Abstract
Despite many recent studies on non-alcoholic fatty liver disease (NAFLD) therapeutics, the optimal treatment has yet to be determined. In this unfinished project, we combined secondary metabolites (SMs) from the gut microbiota (GM) and Hordeum vulgare (HV) to investigate their combinatorial effects via network pharmacology (NP). Additionally, we analyzed GM or barley – signalling pathways – targets – metabolites (GBSTMs) in combinatorial perspectives (HV, and GM). A total of 31 key targets were analysed via a protein-protein interaction (PPI) network, and JUN was identified as the uppermost target in NAFLD. On a bubble plot, we revealed that apelin signalling pathway, which had the lowest enrichment factor antagonize NAFLD. Holistically, we scrutinized GBSTM to identify key components (GM, signalling pathways, targets, and metabolites) associated with the Apelin signalling pathway. Consequently, we found that the primary GMs (Eubacterium limosum, Eggerthella sp. SDG-2, Alistipes indistinctus YIT 12060, Odoribacter laneus YIT 12061, Paraprevotella clara YIT 11840, Paraprevotella xylaniphila YIT 11841) to ameliorate NAFLD. The molecular docking test (MDT) suggested that tryptanthrin-JUN is an agonist, conversely, dihydroglycitein-HDAC5, 1,3-diphenylpropan-2-ol-NOS1, and (10[(Acetyloxy)methyl]-9-anthryl)methyl acetate-NOS2, which are antagonistic conformers in the apelin signalling pathway. Overall, these results suggest that combination therapy could be an effective strategy for treating NAFLD.
Introduction
Non-alcoholic fatty liver disease (NAFLD) is the most persistent hepatic disease that encompasses a wide range of diseases, including steatosis, non-alcoholic steatohepatitis (NASH), fibrosis, cirrhosis, and liver failure, such as hepatocellular carcinoma [Citation1]. NAFLD, a chronic liver disease, is caused by excessive fat accumulation, which is manifested by metabolic imbalance related to obesity, diabetes mellitus, and insulin resistance, further triggering extreme cardiovascular diseases (CVDs) [Citation2]. The evidence demonstrating connectivity between the gut-liver axis and NAFLD pathogenesis has been revealed in the context of therapeutics [Citation3]. The key players in the digestion of carbohydrates are gut bacteria, which produce short-chain fatty acid (SCFA) metabolites (acetate, butyrate, and propionate) [Citation4]. The short-chain fatty acids (SCFAs) might play central roles in enhancing glucose and fat metabolism and driving intestinal homeostasis, and increased SCFAs might alleviate obesity and liver steatosis and facilitate nutrient utilizations in the body [Citation5]. Hordeum vulgare (common name; barely) was domesticated approximately 10,000 years ago and is a healthy food used to treat metabolic disorders and prevent colon cancer [Citation6,Citation7]. Notably, an animal test demonstrated that feeding mice barley (Hordeum vulgare; HV) combined with β-glucan (BG) for 10 weeks diminished the severity of liver fibrosis and decreased the expression level of transforming growth factor beta (TGFβ) [Citation8]. Another report showed that the consumption of rich-BG can hamper fat absorption, leading to the amelioration of NAFLD [Citation9]. However, a clinical study on the effect of BG polysaccharide in 10 healthy male groups reported that BG was not detected in blood samples after taking 1 g every one time for 1 week [Citation10], indicating low bioavailability.
In parallel, BG, which is highly hydrophilic, can lead to diverse unexpected side effects, such as diarrhoea, nausea, vomiting, dizziness, and joint pain [Citation11]. Therefore, we selected only lipophilic compounds with drug-like properties from HVs based on Lipinski’s rule. Additionally, it has been reported that the antiobesity efficacy of ginseng is dependent upon the diversity of the gut microbiota (GM) [Citation12]. Body weight (BW) and body mass index (BMI) are controlled by the distribution of Subdoligranulum, Oscillibacter, and Akkermansia in the gut, whereas the body fat percentage is determined by Lactobacillus [Citation12]. The composition of the GM is a key factor in managing metabolism in the body, and extrinsic elements such as diet also determine the diversity of gut microbes, including those involved in NAFLD. Similarly, we investigated the synergistic effects of secondary metabolites (SMs) from both the GM and HV on NAFLD.
With the assistance of network pharmacology (NP), we took a holistic approach on SMs from the GM and HV to determine their effects on NAFLD. Distinctively, NP analysis is a significant structural method for revealing interconnected biological data via complex microbiome analysis [Citation13,Citation14]. The merged analysis of Chinese herbal medicine and harmful GM was conducted to examine the key components and mechanisms involved in treating ulcerative colitis (UC) via the NP [Citation15].
The collective analytical approach, including nutrigenomics, metabolomics, and microbiota data, might be a keynote to uncovering the coaction between SMs, the GM, and host responses in determining hepatocellular function and healing [Citation16]. Such studies are necessary to determine the therapeutic potential of SMs from the GM and natural product resources. As suggested, we described the therapeutic value of integrated information on the GM and HV via the NP concept. Significantly, this approach will provide insight into the mechanism of action and therapeutic benefits of exogenous species (herbal medicines, plants or food) and endogenous species (SMs from the GM) with orchestrated systems. Accordingly, we established a GM or barley – signalling pathway – target – metabolite (GBSTM) network to confirm their relationships in the treatment of NAFLD. Thus, our study showed that the combined application of SMs from the GM and HV might have favourable synergistic effects, suggesting that dual use might be an alleviative strategy for the treatment of NAFLD. The stepwise process is shown in .
Although there are few microbiome data on the critical mechanistic underpinnings of NAFLD treatment, our suggestion provides significant insight into the key components that are important for distinguishing between SMs from the GM and HVs, despite limited resources.
Materials and methods
The utilization of public bioinformatics databases
Specifically, with the employment of biologically significant databases, we analyzed this study to identify pharmacological relationships via the combination of GM and HV. Web-accessible platforms are available for individuals who attempt to obtain beneficial data; furthermore, we applied these platforms to reveal key components (GM, signalling pathways, targets, and secondary metabolites) from combinatorial utilization. The list of the databases is displayed in . The SMs from HV were retrieved from the Natural Product Activity & Species Source (NPASS) repository (http://bidd.group/NPASS/) (retrieved on 5 August 2022) [Citation17], suggesting that significant targets associated with the bioactives were identified via the similarity ensemble approach (SEA) (https://sea.bkslab.org/) (retrieved on 5 August 2022) [Citation18] and SwissTargetPrediction (STP) (http://www.swisstargetprediction.ch/) (retrieved on 5 August 2022) [Citation19]. The SMs from the GM were obtained from gutMGene (http://bio-computing.hrbmu.edu.cn/gutmgene/) (retrieved on 6 August 2022). Similarly, we performed a meta-analysis to search for targets related to SMs through SEA and STP. NAFLD targets were selected by DisGeNET (https://www.disgenet.org/) (retrieved on 7 August 2022) [Citation20] and OMIM (https://www.omim.org/) (accessed on 7 August 2022) [Citation21]. Overall, we identified crucial protein targets for the treatment of NAFLD via the STRING platform (https://string-db.org/) (accessed on 8 August 2022) [Citation22]. We constructed a protein–protein interaction (PPI) network, Gut microbiota – Barley – Signalling pathways – Targets – Metabolites (GBSTM) network using the R package, and a molecular docking test (MDT) via AutoDockTools-1.5.6 software [Citation23].
Table 1. The Index of available databases for the study.
The selection of SMs from HVs
We identified SMs of HVs from NPASS by browsing the target proteins associated with SMs via SEA and STP. In parallel, we found common target proteins between SEA and STP. The intersecting targets were allowed to contain significant elements due to piling on the two significant databases (SEA and STP). Importantly, the SEA dataset repository was developed by Dr. Shoichet to verify the binding level of the ligand–protein complex and ultimately determine its affinity [Citation18]. Specifically, 23 out of the 30 protein targets predicted by SEA were confirmed via experimentation [Citation24]. Evidently, STP was employed as a key to decrypt targets for cudraflavone C, the potential targets of the compound hit via experimentation [Citation25].
The attainment of SMs from the GM
The gutMGene database was used for microbial informatics to obtain SMs of the GM, indicating that the protein targets linked to SMs were identified through SEA and STP. Accordingly, we employed the intersecting protein targets identified in the two repositories to improve the success rate of this study.
The mining of crux targets against NAFLD from HV and GM
We selected the overlapping targets related to both HV and GM targets and then identified crux targets by comparison with NAFLD-associated protein targets obtained through the DisGeNET and OMIM repositories. The identified targets are critical protein-coding genes affected by SMs from HVs and the GM for the treatment of NAFLD.
PPI network construction
The crux targets were loaded in the STRING platform to construct the PPI network, and the R package was used to construct the PPI network in detail. Overall, we identified a hub target for alleviating NAFLD via PPI analysis. The hub target was defined as the target with “the highest degree value”, meaning that it can have the greatest effect on other targets.
Bubble diagram construction
Bubble diagram shows the results of Kyoto Encyclopaedia of Genes and Genomes (KEGG) pathway [Citation26] enrichment analysis. Through KEGG pathway enrichment analysis, we identified a key signalling pathway involved in NAFLD, indicating that this signalling pathway might antagonise NAFLD. The R package was used to construct the bubble diagram.
The GBSTM network construction
We constructed a GBSTM network to uncover the relationships between the GM, barley, signalling pathway, target, and metabolite via the R package. The diameter of each circle indicates the number of links among each component. The significance of the GM, barley, signalling pathway, target, or metabolite stood for the edge level of GM, barley, signalling pathway, target, or metabolite. The indicated GM are defined as converters that enable SMs to be produced in the gut.
MDT test to identify key SMs
We performed MDT using AutoDock 1.5.6 [Citation27] to select the most stable SMs in both the HV and GM on a key signalling pathway. The cut-off value to screen the SMs was −6.0 kcal/mol [Citation28], or SM with the lowest binding energy (the highest negative value) was considered the crucial SM for exerting favourable effects on NAFLD. The SMs were extracted in the .sdf form from PubChem and converted into the .pdb form using PyMOL. The .pdb form was transformed into an .pdbqt file to construct the MDT through AutoDockTools-1.5.6 software. The PDB IDs of the targets were confirmed via the RCSB PDB (https://www.rcsb.org/) (accessed on 08 August 2022). The MDT was implemented to corroborate the affinity of each complex on the .pdbqt files of SMs and targets. The docking binding site was constructed with cubiform (x = 40 Å, y = 40 Å, and z = 40 Å) on the centre of each target: HDAC5 (PDB ID: 5UWI) (x= −8.577, y = 42.932, z = 30.245), NOS1 (PDB ID: 4D1N) (x = 218.219, y = 22.957, z = 42.162), and NOS2 (PDB ID: 4NOS) (x = 17.077, y = 63.563, z = 21.453). The hydrophilic and hydrophobic interactions were identified by LigPlot + 2.2 software (https://www.ebi.ac.uk/thornton-srv/software/LigPlus/download2.html) (retrieved on 09 August 2022) [Citation29]. Overall, MDT was used to search for the most stable SMs among the key targets.
Validation of the drug-like properties and cytotoxicity of the key SMs
We utilized the SwissADME (http://www.swissadme.ch/) (retrieved on 8 August 2022) platform to determine the drug-like properties of the key SMs [Citation30]. The following criteria were used: molecular weight (<500 g/mol), topological polar surface area (TPSA) (<140 Å2), Moriguchi octanol–water partition coefficient (MLogP) (≤4.15), hydrogen bonding acceptor (HBA) (<10) or hydrogen bonding donor (HBD) (≤5). Additionally, to develop a therapeutic agent, significant factors are needed to confirm its nontoxicity. Thus, we established the toxicity parameters via ADMETlab 2.0 [Citation33] and admetSAR 1.0 [Citation34] as follows: human ether-a-go-go-related gene (hERG) [Citation31]; human hepatotoxicity (H-HT) [Citation32]; carcinogens [Citation33]; lethal dose for 50% kill (LD50) [Citation34]; rat oral acute toxicity [Citation35]; and eye corrosion [Citation36].
Results
The SMs of HVs and their screening
A total of 24 SMs from HV were reported in the NPASS repository, and their corresponding targets were obtained from the SEA (574) and STP (514) archives (; Supplementary Table S1). CHEMBL566278 (PubChem ID: 5281113) and Ins (1,2,5,6)P4 (PubChem ID: 14033625) were excluded from the 24 SMs retrieved because of violations of Lipinski’s rule. A total of 79 intersecting targets were identified via SEA and STP, which were ultimately accepted as valid targets associated with HV ().
Figure 2. (a) The 79 overlapping targets associated with SMs from HV between SEA and STP. (b) The 668 overlapping targets related to SMs from GM between SEA and STP. (c) The number of 66 intersecting targets associated with SMs between 79 targets from (A) and 668 targets from (B). (d) The identification of 31 crux targets between 66 targets from (C) and NAFLD (1836 targets).
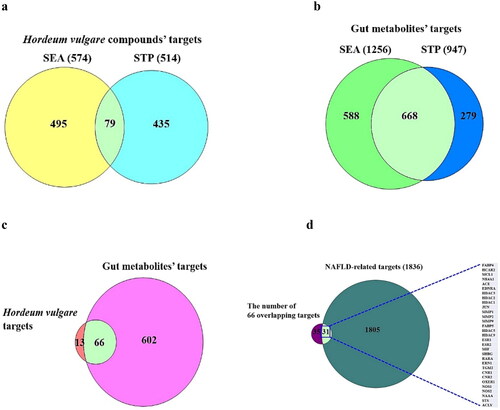
Table 2. The number 24 secondary metabolites of HV from NPASS database.
The SMs of the GM and confirmation of the crux targets for treating NAFLD
A total of 208 SMs (Supplementary Table S1) were identified from the gutMGene database, and we identified 668 overlapping targets between the SEA (1256) and STP (947) repositories (Supplementary Table S1) (). A total of 66 intersecting targets were identified by comparison with the overlapping targets shown in ,b), suggesting that the 66 overlapping targets are considered critical targets that inherently affect SMs from GM and SMs from HV (Supplementary Table S1; ). The 66 overlapping targets identified by the comparison analysis were compared with NAFLD targets; therefore, 31 crux targets were classified in the middle of the 66 overlapping targets and NAFLD targets (1836) (Supplementary Table S1; ).
PPI network analysis
To determine the detailed interaction between each target of HV and GM in NAFLD, the final 31 key proteins of SMs and NAFLD were loaded into the STRING platform to construct the PPI network. The information extracted from the STRING database was entered into the R package to determine the hub targets. The network analysis suggested that each target was associated with its neighbouring target(s) by establishing 30 nodes linked to 74 edges (). Among the 31 crux targets, OXER1 was not associated with one another. A newly constructed network via the R package indicated that Jun proto-oncogene (JUN) was the most prominent protein-coding gene in the entire PPI network, with the highest degree value (16). The classified ranking of each target in the PPI network is shown in Supplementary Table S2.
Figure 3. (a) PPI networks (30 nodes and 74 edges). (b) A heatmap of binding energy of SMs on JUN (PDB ID: 1FOS). (c) The molecular conformation on tryptanthrin (PubChem ID: 73549) – JUN (PDB ID: 1FOS) complex. (d) Bubble diagram of 6 signalling pathways associated with occurrence and progression of NAFLD.
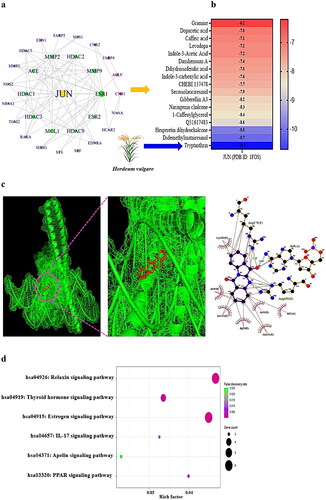
MDT test on the JUN target and bubble diagram
On MDT, we confirmed the most significant SMs on the JUN protein. Tryptanthrin derived from HV formed the most stable complex with the JUN protein, with a binding energy of −10.7 kcal/mol (); Supplementary Table S3). It can thus be concluded that HV has positive effects on alleviating NAFLD. Next, the bubble diagram suggested that the 16 overlapping targets were related directly to 6 signalling pathways ().
The most critical signalling pathway was the apelin signalling pathway, which had the lowest enrichment factor, indicating that the signalling pathway dampens to ameliorate NAFLD via SMs from HVs and the GM (Supplementary Table S4).
GBSTM network investigation
The GBSTM networks demonstrated that 45 GM, HV, a key signalling pathway, 3 targets, and 33 metabolites (83 nodes and 93 edges) were correlated with the apelin signalling pathway (). In the GBSTM network, green circles (nodes) represent the GM, red circles (nodes) represent the Apelin signalling pathway, orange circles (nodes) indicate targets, and sky-blue circles (nodes) indicate SMs. The gray line (edge) represents the relationship of each node.
MDT on key targets, and molecules
On MDT, we confirmed the most significant SMs on each target. In detail, dihydroglycitein (−7.8 kcal/mol) converted by Eubacterium limosum [Citation37] and bound stably to HDAC5 (PDB ID: 5UWI) had hydrogen bond interactions with Ser94 and Ser950 and hydrophobic interconnections with Thr97, Gly19, Asp18, Asn100, Glu70, Gly68, Lys949, and Val96, resulting in the HDAC5-dihydroglycitein complex (). Additionally, 1,3-diphenylpropan-2-ol (known as DA-05096) (−7.7 kcal/mol) converted by Eggerthella sp. SDG-2 [Citation38] had the greatest affinity for NOS1 (PDB ID: 4D1N), and the complex provided hydrogen bond interactions between Arg419 and hydrophobic interactions with Leu705, Arg704, Ser708, Phe709, Glu710, Met575, Tyr711, Ser418, Asn416, and Ala417 (). Then, (10[(Acetyloxy)methyl]-9-anthryl)methyl acetate (−7.9 kcal/mol) converted by Alistipes indistinctus YIT 12060 [Citation39], Odoribacter laneus YIT 12061 [Citation39], Paraprevotella clara YIT 11840 [Citation40], and Paraprevotella xylaniphila YIT 11841 [Citation40] had the greatest affinity on NOS2 (PDB ID: 4NOS), containing two hydrogen bonds (Gln149, and Gln192) and six hydrophobic interactions (Ser486, Glu145, Phe146, Asn148, Leu485, and Arg454) (). Additionally, its binding energy and interactive residue information are shown in Supplementary Table S5.
Figure 5. (a) The molecular conformation on dihydroglycitein (PubChem ID: 101101166) – HDAC5 (PDB ID: 5UWI). (b) The molecular conformation on 1,3-diphenylpropan-2-ol (PubChem ID: 138478) – NOS1 (PDB ID: 4D1N). (c) The molecular conformation on (10[(acetyloxy)methyl]-9-anthryl)methyl acetate (PubChem ID: 613145) -NOS2 (PDB ID: 4NOS).
![Figure 5. (a) The molecular conformation on dihydroglycitein (PubChem ID: 101101166) – HDAC5 (PDB ID: 5UWI). (b) The molecular conformation on 1,3-diphenylpropan-2-ol (PubChem ID: 138478) – NOS1 (PDB ID: 4D1N). (c) The molecular conformation on (10[(acetyloxy)methyl]-9-anthryl)methyl acetate (PubChem ID: 613145) -NOS2 (PDB ID: 4NOS).](/cms/asset/6fc39d92-703b-4465-a15f-3009b641dfb0/ianb_a_2347380_f0005_c.jpg)
Drug-like properties and cytotoxicity screening of the four crux SMs
Finally, four SMs (tryptanthrin, dihydroglycitein, 1,3-diphenylpropan-2-ol, and (10[(Acetyloxy)methyl]-9-anthryl)methyl acetate) were confirmed, and their drug-likeness properties and toxicity levels were established via in silico verification (Supplementary Table S6). However, SMs have no biophysical impediments for their development as new agents. The chemical structures of the four key SMs are displayed in .
The identification of key components against NAFLD
As described above, the complexes of the four key SMs and their targets were transduced to four signalling pathways—the relaxin signalling pathway, oestrogen signalling pathway, and IL-17 signalling pathway—as agonistic mechanisms by forming the Tryptanthrin-JUN complex; conversely, the apelin signalling pathway was shown to act as an antagonistic mechanism by exerting strong affinity on dihydroglycitein-HDAC5, 1,3-diphenylpropan-2-ol-NOS1, and (10[(Acetyloxy)methyl]-9-anthryl)methyl acetate -NOS2 complexes.
Discussion
The PPI networks showed that JUN is the most significant protein-coding gene that regulates adjacent targets for the treatment of NAFLD. Previous studies have demonstrated that JUN is a crucial target against acute stress, such as hepatocyte injury, and is a key element in regulating lipid metabolism in hepatocytes [Citation41,Citation42]. Furthermore, JUN promotes severe biological damage, including oxidative attack and DNA destruction [Citation43]. Tryptanthrin is a natural alkaloid with potent anti-inflammatory effects; likewise, jerantinine B is an alkaloid that induces the activation of c-JUN (known as JUN) for the treatment of acute myeloid leukaemia (AML) [Citation44,Citation45]. This finding suggested that Tryptanthrin might diminish acute stress in hepatocytes by activating JUN to mitigate NAFLD.
The correlations of six signalling pathways with NAFLD are briefly considered below. (1) Relaxin signalling pathway: Relaxin is a notable therapeutic target for diminishing hepatic steatosis by activating endothelial nitric oxide synthase (eNOS) in high-fat diet (HFD)-fed mice [Citation46]. (2) Oestrogen signalling pathway: A lack of oestrogen accelerates the progression of hepatic steatosis, particularly in postmenopausal women with metabolic disorders [Citation47]. (3) Interleukin-17 (IL-17) signalling pathway: IL-17 can enhance the intestinal epithelial layer and diminish dysbacteriosis-induced intestinal damage and other organ injuries induced by diet [Citation48]. Notably, the relaxin signalling pathway, oestrogen signalling pathway, and IL-17 signalling pathway are directly associated with the anti-NAFLD effects of JUN, indicating that these three signalling pathways might exert therapeutic effects on NAFLD. The results are consistent with our suggestions. This means that the Relaxin signalling pathway, which is among the top-ranked enrichment factors, can be strongly activated among the six signalling pathways according to the bubble diagram. Our study revealed that Tryptanthrin from HVs is a promising SM for use as a therapeutic agent against NAFLD by activating the Relaxin signalling pathway, which is associated with a hub target (JUN). (4) Thyroid hormone signalling pathway: Thyroid hormone (TH) interacts strongly between the liver and thyroid for lipid metabolism [Citation49]. (5) PPAR signalling pathway: A study reported that dual agonist peroxisome proliferator-activated receptor (PPAR) might have a positive effect on NAFLD; however, its therapeutic efficacy has not been confirmed in clinical trials [Citation50]. The optimal agents for the PPAR signalling pathway remain unclear. (6) Apelin signalling pathway: A study suggested that the activation of the Apelin signalling pathway protects hepatocytes from lipid accumulation [Citation51]. Conversely, another study demonstrated that apelin aggravates hepatic fibrosis, accelerating metabolic disorders and even homeostasis imbalance [Citation52].
Coincidentally, our study revealed that inactivation of the Apelin signalling pathway is a crucial pathway in NAFLD. Specifically, the effectors that we identified are dihydroglycitein from Eubacterium limosum, 1,3-diphenylpropan-2-ol from Eggerthella sp. SDG-2, and (10[(Acetyloxy)methyl]-9-anthryl)methyl acetate from Alistipes indistinctus YIT 12060, Odoribacter laneus YIT 12061, Paraprevotella clara YIT 11840, and Paraprevotella xylaniphila YIT 11841. In the apelin signalling pathway, all three promising SMs are derived from the GM and are not potential SMs in phytochemicals from HVs. For example, Eubacterium limosum is abundant on germinated barley foodstuff (GBF) and can produce more dihydroglycitein in humans [Citation53]. Additionally, derivatives processed from barley malts enhanced the density of SCFAs in the caecum of rats [Citation54]. Although there was no clear feedback between HV (barley) and the six GMs, we were able to confirm that the potential anti-NAFLD alleviator effects of the four SMs: (1) Tryptanthrin from HV, (2) Dihydroglycitein, (3) 1,3-Diphenylpropan-2-ol, and (4) (10[(Acetyloxy)methyl]-9-anthryl)methyl acetate from GM.
Taken together, these findings reveal the significance of the GM as a key determinant of HV (barley).
The highlights and challenges of this study
With the assistance of NPs, the incorporation of HV and GM is a methodology for elucidating novel applications for existing, verified, or known medicinal agents by merging key molecules from exogenous species (or HV) and endogenous species (or GM) in complicated microbiome studies.
This study investigated whether molecules from HVs and metabolites from the GM affect certain key mechanism(s) of NAFLD. At present, bioinformatics, cheminformatics, and microbiome databases have been developed to gather significant therapeutic information in this extensive scientific field. To clarify, this study aimed to determine the key GM, mechanism(s), target(s), and molecule(s) involved in treating NAFLD by incorporating them into HVs. Currently, a new platform devised in this study can be used to input new data in the context of translational science. Nonetheless, it remains a certain challenge that our study should be performed further in vivo or in clinical trials.
Conclusion
In conclusion, our study indicates the significance of the combined administration of GM and HV (barley) against NAFLD, suggesting that both an agonist (tryptanthrin derived from HV) of JUN and antagonists (dihydroglycitein, 1,3-diphenylpropan-2-ol, and (10[(Acetyloxy)methyl]-9-anthryl)methyl acetate) are potential agents for inactivating the Apelin signalling pathway. Accordingly, the use of the apelin signalling pathway might have beneficial effects on NAFLD, blocking the underlying mechanism. These findings provide new insight into how key components can facilitate interactions between HVs (barley) and dietary fibres and the GM.
Author contributions
Ki-Tae Suk, Dong Joon Kim and Ki-Kwang Oh had substantial contributions to the conception or design for the work. Ki-Tae Suk, Ki-Kwang Oh, Su-Been Lee, Min-Gi Cha, Goo-Hyun Kwon, Haripriya Gupta, and Raja Ganesan were involved in the acquisition, or analysis for the work. Satya Priya Sharma, Sung-Min Won, Jin-Ju Jeong, Min-Kyo Jeong, Byeong-Hyun Min, and Ji-Ye Hyun performed the interpretation of data for the work. Jung-A Eom, Hee-Jin Park, Sang-Jun Yoon, Sang Youn Lee, and Mi-Ran Choi drafted the work or conducted the revising it critically for important intellectual content. All authors agree to be accountable for all aspects of the work in ensuring that questions related to the accuracy or integrity of any part of the work are appropriately investigated and resolved.
Supplemental Material
Download MS Excel (10.2 KB)Supplemental Material
Download MS Excel (17.1 KB)Supplemental Material
Download MS Excel (10.6 KB)Supplemental Material
Download MS Excel (11.8 KB)Supplemental Material
Download MS Excel (11 KB)Supplemental Material
Download MS Excel (68.7 KB)Acknowledgements
A preliminary version of this paper appeared as a Preprint [Citation55], which was re-elaborated during authors’ stay at Center for Microbiome, Institute for Liver and Digestive Diseases in October 2022 and May 2023 respectively. The authors wish to thank the Hallym university for exceptional working conditions.
Disclosure statement
No potential conflict of interest was reported by the author(s).
Data availability statement
The authors confirm that the data supporting the findings of this study are available within the article and its supplementary materials.
Additional information
Funding
References
- Adams LA, Lindor KD. Nonalcoholic fatty liver disease. Ann Epidemiol. 2007;17(11):863–869. doi: 10.1016/j.annepidem.2007.05.013.
- Targher G, Byrne CD, Lonardo A, et al. Non-alcoholic fatty liver disease and risk of incident cardiovascular disease: a meta-analysis. J Hepatol. 2016;65(3):589–600. doi: 10.1016/j.jhep.2016.05.013.
- Galatou E, Mourelatou E, Hatziantoniou S, et al. Nonalcoholic steatohepatitis (NASH) and atherosclerosis: explaining their pathophysiology, association and the role of incretin-based drugs. Antioxidants. 2022;11(6):1060. doi: 10.3390/antiox11061060.
- LeBlanc JG, Chain F, Martín R, et al. Beneficial effects on host energy metabolism of short-chain fatty acids and vitamins produced by commensal and probiotic bacteria. Microb Cell Fact BioMed Central Ltd. 2017;16:1–10.
- Zhang X, Ji X, Wang Q, et al. New insight into inter-organ crosstalk contributing to the pathogenesis of non-alcoholic fatty liver disease (NAFLD). Protein Cell. 2017;9(2):164–177. doi: 10.1007/s13238-017-0436-0.
- Badr A, Müller K, Schäfer-Pregl R, et al. On the origin and domestication history of barley (Hordeum vulgare). Mol Biol Evol. 2000;17(4):499–510. doi: 10.1093/oxfordjournals.molbev.a026330.
- Jan S, Khan MN, Bhat MA, et al. Barley (Hordeum vulgare L.) classification, diversity and health benefits: a review. Eur J Exp Biol. 2022;12:1–6.
- Ishiyama S, Kimura M, Umihira N, et al. Consumption of barley ameliorates the diabetic steatohepatitis and reduces the high transforming growth factor β expression in mice grown in α-minimum essential medium in vitro as embryos. Biochem Biophys Rep. 2021;27, 101029. doi: https://doi.org/10.1016/j.bbrep.2021.101029.
- Liu H, Chen T, Xie X, et al. Hepatic lipidomics analysis reveals the ameliorative effects of highland barley β-Glucan on Western diet-induced nonalcoholic fatty liver disease mice. J Agric Food Chem. 2021;69(32):9287–9298. doi: 10.1021/acs.jafc.1c03379.
- Leentjens J, Quintin J, Gerretsen J, et al. The effects of orally administered beta-glucan on innate immune responses in humans, a randomized open-label intervention pilot-study. PLOS One. 2014;9(9):e108794. doi: 10.1371/journal.pone.0108794.
- Rahar S, Swami G, Nagpal N, et al. Preparation, characterization, and biological properties of β-glucans. J Adv Pharm Technol Res. 2011;2:94.
- Song MY, Kim BS, Kim H. Influence of Panax ginseng on obesity and gut microbiota in obese middle-aged Korean women. J Ginseng Res. 2014;38(2):106–115. doi: 10.1016/j.jgr.2013.12.004.
- Chen X, Wang X, Ma L, et al. The network pharmacology integrated with pharmacokinetics to clarify the pharmacological mechanism of absorbed components from Viticis fructus extract. J Ethnopharmacol. 2021;278:114336. doi: 10.1016/j.jep.2021.114336.
- Zhu H, Wang R, Hua H, et al. Network pharmacology exploration reveals gut microbiota modulation as a common therapeutic mechanism for anti-fatigue effect treated with MACA compounds prescription. Nutrients. 2022;14(8):14. doi: 10.3390/Nu14081533.
- Ding Y, Chen M, Wang Q, et al. Integrating pharmacology and microbial network analysis with experimental validation to reveal the mechanism of composite Sophora colon-soluble capsule against ulcerative colitis. Evid Based Complement Alternat Med. 2020;2020:9521073.
- Liu HX, Keane R, Sheng L, et al. Implications of microbiota and bile acid in liver injury and regeneration. J Hepatol. 2015;63(6):1502–1510. doi: 10.1016/j.jhep.2015.08.001.
- Zeng X, Zhang P, He W, et al. NPASS: natural product activity and species source database for natural product research, discovery and tool development. Nucleic Acids Res. 2018;46(D1):D1217–D1222. doi: 10.1093/nar/gkx1026.
- Keiser MJ, Roth BL, Armbruster BN, et al. Relating protein pharmacology by ligand chemistry. Nat Biotechnol. 2007;25(2):197–206. doi: 10.1038/nbt1284.
- Daina A, Michielin O, Zoete V. SwissTargetPrediction: updated data and new features for efficient prediction of protein targets of small molecules. Nucleic Acids Res. 2019;47(W1):W357–W364. doi: 10.1093/nar/gkz382.
- Piñero J, Saüch J, Sanz F, et al. The DisGeNET cytoscape app: exploring and visualizing disease genomics data. Comput Struct Biotechnol J. 2021;19:2960–2967. doi: 10.1016/j.csbj.2021.05.015.
- Amberger JS, Bocchini CA, Schiettecatte F, et al. OMIM.org: online Mendelian inheritance in man (OMIM®), an online catalog of human genes and genetic disorders. Nucleic Acids Res. 2015;43(Database issue):D789–98. doi: 10.1093/nar/gku1205.
- Szklarczyk D, Gable AL, Nastou KC, et al. The STRING database in 2021: customizable protein-protein networks, and functional characterization of user-uploaded gene/measurement sets. Nucleic Acids Res. 2021;49(D1):D605–D612. doi: 10.1093/nar/gkaa1074.
- Morris GM, Huey R, Lindstrom W, et al. AutoDock4 and AutoDockTools4: automated docking with selective receptor flexibility. J Comput Chem. 2009;30(16):2785–2791. doi: 10.1002/jcc.21256.
- Singh N, Chaput L, Villoutreix BO. Virtual screening web servers: designing chemical probes and drug candidates in the cyberspace. Brief Bioinform. 2021;22(2):1790–1818. doi: 10.1093/bib/bbaa034.
- Soo H-C, Chung FF-L, Lim K-H, et al. Cudraflavone C induces tumor-specific apoptosis in colorectal cancer cells through inhibition of the phosphoinositide 3-kinase (PI3K)-AKT pathway. PLOS One. 2017;12(1):e0170551. doi: 10.1371/journal.pone.0170551.
- Kanehisa M, Furumichi M, Sato Y, et al. KEGG for taxonomy-based analysis of pathways and genomes. Nucleic Acids Res. 2023;51(D1):D587–D592. doi: 10.1093/nar/gkac963.
- Trott O, Olson AJ. AutoDock vina: improving the speed and accuracy of docking with a new scoring function, efficient optimization, and multithreading. J Comput Chem. 2010;31(2):455–461. doi: 10.1002/jcc.21334.
- Shityakov S, Förster C. In silico predictive model to determine vector-mediated transport properties for the blood-brain barrier choline transporter. Adv Appl Bioinform Chem. 2014;7:23–36. doi: 10.2147/AABC.S63749.
- Laskowski RA, Swindells MB. LigPlot+: multiple ligand-protein interaction diagrams for drug discovery. J Chem Inf Model. 2011;51(10):2778–2786. doi: 10.1021/ci200227u.
- Daina A, Michielin O, Zoete V. SwissADME: a free web tool to evaluate pharmacokinetics, drug-likeness and medicinal chemistry friendliness of small molecules. Sci Rep. 2017;7(1):42717. doi: 10.1038/srep42717.
- Lamothe SM, Guo J, Li W, et al. The human ether-a-go-go-related gene (hERG) potassium channel represents an unusual target for protease-mediated damage. J Biol Chem. 2016;291(39):20387–20401. doi: 10.1074/jbc.M116.743138.
- Mulliner D, Schmidt F, Stolte M, et al. Computational models for human and animal hepatotoxicity with a global application scope. Chem Res Toxicol. 2016;29(5):757–767. doi: 10.1021/acs.chemrestox.5b00465.
- Bourcier T, McGovern T, Stavitskaya L, et al. Improving prediction of carcinogenicity to reduce, refine, and replace the use of experimental animals. J Am Assoc Lab Anim Sci. 2015;54:163.
- Lei T, Li Y, Song Y, et al. ADMET evaluation in drug discovery: 15. Accurate prediction of rat oral acute toxicity using relevance vector machine and consensus modeling. J Cheminform. 2016;8:1–19.
- Fan Y, Fu Y, Zhou Y, et al. Acute, subacute oral toxicity and Ames test of Py-mulin: an antibacterial drug candidate. BMC Pharmacol Toxicol. 2022;23:1–12.
- Wang Q, Li X, Yang H, et al. In silico prediction of serious eye irritation or corrosion potential of chemicals. RSC Adv. 2017;7(11):6697–6703. doi: 10.1039/C6RA25267B.
- Ozdal T, Sela DA, Xiao J, et al. The reciprocal interactions between polyphenols and gut microbiota and effects on bioaccessibility. Nutrients. 2016;8(2):78. doi: 10.3390/nu8020078.
- Wang LQ, Meselhy MR, Li Y, et al. The heterocyclic ring fission and dehydroxylation of catechins and related compounds by Eubacterium sp. strain SDG-2, a human intestinal bacterium. Chem Pharm Bull. 2001;49(12):1640–1643. doi: 10.1248/cpb.49.1640.
- Nagai F, Morotomi M, Watanabe Y, et al. Alistipes indistinctus sp. nov. and Odoribacter laneus sp. nov., common members of the human intestinal microbiota isolated from faeces. Int J Syst Evol Microbiol. 2010;60(Pt 6):1296–1302. doi: 10.1099/ijs.0.014571-0.
- Morotomi M, Nagai F, Sakon H, et al. Paraprevotella clara gen. nov., sp. nov. and Paraprevotella xylaniphila sp. nov., members of the family “prevotellaceae” isolated from human faeces. Int J Syst Evol Microbiol. 2009;59(Pt 8):1895–1900. doi: 10.1099/ijs.0.008169-0.
- Fuest M, Willim K, MacNelly S, et al. The transcription factor c-jun protects against sustained hepatic endoplasmic reticulum stress thereby promoting hepatocyte survival. Hepatology. 2012;55(2):408–418. doi: 10.1002/hep.24699.
- Hasenfuss SC, Bakiri L, Thomsen MK, et al. Regulation of steatohepatitis and PPARγ signaling by distinct AP-1 dimers. Cell Metab. 2014;19(1):84–95. doi: 10.1016/j.cmet.2013.11.018.
- Shaulian E, Karin M. AP-1 as a regulator of cell life and death. Nat Cell Biol. 2002;4(5):E131–E136. doi: 10.1038/ncb0502-e131.
- Kaur R, Manjal SK, Rawal RK, et al. Recent synthetic and medicinal perspectives of tryptanthrin. Bioorg Med Chem. 2017;25(17):4533–4552. doi: 10.1016/j.bmc.2017.07.003.
- Alhuthali HM, Bradshaw TD, Lim KH, et al. The natural alkaloid jerantinine B has activity in acute myeloid leukemia cells through a mechanism involving c-jun. BMC Cancer.. 2020;20:1–9.
- Lee K-C, Hsieh Y-C, Chan C-C, et al. Human relaxin-2 attenuates hepatic steatosis and fibrosis in mice with non-alcoholic fatty liver disease. Lab Invest. 2019;99(8):1203–1216. doi: 10.1038/s41374-019-0240-y.
- Quinn MA, Xu X, Ronfani M, et al. Estrogen deficiency promotes hepatic steatosis via a glucocorticoid receptor-dependent mechanism in mice. Cell Rep. 2018;22(10):2690–2701. doi: 10.1016/j.celrep.2018.02.041.
- He S, Cui S, Song W, et al. Interleukin-17 weakens the NAFLD/NASH process by facilitating intestinal barrier restoration depending on the gut microbiota. mBio. 2022;13(2):e0368821. doi: 10.1128/mbio.03688-21.
- Ritter MJ, Amano I, Hollenberg AN. Thyroid hormone signaling and the liver. Hepatology. 2020;72(2):742–752. doi: 10.1002/hep.31296.
- Liss KHH, Finck BN. PPARs and nonalcoholic fatty liver disease. Biochimie. 2017;136:65.
- Huang J, Kang S, Park SJ, et al. Apelin protects against liver X receptor-mediated steatosis through AMPK and PPARα in human and mouse hepatocytes. Cell Signal. 2017;39:84–94. doi: 10.1016/j.cellsig.2017.08.003.
- Wang Y, Song J, Bian H, et al. Apelin promotes hepatic fibrosis through ERK signaling in LX-2 cells. Mol Cell Biochem. 2019;460(1–2):205–215. doi: 10.1007/s11010-019-03581-0.
- Kanauchi O, Fujiyama Y, Kehchimitsuyama Araki Y, et al. Increased growth of Bifidobacterium and Eubacterium by germinated barley foodstuff, accompanied by enhanced butyrate production in healthy volunteers. Int J Mol Med. 1999;3:175–179.
- Teixeira C, Prykhodko O, Alminger M, et al. Barley products of different fiber composition selectively change microbiota composition in rats. Mol Nutr Food Res. 2018;62:1701023.
- Lee S-B, Gupta H, Ganesan R, et al. The combined application of Hordeum vulgare and gut microbiota against non-alcoholic fatty liver disease via network pharmacology approach. 2022; 1-20. doi: 10.21203/RS.3.RS-2107064/V1.