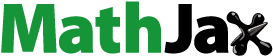
Abstract
Land cover changes in the Tampa Bay watershed (Florida) over the past four decades were examined along with precipitation and wind observations to help understand causes of long-term changes in turbidity and chlorophyll concentration within the Tampa Bay estuary. Water quality showed a statistically significant relationship to land cover fraction in the watershed compared to long-term precipitation or wind stress. Redundancy Analyses with Akaike’s Information Criterion and non-parametric multiple regressions determined that turbidity and chlorophyll concentration decreased bay-wide from 1974–2012 with increased developed land fraction (R2 > 0.75, p-value < 0.05). Various segments of the estuary showed different significant responses to developed land (R2 > 0.75, p-value < 0.05), agricultural land (R2 > 0.93, p-value < 0.02), bare land (R2 = 0.77, p-value = 0.001), and wind stress (R2 = 0.91, p-value = 0.04) at different times of year.
Public Interest Statement
Monitoring coastal water quality and understanding how and why it changes is important for anyone interested in enjoying activities such as swimming, boating, and fishing, as well as for predicting and responding to harmful algal blooms that can cause respiratory issues. This paper identifies the factors that affect water quality in Tampa Bay, Florida. We found that development of much of the land surrounding Tampa Bay coincides with improved water quality since the 1970s. This is likely due to a decrease of sediment input to the bay that has been shown to follow land development (e.g. parking lots and roads). These results do not necessarily indicate that they bay is “healthier” with more development, and should be considered alongside the policy and management efforts that have reduced nutrient pollution to the bay, resulting in improved water quality.
1. Introduction
The quality of estuarine and other coastal waters is a complex function of hydrological, meteorological, oceanographic, and human drivers (Chen, Hu, Muller-Karger, & Luther, Citation2010; Eleveld, van der Wal, & van Kessel, Citation2014; Moreno Madriñán, Al-Hamdan, Rickman, & Ye, Citation2012; Schmidt, Luther, & Johns, Citation2004; Yin et al., Citation2005). The interplay between these different processes may lead to different water-quality outcomes over time. In Tampa Bay, Florida, turbidity and average chlorophyll concentration have decreased since the 1970s (Janicki, Pribble, Janicki, & Winowitch, Citation2001; Moreno Madriñán et al., Citation2012). This is a result of the upgrade of waste water treatment plants to tertiary level starting in 1979 and other voluntary and regulated reductions in point-source pollution. However, nonpoint sources have not been managed as effectively. Greening, Janicki, Sherwood, Pribble, and Johansson (Citation2014) found that nitrogen contributions of point and nonpoint sources to Tampa Bay were 60.3 and 23.9%, respectively, of the total nitrogen loadings in the 1970s. By the 2000s, the relative contributions were inverted, with point sources contributing about 19.5% and nonpoint 57.4% to nitrogen discharges into the bay. Major nonpoint sources in the Tampa Bay watershed include urban areas, phosphate mining, and agricultural land use (Greening et al., Citation2014). In this study, we evaluate the role of land cover changes, precipitation, and wind stress on turbidity and chlorophyll-a in Tampa Bay between the 1970s and 2010.
Turbidity is a measure of light transparency in aquatic environments used by the US Environmental Protection Agency (EPA) as an index of water quality. Turbidity may be influenced by changes in the concentration of colored dissolved matter and suspended particulates including sediment and phytoplankton. These variables are affected by changes in hydrological, meteorological, and oceanographic phenomena (Chen, Hu, Conmy, Muller-Karger, & Swarzenski, Citation2007; Chen et al., Citation2010; Eleveld et al., Citation2014; Miller, Liu, Buonassissi, & Wu, Citation2011). Turbid water typically increases light attenuation, thereby reducing the amount of light available to reach benthic vegetation such as seagrass, which may kill seagrass beds and degrade the associated ecosystem. Turbidity caused by phytoplankton blooms may result in harmful red tides, or hypoxia events, which starve local benthic ecosystems of oxygen and subsequently kill local organisms. These types of turbidity events are closely monitored by environmental agencies that may issue management actions in response or release public health alerts as needed.
Land cover and land use affect downstream water quality through run-off of freshwater, nutrients, sediment, and pollution (Bateni, Fakheran, & Soffianian, Citation2013; Jordan, Ghulam, & Herrmann, Citation2012; Miller, Schoonover, Williard, & Hwang, Citation2011; Wickham, Riitters, Wade, & Jones, Citation2005). Nelson and Booth (Citation2002) conducted a watershed-scale sediment budget analysis in western Washington state, and found that urban development and subsequent stream-channel erosion has contributed an increase of nearly 50% in the annual sediment yield. However, impervious surfaces (e.g. roads, buildings, parking lots) enhance sediment-free stormwater run-off by reducing the amount of exposed soil that may be carried downstream (Estes et al., Citation2009; Miller, Schoonover, et al., Citation2011; Moreno Madriñán et al., Citation2012). Miller, Schoonover, et al. (Citation2011) developed regression models for 43 watersheds in Illinois. They found that during base flow conditions, agriculture-dominated watersheds had significantly higher turbidity and total suspended solid concentrations compared with urban watersheds, likely due to erosion of exposed soil from conventional tillage or crop harvesting. Turbidity during storm flow conditions was also significantly lower in urban watersheds. Moreno Madriñán et al. (Citation2012) found a negative relationship between the fraction of developed land in each watershed segment and turbidity at the mouths of the rivers entering Tampa Bay. Reduced sediment concentrations in stormwater run-off may enhance downstream light penetration of the affected water bodies, thereby increasing photosynthetically available radiation to benthic vegetation, while increased stream sediment concentrations can severely harm aquatic life (Sheeder & Evans, Citation2004).
Precipitation in the watershed affects estuarine water quality through increased nutrient and sediment discharge into rivers (Al-Taani, Citation2014; Estes et al., Citation2009; Jordan et al., Citation2012; Miller, Liu, et al., Citation2011). Le, Hu, English, Cannizzaro, and Kovach (Citation2013) used satellite-derived chlorophyll concentration maps of Tampa Bay from 1998–2011 to show that river discharge explains approximately 60% of seasonal variability and about 90% of interannual variability in chlorophyll in the bay. Interannual variability was strongly influenced by El Niño-Southern Oscillation cycles and tropical cyclones. Le et al. (Citation2013) were not able to detect long-term variations, in part because the satellite data record used was short. Eleveld et al. (Citation2014) compared satellite-derived water-quality products with modeled water quality and found that sun-synchronous satellites alias tidal patterns and are also biased by acquiring usable data under cloud-free conditions. These constraints led to biases in satellite-derived water-quality products (Eleveld et al., Citation2014). Schoonard, Collins, Paxton, and Meindl (Citation2014) examined spatial patterns of precipitation in Pinellas County, which forms the western boundary of Tampa Bay, from 2003 to 2007 and found that convective storms related to the seabreeze during the summer wet season were highly spatially variable and heavily influenced by dominant wind direction. This process results in a broad and diffuse discharge into Tampa Bay which cannot be quantified as river discharge. Most of the river discharge enters Tampa Bay from the north and eastern sides of the bay.
Winds also influence sediment load by resuspension in Tampa Bay and in other coastal areas (Chen, Hu, & Muller-Karger, Citation2007; Chen, Muller-Karger, & Hu, Citation2007; Hu et al., Citation2004; Miller & McKee, Citation2004). Wind stress is a well-known driver of sediment resuspension (Demers, Therriault, Bourget, & Bah, Citation1987; Madsen, Wright, Boon, & Chisholm, Citation1993; Schoen, Stretch, & Tirok, Citation2014; Sheng & Lick, Citation1979). In Tampa Bay, turbidity is directly related to seasonal wind forcing, especially in the lower segment of the estuary (Chen, Hu, & Muller-Karger, Citation2007, Chen et al., Citation2010).
1.1. Study area
Tampa Bay (27.5–28.08° N and 82.36–82.75° W) is the largest open-water estuary in Florida (Figure ). It covers over 1,000 km2 at high tide, with an average depth of 3.4 m. The watershed area covers over 6,500 km2. Six counties and the city of Tampa, the second largest metropolitan area in Florida, intersect the watershed. An estimated 2.3 million people lived in the Tampa Bay watershed in 2003, with population growth between 1990 and 2003 reaching ~22% (US Census, Citation2007). Approximately 500 new residents moved to counties surrounding Tampa Bay each week during this timeframe.
Figure 1. The Tampa Bay watershed study area as defined by the National Estuary Program.

The Tampa Bay watershed has historically been divided into segments (Janicki et al., Citation2001; see Figure ). The landward extent of the watershed for each segment was defined using the level eight hydrologic units of the Tampa Bay watershed. Each land cover map (described below) was clipped to match the watershed for each bay segment. The Hillsborough Bay (HB) watershed (3,192 km2) is dominated by wetland and agricultural land. We limited Old Tampa Bay (OTB) to the area north of a causeway (specifically Gandy Bridge) because this structure limits water exchange with the rest of Tampa Bay (Zhu, Weisberg, Zheng, & Han, Citation2014). Figure shows the gap we created by limiting the coverage of what is formally known as OTB. The OTB watershed (822 km2) is dominated by developed land and wetland. The Middle Tampa Bay (MTB) watershed (1,073 km2) is dominated by agricultural land and wetland. Land cover area from each of these segments was combined for an aggregated assessment (hereafter referred to as Bay-Wide or Upper TB). The Lower TB segment was excluded from this study because water quality here is more strongly influenced by oceanographic processes of exchange with the Gulf of Mexico than by the adjacent watershed (Zhu et al., Citation2014). Thirty-year precipitation and temperature normals based on data during the period 1981–2010 from this station are presented in Table .
Figure 2. Top panel: USGS map representing land use classes for 1970–1985. Bottom panel: C-CAP map representing land use classes during the three-year period centered on 2010 (2009–2011).

Table 1. Annual and seasonal precipitation and temperature normals (1981–2010)
1.2. Hypotheses
The following null hypotheses guided this study:
(1) | Neither bay-segment nor bay-wide turbidity nor chlorophyll-a are significantly related to land cover, precipitation, or wind stress (p > 0.05); | ||||
(2) | Neither bay-segment nor bay-wide periods of high turbidity or high chlorophyll concentration are significantly related to land cover, precipitation, or wind stress (p > 0.05); | ||||
(3) | Neither bay-segment nor bay-wide periods of low turbidity or low chlorophyll concentration are significantly related to land cover, precipitation or wind stress (p > 0.05); |
We examined precipitation, wind stress, and historical land use changes as possible drivers of changes in water quality within Tampa Bay over the period 1970s–2010. Turbidity and chlorophyll concentration served as indices of water quality. Analyses focused on possible relationships between water quality within each segment of the bay and changes in the watershed. A similar analysis was done for bay-wide conditions. We examined changes over time since the 1970s, within annual periods as well as during periods of high and low turbidity and chlorophyll concentration separately. The null hypotheses tested leave room for the possibility that other variables not addressed in this work may explain the long-term water-quality patterns of Tampa Bay.
2. Materials and methods
2.1. Land cover data
Land cover maps were downloaded from the United States Geological Survey (USGS) Enhanced Historical Land-Use and Land-Cover Data Sets (http://water.usgs.gov/GIS/dsdl/ds240/index.html) and the National Oceanic and Atmospheric Administration (NOAA) Coastal-Change Analysis Program (C-CAP; http://csc.noaa.gov/ccapftp/). The former was created using high-altitude aerial photographs of the US collected in 1970–1985. The USGS land cover data-sets were created to support the National Water-Quality Assessment Program and other environmental impact assessments (Anderson, Hardy, Roach, & Witmer, Citation1976; Price, Nakagaki, Hitt, & Clawges, Citation2007). The data represent the initial land cover status (1970–1985; Figure ) and will hereafter be referred to as the 1977 map as the central year of this period. The specific date of each classified region within this period is not documented. The USGS maps were digitized at a scale of 1:250,000. The minimum size of most class polygons is 4 hectares, which equates to approximately five 900 m2 C-CAP pixels, although for some it is 16 hectares. Higher resolution mapping units would be more appropriate for a comparison with C-CAP maps, but were not available for the temporal, geographic and thematic requirements of this research. Seven class groups were identified for this region based on the Anderson Level II land use codes: urban or built-up land, agricultural land, rangeland (i.e. grass/brush), forest land, water, wetland, and barren land.
NOAA C-CAP maps were created from the National Land Cover Database (NLCD). These were derived from 30 m spatial resolution Landsat satellite images. Each mapped product was based on imagery from multiple years centered on a nominal date, specifically 1996 (1995–1997), 2001 (2000–2002), 2006 (2005–2007), and 2010 (2009–2011) (Figure ). Multiple years of satellite imagery were required to create the maps to minimize interference from cloud cover. C-CAP maps for this region include class groups similar to those described above from the USGS: developed land, agricultural land, grassland, forest land, scrubland, barren land, unconsolidated shore, palustrine wetland, estuarine wetlands, and water and submerged lands. Based on the definitions of the classes, C-CAP grassland and scrubland classes were merged into one “rangeland” class for consistency with the USGS maps. Unconsolidated shore effectively refers to beaches for this area, which were not identified in the USGS maps, but was retained as an explanatory variable as it constitutes a source of erodible sediment immediately adjacent to the water body and may therefore modulate turbidity.
A comparison of the USGS and NOAA land use products with wetland maps produced by the National Wetlands Inventory (NWI) and the Southwest Florida Water Management District (SWFWMD) (Rains, Landry, Seidel, & Crisman, Citation2012) suggested that the USGS and NOAA products substantially overestimate wetland area at the expense of forest area. C-CAP maps show over 2,000 km2 of wetlands for 1996, 2001, and 2006. NWI and SWFWMD report approximately 800–900 km2 in wetlands for the same time periods. A previous C-CAP accuracy assessment indeed reports high errors of omission and of commission (up to 94%) for wetland and forest classes (Assessment Report, Citation2013). To minimize biases in our conclusions, we merged wetland and forest land classes for USGS and C-CAP maps into one forest/wetland class. We were left with seven land cover explanatory variables: agriculture, bare, developed, rangeland, water, unconsolidated shore, and forest/wetland.
2.2. Water-quality data
In situ water-quality data for each segment of the Tampa Bay estuary were obtained from the Environmental Protection Commission of Hillsborough County (EPCHC; the Tampa Bay Water Atlas: http://www.tampabay.wateratlas.usf.edu). Samples were collected monthly at over 60 stations in Tampa Bay from 1974–2011. Anomalously high turbidity values (i.e. turbidity > 24 NTU) were removed following Chen et al. (Citation2010). Data were averaged over the following epochs to match the land cover map periods: 1976–1978, 1995–1997, 2000–2002, 2005–2007, and 2009–2011. The first epoch (1976–1978) was chosen as the middle three years of the USGS land cover map period, instead of the entire period (1970–1985), to maintain consistency with the other three-year epochs examined.
Monthly water-quality climatologies (averages calculated for each month from every year of available data) were computed based on data from 1974–2011. These climatologies were used to identify periods of higher or lower turbidity, and higher or lower chlorophyll concentration. Periods of reduced and enhanced water quality were assessed separately to help understand possible interactions between land cover and precipitation-driven run-off on water quality. Separating these seasons as opposed to conducting an analysis that simply aggregates data by epoch over entire annual periods helped to minimize possible confounding of zero-precipitation periods and the land cover change.
High-turbidity and high-chlorophyll periods were identified as those months in which climatology values exceeded the climatological average plus one standard deviation. Low turbidity and low-chlorophyll periods were identified simply as those months in which climatology values fell below the climatological mean. High-turbidity and low-turbidity months were examined separately from high-chlorophyll and low-chlorophyll months. Low turbidity and low chlorophyll months represent “background” water-quality conditions. Averages for each of those periods, and annual overall averages, were computed for all epochs. These were used as the “response variable” in analyses over the corresponding epochs.
2.3. Meteorological data
Daily precipitation and wind speed data for Tampa International Airport (1893–2012) were downloaded from the National Climate Data Center (NCDC; http://www.ncdc.noaa.gov/). These data were binned to monthly values. Precipitation was assumed to have a cumulative effect and was therefore binned monthly by summing daily data. Daily East-West (u) and North-South (v) wind components were averaged separately before recombining to compute average daily wind speed and direction (see method of Gilhousen, Citation1987). Wind stress (τ) was computed from the daily wind speed data (Equation 1), and binned as monthly averages (Wu, Citation1969).
Equation 1:
where CD is a drag coefficient (1.2 × 10−3), ρair is the density of air (1.22 kg/m3), and U is wind velocity.
Averages of monthly precipitation and wind stress were computed for annual, high, and low water-quality periods. Both meteorological variables (precipitation and wind stress) were used as explanatory variables.
2.4. Statistical analyses
Standard normal homogeneity tests (SNHT) were run on all variables (the original wind speed data was used to represent wind stress) to find breaks in the data-sets which may indicate changes in sampling technique or location. Significance values were computed using 10,000 Monte Carlo simulations. The Monte Carlo Simulation is a technique that converts uncertainties in input variables of a model into probability distributions over output variables. By combining the distributions and randomly selecting values from them, it recalculates the simulated model many times and brings out the probability of the output (Kuo, Chu, Pan, & Yu, Citation2011; Yue, Pilon, & Cavadias, Citation2002). Mann–Kendall Trend Tests (MK) were run on each time series of monthly values to evaluate possible trends (Mei et al., Citation2014). The MK test does not assume any particular distributional form and accommodates values below the detection limit by assigning them a common value, including censored and missing data (Hamed, Citation2007; Méndez-Lázaro, Muller-Karger, Otis, McCarthy, & Peña-Orellana, Citation2014; Méndez-Lázaro, Nieves-Santiango, & Miranda-Bermúdez, Citation2014; Xu, Liu, Fu, & Chen, Citation2010).
Multiple regressions alone would be insufficient for this study because of the high ratio of explanatory variables (7 land cover classes, precipitation and wind stress) to sample size (9:5). Therefore, Redundancy Analyses with Akaike’s Information Criterion (RDA AIC) were used first to identify those explanatory variables that explained the most variation in the response variable. The RDA AIC function standardized all input explanatory variables and determined the best explanatory variables through constrained ordination. This assessed how much of the variation in one set of variables explained variation in another set, while accounting for explanatory variable multicollinearity (van den Wollenberg, Citation1977). Akaike (Citation1973) proposed an information criterion to quantify the amount of information and statistically determine the number of parameters for an equation that represents a group of experimental data. The equation with the minimum AIC is considered the best representation of the experimental data (Yamaoka, Nakagawa, & Uno, Citation1978). A null model is created by assigning a value below which the best equation’s AIC value must be in order to be considered viable to explain variation in the dependent variable. If no equation explains more variation than a null model, no independent variable is selected.
Multiple regressions were run on the variable(s) identified as “best” by the RDA AIC. One thousand iterations were run for each regression to compute permutation-based p-values because much of the data were not normally distributed. Finally, an outlier test was run on all significant relationships because any one outlier could have significantly influenced results since we only have five sample epochs. For each observation, an outlying value was identified as exceeding “N” divided by the sum of squared differences, normalized by subtracting the median and dividing by the median absolute deviation (i.e. median of absolute value of each sample minus the median of the array). Breiman and Culter (Citation2003) suggested that values > 10 be considered outliers when using this method.
3. Results
Percent of watershed area that was covered by each class for each map epoch are shown in Tables . Total percent is shown at the bottom of each table. This number is <100 for each 1970s map because these used an additional class called “transitional areas”. “Transitional areas” were defined “by the lack of remote sensor information which could enable the interpreter to predict reliably the future use or discern the past use” (Anderson et al., Citation1976). Because land of this class could not be accurately described, and it could not be reconciled with any C-CAP class, it was excluded from this analysis. Percent cover of each class for each bay segment is shown in Tables .
Table 2. Hillsborough Bay land cover class as percent of watershed
Table 3. Old Tampa Bay land cover class as percent of watershed
Table 4. Middle Tampa Bay land cover class as percent of watershed
Table 5. Upper Tampa Bay land cover class as percent of watershed
Turbidity and chlorophyll averages for each segment and respective segment area are presented in Table . Upper Tampa Bay turbidity and chlorophyll, and Tampa International Airport precipitation, wind speed time series, and climatologies are shown in Figure . Monthly turbidity and chlorophyll climatologies for each bay segment and Upper Tampa Bay are shown in Figures and . Table lists the months that were identified for each bay segment as “high” and “low” for turbidity and chlorophyll.
Table 6. Average turbidity and chlorophyll concentrations for the study areas with their respective bay and watershed areas (1974–2012)
Figure 3. Monthly time series (blue) and mean annual cycle (red) for bay-wide turbidity and chlorophyll, and Tampa International Airport precipitation and wind speed.

Table 7. Months corresponding to high and low turbidity and chlorophyll for each bay segment
SNHT evaluations found significant changes in wind speed, chlorophyll concentration, and turbidity time series. The time series of wind speed shows a significant decrease in values starting in May 1993. The mean of the data before May 1993 was 3.45 m/s and average wind speed decreased gradually over time through December 2012, to give an average of 3.02 m/s for this period following the break (Figure (a)). Bay-wide chlorophyll concentration showed a change in December of 1983. The mean of the data prior to December 1983 was 16.1 and 9.6 mg/m3afterward (Figure (b)). This change corresponds to the period of intensive wastewater treatment plant improvements, but it also marks a period of substantial increase in turbidity in Tampa Bay. Bay-wide turbidity showed marked variability over time. Turbidity was about 5 NTU on average before 1989. Turbidity was anomalously high between 1990 and 1993. The mean of the data after this period was about 3.4 NTU (Figure (c)). Precipitation data tested as homogenous (i.e. no apparent mid-series breaks; Figure (d)).
Figure 6. Monthly time series plots of bay-wide wind speed, chlorophyll concentration, turbidity and precipitation with averages (mu) of break periods where applicable as identified by SNHT tests.

SNHT evaluations on monthly turbidity (Figure (a–c)) and chlorophyll concentration (Figure (a–c)) binned within each bay segment also show discontinuities. Turbidity breaks occurred in June of 1997, May of 1996, and June of 2000 for HB, OTB and MTB, respectively. The means of the data before and after the breaks were 6.55 NTU and 4.21 NTU (HB), 5.05 NTU and 3.41 NTU (OTB), and 4.21 NTU and 2.70 NTU (MTB). All three breaks follow a period of high turbidity that was sustained for several years during the early 1990s.
Figure 7. Monthly time series plots of turbidity for HB (a), OTB (b), and MTB (c) with averages (mu) of break periods, where applicable, as identified by SNHT tests.

Figure 8. Monthly time series plots of chlorophyll concentration for HB (a), OTB (b), and MTB (c) with averages (mu) of break periods where applicable as identified by SNHT tests.

Chlorophyll concentration breaks occurred in December of 1983, January of 1984, and December of 1985 for HB, OTB and MTB, respectively. Again, these changes coincide with the initial indications of the increase in turbidity leading to the turbidity maxima observed in the early 1990s. The means of the data before and after the breaks were 26.48 and 12.91 mg/m3 (HB), 13.52 and 9.50 mg/m3 (OTB), and 12.78 and 7.14 mg/m3 (MTB).
MK tests found that monthly wind speed and stress decreased significantly (p < 0.017) every month during this 39-year period. No trends were found in monthly precipitation with the exception of marginally significant increases in April and decreases in May (p = 0.02 and p = 0.068, respectively). Bay-wide chlorophyll concentration decreased significantly every month (p < 0.022) except for September and October, during which it showed no change (p > 0.1). On average, bay-wide turbidity also decreased every month (p < 0.05) except for August and September, which again showed no change (p > 0.1). The months with no trend in turbidity coincided with the months showing peak chlorophyll concentration.
Variables identified as “best” by RDA AIC analyses are presented here with the corresponding multiple regression results (Tables ). OTB year-round turbidity was significantly negatively related to developed land percent cover (R2 = 0.76, p = 0.001; Figure (a)). MTB year-round turbidity was significantly positively related to agricultural land percent cover (R2 = 0.97, p = 0.001; Figure (b)). None of our variables explained HB year-round turbidity patterns over time.
Table 8. RDA AIC-identified “best” variables and multiple regression results for land cover, precipitation, and wind stress vs. turbidity for the Tampa Bay estuary and each segment
Table 9. RDA AIC-identified “best” variables and multiple regression results for land cover, precipitation, and wind stress vs. chlorophyll for the entire Tampa Bay estuary and for each separate segment
Figure 9. (a) Plot of annual average turbidity in Old Tampa Bay against developed land percent cover. (b) Plot of annual average turbidity in Middle Tampa Bay against agricultural land percent cover. (c) Plot of high-period turbidity in Old Tampa Bay against developed land percent cover. (d) Plot of high-period turbidity in Middle Tampa Bay against agricultural land percent cover. (e) Plot of low-period turbidity in Middle Tampa Bay against agricultural land percent cover. (f) Plot of low-period turbidity in Hillsborough Bay against wind stress.

Similar trends were observed for high turbidity months. OTB high-turbidity was significantly negatively related to developed land percent cover (R2 = 0.92, p = 0.001; Figure (c)). MTB high-turbidity was significantly positively related to agricultural land percent cover (R2 = 0.93, p = 0.021; Figure (d)). No variable explained HB high-turbidity.
During low-turbidity months, MTB turbidity was significantly positively related to agricultural land percent cover (R2 = 0.98, p = 0.001; Figure (e)). HB low-turbidity was also significantly positively related to wind stress (R2 = 0.91, p = 0.041; Figure (f)). This suggests that, during background turbidity months, increased wind stress led to an increase in turbidity. No variable could explain OTB low-turbidity better than a null model during this period.
HB year-round chlorophyll concentration was significantly positively related to agricultural land percent cover (R2 = 0.96, p = 0.001). This relationship was heavily influenced by an outlier in 1977, which, when removed, rendered the relationship insignificant. MTB year-round chlorophyll was significantly negatively related to developed land percent cover (R2 = 0.98, p = 0.019; Figure (a)). OTB year-round chlorophyll was negatively related to developed land percent cover, but the relationship was only marginally significant (R2 = 0.86, p = 0.095).
Figure 10. (a) Plot of annual chlorophyll concentration in Middle Tampa Bay against developed land percent cover. (b) Plot of high-period chlorophyll concentration in Middle Tampa Bay against bare land percent cover. (c) Plot of low-period chlorophyll concentration in Middle Tampa Bay against developed land percent cover.

During high-chlorophyll months, HB chlorophyll was significantly negatively related to forest/wetland percent cover (R2 = 0.91, p = 0.001). Again, this relationship was influenced by an outlier in 1977, which, when removed, rendered the relationship insignificant. MTB high-chlorophyll was found to be significantly negatively related to bare land percent cover (R2 = 0.77, p = 0.001; Figure (b)) during this period. No variable could explain OTB high-chlorophyll better than a null model.
During low-chlorophyll months, HB chlorophyll was significantly positively related to agricultural land percent cover (R2 = 0.98, p = 0.001), but this relationship was heavily influenced by an outlier in 1977, which, when removed, rendered the relationship insignificant. OTB low chlorophyll was found to be significantly negatively related to developed land percent cover (R2 = 0.92, p = 0.001), but this relationship was also heavily influenced by an outlier in 1977, which, when removed, rendered the relationship insignificant. MTB low chlorophyll was found to be significantly negatively related to developed land percent cover (R2 = 0.97, p = 0.02; Figure (c)).
Analyses for the entire upper bay found that year-round turbidity were significantly negatively related to developed land percent cover (R2 = 0.76, p = 0.02; Figure (a)). Year-round chlorophyll was significantly positively related to agricultural land percent cover (R2 = 0.97, p = 0.001). This relationship was influenced by an outlier in 1977, which, when removed, renders the relationship the insignificant. Bay-wide high turbidity was significantly negatively related to developed land percent cover (R2 = 0.83, p = 0.02; Figure (b)), but no variable could explain bay-wide low turbidity better than a null model. Bay-wide high chlorophyll was negatively related to developed land percent cover (R2 = 0.82, p = 0.043; Figure (c)). An outlier influenced the otherwise significant bay-wide low-chlorophyll relationship with agricultural land percent cover and rendered it insignificant when removed.
4. Discussion
Overall, monthly turbidity and chlorophyll in Tampa Bay show decreasing trends for all months from 1974 to 2011 (p < 0.05) except for August, September, and October, for which they show no significant trend. These three months correspond to the annual chlorophyll concentration peaks (Table ), which follow summer precipitation maxima for the region (Figure ). The lack of a significant trend in summer chlorophyll concentration over the study period suggests that variables or policies that have effected improved water quality for the rest of the year have had minimal impact on peak chlorophyll each year. This pattern may indicate that natural algal phenology during the summer chlorophyll concentration peak period overwhelms any anthropogenic or land use change signals. It could also, however, be related to elevated agricultural nutrient run-off during the summer rainy season. Further research into this specific period is warranted.
Figure 12. Monthly climatologies of bay-wide turbidity and chlorophyll, and Tampa International Airport precipitation and wind speed.

Increasing urbanization led to a threefold increase in developed-land within the Tampa Bay watershed from 1991–2002. Developed land replaced other land cover classes. This has led to a significant increase in impervious land surface in the watershed and resulted in increased surface rainfall–run-off in a watershed dominated by karst geology that otherwise would allow percolation (Xian & Crane, Citation2005). Developed land percent cover showed a significant inverse relationship with both annual and high turbidity periods, as well as high chlorophyll concentration periods. As developed land area increased, turbidity and chlorophyll concentration decreased, especially during the low discharge season. This is consistent with previous research that concluded that increased impervious surfaces associated with developed land cover leads to sediment-free run-off and lower total suspended solid concentrations compared with agricultural watersheds (Estes et al., Citation2009; Miller, Schoonover, et al., Citation2011; Moreno Madriñán et al., Citation2012).
Interannual variation in turbidity and chlorophyll in Tampa Bay were strongly affected by land cover changes, but they are also consistent with the long-term weakening of the wind. The changing turbidity and chlorophyll concentrations are otherwise inconsistent with the lack of a long-term trend in precipitation. Normally, increased precipitation is expected to diminish water quality. In some areas of the bay, such as in MTB, low-turbidity was significantly related to reduction of agricultural land percent cover. Agriculture may modulate water quality through irrigation and changes in fertilizer application practices, and thus a connection with precipitation is not always obvious. Overall, turbidity was most clearly and significantly related to wind stress during the low-turbidity months in HB. Wind stress is also an important driver on very short time scales (i.e. hours; see Chen et al., Citation2010; Demers et al., Citation1987).
Annual, high, and low turbidity periods were significantly positively related to agricultural land percent cover in MTB. Annual and low chlorophyll concentration periods were also significantly related to agricultural land percent cover in HB and the entire upper bay, but these relationships were influenced by outliers. All outliers identified occurred in the 1977 period and appear to be due largely to land cover changes. The period between 1977 and 1996 experienced significant growth and thus may appear as outliers relative to change over the shorter gaps in subsequent land use assessments. In any case, turbidity and chlorophyll in Tampa Bay segments always decreased with decreased agricultural land; similar to what has been found elsewhere (Harding, Young, Hayes, Shearer, & Stark, Citation1999; Schlosser & Karr, Citation2007; Sharpley, Chapra, Wedepohl, & Sims, Citation1994).
Different segments of Tampa Bay have different, localized water-quality drivers. OTB is separated by a peninsula from HB and lacks the large riverine influxes of HB and MTB. OTB is also traversed by three causeways and bridges that impact flushing time (Zhu et al., Citation2014). The only variable assessed here that had an influence on OTB annual or periodic turbidity or chlorophyll concentration was developed land percent cover.
HB is the smallest of the three bay segments, but it has the largest watershed and the highest average turbidity observed over the study period (Table ). The only variable found to be significantly related to turbidity here was wind stress. Higher wind stress led to increased turbidity during low-turbidity periods (Figure (f)). Winds likely led to resuspension of sediments (Demers et al., Citation1987; Madsen et al., Citation1993; Schoen et al., Citation2014; Sheng & Lick, Citation1979). No variables were significantly related to chlorophyll concentration when outliers were removed. HB annual and high turbidity periods were not found to be significantly related to any variable, which may be a reflection of the minimal (0.62%) change in developed land in this watershed from the 1970s to 2010 relative to respective changes in OTB (9.03%) and MTB (3.39%).
The number of sampling observations of this study was limited to the epochs for which land cover maps were available. The analysis spans four decades. One variable, specifically developed land percent cover, was consistently the most strongly and significantly related to water quality (Figure ). Our results indicate that increased developed land percent cover was strongly associated with continued decrease in turbidity and chlorophyll concentration over time. The effect may be due to both increased low-sediment run-off during precipitation events, as well as to reduced inputs of nutrients and sediments due to reduced agricultural land cover. Other studies that have assessed other water-quality parameters (e.g. pollution, nitrate concentration, pH, sulfate concentration) found positive relationships with developed land cover (Burgos-Caraballa, Cantrell, & Ramirez, Citation2014; Coulter, Kolka, & Thompson, Citation2004; Ren et al., Citation2003). Clearly, “water quality” is a complex concept that must be defined appropriately for the research question being assessed. In any case, given the extensive efforts to improve point-source pollution to Tampa Bay, there is likely a dynamic combination of targeted pollution reduction and non-point source land cover change that has contributed to the general water-quality improvement in the bay over the past 40 years. Constraining the contribution of each land cover type is difficult. Greening et al. (Citation2014) point out that the substantial decrease of point source pollution to the bay has led to an increase in the relative contribution of non-point source pollution. Therefore, identifying substantial non-point sources and evaluating their trends and impacts on Tampa Bay are essential for continued water-quality improvement.
Figure 13. Plot of Tampa Bay-wide turbidity with developed land percent of watershed area for each epoch.

Tampa Bay has also undergone substantial changes as a result of concerted efforts to restore wetland and seagrass coverage to historic levels, and reduce point- and non-point-source pollution. Starting in 1992, almost 1,000 individual projects designed to restore seagrass and reduce pollution may have also contributed to improved long-term water-quality conditions (https://apdb.tbeptech.org/). Efforts like these should be considered by managers for their relevance to addressing water-quality issues, but were beyond the scope of this work. There was also a period of substantially elevated turbidity (Figure ) spanning the late1980s to early 1990s that did not coincide with land cover map years and was therefore not evaluated here, but is worth evaluating further in future research. No independent variables appeared to explain this prolonged deviation, which suggests that additional elements not investigated here (e.g. channel-dredging activities) may substantially influence water quality.
5. Conclusions
Land cover and water quality show a relationship in Tampa Bay from scales spanning local river mouths to the entire estuary. The long-term decrease in turbidity and chlorophyll concentration shows a strong negative correlation with developed land percent cover. There was no clear long-term change in precipitation between the 1970s and the 2000s, but the trend in water quality may have also been in part driven by a small decrease in average annual wind speed over the study period.
Changes in agricultural land cover were significantly related to turbidity only for MTB. Improving water quality in this bay segment seems to have been related to the decrease in agricultural land use in the adjacent watershed. Wind stress was significantly positively related to low-turbidity periods in HB (i.e. higher wind stress was correlated with higher turbidity during these generally low-turbidity months). There was no relationship between precipitation and turbidity or chlorophyll concentration over any spatial scale or period. The increase of impervious cover likely led to increased low-nutrient and low-sediment run-off, which helped improve water quality. Future research should focus on higher temporal resolution data and extreme events to identify drivers of short-term water-quality events that may have greater impact on the bay’s overall health as inter-annual water quality continues to improve.
Funding
This work was supported by EPA Star [grant number RD835193010] to FMK. This work was supported by NASA [grant number NNX14AP62A] ‘National Marine Sanctuaries as Sentinel Sites for a Demonstration Marine Biodiversity Observation Network (MBON)’ funded under the National Ocean Partnership Program (NOPP RFP NOAA-NOS-IOOS-2014-2003803 in partnership between NOAA, BOEM, and NASA), and the U.S. Integrated Ocean Observing System (IOOS) Program Office.
Acknowledgments
The study benefitted from discussions with L. Cross and H. Greening from the Tampa Bay National Estuary Program.
Additional information
Notes on contributors
Matthew J. McCarthy
This work constituted one chapter of Dr McCarthy’s dissertation research, which focused on coastal water quality and wetland evaluations. Using existing land cover maps to study the effects of land cover change on long-term water-quality patterns was made possible by remote sensing research. Dr Muller-Karger’s Institute for Marine Remote Sensing specializes in the exploitation of a suite of remote sensing data sources to study marine biodiversity, public health, coral reefs, wetland, water quality and more. The results of this paper were incorporated into additional research that evaluated drivers of water-quality patterns in 11 estuaries throughout the Gulf of Mexico by deriving water-quality data from satellite imagery to fill in gaps and improve the temporal resolution of the data.
References
- Akaike, H. (1973). Information theory and an extension of maximum likelihood principle. In B. N. Petrov & F. Csaki (Eds.), Second international symposium on information theory (pp. 267–281). Budapest: Akademiai Kiado.
- Al-Taani, A. A. (2014). Trend analysis in water quality of Al-Wehda Dam, north of Jordan. Environmental Monitoring and Assessment. doi:10.1007/s10661-014-3850-2
- Anderson, J. R., Hardy, E. E., Roach, J. T., & Witmer, R. E. (1976). A land use and land cover classification system for use with remote sensor data (U.S. Geological Survey Professional Paper 964, p. 28). Retrieved from http://pubs.er.usgs.gov/pubs/pp/pp964
- Assessment report of wetland mapping improvement to NOAA’s Coastal Change Analysis Program (C-CAP) land cover in western Washington State. (2013). State of Washington Department of Ecology. Retrieved April 20, 2014, from http://www.ecy.wa.gov/programs/sea/wetlands/pdf/C-CAPWetlandAssessmentReport.pdf
- Bateni, F., Fakheran, S., & Soffianian, A. (2013). Assessment of land cover changes & water quality changes in the Zayandehroud River Basin between 1997–2008. Environmental Monitoring and Assessment. doi:10.1007/s10661-013-3348-3
- Breiman, L., & Culter, A. (2003). Manual on setting up, using, and understanding Random Forests v4.0 (Technical Report).
- Burgos-Caraballa, S., Cantrell, S. A., & Ramirez, A. (2014). Diversity of benthic biofilms along a land use gradient in tropical headwater streams, Puerto Rico. Microbial Ecology. doi:10.1007/s00248-014-0401-x
- Chen, Z., Hu, C., Conmy, R. N., Muller-Karger, F., & Swarzenski, P. (2007). Colored dissolved organic matter in Tampa Bay, Florida. Marine Chemistry, 104, 98–109.10.1016/j.marchem.2006.12.007
- Chen, Z., Hu, C., & Muller-Karger, F. (2007). Monitoring turbidity in Tampa Bay using MODIS/Aqua 250-m imagery. Remote Sensing of Environment, 109, 207–220.10.1016/j.rse.2006.12.019
- Chen, Z., Hu, C., Muller-Karger, F., & Luther, M. E. (2010). Short-term variability of suspended sediment and phytoplankton in Tampa Bay, Florida: Observations from a coastal oceanographic tower and ocean color satellites. Estuarine, Coastal and Shelf Science, 89, 62–72.10.1016/j.ecss.2010.05.014
- Chen, Z., Muller-Karger, F., & Hu, C. (2007). Remote sensing of water clarity in Tampa Bay. Remote Sensing of Environment, 109, 249–259.10.1016/j.rse.2007.01.002
- Coulter, C. B., Kolka, R. K., & Thompson, J. A. (2004). Water quality in agricultural, urban, and mixed land use watersheds. Journal of the American Water Resources Association, 40(6), 1593–1601.10.1111/jawr.2004.40.issue-6
- Demers, S., Therriault, J., Bourget, E., & Bah, A. (1987). Resuspension in the shallow sublittoral zone of a macrotidal estuarine environment: Wind influence. Limnology and Oceanography, 32(2), 327–339.10.4319/lo.1987.32.2.0327
- Eleveld, M. A., van der Wal, D., & van Kessel, T. (2014). Estuarine suspended particulate matter concentrations from sun-synchronous satellite remote sensing: Tidal and meteorological effects and biases. Remote Sensing of Environment, 143, 204–215.10.1016/j.rse.2013.12.019
- Estes, Jr M. G., Al-Hamdan, M., Thom, R., Quattrochi, D., Woodruff, D., Judd, C., … Johnson, H. (2009, October 26–29). Watershed and hydrodynamic modeling for evaluating the impact of land use change on submerged aquatic vegetation and seagrasses in Mobile Bay. OCEANS IEEE, Biloxi, MS.
- Gilhousen, D. B. (1987). A field evaluation of NDBC moored buoy winds. Journal of Atmospheric and Oceanic Technology, 4, 94–104.10.1175/1520-0426(1987)004<0094:AFEONM>2.0.CO;2
- Greening, H., Janicki, A., Sherwood, E. T., Pribble, R., & Johansson, J. O. R. (2014). Ecosystem responses to long-term nutrient management in an urban estuary: Tampa Bay, Florida, USA. Estuarine, Coastal and Shelf Science, 151, A1–A16.10.1016/j.ecss.2014.10.003
- Hamed, K. H. (2007). Trend detection in hydrologic data: The Mann-Kendall trend test under the scaling hypothesis. Journal of Hydrology, 349, 350–363. doi:10.1016/j.jhydrol.2007.11.009
- Harding, J. S., Young, R. G., Hayes, J. W., Shearer, K. A., & Stark, J. D. (1999). Changes in agricultural intensity and river health along a river continuum. Freshwater Biology, 42, 345–357.10.1046/j.1365-2427.1999.444470.x
- Hu, C., Chen, Z., Clayton, T. D., Swarzenski, P., Brock, J. C., & Muller-Karger, F. (2004). Assessment of estuarine water-quality indicators using MODIS medium-resolution bands: Initial results from Tampa Bay, FL. Remote Sensing of Environment, 93, 423–441.10.1016/j.rse.2004.08.007
- Janicki, A., Pribble, R., Janicki, S., & Winowitch, M. (2001). An analysis of long-term trends in Tampa Bay water quality (Technical Report). Retrieved August 2, 2014, from http://www.tbeptech.org/TBEP_TECH_PUBS/2001/TBEP_04_01WQTrends.pdf
- Jordan, Y. C., Ghulam, A., & Herrmann, R. B. (2012). Floodplain ecosystem response to climate variability and land-cover and land-use change in Lower Missouri River basin. Landscape Ecology, 27, 843–857.10.1007/s10980-012-9748-x
- Kuo, Y. M., Chu, H. J., Pan, T. Y., & Yu, H. L. (2011). Investigating common trends of annual maximum rainfalls during heavy rainfall events in southern Taiwan. Journal of Hydrology, 409, 749–758. 10.1016/j.jhydrol.2011.09.015
- Le, C., Hu, C., English, D., Cannizzaro, J., & Kovach, C. (2013). Climate-driven chlorophyll-a changes in a turbid estuary: Observations from satellites and implications for management. Remote Sensing of Environment, 130, 11–24.10.1016/j.rse.2012.11.011
- Madsen, O. S., Wright, L. D., Boon, J. D., & Chisholm, T. A. (1993). Wind stress, bed roughness and sediment resuspension on the inner shelf during an extreme storm event. Continental Shelf Research, 13(11), 1303–1324.10.1016/0278-4343(93)90054-2
- Mei, K., Liao, L., Zhu, Y., Lu, P., Wang, Z., Dahlgren, R. A., & Zhang, M. (2014). Evaluation of spatial-temporal variations and trends in surface water quality across a rural-suburban-urban interface. Environmental Science and Pollution Research. doi:10.1007/s11356-014-2716-z
- Méndez-Lázaro, P. A., Nieves-Santiango, A., & Miranda-Bermúdez, J. (2014). Trends in total rainfall, heavy rain events, and number of dry days in San Juan, Puerto Rico, 1955–2009. Ecology and Society, 19. 10.5751/ES-06464-190250
- Méndez-Lázaro, P., Muller-Karger, F. E., Otis, D., McCarthy, M. J., & Peña-Orellana, M. (2014). Assessing climate variability effects on dengue incidence in San Juan, Puerto Rico. International Journal of Environmental Research and Public Health, 11, 9409–9428.10.3390/ijerph110909409
- Miller, J. D., Schoonover, J. E., Williard, K. W. J., & Hwang, C. R. (2011). Whole catchment land cover effects on water quality in the Lower Kaskaskia River watershed. Water, Air & Soil Pollution, 221, 337–350.10.1007/s11270-011-0794-9
- Miller, R. L., Liu, C., Buonassissi, C. J., & Wu, A. (2011). A multi-sensor approach to examining the distribution of total suspended matter (TSM) in the Albemarle-Pamlico estuarine system, NC, USA. Remote Sensing, 3, 962–974.10.3390/rs3050962
- Miller, R. L., & McKee, B. A. (2004). Using MODIS Terra 250 m imagery to map concentrations of total suspended matter in coastal waters. Remote Sensing of Environment, 93, 259–266.10.1016/j.rse.2004.07.012
- Moreno Madriñán, M. J., Al-Hamdan, M. Z., Rickman, D. L., & Ye, J. (2012). Relationship between watershed land-cover/land-use change and water turbidity status of Tampa Bay major tributaries, Florida, USA. Water, Air & Soil Pollution, 223, 2093–2109.10.1007/s11270-011-1007-2
- Nelson, E. J., & Booth, D. B. (2002). Sediment sources in an urbanizing, mixed land-use watershed. Journal of Hydrology, 264, 51–68.10.1016/S0022-1694(02)00059-8
- Price, C. V., Nakagaki, N., Hitt, K. J., & Clawges, R. M. (2007). Enhanced historical land-use and land-cover data sets of the U.S. Geological Survey. U.S. Department of the Interior. U.S. Geological Survey. Data Series 240. Retrieved from http://pubs.usgs.gov/ds/2006/240/
- Rains, M., Landry, S., Seidel, V., Crisman, T. (2012). Prioritizing habitat restoration goals in the Tampa Bay watershed (Technical report to the Tampa Bay Estuary Program # 10–12). Retrieved April 26, 2014, from http://www.tbeptech.org/TBEP_TECH_PUBS/2012/TBEP_10_12_USF_Prioritizing_Habitat_Restoration_Goals_2012_04.pdf
- Ren, W., Zhong, Y., Meligrana, J., Anderson, B., Watt, W. E., Chen, J., & Leung, H. (2003). Urbanization, land use, and water quality in Shanghai 1947–1996. Environment International, 29, 649–659.10.1016/S0160-4120(03)00051-5
- Schlosser, I. J., & Karr, J. R. (2007). Water quality in agricultural watersheds: Impact of riparian vegetation during base flow. Journal of the American Water Resources Association, 17(2), 233–240.
- Schmidt, N., Luther, M. E., & Johns, R. (2004). Climate variability and estuarine water resources: A case study from Tampa Bay, Florida. Coastal Management, 32, 101–116.10.1080/08920750490275895
- Schoen, J., Stretch, D., & Tirok, K. (2014). Wind-driven circulation patterns in a shallow estuarine lake: St Lucia, South Africa. Estuarine, Coastal and Shelf Science. doi:10.1016/j.ecss.2014.05.007
- Schoonard, C. M., Collins, J., Paxton, C., & Meindl, C. (2014). Explaining the spatial variability of summer rainfall in Pinellas County, Florida using ArcGIS. Physical Geography. 10.1080/02723646.2014.895893
- Sharpley, A. N., Chapra, S. C., Wedepohl, R., & Sims, J. T. (1994). Managing agricultural phosphorous for protection of surface waters: Issues and options. Journal of Environment Quality, 23, 437–451.10.2134/jeq1994.00472425002300030006x
- Sheeder, S. A., & Evans, B. M. (2004). Estimating nutrient and sediment threshold criteria for biological impairment in Pennsylvania watersheds. Journal of the American Water Resources Association, 40, 881–888.10.1111/jawr.2004.40.issue-4
- Sheng, Y. P., & Lick, W. (1979). The transport and resuspension of sediments in a shallow lake. Journal of Geophysical Research, 84(C4), 1809–1826. doi:10.1029/JC084iC04p01809
- US Census. (2007, April 5). US Census Bureau, annual estimates of the population of metropolitan and micropolitan statistical areas: April 1, 1990 to July 1, 2003. Retrieved September 26, 2014, from http://www.census.gov/prod/2005pubs/p25-1134.pdf
- van den Wollenberg, A. L. (1977). Redundancy analysis: An alternative for canonical correlation analysis. Psychometrika, 42(2), 207–219.10.1007/BF02294050
- Wickham, J. D., Riitters, K. H., Wade, T. G., & Jones, K. B. (2005). Evaluating the relative roles of ecological regions and land-cover composition for guiding establishment of nutrient criteria. Landscape Ecology, 20, 791–798.10.1007/s10980-005-0067-3
- Wu, J. (1969). Froude number scaling of wind-stress coefficients. Journal of the Atmospheric Sciences, 26, 408–413.10.1175/1520-0469(1969)026<0408:FNSOWS>2.0.CO;2
- Xian, G., & Crane, M. (2005). Assessments of urban growth in the Tampa Bay watershed using remote sensing data. Remote Sensing of Environment, 97, 203–215.10.1016/j.rse.2005.04.017
- Xu, Z., Liu, Z., Fu, G., & Chen, Y. (2010). Trends of major hydroclimatic variables in the Tarim River basin during the past 50 years. Journal of Arid Environments, 74, 256–267. 10.1016/j.jaridenv.2009.08.014
- Yamaoka, K., Nakagawa, T., & Uno, T. (1978). Application of Akaike’s Information Criteria (AIC) in the evaluation of linear pharmacokinetic equations. Journal of Pharmacokinetics and Biopharmaceutics, 6(2), 165–175.10.1007/BF01117450
- Yin, Z., Walcott, S., Kaplan, B., Cao, J., Lin, W., Chen, M., … Ning, Y. (2005). An analysis of the relationship between spatial patterns of water quality and urban development in Shanghai, China. Computers, Environment and Urban Systems, 29, 197–221.10.1016/j.compenvurbsys.2003.10.001
- Yue, S., Pilon, P., & Cavadias, G. (2002). Power of the Mann-Kendall and Spearman’s rho tests for detecting monotonic trends in hydrological series. Journal of Hydrology, 259, 254–271.10.1016/S0022-1694(01)00594-7
- Zhu, J., Weisberg, R. H., Zheng, L., & Han, S. (2014). On the flushing of Tampa Bay. Estuaries and Coasts. doi:10.1007/s12237-014-9793-6