables in both models included age, gender, subject group (PLWHA who smoke versus OUD who smoke), cigarette cue type (people smoking cigarette cues and neutral cues versus cigarette paraphernalia cues and neutral cues), cue presentation time (1000 ms versus 2000 ms of presentation time for cue pairs) and FTND scores. All predictor variables except subject ID were used as fixed effects and subject ID was a random effect (Judd et al., Citation2017).
In the second analysis, we calculated average values for trial level FT and cigarette-cue AB scores for each participant. We entered these scores into separate linear mixed effects regression models. Outcome variables in one model was FT on cigarette cues and AB score in the other model. These results are listed in the supplementary material. Predictor variables in both models included age, gender, subject group (PLWHA who smoke versus OUD who smoke), cigarette cue type (people smoking cigarette cues and neutral cues versus cigarette paraphernalia cues and neutral cues), cue presentation time (1000 ms versus 2000 ms of presentation time for cue pairs) and FTND scores. All predictor variables except subject ID were used as fixed effects and subject ID was a random effect.
We performed all analyses using Matlab version R2022a (Natick, Massachussetts: The Mathworks Inc.). The MATLAB code used for analyses is listed in Appendix 1 (in Supplementary Material).
3. Results
An independent-samples t-test revealed statistically significant difference in age between the two groups. (t(18) = 3.39, p = 0.003). The mean age in the PLWHA who smoke group was 44.6 years (SEM = ±3.14) and 33 years (SEM = ±1.76) for people with OUD who smoke. A separate independent samples t-test revealed no statistically significant difference in FTND scores (t(18) = 0.91, p = 0.37). The respective mean FTND scores for the PLWHA and OUD groups were 6.10 and 7 (SEMs = ± 0.87 and 0.56, respectively). A chi-square test revealed that a significant difference in the sex distribution (χ2(1) = 17.35, p = 0.02). Given our limited sample, we did not include age or gender as predictor variables in our regression models due to the fact that the majority of PLWAH are men who have sex with men (MSM) and are older (HIV.gov, Citationn.d). FT was highest in the PLWHA group presented with cues of people smoking cigarettes and neutral cues, with stimulus presentation time of 2000 ms ( and ).
Figure 1. Showcases bars encapsulating average fixation time (FT) in milliseconds for cigarette cues and neutral cues. The X-axis lists cue presentation times (1000 ms versus 2000 ms), cue type (people smoking cigarettes with matched neutral cues versus cigarette paraphernalia with matched neutral cues) and subject group (PLWHA who smoke versus patients with OUD who smoke). The Y-axis shows average fixation time in milliseconds. Bars in blue represent FT on cigarette cues and bars in orange represent FT on neutral cues. PLWHA who smoke had higher FT on both cigarette cue types when presented for 2000 ms, compared to people with OUD who smoke. Across both subject groups, cues of people smoking cigarettes elicited significantly higher FT than cues of cigarette paraphernalia when presented for 2000 ms.
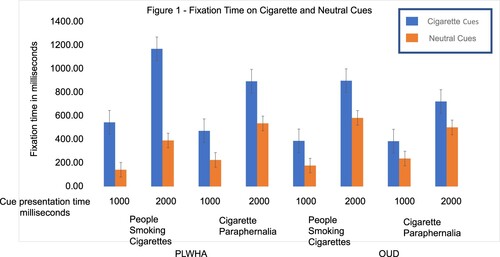
Table 1. Fixation Time (FT) and Cigarette-cue AB score Mean values in cells and standard error of mean in parentheses.
shows a linear mixed effects regression model with trial level FT on cigarette cues as outcome. Cues of people smoking cigarettes elicited greater FTs than cues of cigarette paraphernalia across both subject groups (). This is corroborated by significant association between FT and cue type (t(1446) = 1.96, p = 0.05) and no interaction between subject group and cue type (t(1446) = −0.09, p = 0.93) (). Across both subject groups, cues of people smoking cigarettes showed significantly higher FT than cigarette paraphernalia cues with 2000ms but not with 1000 ms, as reflected by the significant association between cue presentation time and FT (t(1446) = 11.43, p < 0.00001) and significant interaction between cue type and cue presentation time (t(1446) = −3.28, p = 0.001) (). Although PLWHA showed greater FT for cigarette cues than patients with OUD () this was not significant when controlling for other predictors (). In a linear mixed effects regression models with average FT as the outcome measure, only cue presentation time showed a significant association with FT (t(70) = 722.92, p = 0.003) (Supplementary material table 1).
Table 2. Linear mixed effects model trial level fixation time (FT) on cigarette cues as outcome measure trial level FT on cigarette cues (milliseconds) ∼ 1 + subject group (SG)(PLWHA who smoke versus OUD who smoke) + cue presentation time (CPT) (1000 milliseconds versus 2000 milliseconds of cue presentation) + Fagerstrom test for nicotine dependence (FTND) scores + cue type (cues of people smoking cigarette versus cigarette paraphernalia cues) + SG * cue type + SG * CPT + cue type * CPT + SG * CPT * Cue type + 1|Subject ID. (R2 = 0.44).
shows a linear mixed effects regression models with trial level cigarette-cue AB score as the outcome measure. Neither cue type nor cue presentation time showed significant associations with cigarette-cue AB score (). PLWHA who smoke exhibited significantly greater cigarette-cue AB score for people smoking cigarette cues when presented for 2000 ms instead of 1000 ms compared to people with OUD who smoke, as shown by a significant three-way interaction between diagnostic group, cue presentation time and cue type (t(1446) = −1.98, p = 0.05) (). In a linear mixed effects regression model with average cigarette-cue AB score as outcome measure, these associations were not significant (Supplementary material table 2).
Table 3. Linear mixed effects model trial level cigarette-cue AB score as outcome measure trial level cigarette-cue AB score ∼ 1 + subject group (SG)(PLWHA who smoke versus OUD who smoke) + cue presentation time (CPT) (1000 milliseconds versus 2000 milliseconds of cue presentation) + Fagerstrom test for nicotine dependence (FTND) scores + cue type (cues of people smoking cigarette versus cigarette paraphernalia cues) + SG * cue type + SG * CPT + cue type * CPT + SG * CPT * Cue type + 1|Subject ID. (R2 = 0.18).
4. Discussion
To the best of our knowledge, this is the first study to compare cigarette-cue AB between populations who smoke at elevated rates due to their comorbid illnesses (HIV/AIDS and OUD). PLWHA who smoke showed greater cigarette-cue AB than people with OUD who smoke. In addition, presenting cues for 2000 ms elicited greater cigarette-cue AB than 1000 ms. Cues of people smoking cigarettes elicited greater FT than cigarette paraphernalia in both groups.
Ascertaining why both these populations smoke at elevated rates is difficult given lack of research on underlying neurobiology. Some preliminary directions suggest increased central nervous system oxidative stress and inflammation in people with HIV who smoke (Chang et al., Citation2020); and shared downstream effects between mu opioid receptors (MOR) and alpha-4-beta-2 (α4β2) subtype of acetylcholine receptors in decreasing dopaminergic transmission in people with OUD who smoke (Kuwabara et al., Citation2014; McGehee, Citation2006). It is plausible that differences in smoking rates between these populations, compared to those without these comorbidities are driven by neurobiological differences. Although both populations have premature mortality attributable to smoking (Bandiera et al., Citation2015; Hser et al., Citation1994; Pacek & Crum, Citation2015), there is some data to suggest that PLWHA have more symptom burden and earlier mortality from smoking compared to people with OUD who smoke (Helleberg et al., Citation2015; Reddy et al., Citation2017). Our results corroborate this.
Although these analyses were performed in an exploratory post-hoc manner, our manuscript is an effort to spearhead three goals: (1) A proof of concept to quantify the neurobiology of increased cigarette craving and cigarette consumption in two special populations who smoke at elevated rates using cigarette-cue AB; (2) Optimizing cue types and cue presentation times to measure cigarette-cue AB; and (3) Extending the results from these analyses to advocate for interventions individualized towards these populations. Both populations included in this manuscript have poor efficacy and effectiveness with current smoking cessation interventions (Nahvi et al., Citation2014; Pacek & Crum, Citation2015; Stein et al., Citation2013). In the absence of any previous studies comparing physiological parameters of smoking severity between these special populations with elevated smoking rates, our results showing greater cigarette-cue AB in PLWHA who smoke compared to people with OUD who smoke makes our manuscript a unique contribution to the literature.
In alignment with our results, the SmoCuDa database showed greater urge to smoke and valence for cues of people smoking cigarettes than cigarette paraphernalia (Manoliu et al., Citation2021). Two other studies did not show any association between affective nature of cues and FT for cigarette cues in people who smoke (Bradley et al., Citation2008; Mogg et al., Citation2003).
Comparing patients with tobacco use disorder to controls, four notable studies showed an AB towards cigarette cues using response times and three studies showed an AB towards cigarette cues using FT via eye tracking (Bradley et al., Citation2003, Citation2008; Creswell & Skrzynski, Citation2021; Ehrman et al., Citation2002; Marks et al., Citation2016; Mogg et al., Citation2003; Waters et al., Citation2003). These seven studies used variable cue presentation times: 500 ms (Ehrman et al., Citation2002; Waters et al., Citation2003), 1000 ms (Marks et al., Citation2016), only 2000 ms (Creswell & Skrzynski, Citation2021), 200 and 2000 ms (Bradley et al., Citation2008), 500 ms and 2000 ms (Bradley et al., Citation2003). Except for one study which showed an AB when cues were presented for 500 ms (Ehrman et al., Citation2002), all other studies showed an AB towards cigarette cues were presented for 1000 and 2000ms, but not for 200 or 500 ms (Bradley et al., Citation2003, Citation2008; Creswell & Skrzynski, Citation2021; Marks et al., Citation2016; Mogg et al., Citation2003; Waters et al., Citation2003). Although previous studies have compared cue presentation times of 500 ms and 2000 ms, ours is the first to compare 1000 and 2000 ms.
Both groups differed in age and gender distributions. PLWHA who smoke comprised mostly males (n = 9) and people with OUD who smoke comprised mostly females (n = 6). In addition, PLWHA who smoke were older than people with OUD who smoke. This is consistent with previously established demographics of PLWHA. Majority of PLWHA are MSM (HIV.gov, Citationn.d). In 2020, MSM accounted for 71% of new HIV diagnoses in the United States (HIV.gov, Citationn.d). With opioid use disorder, females have been found to have greater prescription opioid use, and psychiatric comorbidity with opioid use disorder compared to males (McHugh, Citation2020). However, given the demographics of HIV and OUD, our small sample size and pilot nature of analyses, it would not be meaningful to factor age or gender as predictor variables in our analyses.
Although FT on cigarette cues could have some conceptual overlap with cue reactivity, the goal with FT measurement is not to elicit craving in response to cue presentation (Berridge, Citation2007; Field & Cox, Citation2008). The neurobiological basis of cigarette-cue reactivity from studies done in populations who smoke and do not have any comorbid illness suggests dopaminergic sensitization in the mesolimbic pathway, decrease in central executive network (CEN) functional connectivity, and increase in salience network functional connectivity (Engelmann et al., Citation2012; Jasinska et al., Citation2014). The neurobiological mechanism for how cue type or cue presentation time affects cigarette-cue AB is unknown (Zilverstand et al., Citation2018). Future studies examining the neural correlates of cigarette-cue AB in people who smoke with and without comorbid illness are warranted. Given suboptimal quit rates with current smoking cessation interventions in PLWHA and people with OUD who smoke, delineating the neurobiology of cigarette-cue AB will be instrumental in spearheading novel smoking cessation interventions in these populations.
5. Conclusions and limitations
The sample size was small limiting generalizability. We found greater cigarette-cue AB score when cues were presented for 2000 ms compared to 1000 ms for both special populations who smoke at elevated rates. Cues comparing people smoking cigarettes and equivalent neutral cues elicited greater FT in both populations than cues of cigarette paraphernalia with corresponding neutral cues. PLWHA who smoke showed greater cigarette-cue AB score than people with OUD who smoke for cues of people smoking cigarettes when presented for 2000 ms. These preliminary results do support exploring neural correlates of these cue presentations, to identify a valid behavioral marker of cigarette use and relapse.
Supplemental Material
Download MS Word (566.6 KB)Acknowledgements
We thank Madona Elias, MSW who assisted with data collection and Christopher McLouth, PhD who reviewed the statistical analyses in the manuscript.
Disclosure statement
No potential conflict of interest was reported by the author(s).
Data availability statement
Our study data is available on Mendeley Data. The DOI is http://dx.doi.org/10.17632/g6yzzr34sx.1
Additional information
Funding
References
- Bandiera, F. C., Anteneh, B., Le, T., Delucchi, K., & Guydish, J. (2015). Tobacco-related mortality among persons with mental health and substance abuse problems. PLoS One, 10(3), e0120581. https://doi.org/10.1371/journal.pone.0120581
- Berridge, K. C. (2007). The debate over dopamine's role in reward: The case for incentive salience. Psychopharmacology, 191(3), 391–431. https://doi.org/10.1007/s00213-006-0578-x
- Bradley, B. P., Field, M., Healy, H., & Mogg, K. (2008). Do the affective properties of smoking-related cues influence attentional and approach biases in cigarette smokers? Journal of Psychopharmacology, 22(7), 737–745. https://doi.org/10.1177/0269881107083844
- Bradley, B. P., Mogg, K., Wright, T., & Field, M. (2003). Attentional bias in drug dependence: Vigilance for cigarette-related cues in smokers. Psychology of Addictive Behaviors, 17(1), 66–72. https://doi.org/10.1037/0893-164X.17.1.66
- Carlson, J. M., & Fang, L. (2020). The stability and reliability of attentional bias measures in the dot-probe task: Evidence from both traditional mean bias scores and trial-level bias scores. Motivation and Emotion, 44(5), 657–669. https://doi.org/10.1007/s11031-020-09834-6
- CDC. (n.d). Current cigarette smoking among adults in the United States. Retrieved June 23, 2021, from https://www.cdc.gov/tobacco/data_statistics/fact_sheets/adult_data/cig_smoking/index.htm#references
- Chang, L., Liang, H., Kandel, S. R., & He, J. J. (2020). Independent and combined effects of nicotine or chronic tobacco smoking and HIV on the brain: A review of preclinical and clinical studies. Journal of Neuroimmune Pharmacology, 15(4), 658–693. https://doi.org/10.1007/s11481-020-09963-2
- Christiansen, P., Mansfield, R., Duckworth, J., Field, M., & Jones, A. (2015). Internal reliability of the alcohol-related visual probe task is increased by utilising personalised stimuli and eye-tracking. Drug and Alcohol Dependence, 155, 170–174. https://doi.org/10.1016/j.drugalcdep.2015.07.672
- Christiansen, P., Schoenmakers, T. M., & Field, M. (2015). Less than meets the eye: Reappraising the clinical relevance of attentional bias in addiction. Addictive Behaviors, 44, 43–50. https://doi.org/10.1016/j.addbeh.2014.10.005
- Creswell, K. G., & Skrzynski, C. J. (2021). The influence of smoking motivation on the associations among cigarette craving, attentional bias to smoking cues, and smoking behavior. Nicotine & Tobacco Research, 23(10), 1727–1734. https://doi.org/10.1093/ntr/ntab028
- Ehrman, R. N., Robbins, S. J., Bromwell, M. A., Lankford, M. E., Monterosso, J. R., & O'Brien, C. P. (2002). Comparing attentional bias to smoking cues in current smokers, former smokers, and non-smokers using a dot-probe task. Drug and Alcohol Dependence, 67(2), 185–191. https://doi.org/10.1016/S0376-8716(02)00065-0
- Elkader, A. K., Brands, B., Selby, P., & Sproule, B. A. (2009). Methadone-nicotine interactions in methadone maintenance treatment patients. Journal of Clinical Psychopharmacology, 29(3), 231–238. https://doi.org/10.1097/JCP.0b013e3181a39113
- Engelmann, J. M., Versace, F., Robinson, J. D., Minnix, J. A., Lam, C. Y., Cui, Y., Brown, V. L., Cinciripini, P. M., (2012). Neural substrates of smoking cue reactivity: A meta-analysis of fMRI studies. Neuroimage, 60(1), 252–262. https://doi.org/10.1016/j.neuroimage.2011.12.024
- Field, M., & Cox, W. M. (2008). Attentional bias in addictive behaviors: A review of its development, causes, and consequences. Drug and Alcohol Dependence 97(1–2), 1–20. https://doi.org/10.1016/j.drugalcdep.2008.03.030
- Guydish, J., Passalacqua, E., Tajima, B., Chan, M., Chun, J., & Bostrom, A. (2011). Smoking prevalence in addiction treatment: A review. Nicotine & Tobacco Research, 13(6), 401–411. https://doi.org/10.1093/ntr/ntr048
- Helleberg, M., May, M. T., Ingle, S. M., Dabis, F., Reiss, P., Fätkenheuer, G., Costagliola, D., d’Arminio, A., Cavassini, M., Smith, C., Justice, A. C., Gill, J., Sterne, J. A. C., Obel, N. (2015). Smoking and life expectancy among HIV-infected individuals on antiretroviral therapy in Europe and North America. Aids (London, England), 29(2), 221–229. https://doi.org/10.1097/QAD.0000000000000540
- HIV.gov. (n.d). U.S. statistics. https://www.hiv.gov/hiv-basics/overview/data-and-trends/statistics
- Hser, Y. I., McCarthy, W. J., & Anglin, M. D. (1994). Tobacco use as a distal predictor of mortality among long-term narcotics addicts. Preventive Medicine, 23(1), 61–69. https://doi.org/10.1006/pmed.1994.1009
- Jasinska, A. J., Stein, E. A., Kaiser, J., Naumer, M. J., & Yalachkov, Y. (2014). Factors modulating neural reactivity to drug cues in addiction: A survey of human neuroimaging studies. Neuroscience & Biobehavioral Reviews, 38, 1–16. https://doi.org/10.1016/j.neubiorev.2013.10.013
- Judd, C. M., Westfall, J., & Kenny, D. A. (2017). Experiments with more than one random factor: Designs, analytic models, and statistical power. Annual Review of Psychology, 68(1), 601–625. https://doi.org/10.1146/annurev-psych-122414-033702
- Kohut, S. J. (2017). Interactions between nicotine and drugs of abuse: A review of preclinical findings. The American Journal of Drug and Alcohol Abuse, 43(2), 155–170. https://doi.org/10.1080/00952990.2016.1209513
- Kuwabara, H., Heishman, S. J., Brasic, J. R., Contoreggi, C., Cascella, N., Mackowick, K. M., Taylor, R., Rousset, O., Willis, W., Huestis, M. A., Concheiro, M., Wand, G., Wong, D. F., Volkow, N. D. (2014). Mu opioid receptor binding correlates with nicotine dependence and reward in smokers. PLoS One, 9(12), e113694. https://doi.org/10.1371/journal.pone.0113694
- Lifson, A. R., & Lando, H. A. (2012). Smoking and HIV: Prevalence, health risks, and cessation strategies. Current HIV/AIDS Reports, 9(3), 223–230. https://doi.org/10.1007/s11904-012-0121-0
- Lin, X., Deng, J., Shi, L., Wang, Q., Li, P., Li H., Liu, J., Que, J., Chang, S., Bao, Y., Shi, J., Weinberger, D. R., Wu, P., Lu, L. (2020). Neural substrates of smoking and reward cue reactivity in smokers: A meta-analysis of fMRI studies. Translational Psychiatry 10(1), 97. https://doi.org/10.1038/s41398-020-0775-0
- Manoliu, A., Haugg, A., Sladky, R., Hulka, L., Kirschner, M., Brühl, A. B., Seifritz, E., Quednow, B., Herdener, M., Scharnowski, F. (2021). Smocuda: A validated smoking cue database to reliably induce craving in tobacco use disorder. European Addiction Research, 27(2), 107–114. https://doi.org/10.1159/000509758
- Marks, K. R., Alcorn, J., III, Stoops, W. W., & Rush, C. R. (2016). Cigarette Cue attentional bias in cocaine-smoking and non-cocaine-using cigarette smokers. Nicotine & Tobacco Research, 18(9), 1915–1919. https://doi.org/10.1093/ntr/ntw026
- Marks, K. R., Pike, E., Stoops, W. W., & Rush, C. R. (2015). The magnitude of drug attentional bias is specific to substance use disorder. Psychology of Addictive Behaviors, 29(3), 690–695. https://doi.org/10.1037/adb0000084
- Marks, K. R., Roberts, W., Stoops, W. W., Pike, E., Fillmore, M. T., & Rush, C. R. (2014). Fixation time is a sensitive measure of cocaine cue attentional bias. Addiction, 109(9), 1501–1508. https://doi.org/10.1111/add.12635
- Masiero, M., Lucchiari, C., Maisonneuve, P., Pravettoni, G., Veronesi, G., & Mazzocco, K. (2019). The attentional bias in current and former smokers. Frontiers in Behavioral Neuroscience, 13(154), https://doi.org/10.3389/fnbeh.2019.00154
- McGehee, D. S. (2006). Nicotinic and opioid receptor interactions in nicotine addiction. Molecular Interventions, 6(6), 311–314. https://doi.org/10.1124/mi.6.6.4
- McHugh, R. K. (2020). The importance of studying Sex and gender differences in opioid misuse. JAMA Network Open, 3(12), e2030676. https://doi.org/10.1001/jamanetworkopen.2020.30676
- Mogg, K., Bradley, B. P., Field, M., & De Houwer, J. (2003). Eye movements to smoking-related pictures in smokers: Relationship between attentional biases and implicit and explicit measures of stimulus valence. Addiction, 98(6), 825–836. https://doi.org/10.1046/j.1360-0443.2003.00392.x
- Mutschler, N. H., Stephen, B. J., Teoh, S. K., Mendelson, J. H., & Mello, N. K. (2002). An inpatient study of the effects of buprenorphine on cigarette smoking in men concurrently dependent on cocaine and opioids. Nicotine & Tobacco Research, 4(2), 223–228. https://doi.org/10.1080/14622200210124012
- Nahvi, S., Ning, Y., Segal, K. S., Richter, K. P., & Arnsten, J. H. (2014). Varenicline efficacy and safety among methadone maintained smokers: A randomized placebo-controlled trial. Addiction, 109(9), 1554–1563. https://doi.org/10.1111/add.12631
- Nahvi, S., Richter, K., Li, X., Modali, L., & Arnsten, J. (2006). Cigarette smoking and interest in quitting in methadone maintenance patients. Addictive Behaviors, 31(11), 2127–2134. https://doi.org/10.1016/j.addbeh.2006.01.006
- Pacek, L. R., & Crum, R. M. (2015). A review of the literature concerning HIV and cigarette smoking: Morbidity and mortality, associations with individual- and social-level characteristics, and smoking cessation efforts. Addiction Research & Theory, 23(1), 10–23. https://doi.org/10.3109/16066359.2014.920013
- Pacek, L. R., Latkin, C., Crum, R. M., Stuart, E. A., & Knowlton, A. R. (2014). Current cigarette smoking among HIV-positive current and former drug users: Associations with individual and social characteristics. AIDS and Behavior, 18(7), 1368–1377. https://doi.org/10.1007/s10461-013-0663-1
- Reddy, K. P., Kong, C. Y., Hyle, E. P., Baggett, T. P., Huang, M., Parker, R. A., Paltiel, A. D., Losina, E., Weinstein, M. C., Freedberg, K. A., Walensky, R. P. (2017). Lung cancer mortality associated with smoking and smoking cessation among people living with HIV in the United States. JAMA Internal Medicine, 177(11), 1613–1621. https://doi.org/10.1001/jamainternmed.2017.4349
- Richter, K. P., Hamilton, A. K., Hall, S., Catley, D., Cox, L. S., & Grobe, J. (2007). Patterns of smoking and methadone dose in drug treatment patients. Experimental and Clinical Psychopharmacology, 15(2), 144–153. https://doi.org/10.1037/1064-1297.15.2.144
- Stein, M. D., Caviness, C. M., Kurth, M. E., Audet, D., Olson, J., & Anderson, B. J. (2013). Varenicline for smoking cessation among methadone-maintained smokers: A randomized clinical trial. Drug and Alcohol Dependence, 133(2), 486–493. https://doi.org/10.1016/j.drugalcdep.2013.07.005
- Wang, T. W., Asman, K., Gentzke, A. S., Cullen, K. A., Holder-Hayes, E., Reyes-Guzman, C., Jamal, A., Neff, L., & King, B. A. (2018). Tobacco product use among adults – United States, 2017. MMWR. Morbidity and Mortality Weekly Report, 67(44), 1225–1232. https://doi.org/10.15585/mmwr.mm6744a2
- Waters, A. J., Shiffman, S., Bradley, B. P., & Mogg, K. (2003). Attentional shifts to smoking cues in smokers. Addiction, 98(10), 1409–1417. https://doi.org/10.1046/j.1360-0443.2003.00465.x
- Yang, M. J., Borges, A. M., Emery, N. N., & Leyro, T. M. (2022). Trial-level bias score versus mean bias score: Comparison of the reliability and external validity using dot-probe task among daily smokers. Addictive Behaviors, 135, 107456. https://doi.org/10.1016/j.addbeh.2022.107456
- Yoon, J. H., Lane, S. D., & Weaver, M. F. (2015). Opioid analgesics and nicotine: More than blowing smoke. Journal of Pain & Palliative Care Pharmacotherapy, 29(3), 281–289. https://doi.org/10.3109/15360288.2015.1063559
- Zilverstand, A., Huang, A. S., Alia-Klein, N., & Goldstein, R. Z. (2018). Neuroimaging impaired response inhibition and salience attribution in human drug addiction: A systematic review. Neuron, 98(5), 886–903. https://doi.org/10.1016/j.neuron.2018.03.048