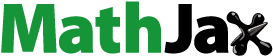
Abstract
We present an age-structured dynamic transmission model for cytomegalovirus (CMV) in the United States, based on natural history and available data, primarily aiming to combine the available qualitative and quantitative knowledge toward more complex modeling frameworks to better reflect the underlying biology and epidemiology of the CMV infection. The model structure explicitly accounts for primary infections, reactivations and re-infections. Duration of infectiousness and likelihood of reactivation were both assumed to be age-dependent, and natural reduction in the re-infection risk following primary infection was included. We used an empirical social contact matrix (POLYMOD-based) as support for CMV transmission between different age groups. The baseline model reproduced well the age-stratified seroprevalence data (National Health and Nutrition Examination Survey III) used for calibration. The model was further used to explore the potential impact of hypothetical vaccination on reducing congenital CMV infection under various vaccine profiles and vaccination scenarios. Our preliminary model-based simulations suggested that while infant vaccination may represent an attractive way to reduce congenital CMV infection over time, adolescent female vaccination with an adequate routine booster platform may, under certain conditions, provide an alternative. However, for such tools to be considered toward actual decision-making, enhanced validations based on additional studies and data would be further necessary. The modeling framework presented in this paper was designed to be sufficiently general and flexible, such that it can allow for further adaptations to reflect new knowledge or data that may become available in the future.
Introduction
Human cytomegalovirus (CMV) is an ubiquitous β-herpes virus that causes mostly mild and asymptomatic infections in immunocompetent individuals, but can cause serious disease in fetuses and immunocompromised individuals (e.g. HIV-infected individuals, organ and stem cell transplant recipients).Citation1 Its natural history is characterized by a primary CMV infection followed by a life-long latent infection that can reactivate periodically resulting in shedding of infectious viral particles.Citation2 Re-infection with a different CMV strain can also occur, with considerable evidence suggesting that immunity resulting from initial infection may provide some level of protection against re-infection.Citation3
CMV is transmitted through contact with CMV-infected body fluids (urine, blood, saliva, genital secretions) of individuals with symptomatic or asymptomatic CMV infection, via close non-sexual contact, sexual contact, breastfeeding, blood transfusion, or organ transplantation.Citation4,5 CMV infection may also be transmitted from mother to fetus, congenital CMV (cCMV) infection being the most common intrauterine infection with severe sequelae, such as deafness, neurological disabilities and visual impairment.Citation6
Congenital CMV infection results from in utero transmission from the mother to her fetus, either because she undergoes a primary CMV infection during pregnancy or because she experiences a recurrent CMV infection, defined as either viral reactivation or re-infection with a different strain of CMV during pregnancy.Citation7 A fetus is at highest risk of contracting CMV infection when the mother has a primary infection during pregnancy; the risk is substantially lower for fetuses with mothers experiencing recurrent infections while pregnant.Citation1 However, a large proportion of congenital CMV infections can be attributed to non-primary infections in mothers.Citation8 In the United States (US), 30% to 50% of women of childbearing age have never been infected with CMV; 1% to 4% of uninfected women experience a primary CMV infection during a pregnancy and in about 33% of these cases the virus is transmitted to the fetus.Citation9
In the US, CMV is the most common viral infection diagnosed at birth: about 1 in 150 newborns has a congenital CMV infection, while 1 in 750 newborns has or develops permanent sequelae due to cCMV infection.Citation9 Hence, every year, of the approximated 28000 children born with congenital CMV infection, more than 5500 suffer permanent disabilities.Citation10 Congenital CMV infection in the US causes more long-term problems and childhood deaths than the Down syndrome, the fetal alcohol syndrome, or neural tube defects.Citation11,12 Overall it has been estimated that 17–20% of the newborns with symptomatic and asymptomatic cCMV infection, at birth, develop permanent sequelae.Citation4 About 13% of newborns diagnosed with cCMV infection are symptomatic, with rates of permanent sequelae ranging from 40% to >80% according to the severity of their disease. Intrauterine growth retardation, prematurity, hepato- and splenomegaly, petechiae, microcephaly, neurological deficits, seizures, or chorioretinitis are few of the sequelae observed in newborns with symptomatic cCMV infections.Citation1,13,14 In case of asymptomatic cCMV infected children, sensorineural hearing loss was observed in 7.4−21% of children 3–4 y old, but late-onset hearing loss after the age of 7 y was also reported.Citation15,16 Therefore, congenital CMV infection and disease are primary targets for prevention, and there is scientific evidence suggesting that viral transmission can be reduced by limiting the risk of infection through hygiene measures or vaccination.Citation17,18 Hence, a better understanding of CMV transmission is important to evaluate potential impact of preventative measures, such as vaccination, at population-level.
In 1999, the Institute of Medicine has included the development of a CMV vaccine among the high priority targets, thus recognizing its potential implications for public health in terms of reducing the economic costs and human morbidity.Citation19 Despite significant progress in virology, immunology, epidemiology, and the availability of several candidate vaccines in early development stages, no product is yet under consideration for licensure.Citation20,21 There is a need for vaccines able to prevent primary infection with CMV and capable of boosting immune responses in already infected individuals.Citation19 Although a CMV vaccine would mainly target adolescents and women of childbearing age, young children could also be targeted since vaccination in this age group may decrease transmission to adults. In addition, the vaccine might be beneficial for patients with AIDS or prospective recipients of transplants.Citation2
Clinical trials of CMV vaccines in women should evaluate protection against cCMV infection, an essential precursor of cCMV disease.Citation19 Additional research should include determination of age-specific incidence and transmission rates, description of the processes involved and relative contributions of maternal reactivation as well as re-infection with cCMV disease, developing assays that can distinguish between reactivation and re-infection.Citation19
Dynamic transmission models are valuable mathematical tools to simulate the transmission and spread of infections in a population and to assess the potential impact of preventative measures.
A dynamic transmission modeling framework enables the integration of multiple natural history infection data with relevant biological/medical quantitative and qualitative information to create a unifying working platform. The resulting validated model can then be employed to project at population level the impact of various interventions, including vaccination, using different working assumptions.
To date, limited work on CMV transmission modeling has been published.Citation22-24 In general, a key rationale for developing mathematical models is to help bridge what is observed with what is not yet known, and to generate and test hypotheses. The work presented here is intended as a step toward more advanced modeling frameworks that could more realistically reflect the biology and epidemiology of CMV infection, based on qualitative knowledge combined with available data. To this end, we developed an epidemiologically realistic, age-structured, dynamic transmission model for CMV in the US, based on natural history and available epidemiological data, and explored a few different hypothetical vaccine profiles and vaccination schemes and their potential impact on reducing congenital CMV infection.
Results
Baseline model
The best-fit model against the age-stratified National Health and Nutrition Examination Survey III (NHANES III) CMV seroprevalence data for the US populationCitation25 is illustrated in . The corresponding model-estimated force of infection (FOI: per susceptible risk of infection per year) as a function of age is shown in . The model succeeded in capturing very well the NHANES III CMV age-specific seroprevalence, with a p-value for model-data of 7*10−7 (correlation coefficient value 0.97).
Figure 1. Illustration of best-fit model-projected age-stratified CMV seroprevalence in the US population vs. the corresponding nationally-representative NHANES III CMV seroprevalence data (A). Model-based force of infection (FOI: per susceptible risk of infection per year) as a function of age corresponding to best model-data fit in (A) (B).
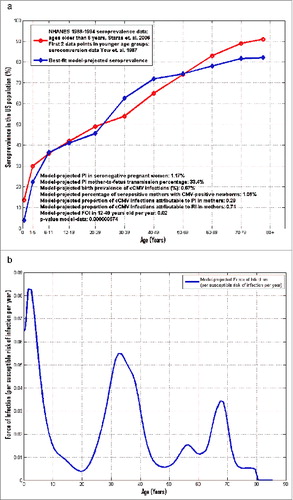
The underlying age-dependent average duration of infectiousness, reactivation rate, and transmission matrix yielded by model calibration under the current model working hypotheses are all fully illustrated in Supplemental File 1. The model-estimated value for the natural reduction k in the risk of an individual to acquire a new infection following primary infection was around 65%. The 0.67% target birth prevalence of congenital CMV infections here was successfully recovered by the model with estimated mother-to-newborn transmission rates of 33.4% and 8.5% following primary versus recurrent maternal infection, respectively. Based on the present model outcomes for the best-fit shown in , an estimated 1.2% of seronegative pregnant women would experience a primary infection, while the corresponding model-projected percentage of seropositive mothers with CMV-positive newborns was 1.1%.
The model-projected percentage of congenital CMV infections attributable to primary infection in mothers was estimated at 29% vs. the percentage of congenital CMV infections attributable to recurrent infection in mothers, estimated at 71% (). This is in-line with the corresponding estimates of 25% vs. 75%, respectively, published by Cannon.Citation11
The model-based force of infection shown in appears highest in very young individuals, with the dominant peak occurring between 0−2 y of age. A secondary peak is visible between 20−40 y of age, with a local maximum around the age of 32 y, likely reflecting the child-bearing years coupled with the associated risks of acquiring infection from young children.Citation26 The model-based force of infection in the pooled 12−49 year-old age group was estimated at 2% per year, in-line with the corresponding estimate from Colugnati et al,Citation2 who estimated an average age of infection at 28.6 y.
Model with vaccination
This modeling framework enables consideration of many different vaccination scenarios. The present work was not intended as exhaustive or final, but as a starting point for illustrative purposes. Thus, for the time being, 8 hypothetical vaccination scenarios we considered a priori of primary interest in the context were included in the analysis on top of the baseline model: vaccination of seronegative or seronegative and seropositive female adolescents with/without booster and with/without catch-up strategies; or vaccination of infants <1 y of age irrespective of their CMV serological status with/without booster and with/without catch-up. Routine vaccination in our model is considered at 6 months and 10 y of age for infants and adolescent females, respectively.
In the present study, similar vaccine coverage and efficacy were considered for routine primary, catch-up and routine booster vaccination. We worked under the plausible assumption that if a platform for boosting later in life is in place, then it would be equally available and offered to both previously vaccinated individuals in whom vaccine protection has waned as well as unvaccinated individuals of the right age. Appropriate set-up of routine booster programs in practice can have an impact by extending protection in those previously vaccinated as well as providing immunity to those previously unvaccinated. Throughout our simulations here, for booster in seronegative women, if the mean duration of vaccine protection was assumed to be 10 y, then routine booster administration applied to seronegative females at 20 y of age (upon vaccine protection waning). If the mean duration of vaccine protection was assumed 20 y, then routine booster applied to seronegative women at 30 y of age (upon vaccine protection waning). In the case of infant vaccination with booster, a routine booster was set at 10 y of age.
In the present simulations, we considered vaccine coverage of 30% (conservative case) and 70% (best case). Target vaccine efficacy was considered 70% (desirable) and 90% (ideal case), with vaccine-induced duration of protection of 10 and 20 y. Our main interest was to assess reduction of congenital CMV infection in each vaccination scenario under the different combinations of these assumed target vaccine characteristics (coverage, efficacy and duration of protection).
Of note, all the hypothetical vaccination scenarios presented here were defined a priori, at the start of the actual analyses and before running any model simulations. In particular, the age of 10 y for routine adolescent vaccination was selected a priori of interest as a potential early age that could be aligned with the existing HPV vaccination platform in the US, where 2 HPV vaccines (Gardasil™ and Cervarix™) are currently in-use for females starting at 9 y of age, given in 3 doses over 6 months, with current routine vaccination recommended by the Advisory Committee on Immunization Practices around the age of 11–12 y. The vaccine coverage rates considered a priori of potential interest here were also based on prior experiences with the HPV vaccine implementation globally. A vaccine efficacy of 70% was also considered in ref., Citation23 while the selected 90% value was a priori defined as an ideal case for comparison purposes.
The most relevant results are shown in , illustrating the model-projected reduction in congenital CMV infection birth prevalence over time post-vaccination under different vaccination scenarios. The magnitude of reduction clearly will depend on specific vaccine characteristics: efficacy, coverage, duration of protection, and the various possible subsequent combinations.
The outcomes in correspond to scenario 1 in : seronegative adolescent female vaccination without booster, with a one-time catch-up in 10–17 y old at start-up, in the base-case vaccination assuming no vaccine impact on reactivation. This is a worst-case scenario in terms of reducing congenital CMV infection, where even in the best case (case 3), with 70% vaccine coverage, 90% vaccine efficacy and 20 y duration of protection, only a reduction of about 5%, 15% and 18% in congenital CMV infection from baseline pre-vaccination could be achieved after 10, 20 and 50 y post-vaccination, respectively. Vaccination impact is mainly driven by direct protection effects in this case. Vaccine duration of protection is a key factor here for adolescent women vaccination, as longer durations of protection can extend protection against infection into the child-bearing age, critical for subsequently reducing congenital CMV infection. This could be achieved in practice by appropriate booster platforms later in life.
Table 1. Vaccine scenarios
Figure 2. Model-projected congenital CMV infection birth prevalence over time post-vaccination of seronegative adolescent females without booster, with one-time catch-up in 10–17 y old at start-up, under scenario 1 in the base-case vaccination framework (no vaccine impact on reactivation).
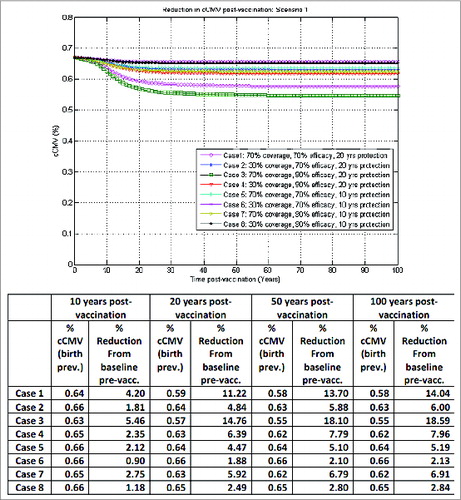
Figure 3. Model-projected congenital CMV infection birth prevalence over time post-vaccination of seronegative adolescent females with booster, one-time catch-up in 10–17 y old at start-up, under scenario 3 in the base-case vaccination framework (no vaccine impact on reactivation).
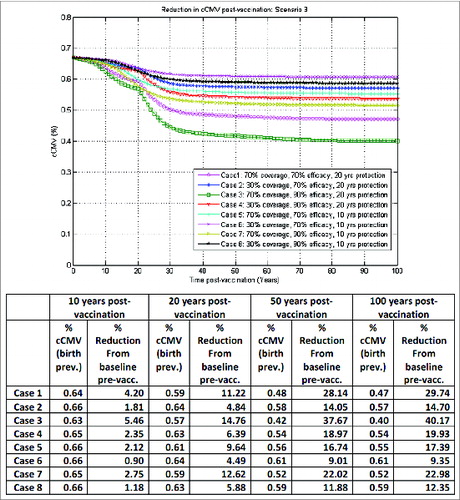
Figure 4. Model-projected congenital CMV infection birth prevalence over time post-vaccination of both seronegative and seropositive adolescent females without booster, one-time catch-up in 10–17 y old at start-up, under scenario 2 in the optimistic vaccination framework (with vaccine impact on reactivation).
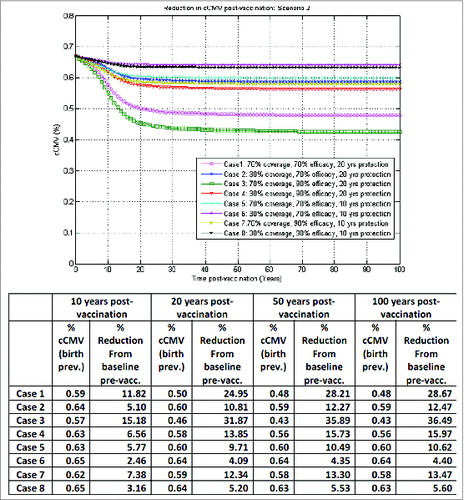
Figure 5. Model-projected congenital CMV infection birth prevalence over time post-vaccination of both seronegative and seropositive adolescent females with booster, one-time catch-up in 10–17 y old at start-up, under scenario 4 in the optimistic vaccination framework (with vaccine impact on reactivation).
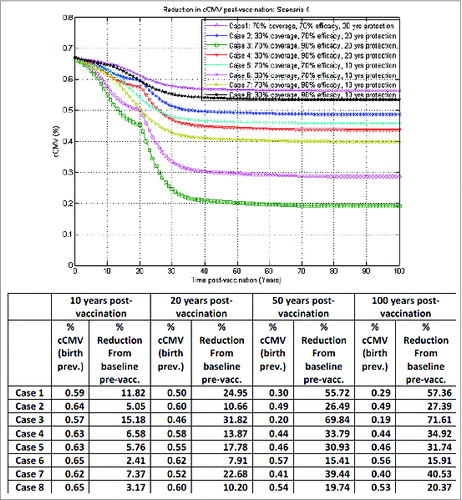
The importance of a booster platform is illustrated in , corresponding to scenario 3 in : seronegative adolescent female vaccination with booster and a one-time catch-up in 10–17 y old at start-up, in the base-case vaccination assuming no vaccine impact on reactivation. In our simulations here, routine booster at the appropriate age is not only being administered to previously vaccinated women with waned immunity, but also equally to those who have not been previously vaccinated. In the best case (case 3), with 70% vaccine coverage, 90% vaccine efficacy and 20 y duration of protection, with a one-time catch-up in 10–17 y old at start-up and routine booster later in life, the reduction in congenital CMV infection from baseline pre-vaccination could go up to about 38% 50 y after vaccination, compared to only 18% before in the same case but without a routine booster platform, also highlighting that the impact of implementing a booster platform later in life for female vaccination would only become more apparent in the longer term.
If the vaccine was assumed to have no impact on reactivation, vaccinating seropositive in addition to seronegative adolescent females did not add notable benefits for overall reduction of congenital CMV infection. However, in an optimistic vaccination framework, the outcomes can be significantly improved if the vaccine had a similar efficacy on both infection acquisition and reactivation, acting by reducing both the risk of infection and the likelihood of reactivation in vaccinated protected individuals.
Such outcomes are illustrated in corresponding to scenario 2 in : seronegative and seropositive adolescent female vaccination without booster and a one-time catch-up in 10–17 y old at start-up, now in the optimistic vaccination framework, assuming equal vaccine impact on both infection acquisition and reactivation. In the best case (case 3) here, with 70% vaccine coverage, 90% vaccine efficacy and 20 y duration of protection, a reduction of about 15%, 32% and 36% in congenital CMV infection from baseline pre-vaccination could be achieved 10, 20 and 50 y, respectively, after vaccination. With the addition of an appropriate routine booster platform, as before, the reduction in congenital CMV infection 50 y after vaccination could go up to about 70%, as illustrated in .
Clearly, illustrates the outcomes for congenital CMV infection reduction in a best-case vaccination framework for adolescent female vaccination, while by contrast depicts a worst-case vaccination framework for adolescent female vaccination.
The variability in outcomes between these 2 extremes here is considerable.
By contrast, the model-based projections in the case of infant vaccination under scenario 5 in , in the base-case vaccination assuming no vaccine impact on reactivation, indicate that routine infant vaccination without booster or catch-up may provide a reduction in congenital CMV infection of about 18%, 30% and 68% from baseline (pre-vaccination) at 10, 20, and 50 y post-vaccination, respectively, in the worst case (30% vaccine coverage, 70% vaccine efficacy and 10 y duration of protection, e.g., case 6 in ), while in the best case (70% vaccine coverage, 90% vaccine efficacy and 20 y duration of protection, e.g. case 3 in ) 33%, 47%, and 97% reduction from baseline (pre-vaccination) at 10, 20, and 50 y post-vaccination, respectively, could be achieved. Duration of protection in this case is less critical than above in the adolescent female vaccination framework, provided it is long enough for the young children to remain protected for a critical time-window (e.g., up to 5 y of age). Adding a booster dose at 10 y of age or a catch-up campaign in this case did not make any further significant impact; neither did vaccine impact on reactivation (results not shown here). Elimination could in theory be achieved at 30% vaccine coverage, 90% vaccine efficacy and 10 y vaccine duration of protection, for instance, but it would take well beyond 100 y post-vaccination. This however corresponds to an ideal case where there would be no importation of the virus; in more realistic simulations, if importation of the virus is mimicked for instance via a small percentage in the force of infection not directly caused by person-to-person transmission (results not shown here), then elimination is no longer possible.
Discussion
We proposed and tested a complex dynamic transmission model for CMV in the US population, where we primarily aimed to combine the available qualitative and quantitative knowledge toward more complex modeling frameworks to better reflect the underlying biology and epidemiology of the CMV infection. A few other valuable mathematical models to explore potential impact of vaccination at population-level and rationalize implementation of potential vaccination strategies existCitation22-24. Our modeling framework has added significant complexity in a first attempt to integrate additional desirable features into a CMV dynamic modeling framework. Novel features include:
– | fine age structure and realistic demography | ||||
– | gender stratification | ||||
– | distinction between primary infections, reactivations and re-infections, with differential impact on congenital CMV | ||||
– | age-dependent infectiousness and reactivation | ||||
– | transmission estimates based on empirical social contact patterns | ||||
– | model calibration framed as a sophisticated constrained optimization problem, based on the currently available relevant information further translated into corresponding constraints to enforce epidemiological plausibility | ||||
– | methodology not restricted to steady-state and can be applied in the presence of longitudinal data as well | ||||
– | versatility, allowing for potential consideration of different vaccine modes-of-action (e.g., reduced susceptibility, reduced infectiousness, reduced risk of mother-to-fetus transmission). |
The modeling framework presented in this paper was designed to be sufficiently general and flexible, such that it can be adapted to different settings and data-sets, and also readily allow for further changes to reflect new knowledge that may become available in the future. We do not regard this work as exhaustive or final, but rather as a starting point toward challenging and advancing the status quo in the field, highlighting the need for more studies and data necessary to bring such modeling tools closer to robust quantitative validations and subsequent use to help inform decision making.
The calibrated baseline model successfully reproduced the NHANES III CMV age-stratified seroprevalence data, while simultaneously aligning with other reports.Citation1,2,11,22-24 For simplicity, and in the absence of compelling data indicating otherwise, the baseline model was calibrated at steady-state, under an assumption of an endemic equilibrium of the prevalence of CMV infection in the US population, supported by the limited available data.Citation10
The parameter estimation problem related to the baseline model calibration (e.g., in the absence of vaccination) was framed here as a fairly constrained optimization problem, where qualitative and quantitative available information was translated in constraints, in order to reduce the number of possible combinations. Other dynamic CMV transmission models at population level have worked with simpler assumptions of constant average duration of infectiousness and reactivation rate. In ref. Citation22 the authors used different values for the average duration of infectiousness, including a 6-month duration (in-line with other published reports e.g. ref. Citation27) that was subsequently employed in a similar manner in an adaptation of the model by the Centers for Disease Control and Prevention in the US.Citation23 The 6-month duration of infectiousness was also the estimate that yielded the best fit against the seroprevalence data for our baseline model in the older age groups. Regarding the reactivation rate, the assumption of an S-shaped function characterized by a gradual increase from low levels at young ages to higher levels at old age appeared more biologically realistic to us than just a constant rate across all age groups. This assumption is based on qualitative information that seem to indicate that aging of the immune system may correlate with a progressive decreased ability to properly control CMV reactivation over time, a biological process often referred to as immunesenescence.Citation28-30 A constant reactivation is a simpler assumption that has been considered in other models.Citation22-24 In either case, to our knowledge, there is no direct data that could be readily and robustly used for actual quantitative estimates, which still need to be found via calibration.
As explained in our Methods section, POLYMOD social contact data for physical contacts longer than 15 minutes was employed in this modeling framework solely as a plausible empirical support for transmission for CMV in the US population, under the assumption that the social contact patterns are not very different in developed countries and in EU vs. US. For actual infection transmission, we introduced a correction factor, which is a bivariate parametric function, on top of the social contacts. In the Methods section, we provided a justification for the choice that in this instance led to the best fit against the age-stratified seroprevalence data, but in reality, it is not always easy to attach an appropriate biological rationale to the mathematical expression of this correction factor, and its definition is not obvious a priori. The final form we eventually converged to here, given in the Methods section, was basically reached via an educated trial-and-error process. In a related simpler model in ref., Citation22 the authors actually proposed an ad-hoc bivariate parametric function for the transmission matrix for CMV, which was calibrated based on seroprevalence data from Brazil, also yielding a bimodal shape of the force of infection with respect to age, dominated by a sharp peak in the youngest and followed by a secondary peak later in life.
The profile of the model-based force of infection as a function of age displays some qualitative trends similar to those previously reported in ref. Citation22 However, in our model projections, the magnitudes of the primary and secondary peaks are considerably closer, with the primary peak in the very young individuals only about 1.5 times higher than the secondary peak occurring later in life. These differences between the age-specific profiles of the force of infection between our model and those reported by the authors in ref. Citation22 are reflective of the differences in the age-specific CMV seroprevalence data from US and Brazil, respectively. In the US, the seroprevalence data shows an almost linear increase with age, while the seroprevalence data from Brazil exhibits a more classical sigmoid shape, with a rapid increase in early childhood followed by plateauing around the age of 30 y.Citation22,31 As a consequence, the average age of infection is likely to be considerably lower in Brazil (estimated at 19.84 y in ref. Citation31, based on seroprevalence data for ages 10−40 y old) compared to US (estimated at 28.6 y in ref. Citation2 based on seroprevalence data for ages 12−49 y old). Intuitively, the secondary peak in our current US model corresponds to an enhanced risk of infection in a population most likely to have close physical contact with young children. Fertility rates in the US are about 3 times higher in the 25−30 y old than in the 15−19 y old.Citation32 In addition, at calibration, we did not only fit the age-stratified seroprevalence data in ref. Citation25 but also the CDC-reported birth prevalence of congenital CMV infection,Citation9 along with the estimated value provided in the literature for the force of infection in the 12−49 y old2 with a number of appropriate constraints as described in the Methods section under Model calibration and validation. The third peak projected by our model here in the older ages is likely a reflection of the still increasing seroprevalence in old age groups, as indicated by the age-stratified seroprevalence data, combined with the assumed empirical contact pattern. Removing the third peak or constraining it to be flatter leads to less good fits against the seroprevalence data in the old age groups; in other words, in order for the seroprevalence curve to keep going up in old age groups, as indicated by the data, something needs to drive it, which in practice may be a consequence of likelihood to have and interact with young grandchildren, for instance. In the present context, however, where our focus is on congenital CMV infection, such potential peaks in the older age groups have little practical impact, as transmission from this segment or likelihood of first-generation offspring are low. We would like to also note here that the force of infection shown in is estimated based on a fine age structure, as enabled by the model, and thus the usual smoothing that naturally occurs in practice by pooling larger age groups is not present here.
To our knowledge, no gold standards currently exist for the shape of the force of infection as a function of age. Traditionally, under the assumption of steady-state, simpler catalytic models can be employed to render a profile for the force of infection with respect to age so that the age-stratified seroprevalence data is matched. However, such approaches often imply using pre-defined mathematical expressions involving a number of unknown parameters that are estimated by fitting the available seroprevalence data. This is closer to a curve-fitting exercise, with limited epidemiological substance, and different assumed profiles may lead to similar fits against the seroprevalence data.Citation33 In our case, in the absence of strong evidence, no prior assumption was made about the shape of the force of infection, and the resulting profile is a model outcome based on all the complex underlying epidemiological information included in the modeling framework. This approach is not methodologically restricted to steady-state assumptions, and can well be employed in the presence of longitudinal seroprevalence data as well. If strong evidence becomes available that could be used to enforce more plausible or expected profiles of the force of infection as a function of age, then this can and should be added in the list of constraints for model calibration, and model re-calibrated accordingly. This would naturally impact subsequent model projections for vaccination.
Regarding the model with vaccination, our objective was to use a more realistic calibrated model toward assessing the potential impact of vaccination on reducing congenital CMV infection at population-level. There are many various vaccination scenarios that could be simulated in this modeling framework; for our main goals for the time being, however, the scope was restricted to some illustrative analyses that were a priori considered of primary interest in the context. We want to further reinforce the fact that our aim here was not to find critical threshold values for vaccine efficacy or coverage or age at vaccination that may impact cost-effectiveness analyses and subsequent decision making. Cost-effectiveness analyses and selection of cost-efficient strategies, etc. were out of the scope of the current work. While epidemiological modeling frameworks need to be challenged and evolve toward more realistic ones, this evolution needs to be accompanied by additional studies and data needed further for more robust model assessment and validation to facilitate the appropriate choice of model for actual decision making.
In line with other reports,Citation34,35 our preliminary simulations presented here indicated that infant vaccination may lead to a more efficient reduction in congenital CMV infection over time compared with adolescent female vaccination. The model projected a reduction of congenital CMV infection at 50 y post-vaccination in the case of routine vaccination of all infants 6 months of age, irrespective of their serological serostatus and without any catch-up or booster dose (), ranging from 68% to 97% across the 8 combinations of vaccine coverage, efficacy and duration of protection considered. By comparison, in an optimistic vaccination framework assuming equal vaccine impact on infection acquisition and reactivation, routine adolescent vaccination of both seropositive and seronegative females at the age of 10 y without booster, with a one-time catch-up in 10–17 y old at start-up, (), may lead to congenital CMV infection reductions from baseline (pre-vaccination) of 4% to 36% at 50 y post-vaccination. In the same optimistic vaccination framework, adding on top of routine female adolescent vaccination an adequate routine booster platform for vaccination of both seronegative and seropositive females later in life (with a one-time catch-up in 10–17 y old at start-up) () may lead to congenital CMV infection reductions from baseline (pre-vaccination) of 15% to 70% at 50 y post-vaccination, depending on the combinations of vaccine coverage, efficacy and duration of protection considered. In an ideal case scenario, results for adolescent female vaccination here could be further improved in the event that newborns of vaccinated and protected mothers may also be temporarily protected against infection (not presented).
For all practical purposes, while model-based projections were shown here up to 100 y post-vaccination for a more complete picture, in reality it is highly likely that the validity of such projections decreases significantly with time, as other natural epidemiological changes are likely to occur over time, which are not considered here.
In our present modeling framework, due to the underlying transmission matrix combined with longer duration of infectiousness in children younger than 5 y of age, notable herd protection effects could only be achieved if there is some level of vaccine-induced protection in this particular age group. Even at relatively low effective coverage (e.g., around 30%) of vaccination in infants, for instance, subsequent impact on transmission and herd protection can be significant, with impact on congenital CMV infection reduction already apparent at 10 y post-vaccination. By contrast, such effects cannot be achieved in our current modeling framework solely via adolescent female vaccination, where impact of vaccination on congenital CMV infection reduction appears mostly driven by direct protection effects in vaccinated women of child-bearing age.
However, if vaccinating young children may not be feasible, the alternative could remain for adolescent female vaccination with booster, where aspects like vaccine impact on reactivation may play an important role.
Model-based simulations in ref. Citation22 indicated that the best strategy in preventing congenital CMV infection in Brazil may be universal CMV vaccination before the age of 12 months followed by a booster dose at 10–11 years, assuming duration of protection of at least 20 y. However, the same modelCitation22 also suggested that in the Brazilian setting, infant vaccination without booster could potentially increase congenital CMV infection if vaccine duration of protection is lower than 20 y. Preliminary analysis in refs. Citation23, 24 based on the previous Brazilian modeling framework from ref. Citation22 and adapted to the US data suggested that such a scenario would not occur in the US settings, even at lower duration of vaccine protection (e.g. 5 y or less), and argued that shorter duration of vaccine protection may in some cases have some adverse effects on congenital CMV infection in high CMV seroprevalence settings such as Brazil. In our model-based simulations, we did not find evidences supporting a potential negative impact of vaccination on congenital CMV infections in the US setting in the vaccine scenarios considered.
The vaccine duration of protection is a key factor that can have a big impact on subsequent model projections, particularly in the adolescent female vaccination framework, and we have to acknowledge that the values considered in our analyses here, of 10 and 20 y duration of vaccine-induced immunity respectively, may well be overly optimistic. However, based on the HPV vaccine experience, where long term (>6 y) immunity has been demonstrated,Citation36 we considered the 10 y duration of protection a desirable value, albeit optimistic. For practical purposes, this could be achieved with 5 y duration of protection followed by routine booster for an additional 5 y duration of protection, for instance. The 20 y duration of protection here represents an idealized case, for comparison purposes.
Given the high dimensionality and nonlinear dynamic nature of the problem, different sensitivity analyses can be envisioned in theory, resulting in complex and computationally-intense exercises. In order to retain value and maintain tractability, they need to be systematic and well defined in scope; to that end, further studies are necessary to collect and collate such data in order to ascertain actual ranges and variability for the different input parameters so as to guide sensitivity analyses. For the baseline model here, we performed a constrained optimization in a parameter space, with the forward problem governed by a fully deterministic model, and we found a set of coupled model parameters that led to the best fit against all available quantitative data. This procedure implicitly means we have searched through the parameter space and ultimately chose an optimal set. There is, clearly, the typical issue that solution of this optimization problem may not be unique, and multiple sets of model parameters (different combinations) may lead to equivalent best fits against the data. We have run the optimization exercise starting from different initial points in the parameter space, with different algorithms (simplex, gradient-based, etc.), and retrieved equivalent solutions in terms of overall system behavior.
In general, for nonlinear and complex models, univariate sensitivity analysis is insufficient for a comprehensive study of the model.Citation37 Multivariate sensitivity analysis is more appropriate, based on the inherent coupling and nonlinear relationships in the model. When parameter values have large uncertainties or models are nonlinear, global sensitivity analysis techniques are more appropriate.Citation38 Global sensitivity analysis techniques investigate the effects of simultaneous parameter variations over large but finite ranges and allow the effects of interactions between parameters to be explored.Citation38 Such analyses constitute a significant piece of work themselves and can make the object of subsequent manuscripts. In the current work, we focused on developing the model, calibrating it, and performing comparative analyses with respect to vaccination parameters under a few different vaccination scenarios; this already resulted in a large amount of information that needed to be interpreted and illustrated in a coherent and concise manner in the present manuscript.
Further work here could also involve various additional analyses on model outcomes including investigating impact of the actual transmission matrix or contact patterns, which in itself could make the object of a separate piece of work. For example, Van Effelterre et al in ref. Citation39 conducted a comparison looking at the impact of the transmission matrix in the context of varicella using more established approaches, in a stand-alone dedicated paper. The work that we presented here is not intended as exhaustive or definitive, but rather as a proof of concept and a starting point.
In summary, we aimed to develop a more realistic CMV transmission modeling framework with novel features, bringing together in an integrated fashion qualitative and quantitative current knowledge related to the CMV infection. The work presented here is intended as a step toward more advanced frameworks that can more realistically reflect the biology and epidemiology of CMV infection. After calibration and validation against available CMV epidemiological data from the US, the proposed modeling framework was employed to assess the potential impact to reduce congenital CMV infection at population level via vaccination with a hypothetical CMV vaccine. In the analyses presented here, we tested some CMV vaccination scenarios that were considered a priori of potential primary interest in the context, in an attempt to illustrate some of the current challenges associated with developing and implementing a CMV vaccine.Citation40
Our results are based on a number of assumptions which we believe adequately reflect the qualitative knowledge related to CMV infection at present, with model calibration performed as a complex constrained optimization problem where all available relevant information is translated into corresponding model constraints to enforce biological and epidemiological plausibility. However, further studies and analyses are needed for more robust testing and validations in order to increase the level of confidence necessary for such modeling and simulation tools to further help inform decision making. In the absence of stronger evidence that can help select the most appropriate model, different modeling exercises can be conducted with potentially different outcomes.
Ultimately, projections from well validated epidemiological models will need to be evaluated from a cost-effectiveness point of view to complete the picture and support informed decisions. This was beyond the scope of the current work.
Finally, we want to acknowledge that the mechanistic deterministic modeling approach proposed here, while appealing because of its built-in tractability, has some limitations in the sense that everything is assumed as an average at the population level, with no explicit account for various sources of heterogeneity, variability or uncertainty. Alternatives such as individual-based models could be considered if finer levels of granularity (e.g., enhanced transmission in day-care settings, household transmission, differential impact of socio-economic status, individual risks, etc.) are of interest.
Materials and Methods
Overview of the mathematical model
The dynamic transmission modeling framework presented here is mechanistic and deterministic. The model structure, with underlying states and corresponding flows as illustrated in , is designed to reflect qualitatively the known biology of CMV infection. The US population is stratified by gender and by vaccination status (vaccinated/not vaccinated). For each of these sub-populations, the model is further stratified to account for the natural history of CMV, using the following mutually disjoint states: susceptible (seronegative), primary infected (infectious, seropositive), latent (seropositive, non-infectious), endogenously reactivated (seropositive, infectious), exogenously re-infected (seropositive, infectious). For completeness, we also include a state allowing for the possibility (albeit small) that some seropositive individuals may be both reactivated (endogenously) and re-infected (exogenously).
Modeling assumptions
Baseline model
– | The average duration of infectiousness | ||||
– | To account for the fact that CMV reactivation may increase with age,Citation45 the rate of endogenous reactivation |
From a qualitative biological point of view, the choice of such an S-shape for the reactivation was based on the hypothesis that the potency of the immune system to control viral replication in the event of a reactivation changes with age, with relatively higher ability of the immune system to control the virus early in life, and which gradually becomes less and less potent leading to more frequent reactivation events or even a state of chronic infection in older individuals.Citation28 While we acknowledge the absence of detailed quantitative data demonstrating this, we found numerous qualitative elements from the immunosenescence research field that are supportive of such an assumption (e.g., refs. Citation29, 30). Over lifetime, the CMV-specific T cells appear to become gradually less and less efficient in controlling the viral replication (accumulation of high avidity clones with exhausted/dysfunctional profile and progressive accumulation of lower avidity clones that in turn also become dysfunctional). From this understanding of the immunological control of the viral replication, we concluded it is likely that earlier in life, the immune system is able to control reactivations more efficiently than later on (e.g., > 60 y of age). Thus, an S-shaped function, characterized by a gradual increase from low levels at young ages to higher levels at old age, appears to be more biologically plausible for reactivation than just a constant rate across all age groups.Citation28 A flat (constant) reactivation is just a particular (simpler) case that has been considered in other models.Citation22-24
– | CMV is transmitted through direct close physical contact of a susceptible person with oral fluids from infected individuals actively shedding the virus. CMV transmission is facilitated by social mixing. In order to capture adequate mixing between people of different ages, we employed an empirical social contact matrix (based on POLYMOD,Citation46 courtesy of Dr. Niel Hens, Hasselt University, Belgian data) for close physical contacts longer than 15 minutes. We assumed that sexual interactions are implicitly represented in this type of contacts. Such empirical matrices are employed here only as a mean to render contact patterns, assuming similar social contact patterns in Western European countries and the US and scaling appropriately for the US age-stratified demography. While the POLYMOD-type empirical social contact matrices can provide a basic idea about the frequency of social contacts between individuals in different age groups, they do not reflect more infection-specific age characteristics.Citation47 To take this aspect into account, the frequency of social contacts between individuals in different age groups can be multiplied by a “proportionality factor”,Citation47,48 generally modeled as a bivariate parametric function | ||||
– | As considerable evidence indicates that seropositivity induced by a wild-type virus may provide protection against re-infection,Citation3 we modeled this through a corresponding natural reduction factor k in the force of infection for re-infection, as illustrated in . This reduction factor is estimated at calibration. | ||||
– | Congenital CMV infection is modeled through a risk function with 2 parameters accounting for the mother-to-fetus transmission rate following primary maternal infection vs. recurrent maternal infection, respectively, estimated at calibration. Age-specific fertility rates, included as customaryCitation49 in the underlying demographic model based on US Census data, are used to account for the impact of women's age on the corresponding birth rate. |
In summary, for the baseline model, our current framework involves 10 model parameters, whose point-values are estimated at calibration. This is discussed further below.
Vaccination model
One of the goals of this work was to employ a complex modeling framework, designed to reflect the biology and epidemiology of CMV infection, toward exploring the potential impact of a vaccine aimed primarily at preventing CMV infection to subsequently reduce the burden of congenital CMV infection at population level.
The ‘Vaccinated’ component in the model structure replicates the states and corresponding flows from the baseline model, as illustrated in .
– | In a base-case framework, the vaccine mode-of-action would be to reduce the risk of a susceptible vaccinated individual to acquire infection compared to a susceptible unvaccinated individual. Then the ‘vaccine efficacy’ (Ve) denotes a corresponding reduction in the risk of acquiring infection if vaccinated. | ||||
– | The modeling framework is sufficiently versatile to enable consideration of various other potential vaccine modes-of-action, like reduced reactivation rates or reduced infectiousness in vaccinated compared to unvaccinated individuals, or lower mother-to-fetus transmission in vaccinated infected mothers compared to unvaccinated infected mothers. Our base-case vaccination framework illustrated in corresponds to a situation where the vaccine has no impact on reactivation and where all the corresponding flows in the ‘Unvaccinated’ and ‘Vaccinated’ are similar except for a reduction in the corresponding force of infection in the ‘Vaccinated’ component, as highlighted in the explanatory table in . For completeness, however, in our actual simulations we also considered an optimistic vaccination framework where the vaccine would have similar efficacy against reactivation as well, modeled as a corresponding reduction in the reactivation rate in the vaccinated seropositive individuals. | ||||
– | We assumed similar infectiousness for vaccinated and unvaccinated individuals. Vaccinated mothers still experiencing primary or recurrent infection during pregnancy are assumed to have the same risks as their unvaccinated counterparts of giving birth to an infant with congenital CMV infection. In other words, the risk of congenital CMV in the newborns considered here only depends on the infection status of the mother and is independent of the mother's vaccination status. |
Mathematical framework
We employed a mathematical model formulation with continuous age structure and governed by a corresponding set of nonlinear Partial Differential Equations with respect to time and age, as illustrated in Supplemental File 2.
All the outcomes presented are based on corresponding numerical solutions with an age discretization of 1 year-step for all ages >1 y old and <86 y old (which is the assumed life expectancy in this case), and a 6 months-step for the 0–1 y old segment.
Model calibration and validation
Nationally representative age-stratified CMV seroprevalence data for the US have been collected as part of the National Health and Nutrition Examination Survey (NHANES) system and are currently published for 2 time periods: 1988−1994 and 1999−2004.Citation10,25 As no major differences in CMV seroprevalence were observed between these 2 time periods, for simplicity, we assumed endemic equilibrium and we calibrated the baseline model at steady-state vs. the published 1988−1994 NHANES III data for ages 6 to 80+ y, reported in the paper published by Staras et alCitation25 with the appropriate level of detail for the age stratification in the overall US population needed for our purposes. Seroconversion data from Yow 1986,Citation50 which are robust for infants in the first year of life and overall consistent with the NHANES III CMV seroprevalence data for the individuals aged 6−10 y, can be used as a check-point to provide additional information on the age-stratified CMV seroprevalence curve in infants (0−1 y of age).
As described in the Modeling assumptions, 10 model parameters have to be estimated at calibration. The parameter estimation problem here is formulated as a complex constrained optimization problem. Available qualitative and quantitative information is translated into appropriate mathematical constraints used to ensure both biological and epidemiological plausibility and to reduce the likelihood of over-fitting.
The constraints used for model calibration are based on the most recent publicly available estimates related to CMV infection in the US.Citation9,11 Model projections here are considered plausible when leading to 1−4% of seronegative pregnant women experiencing a primary infectionCitation9 with a subsequent mother-to-fetus transmission rate of 30–40%,Citation9 while simultaneously estimating a percentage of seropositive mothers with CMV-positive newborns around 1%Citation11 with an overall target birth prevalence of congenital CMV infections set at 0.67%.Citation9 Regarding the natural reduction k in the risk of an individual to acquire a new infection, due to natural immunity, we varied it between 60% and 70% at calibration, guided by information in refs. Citation3, 51.
In addition, we also considered the value of the force of infection among the US population ages 12−49 y as an additional soft constraint, estimated at a point value of 1.6/100 (with upper bound for the 95% confidence interval around 2/100) per year in ref. Citation2 which was also based on the 1988–1994 NHANES III CMV seroprevalence data in conjunction with a statistical model.
All our numerical simulations are performed in MATLAB 2010b, the MathWorks, Inc., Natick, Massachusetts, United States.
Disclosure of Potential Conflicts of Interest
All authors are employed by the GSK group of companies and own stock options in GSK.
Authors' Contributions
All authors contributed to define the objectives and global model structure. C. Hogea designed the model structure and the underlying mathematical and computational tools. C. Hogea and I. Dieussaert participated to the collection and assembling of the data. C. Hogea, I. Dieussaert and J. Mols performed and/or supervised the analysis. All authors had full access to the data, contributed to the interpretation of the results, reviewed and approved the present manuscript for submission.
Trademark Statements
Gardasil is a trade mark of Merck & Co. Inc.. Cervarix is a trade mark of the GSK group of companies.
Supplemental Material
Supplemental data for this article can be accessed on the publisher's website.
Supplemental_Files.pdf
Download PDF (645.6 KB)Acknowledgments
All authors would like to thank Dr. Niel Hens, Hasselt University, Diepenbeek, and University of Antwerp, Belgium, for providing generic European contact rates POLYMOD-based. They also thank Dr. Adriana Rusu (XPE Pharma & Science on behalf of GSK Vaccines) who provided editorial support and Dr. Sylvie Hollebeeck and Dr. Marie-Line Seret (XPE Pharma & Science on behalf of GSK Vaccines) for publication coordination and management.
Funding
GlaxoSmithKline Biologicals SA was the funding source and supported all costs associated with the development and the publishing of the present manuscript.
References
- Kenneson A, Cannon MJ. Review and meta-analysis of the epidemiology of congenital cytomegalovirus (CMV) infection. Rev Med Virol 2007; 17:253-76; PMID:17579921; http://dx.doi.org/10.1002/rmv.535
- Colugnati FA, Staras SA, Dollard SC, Cannon MJ. Incidence of cytomegalovirus infection among the general population and pregnant women in the United States. BMC Infect Dis 2007; 7:71; PMID:17605813; http://dx.doi.org/10.1186/1471-2334-7-71
- Adler SP, Nigro G. Clinical cytomegalovirus research: Congenital Infection. Cytomegaloviruses: From Molecular Pathogenesis to Intervention. London: Caister Academic Press; 2013. p. 60-61. Reddehase MJ, Lemmermann N, editors. vol 2.
- Malm G, Engman ML. Congenital cytomegalovirus infections. Semin Fetal Neonatal Med 2007; 12:154-9; PMID:17337260; http://dx.doi.org/10.1016/j.siny.2007.01.012
- Centers for Disease Control and Prevention. Cytomegalovirus (CMV) and Congenital CMV Infection (http://www.cdc.gov/cmv/clinical/features.html). Accessed 26 July 2013.
- Engman ML, Malm G, Engstrom L, Petersson K, Karltorp E, Tear Fahnehjelm K, Uhlen I, Guthenberg C, Lewensohn-Fuchs I. Congenital CMV infection: prevalence in newborns and the impact on hearing deficit. Scand J Infect Dis 2008; 40:935-42; PMID:18720260; http://dx.doi.org/10.1080/00365540802308431
- Hyde TB, Schmid DS, Cannon MJ. Cytomegalovirus seroconversion rates and risk factors: implications for congenital CMV. Rev Med Virol 2010; 20:311-26; PMID:20645278; http://dx.doi.org/10.1002/rmv.659
- de Vries JJ, van Zwet EW, Dekker FW, Kroes AC, Verkerk PH, Vossen AC. The apparent paradox of maternal seropositivity as a risk factor for congenital cytomegalovirus infection: a population-based prediction model. Rev Med Virol 2013; 23:241-9; PMID:23559569; http://dx.doi.org/10.1002/rmv.1744
- Centers for Disease Control and Prevention. Congenital CMV Infection Trends and Statistics (http://www.cdc.gov/cmv/trends-stats.html). Accessed 26 July 2013.
- Bate SL, Dollard SC, Cannon MJ. Cytomegalovirus seroprevalence in the United States: the national health and nutrition examination surveys, 1988–2004. Clin Infect Dis 2010; 50:1439-47; PMID:20426575; http://dx.doi.org/10.1086/652438
- Cannon MJ. Congenital cytomegalovirus (CMV) epidemiology and awareness. J Clin Virol 2009; 46(Suppl 4):S6-10; PMID:19800841; http://dx.doi.org/10.1016/j.jcv.2009.09.002
- Manicklal S, Emery VC, Lazzarotto T, Boppana SB, Gupta RK. The “silent” global burden of congenital cytomegalovirus. Clin Microbiol Rev 2013; 26:86-102; PMID:23297260; http://dx.doi.org/10.1128/CMR.00062-12
- Boppana SB, Pass RF, Britt WJ, Stagno S, Alford CA. Symptomatic congenital cytomegalovirus infection: neonatal morbidity and mortality. Pediatr Infect Dis J 1992; 11:93-9; PMID:1311066; http://dx.doi.org/10.1097/00006454-199202000-00007
- Dollard SC, Grosse SD, Ross DS. New estimates of the prevalence of neurological and sensory sequelae and mortality associated with congenital cytomegalovirus infection. Rev Med Virol 2007; 17:355-63; PMID:17542052; http://dx.doi.org/10.1002/rmv.544
- Foulon I, Naessens A, Foulon W, Casteels A, Gordts F. A 10-year prospective study of sensorineural hearing loss in children with congenital cytomegalovirus infection. J Pediatr 2008; 153:84-8; PMID:18571542; http://dx.doi.org/10.1016/j.jpeds.2007.12.049
- Dahle AJ, Fowler KB, Wright JD, Boppana SB, Britt WJ, Pass RF. Longitudinal investigation of hearing disorders in children with congenital cytomegalovirus. J Am Acad Audiol 2000; 11:283-90; PMID:10821506
- Cannon MJ, Davis KF. Washing our hands of the congenital cytomegalovirus disease epidemic. BMC Public Health 2005; 5:70; PMID:15967030; http://dx.doi.org/10.1186/1471-2458-5-70
- Pass RF, Zhang C, Evans A, Simpson T, Andrews W, Huang ML, Corey L, Hill J, Davis E, Flanigan C, et al. Vaccine prevention of maternal cytomegalovirus infection. N Engl J Med 2009; 360:1191-9; PMID:19297572; http://dx.doi.org/10.1056/NEJMoa0804749
- Krause PR, Bialek SR, Boppana SB, Griffiths PD, Laughlin CA, Ljungman P, Mocarski ES, Pass RF, Read JS, Schleiss MR, et al. Priorities for CMV vaccine development. Vaccine 2013; 32:4-10; PMID:24129123; http://dx.doi.org/10.1016/j.vaccine.2013.09.042
- Dasari V, Smith C, Khanna R. Recent advances in designing an effective vaccine to prevent cytomegalovirus-associated clinical diseases. Expert Rev Vaccines 2013; 12:661-76.
- Griffiths P, Plotkin S, Mocarski E, Pass R, Schleiss M, Krause P, Bialek S. Desirability and feasibility of a vaccine against cytomegalovirus. Vaccine 2013; 31(Suppl 2):B197-203; PMID:23750795; PMID:23598482; http://dx.doi.org/10.1016/j.vaccine.2012.10.074
- Azevedo RS, Amaku M. Modelling immunization strategies with cytomegalovirus vaccine candidates. Epidemiol Infect 2011; 139:1818-26; PMID:21396147; http://dx.doi.org/10.1017/S0950268811000343
- Lanzieri T. Modelling the impact of vaccination on the epidemiology of CMV and congenital CMV infection in the United States. San Francisco: CMV meeting; 2012
- Lanzieri TM, Gambhir M, Ortega-Sanchez I, Bialek SR. Modeling the Impact of Vaccination on the Epidemiology of Cytomegalovirus (CMV) and Congenital CMV Infection in the United States - Preliminary Findings. The Fifteen Annual Conference on Vaccine Research; 2012; Baltimore.
- Staras SA, Dollard SC, Radford KW, Flanders WD, Pass RF, Cannon MJ. Seroprevalence of cytomegalovirus infection in the United States, 1988–1994. Clin Infect Dis 2006; 43:1143-51; PMID:17029132; http://dx.doi.org/10.1086/508173
- Mocarski E, Shenk T, Pass RF. Cytomegaloviruses. Fields Virology. Philadelphia: Lippincott, Williams & Wilkins; 2007. p. 2701-73. Knipe DM, Howley PM, Griffin DE, Lamb RA, Martin MA, editors.
- Arora N, Novak Z, Fowler KB, Boppana SB, Ross SA. Cytomegalovirus viruria and DNAemia in healthy seropositive Women. J Infect Dis 2010; 202:1800-3; PMID:21050121; http://dx.doi.org/10.1086/657412
- Stowe RP, Kozlova EV, Yetman DL, Walling DM, Goodwin JS, Glaser R. Chronic herpesvirus reactivation occurs in aging. Exp Gerontol 2007; 42:563-70; PMID:17337145; http://dx.doi.org/10.1016/j.exger.2007.01.005
- Brunner S, Herndler-Brandstetter D, Weinberger B, Grubeck-Loebenstein B. Persistent viral infections and immune aging. Ageing Res Rev 2011; 10:362-9; PMID:20727987; http://dx.doi.org/10.1016/j.arr.2010.08.003
- Buchholz VR, Neuenhahn M, Busch DH. CD8+ T cell differentiation in the aging immune system: until the last clone standing. Curr Opin Immunol 2011; 23:549-54; PMID:21664807; http://dx.doi.org/10.1016/j.coi.2011.05.002
- Almeida LN, Azevedo RS, Amaku M, Massad E. Cytomegalovirus seroepidemiology in an urban community of Sao Paulo, Brazil. Rev Saude Publica 2001; 35:124-9; PMID:11359197; http://dx.doi.org/10.1590/S0034-89102001000200004
- United States Census Bureau (http://www.census.gov/#). Accessed 26 July 2013.
- Hens N, Aerts M, Faes C, Shkedy Z, Lejeune O, Van Damme P, Beutels P. Seventy-five years of estimating the force of infection from current status data. Epidemiol Infect 2010; 138:802-12; PMID:19765352; http://dx.doi.org/10.1017/S0950268809990781
- Griffiths PD. Burden of disease associated with human cytomegalovirus and prospects for elimination by universal immunisation. Lancet Infect Dis 2012; 12:790-8; PMID:23017365; http://dx.doi.org/10.1016/S1473-3099(12)70197-4
- Lanzieri T. Modelling CMV transmission & disease. The Development and Evaluation of Human Cytomegalovirus Vaccines Workshop 2012 Oct 1 (http://www.fda.gov/downloads/BiologicsBloodVaccines/NewsEvents/WorkshopsMeetingsConferences/UCM302082pdf 2012). Accessed 29 July 2013.
- GlaxoSmithKline Vaccine HPV-007 Study Group, Romanowski B, de Borba PC, Naud PS, Roteli-Martins CM, De Carvalho NS, Teixeira JC, Aoki F, Ramjattan B, Shier RM, et al. Sustained efficacy and immunogenicity of the human papillomavirus (HPV)-16/18 AS04-adjuvanted vaccine: analysis of a randomised placebo-controlled trial up to 6.4 years. Lancet 2009; 374:1975-85; PMID:19962185; http://dx.doi.org/10.1016/S0140-6736(09)61567-1
- Hekimoğlu M, Barlas M. Sensitivity analysis of system dynamics models by behavior pattern measures The 28th International Conference of the System Dynamics Society; 2010 July 25–29; Seoul, Korea. Conference Proceedings (http://www.systemdynamics.org/conferences/2010/proceed/papers/P1260.pdf). Accessed 27 February 2014.
- Sumner T, Shephard E, Bogle ID. A methodology for global-sensitivity analysis of time-dependent outputs in systems biology modelling. J R Soc Interface 2012; 9:2156-66; PMID:22491976; http://dx.doi.org/10.1098/rsif.2011.0891
- Effelterre TV, Shkedy Z, Aerts M, Molenberghs G, Damme PV, Beutels P. Contact patterns and their implied basic reproductive numbers: an illustration for varicella-zoster virus. Epidemiol Infect 2009; 137:48-57; PMID:18466660; http://dx.doi.org/10.1017/S0950268808000563
- The development and evaluation of human cytomegalovirus vaccines Workshop 2012 Oct 1 (http://www.fda.gov/downloads/BiologicsBloodVaccines/NewsEvents/WorkshopsMeetingsConferences/UCM302082.pdf). Accessed 29 July 2013.
- Adler SP, Marshall B. Cytomegalovirus infections. Pediatr Rev 2007; 28:92-100; PMID:17332168; http://dx.doi.org/10.1542/pir.28-3-92
- Peckham CS, Johnson C, Ades A, Pearl K, Chin KS. Early acquisition of cytomegalovirus infection. Arch Dis Child 1987; 62:780-5; PMID:2821927; http://dx.doi.org/10.1136/adc.62.8.780
- Tu W, Chen S, Sharp M, Dekker C, Manganello AM, Tongson EC, Maecker HT, Holmes TH, Wang Z, Kemble G, et al. Persistent and selective deficiency of CD4+ T cell immunity to cytomegalovirus in immunocompetent young children. J Immunol 2004; 172:3260-7; PMID:14978134; http://dx.doi.org/10.4049/jimmunol.172.5.3260
- Zanghellini F, Boppana SB, Emery VC, Griffiths PD, Pass RF. Asymptomatic primary cytomegalovirus infection: virologic and immunologic features. J Infect Dis 1999; 180:702-7; PMID:10438357; http://dx.doi.org/10.1086/314939
- McVoy MA, Adler SP. Immunologic evidence for frequent age-related cytomegalovirus reactivation in seropositive immunocompetent individuals. J Infect Dis 1989; 160:1-10; PMID:2543705; http://dx.doi.org/10.1093/infdis/160.1.1
- Mossong J, Hens N, Jit M, Beutels P, Auranen K, Mikolajczyk R, Massari M, Salmaso S, Tomba GS, Wallinga J, et al. Social contacts and mixing patterns relevant to the spread of infectious diseases. PLoS Med 2008; 5:e74; PMID:18366252; http://dx.doi.org/10.1371/journal.pmed.0050074
- Goeyvaerts N, Hens N, Ogunjimi B, Aerts M, Shkedy Z, Van Damme P, Beutels P. Estimating infectious disease parameters from data on social contacts and serological status. J R Stat Soc Ser C Appl Stat 2010; 59:255-77; http://dx.doi.org/10.1111/j.1467-9876.2009.00693.x
- Wallinga J, Teunis P, Kretzschmar M. Using data on social contacts to estimate age-specific transmission parameters for respiratory-spread infectious agents. Am J Epidemiol 2006; 164:936-44; PMID:16968863; http://dx.doi.org/10.1093/aje/kwj317
- Iannelli M, Martcheva M, Milner FA. Gender-Structured Population Modelling: Mathematical Methods, Numerics, and Simulation. SIAM Frontiers in Applied Mathematics; 2005.
- Yow MD, White NH, Taber LH, Frank AL, Gruber WC, May RA, Norton HJ. Acquisition of cytomegalovirus infection from birth to 10 years: a longitudinal serologic study. J Pediatr 1987; 110:37-42; PMID:3025394; http://dx.doi.org/10.1016/S0022-3476(87)80284-6
- Ross SA, Arora N, Novak Z, Fowler KB, Britt WJ, Boppana SB. Cytomegalovirus reinfections in healthy seroimmune women. J Infect Dis 2010; 201:386-9; PMID:20039807; http://dx.doi.org/10.1086/649903
- Centers for Disease Control and Prevention (http://www.cdc.gov/nchs/index.htm). Accessed 26 July 2013.