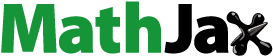
Abstract
Objective
To estimate prevalent ALS cases in the United States for calendar year 2018.
Methods
The National ALS Registry (Registry) compiled data from national administrative databases (from the Centers for Medicare and Medicaid Services, the Veterans Health Administration, and the Veterans Benefits Administration) and enrollment data voluntarily submitted through a web portal (www.cdc.gov/als). We used log-linear capture-recapture (CRC) model-based methodology to estimate the number of cases not ascertained by the Registry.
Results
The Registry identified 21,655 cases of ALS in 2018, with an age-adjusted prevalence of 6.6 per 100,000 U.S. population. When CRC methods were used, an estimated 29,824 cases were identified, for an adjusted prevalence of 9.1 per 100,000 U.S. population. The demographics of cases of ALS did not change from previous year’s reports. ALS continues to impact Whites, males, and persons over 50 years of age more so than other comparison groups. The results from the present report suggest case ascertainment for the Registry has improved, with the estimate of missing prevalent cases decreasing from 44% in 2017 to 27% in in 2018.
Discussion
Consistent with previous estimates that used CRC, ALS prevalence in the United States is about 29,824 cases per year.
Introduction
Amyotrophic lateral sclerosis (ALS) is a progressive and fatal neuromuscular disease (Citation1). While there is currently no cure for ALS, new therapeutics continue to be trialed and approved by the United States (U.S.) Food and Drug Administration (Citation2); however, the identification of risk factors and etiologies for ALS remain elusive. Researchers have identified several environmental exposure variables and occupations that are associated with increased likelihood of ALS (e.g., heavy metal exposure, pesticides, history of military service), although additional validation is needed (Citation3–7). A definitive biomarker or diagnostic test is currently not available; however, recent developments indicate the measurement of neurofilament light chains show promise in determining disease progression (Citation8,Citation9).
The National Amyotrophic Lateral Sclerosis Registry (Registry) is a multi-faceted research platform authorized by the U.S. Congress through the ALS Registry Act, Public Law 110-73 (Citation10). It was created and is managed by the Agency for Toxic Substances and Disease Registry (ATSDR), a sister agency of the Centers for Disease Control and Prevention (CDC). The objectives of the Registry are multi-fold. They include assessing and determining the public health burden of ALS by estimating the incidence, prevalence, and mortality rates, and examining risk factors to identify possible etiologies (Citation11–14). The Registry has published national reports on incidence, mortality, and prevalence for previous calendar years 2010-2017 (Citation15).
Because ALS is not a nationally notifiable disease in the United States in 2022, ATSDR undertook a thorough evaluation to better understand missing cases not captured by the Registry. Specifically, ATSDR sought input from nationally recognized neurologists to evaluate and update the current algorithm to identify ALS cases exclusive of capture-recapture (CRC) methods (Citation16). The algorithm to identify cases was thoroughly evaluated and modified to adjust to the changing ALS disease landscape. This modification led to an increase in the identification of cases by adding a new Registry category called “likely” cases which expanded the inclusion of new and existing therapeutics through 2018 (e.g., Riluzole and Edaravone) by a single prescription only. The previous algorithm only included a single category called “definite” ALS cases. This was changed to “confirmed” and “likely” ALS. This new modification of the algorithm necessitated new CRC analyses. The previous CRC analyses, which used a pair-wise identification of cases in three different administrative databases, was deemed inadequate because it did not take into consideration the new Registry algorithm for case ascertainment (Citation17).
We used the above-mentioned changes to the methodology (a new CRC analyses with the updated Registry algorithm) to determine the prevalence of ALS among adults (ages ≥18 years) in the United Stated for 2018, the most current year of national data available.
Methods
National ALS Registry
The National ALS Registry uses a two-pronged approach to identify prevalent cases of ALS in the United States The first approach identifies confirmed and likely cases reported from three large national administrative databases (Centers of Medicare and Medicaid Services, Veterans Health Administration, and Veterans Benefits Administration) by using an algorithm with elements such as the International Classification of Diseases (ICD) 10th revision code for ALS, frequency of visits to a neurologist, cause of death via national death certificate data, and prescription drug use (Citation18). This approach uses the administrative data to identify persons with ALS based on encounter codes such as having ALS listed as a code in the visit record, an ALS code plus having seen a neurologist, a death certificate listing ALS as a cause or contributing cause of death, or prescription for Riluzole (Citation19). If the patient meets the criteria (e.g., a person aged <65 years with an encounter coded for ALS in Medicare and a neurologist visit), the patient is identified as a “confirmed ALS” case. If the patient only has a prescription for Riluzole from one of the administrative databases, the patient is considered a “likely ALS” case because Riluzole is only prescribed to ALS patients at this time. The Registry categorizes individuals as “confirmed ALS,” “likely ALS,” “undetermined ALS,” and “not ALS.” Only “confirmed ALS” and “likely ALS” cases are entered into the Registry (Citation16).
The second approach for identifying prevalent cases makes use of a secure public web portal that enables persons with ALS to enroll in the Registry, thereby enabling the identification of additional cases not recorded in the national administrative databases. Cases from both sources are then merged and deduplicated on an annual basis. Once an ALS case is identified, the patient remains a case until confirmed deceased through the CDC’s National Death Index. This is referred to as cumulative prevalence of ALS and is calculated by using the deduplicated total number of persons with ALS identified through the two-pronged approach for the numerator. The 2018 U.S. Census population estimate was accessed using the Bridged-Race Population Estimates 1990-2020 (Citation20) and was used as the denominator and to calculate 95% confidence intervals. The original methodology of calculating national prevalence estimates has been previously described in detail (Citation18), which has been adapted to improve case number estimates (Citation11,Citation16,Citation17).
Capture-Recapture
In epidemiology studies, the CRC log-linear modeling framework is widely applied to estimate the number of disease cases based on data collected from multiple overlapping data sources (Citation21). To estimate the number of prevalent ALS cases in the United States in 2018, we applied the CRC log-linear modeling framework to incident ALS cases identified in 2018 by the three national administrative databases (Centers of Medicare and Medicaid Services, Veterans Health Administration, and Veterans Benefits Administration) together with the voluntary registry portal. After estimating the number of incident cases using the log-linear model, the estimated prevalent case total was obtained by summing the observed number of surviving prevalent cases identified in previous years together with the estimated incident case number. When applying the log-linear model, only incident cases were considered to avoid the challenging and error-prone task of assigning 2018 capture histories to prevalent cases that were identified previously.
In the log-linear model paradigm, different dependency structures among the three data sources were explored by including pairwise interaction terms involving capture indicators denoted as and
for Medicare, Veterans Affairs, and voluntary registry portal, respectively. These binary variables,
take values 0 or 1, 1 indicates captured by the
-th data source and 0 indicates not captured. Here we followed a standard convention when applying CRC log-linear models. The models considered in the analysis included all three indicators for the data sources while excluding the three-way interaction term to avoid non-identifiability problems. We then continued to follow standard practice by using Akaike’s information criterion (AIC) to select from the resulting list of candidate models (Citation22). Estimated prevalent case counts were obtained from models with the lowest AIC, and the delta method was used for computing 95% confidence intervals (Citation23).
To obtain estimates within separate categories of sex (male and female), patient age (18-50 years, 51-65 years, and 66 years), and race (e.g., Whites, Blacks, Other), stratified analyses were effectively conducted by incorporating additional binary indicators for those demographic variables into the model paradigm. Specifically, we again applied the log-linear model to incident cases and used the AIC for model selection, where in these analyses candidate models must include 1) all three indicators for the different data sources, 2) one or more indicators to index the levels of the categorical covariate, and 3) two-way interaction terms between indicators for data sources and indicators for the covariate. Again, we excluded the three-way interaction term of indicators for data sources within any level of the categorical covariate.
To address missing covariate data in the stratified CRC analyses, 884 incident cases with unknown race that were identified by the portal but not by the Medicare or Veterans Affairs sources were redistributed into the three race groups for analysis (White, Black, Other) based on the race proportions among incident cases with recorded race that were captured by the portal. Similarly, incident cases for whom age was missing (240 cases) were redistributed into the three age groups for analysis based on the observed proportion in each age group among incident cases based on identification (yes/no) by the Medicare source. Thus, stratified analyses for race and age were performed using adjusted data, while sex-stratified analysis was based on the original data.
We also report estimated under-ascertainment data, which were calculated as the proportion of estimated unobserved ALS cases among estimated total prevalent cases in the overall and stratified analyses. All analyses were conducted using R 4.2.3 (Citation24).
Results
For 2018, the National ALS Registry found 21,665 persons meeting the case definition (i.e., having “confirmed” or “likely” ALS) for a prevalence of 6.6 per 100,000 persons. These cases were found by applying the updated algorithm to “undetermined” cases identified by the administrative databases and the web portal (). Persons aged 18-50 years had the lowest estimated prevalence (1.1 confirmed or likely cases per 100,000 population) and persons older than 65 years had the highest (21.4 per 100,000 population). The prevalence of ALS among males (8.1 cases per 100,000 population) was higher than among females (5.2 per 100,000 population). The prevalence among Whites (6.1 per 100,000 population) was twice that among Blacks (2.8 per 100,000 population) and prevalence rate of those reporting race other than white or black was observed at 4.5 per 100,000 population ().
Table 1. Number and percentage of amyotrophic lateral sclerosis (ALS) cases (N = 21,665) and estimated prevalence, by age group, sex, and race—National ALS Registry, United States, 2018.
In 2018, to account for possible missing cases, we used the estimate of percentage missing (27.4%) calculated from the current log-linear CRC analysis. Using the 2018 registry data, the observed number of cases (n = 21,665) comprised only 72.6% of the estimated total of confirmed and likely cases, producing an adjusted total case count of 29,824 and the estimated number of missing cases of 8,159. We applied the sex-specific estimates of percentage missing from the updated CRC analysis and estimated that 3,730 cases (22.2%) were missing among those reported for males and 5,952 cases (41.0%) were missing for females (). The adjusted prevalence was 9.1 per 100,000 population (). Males had a higher prevalence rate (10.5 per 100,000) than did females (8.7 per 100,000) (). The percentage of missing cases for Blacks was like that of Whites, 31.2% vs. 31.4%, with missing cases for Other races slightly greater at 34.1% (). The prevalence for the White population was twice as high (8.8 per 100,000) as that of the Black population (4.1 per 100,000) with the adjusted rate for Other race estimated in-between at 6.8 per 100,000 population ().
Discussion
These results suggest that case ascertainment for the National ALS Registry has improved and can likely be attributed to the Registry’s revised algorithm. For this analysis, we also applied a new CRC approach. Although two different CRC methods (pair-wise versus three-source log-linear modeling) were used to determine the estimated missing cases, the current log-linear approach has resulted in a reduction in the estimated number of missing cases. Specifically, the estimate of missing cases has been reduced from 14,043 (44.1%) in 2017 to 8,159 (27.4%) in 2018 or a reduction of 5,884 cases (16.7%). We did not conduct CRC pair-wise analyses for the 2018 dataset, so it is not known how use of this method would reduce (if at all) the estimated overall case ascertainment. Previously, the Registry estimated 31,843 cases for 2017 by CRC methodology. The new analyses show a slight reduction to 29,824 cases. The reduction in the estimated number of missing cases did not lead to a meaningful change of the overall estimated number of prevalent ALS cases in the United States which is still around 30,000 patients.
The overall prevalence rate of ALS in the United States for 2018 was estimated to be 9.1 per 100,000 persons. This was slightly reduced from the 2017 rate of 9.9 per 100,000 persons (Citation11). The reduction was also reflected in male population, but not for females, when 2018 data were compared with 2017 data. The differences seen here are likely the result of combined improvement of case definition, which is more inclusive of ALS cases in general, and the new log model CRC approach. Interestingly, the reported missing % for female population was higher at 41.0% (vs. 22.2% for males). For capture-recapture estimation, we used the log-linear model approach with a binary indicator of sex included to estimate the number of ALS cases among females and males. Based on results from the log-linear model selected by the AIC metric, we found that the proportion of ALS cases identified by the web portal among cases not identified by Medicare and Veterans Affairs was estimated to be 0.12 for males and 0.06 for females. In other words, conditioning on non-identification by Medicare and Veterans Affairs, the modeling suggests that male cases were more likely captured by the web portal compared to female cases. This difference might explain the higher missingness percentage observed for females. Specifically, the estimated number of missing female cases was about 1.6 times the estimated number of missing male cases (female: 5952 vs. male: 3730), while the number of observed female cases was only about 0.65 times the number of observed male cases. But we also speculate other factors are at play such as historical pervasiveness of underdiagnosis, misdiagnosis, and delay in proper diagnosis in women resulting in gender-based disparity in medicine and patientcare (Citation25,Citation26). We hope that in our future reporting, utilization of methods such as used here to better estimate missing cases and increase in the awareness of National ALS Registry and enrollment will improve the gap.
The log-linear modeling paradigm, including the AIC-based model selection approach and other conventions applied in our analysis, is commonly used for CRC-based estimation. This includes past analyses conducted within the National ALS Registry (Citation17). Nevertheless, we acknowledge potential shortcomings and uncertainties associated with this approach such as this method is more likely to produce a biased estimate of the population size (Citation27,Citation28). We also note that the summed estimates of prevalent cases obtained from stratified analyses are different from the estimate obtained from the overall analysis, as is to be expected under the log-linear modeling paradigm. However, point estimates are within the same range, and there are overlaps between 95% confidence intervals.
The prevalence of ALS in the U.S. remains unchanged for persons with ALS and their caregivers. That will not change in the foreseeable future unless tangible therapeutics are approved and readily available that will either slow, halt, or reverse disease progression.
The National ALS Registry continues to make the necessary adjustments and changes as the ALS landscape evolves; however, challenges remain. For example, some patients continue to receive care from private insurance companies and might not elect to convert to either Medicare or Veterans Health Administration or Veterans Benefits Administration providers. The estimated 27% missing cases most likely reflect those patients who are uninsured or covered by their current private insurance carriers.
To further improve case ascertainment, the Registry has submitted and received approval for an amendment to the federal Office of Management and Budget to include new data sources such as those from patient-centric care-giver organizations (e.g., ALS Association), state-based registries, and private insurance carriers. Approval of the amendment would allow prospective cases to be added to the Registry and cross-referenced with the national administrative databases to prevent duplicative counts.
Conclusions
The National ALS Registry is an important tool to facilitate research domestically and internationally, and for determining ALS epidemiology in the United States. Comparisons of findings from 2017 to 2018 show improved case ascertainment, but more importantly suggest a current stabilization of ALS prevalent cases at around 30,000 per year. As new therapeutics are approved or prevention measures are identified and implemented, the prevalence of ALS will invariably change. The Registry remains steadfast in adjusting, adapting, and improving methods to evaluate and describe the ALS journey in the United States.
Disclaimer
The findings and conclusions in this report are those of the author(s) and do not necessarily represent the official position of the Centers for Disease Control and Prevention/Agency for Toxic Substances and Disease Registry.
Declaration of interest
The authors report no conflicts of interest. The authors alone are responsible for the content and writing of this article.
References
- Hardiman O, Al-Chalabi A, Chio A, Corr EM, Logroscino G, Robberecht W, et al. Amyotrophic lateral sclerosis. Nat Rev Dis Primers. 2017;3:17071.
- FDA Approves New Treatment Option for Patients with ALS, 2022. Available at: https://www.fda.gov/news-events/press-announcements/fda-approves-new-treatment-option-patients-als
- Andrew AS, Bradley WG, Peipert D, Butt T, Amoako K, Pioro EP, et al. Risk factors for amyotrophic lateral sclerosis: a regional United States case-control study. Muscle Nerve. 2021;63:52–9.
- Su FC, Goutman SA, Chernyak S, Mukherjee B, Callaghan BC, Batterman S, et al. Association of environmental toxins with amyotrophic lateral sclerosis. JAMA Neurol. 2016;73:803–11.
- Yu Y, Su FC, Callaghan BC, Goutman SA, Batterman SA, Feldman EL. Environmental risk factors and amyotrophic lateral sclerosis (ALS): a case-control study of ALS in Michigan. PLoS One. 2014;9:e101186.
- Goutman SA, Boss J, Godwin C, Mukherjee B, Feldman EL, Batterman SA. Associations of self-reported occupational exposures and settings to ALS: a case-control study. Int Arch Occup Environ Health. 2022;95:1567–86.
- Cox PA, Kostrzewa RM, Guillemin GJ. BMAA and neurodegenerative illness. Neurotox Res. 2018;33:178–83.
- Dunlop RA, Banack SA, Cox PA. L1CAM immunocapture generates a unique extracellular vesicle population with a reproducible miRNA fingerprint. RNA Biol. 2023;20:140–8.
- Meyer T. [Amyotrophic lateral sclerosis (ALS) – diagnosis, course of disease and treatment options]. Dtsch Med Wochenschr 2021;146:1613–8.
- US Public Health Service. ALS registry act. Washington, DC: 110th Congress; 2008.
- Mehta P, Raymond J, Punjani R, Han M, Larson T, Kaye W, et al. Prevalence of amyotrophic lateral sclerosis in the United States using established and novel methodologies, 2017. Amyotroph Lateral Scler Frontotemporal Degener. 2023;24:108–16.
- Mehta P, Raymond J, Punjani R, Larson T, Han M, Bove F, et al. Incidence of amyotrophic lateral sclerosis in the United States, 2014-2016. Amyotroph Lateral Scler Frontotemporal Degener. 2022;23:378–82.
- Raymond J, Mehta P, Larson T, Factor-Litvak P, Davis B, Horton K. History of vigorous leisure-time physical activity and early onset amyotrophic lateral sclerosis (ALS), data from the national ALS registry: 2010-2018. Amyotroph Lateral Scler Frontotemporal Degener. 2021;22:535–44.
- Rechtman L, Brenner S, Wright M, Ritsick M, Rahman F, Han M, et al. Impact of the national amyotrophic lateral sclerosis registry: analysis of registry-funded research. Ann Clin Transl Neurol. 2022;9:1692–701.
- Centers for Disease Control and Prevention, National ALS Registry Disease Estimates, 2022. Available at: https://www.cdc.gov/als/National_disease_estimates.html
- Mehta P, Raymond J, Han M, Punjani R, Larson T, Berry JD, et al. A revision to the United States national ALS registry’s algorithm to improve Case-Ascertainment. Amyotroph Lateral Scler Frontotemporal Degener 2023; 24:230–6.
- Nelson LM, Topol B, Kaye W, Raymond J, Horton DK, Mehta P, et al. Evaluation of the completeness of ALS Case Ascertainment in the US National ALS Registry: application of the capture-recapture method. Neuroepidemiology 2022;56:104–14.
- Kaye WE, Sanchez M, Wu J. Feasibility of creating a National ALS Registry using administrative data in the United States. Amyotroph Lateral Scler Frontotemporal Degener. 2014;15:433–9.
- Mehta P, Raymond J, Punjani R, Larson T, Bove F, Kaye W, et al. Prevalence of amyotrophic lateral sclerosis (ALS), United States, 2016. Amyotroph Lateral Scler Frontotemporal Degener. 2022;23:220–5.
- United States Department of Health and Human Services, Centers for Disease Control and Prevention, National Center for Health Statistics. Bridged-race population estimates, United States July 1st resident population by state, county, age, sex, bridged-race, and Hispanic origin.: CDC WONDER Online Database. Available at: http://wonder.cdc.gov/bridged-race-v2020.htm
- Chao A, Tsay P, Lin SH, Shau WY, Chao DY. The applications of capture‐recapture models to epidemiological data. Stat Med. 2001;20:3123–57.
- Akaike H. A new look at the statistical model identification. IEEE Trans Automat Contr. 1974;19:716–23.
- Agresti A. Categorical data analysis. New Jersey: John Wiley & Sons; 2012.
- R Core Team. R: a language and environment for statistical computing. Vienna, Austria: R Foundation for Statistical Computing; 2023.
- Fletcher K, Prigerson H, Paulk E, Temel J, Finlay E, Marr L, et al. Gender differences in the evolution of illness understanding among patients with advanced cancer. J Support Oncol. 2013;11:126–32.
- Vogel B, Acevedo M, Appelman Y, Bairey Merz CN, Chieffo A, Figtree GA, et al. The Lancet women and cardiovascular disease Commission: reducing the global burden by 2030. The Lancet. 2021;397:2385–438.
- Coull BA, Agresti A. The use of mixed logit models to reflect heterogeneity in capture‐recapture studies. Biometrics 1999;55:294–301.
- Jones HE, Hickman M, Welton NJ, De Angelis D, Harris RJ, Ades A. Recapture or precapture? Fallibility of standard capture-recapture methods in the presence of referrals between sources. Am J Epidemiol. 2014;179:1383–93.