ABSTRACT
Gait analysis and physical clinical measures are usually performed in children with cerebral palsy to help the surgeons make therapeutic decision. However, the level of physical activity in daily life is not systematically assessed. The aim of this cross sectional study was to examine the correlations between: three-dimensional gait analysis kinematic and spatiotemporal parameters, clinical measures and physical activity. Participants were 30 children with cerebral palsy (10–18 y), with GMFCS I–III. Daily physical activity was measured with an Actigraph GT3X accelerometer in free living environment during seven consecutive days. The percent of time spent in sedentary, in moderate to vigorous physical activity and the number of steps per day were computed from the accelerometer data. Kinematics parameters did not correlate with physical activity. Moderate correlations were found between spatio-temporal parameters and physical activity, for instance timing of toe-off (r = −0.40, p = 0.03). Few physical examination parameters were correlated with physical activity, such as the hip flexors selective motor control (r = 0.69 with moderate to vigorous activity and r = 0.70 with steps per day, p < 0.05). The physical activity profile cannot be sufficiently determined by a combination of clinical measures.
Introduction
Cerebral palsy (CP), which is the largest cause of motor disability in childhood (Sellier et al. Citation2016), describes a group of permanent disorders of the development of movement and posture, causing activity limitation, that are attributed to non-progressive disturbances to the developing foetal or infant brain (Rosenbaum et al. Citation2007). Motor disorders of CP are often accompanied by secondary musculoskeletal problems, such as reduced joint range of motion, increased joint stiffness and muscle weakness. These secondary alterations progress with age and contribute to a gradual loss of functional capacity, characterised by deterioration in gait and reduced muscular strength throughout adolescence and young adulthood in individuals with CP (Bleasdale Citation1984; Johnson et al. Citation1997).
In the context of planning treatments (include single-event multi-level surgery), a specific evaluation of walking is performed preoperatively using a three-dimensional gait analysis, to assist in surgical decision-making (Gage Citation1983). Gait analysis provides information on the kinematic, kinetic and spatio-temporal parameters of walking. A multivariate measure of overall gait pathology, such as Gait Deviation Index (GDI), a kinematic based index of overall gait pathology, have been developed to facilitate the interpretation of quantitative results (Schwartz and Rozumalski Citation2008). In parallel with this instrumented examination, the clinician performs a neuro-orthopaedic assessment (i.e. clinical measures) measuring passive joint range of motion, structural deformations, muscular strength, spasticity and selective motor control. Multiple parameters are evaluated by these two exams.
In addition to the motor disorders, it appears that children with CP practice less physical activity than typically developing children (TD) (Bjornson et al. Citation2007; Maher et al. Citation2007; Zwier et al. Citation2010; Ryan et al. Citation2015). PA is defined by any bodily movement produced by skeletal muscle that results in energy expenditure (Caspersen et al. Citation1985). Lack of physical activity may contribute to the development of chronic pain, obesity, fatigue and osteoporosis. For children and teenagers, physical activity includes play, sports, travel, daily chores, recreational activities, physical education or planned exercise, in the family, school or community (World Health Organization Citation2010). Participation in exercise and in physical activity, for children with CP, contributes to maintain health and fitness, and also it is essential to physical development. (Fowler et al. Citation2007) The level of daily physical activity may be recorded with an accelerometer that has been validated as an objective measure of ambulatory physical activity in children and adolescents with CP (Capio et al. Citation2010; Clanchy et al. Citation2011; Gorter et al. Citation2012). The parameters commonly recorded are the total step count per day and the percentage of time spent in moderate to vigorous physical activity (MVPA) and in sedentary (SED). This registration is made daily, for 7 days (Ishikawa et al. Citation2013).
If one of the aims of the treatments is to improve activity and participation, including physical activity level, their specific assessments are not systematically done. One of the reasons for this lack of evaluation could be that assessing physical activity is time consuming. Our hypothesis is that clinicians assume that gait analysis and clinical examination are sufficiently relevant and are correlated to the physical activity level of their patient. They could also think that a patient who walk better may have higher PA and conversely.
But, the question of the relationship between the physical activity of children with CP and the clinical examination and the gait analysis remains open. Evaluating specifically physical activity level in children with CP is therefore critical. Its interest is twofold: firstly, the objective assessment of PA provides better information about global child’s health (impairments of body, activity limitations and participation restriction) and could improve the clinical diagnosis; second, it could help clinicians to propose better treatments and to optimally care for these children and youth.
Consequently, the aim of this cross sectional study was to examine the correlations between: three-dimensional gait analysis, clinical measures and physical activity and to evaluate the combined correlated value of clinical measures on the physical activity profile of children with CP.
Recently, Wilson et al. (Wilson et al. Citation2015) showed that there is a correlation between GDI and moderate physical activity (r = 0.47) and a high physical activity (r = 0.51). They also demonstrated a high correlation between GDI and the average steps per day (r = 0.58). In their study, Wilson et al. did not assess the correlations with a set of kinematic parameters nor with clinical examination data.
Considering the multi-dimensional nature of physical activity, it is likely that physical activity does not depend solely on the intrinsic parameters of the subject. As a result, the hypotheses of the study are that there are no correlations between the parameters of the gait analysis, the clinical assessment and physical activity, and that there are no relationships between physical activity, gait analysis and clinical assessment variables.
Method
Participants
Inclusion criteria were: ambulatory and community living children with CP, aged 10–18 years, who functioned at Gross Motor Function Classification System I–III, and who were undergoing a clinically indicated three-dimensional gait analysis for future treatment (including single event multi-level surgery or botulinum neurotoxin treatment). Children were not included if they had significant illnesses, injury or surgery within the last 18 months which may have impacted on usual activity levels in the community, and if they were unable to complete gait analysis because of cognitive deficit or behaviour disorder. Detailed information on the process of recruiting study participants is exposed in the flow diagram (). Written consent was obtained from each child’s parent or guardian and assent from each child. The study was approved by the French independent ethic committee (N°19071004), and by the French National Agency regulating Data Protection (N°1997047V0). Data collection took place between January to August 2016.
Procedure
For this exploratory cross-sectional study, each participant wore the accelerometer Actigraph GT3X® in free-living situation to record daily physical activity. They were also examined by three-dimensional gait analysis followed by standardized clinical examination in the Foundation Ellen Poidatz gait lab. All explicative variables are summarized in Appendix 1.
Accelerometer
The Actigraph® captures and records human activity information using a solid state three-axis accelerometer. Acceleration data from all participants were collected with a 100-Hz sampling frequency. The output recorded by the Actigraph® (counts per minutes) is converted into units of PA intensity using specific algorithm (Freedson et al. Citation2005). The parameters used in this study were percentage spent in Sedentary, in moderate to vigorous physical activity, and step per day. ‘MVPA’ is used when referring to the amount of time a patient spends above a ‘Moderate’ cut point level, thus indicating significant activity, and it is a category of activity intensity that has been consistently shown to reduce the risk of many chronic disease states (Hajna et al. Citation2017). A Freedson’s children equation was used to translate and interpret the accelerometer signal, in Actilife® software. Freedson cut-off point is acceptable to estimate the time spent in MVPA and in SED (Freedson et al. Citation2005; Trost et al. Citation2006). Children wore the accelerometer around the waist for seven days (Ishikawa et al. Citation2013), holidays excluded for all children. Recording was not made simultaneous between children. During the week recorded by the accelerometer, parents or children had to complete a daily diary. They described hour by hour, all activities and sports they practiced. A wear-time validation was made using Actilife software, by applying an automatic filter which remove all period with zero acceleration signals. After, a manual systematic screening of the data was completed between data recorded by the accelerometers and the activities reported in the diary in order to optimize the wear-time validation (). For example, if the child wrote that he or she spent the day at an amusement park, the day was manually deleted as this could skew the results.
Figure 2. Example of daily diary, steps and intensity of physical activity (PA) for one child on one day. A previous verification was made between daily diary and data recorded by accelerometer to validate the wear time. We noticed high level of PA during break time and sport activities, paradoxically moderate to vigorous activity is recorded during videogame, dinner and TV at home. The daily diary was completed by the patient, and it does not discriminate the activities (maybe the child played with active videogame, and they did not specify). Globally, this child spent 18% of his week in MVPA and he made an average of 7187 steps per day
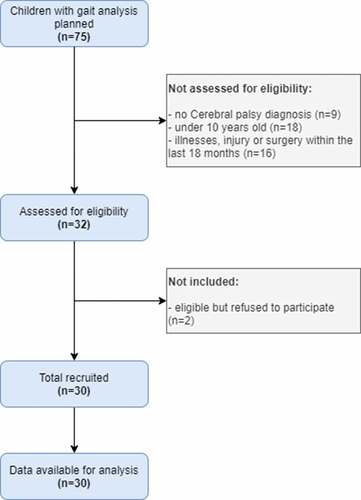
Gait analysis
Kinematic measurements were collected using a fifteen-camera VICON system (8 MX 20, 5 T 40, 2 T 160) (PluginGait marker set, VICON, Oxford Metrics, UK), and four KISTLER force plates (Kistler Holding AG). The subject was asked to walk at comfortable speed down the 10 m walkway. Data were collected for three successful trials by specialized technician. A set of 54 clinically relevant variables were selected a priori from kinematic and spatio-temporal parameters (Benedetti et al. Citation1998; Desloovere et al. Citation2006). The Gait Deviation Index, index of impaired walk, was calculated. (Schwartz and Rozumalski Citation2008). The GDI is based on the calculation of the distance between the patient’s data and the average from the gait lab reference dataset on 15 gait features of kinematics of the pelvis, hip, knee and ankle. This dataset included 1078 children with CP, and is matched on age with individuals of this study. The more impaired lower limb, having the lower GDI, was considered for the statistical analysis.
Clinical examination
Neuro-orthopaedic examination was standardized: joint range of motion, strength, spasticity and selective control motor of hip, knee and ankle’s muscles were described by 28 variables from literature review and clinical experience (Gage et al. Citation2009; Avers and Brown Citation2013; Viehweger et al. Citation2014). Physical examination was conducted by physiotherapists in accordance with a fully considered detailed protocol, which was writing prior in the Rehabilitation centre.
Statistical analysis
The statistical analyses were performed with RStudio version 3.3.3. For the three-dimensional gait analysis and the physical examination, only the more affected lower limb was considered for the final analysis (Sangeux et al. Citation2013). The level of association between the kinematics, the spatio-temporal parameters, the physical examination measurements, the average daily step count and the levels of activity were tested with a Spearman’s rank correlation coefficient (r). A Spearman test uses a non-parametric approach and thus does not require the assumption of a normal distribution of observations. The correlations were poor if r < 0.20; fair if 0.21 < r < 0.40; moderate if 0.41 < r < 0.60; good if 0.61 < r < 0.80; very good 0.81 < r < 1.00 (Altman Citation1990).
A series of multiple linear regression analyses was made to correlate MVPA, SED and steps per day of children from gait analysis and clinical parameters, after Bonferroni’s corrections. The physical activity data were entered as the dependent variables and the clinical measurements and gait analysis data were used as the independent variables. After splitting the dataset into test set and training set (ratio 80/20), the backward elimination approach was used to select the optimal model with correlated variables. The coefficient of determination R-squared (R2), percentage of variance explained by the independent variables to determine a dependant variable, was calculated and adjusted. The standard error of the regression (S) was used to estimate the precision of the model, the threshold considered was S < 2.5 to produce a sufficiently narrow 95% interval. Predictive R-square (Predi-R2) was calculated, to tell how well a regression model determines responses for new observations. A pre-selection was made, because the number of participants was not enough to include all variables in the initial model. For MVPA, SED and steps per day, all independent variables that respect: p < 0.2 and r > 0.5 were included. The initial model evaluated the correlated value of 12 clinical measurements (hip extension, hip abduction evaluated with hip and knee flexed, hip abduction evaluated with hip and knee extended, hip external rotation, bilateral popliteal angle, ankle dorsiflexion evaluated with knee at 0°, femoral anteversion, tibio-femoral angle, Duncan Ely score and strength of hip abductors and ankle dorsi- and plantar flexors) and 9 spatio-temporal and kinematics parameters from gait analysis (step length, timing of toe-off, gait velocity, pelvic range of sagittal motion, hip flexion angle at terminal stance, hip rotation angle at toe-off, hip range of sagittal motion in stance, hip flexion velocity in swing, ankle maximum dorsiflexion in stance phase).
In subgroup analyses, children with a Global Motor Function Classification System II/III were grouped together to obtain consistent group sizes. Student tests were performed to compare MVPA, sedentary time and steps per day averages between children with a GMFCS I versus children with a GMFCS II/III.
A significance threshold of 0.05 was adopted for all statistical analyses.
Results
Thirty patients were included in the final analysis. There is no missing data and no patients were excluded. There were 17 children with GMFCS I, 7 with GMFCS II and 6 with GMFCS III. There were 11 patients with hemiplegia and 19 with diplegia. For the 30 participants with seven full days of accelerometer data, the median daily step count was 5871 (range 1781–12551). Mean percent of time spent in MVPA was 14.6% over 10 hours (range 4.4%-30.8%), in other words 88 minutes per day (range 31–222 minutes per day). Mean percent of time spent in SED was 76.2% over 10 hours (range 52.1%-90.1%), in other words 457 minutes per day (range 313–541 minutes per day), more than 7 hours a day. Children with a GMFCS I practiced more daily physical activity (MVPA and steps per day) than children with a GMFCS II–III. Mean MVPA for children with a GMFCS I was 96.13 minutes (SD 35.55), whereas for children with a GMFCS II/III it was 74.02 minutes (SD 32.09). Mean SED for children with a GMFCS I was 449.83 (SD 50.94), mean SED for children with a GMFCS II/III was 469.75 minutes (SD 32.09). The median steps per day for children with a GMFCS I over 10 hours was 6385 (±2329) whereas for children with a GMFCS II/III was 5030 (± 3042).
Detailed information is presented in . Daily diary of patients showed that only one third of children have claimed that they have practiced sports or exercise. In this study, the sports or activities that the participants declared were: dance, gymnastics, cycling, swimming, horse riding, playing games, wheelchair basketball but the essential of the physical activity recorded is induced by locomotion and school playtime.
Table 1. Descriptive characteristics of the participants by Gross Motor Classification Scale (GMFCS)
The mean GDI in total population was 68.5 (range 46.2–93.2). There was no correlation between the GDI and SED (r = −0.11, p = 0.55), MVPA (r = 0.24, p = 0.21) or steps per day (r = 0.33, p = 0.07).
None of the kinematic parameters were correlated with physical activity. Fair to moderate correlations were found between spatio-temporal parameters and physical activity. Timing of toe-off (r = −0.40, p < 0.03) correlated with MVPA. Walking-speed did not correlate with physical activity (rMVPA = 0.13, rSED = −0.06, rSteps = 0.20, p > 0.05). Few clinical measurements of range of motion, strength and selective motor control were correlated with physical activity. Hip range of motion, strength and selective motor control were correlated with physical activity, such as hip flexor selective motor control (r = 0.69 with MVPA and r = 0.69 with steps per day), maximal hip flexion (r = 0.43), maximal hip rotation (r = 0.45), maximal hip abduction (r = 0.52) with MVPA only. The correlations founded are summarized in .
Table 2. Correlation coefficients between gait analysis and time spent in MVPA, sedentary and steps per day
Table 3. Correlation coefficients between clinical examination and time spent in MVPA, sedentary and steps per day
The results of the multiple linear regression model were:
For MVPA, the correlated variables were: hip extension, hip abduction evaluated with hip and knee extended, hip abduction evaluated with hip and knee flexed, hip external rotation and ankle dorsiflexion evaluated with knee at 0°. The linear regression model was stable (standard error = 0.565). 54% of variation was explained by the model (R2 adjusted = 0.54). Predictive R2 indicated that this model was unable to determine new observations (Predi-R2 = 8.9%).
For SED, the correlated variables were: hip external rotation, ankle dorsiflexion evaluated with knee at 0°, timing of toe-off and ankle maximum dorsiflexion in stance. The linear regression model was stable (standard error = 0.58). 52% of variation was explained by the model (R2 adjusted = 0.52). Predictive R2 indicated that this model was unable to determine new observations (Predi-R2 = 9.2%).
For steps per day, the correlated variables were: hip external rotation, femoral anteversion, timing of toe-off, hip angle at terminal stance, hip rotation angle at toe-off and hip flexion velocity in swing. The linear regression model was stable (standard error = 0.66). 43% of variation was explained by the model (R2 adjusted = 0.43). Predictive R2 indicated that this model was unable to determine new observations (Predi-R2 = 14.0%).
Discussion
This present study explored the link between the gait analysis parameters, the clinical examination and the physical activity recorded in free-living situations for children with cerebral palsy. The aim of this cross sectional study was to examine the correlations between: three-dimensional gait analysis, clinical measurements and physical activity.
The physical activity of children with CP is well documented, they practice less physical activity than typically developing children ones (Bjornson et al. Citation2007; Maher et al. Citation2007; Zwier et al. Citation2010; Ryan et al. Citation2015). The results of this study confirmed this trend. For the 30 participants, the median daily step count was 5582, which was far from the typical 13460 steps per day taken by young people without impairment (Bjornson et al. Citation2007). Nowadays, the recommendation for optimal health is 12,000 steps/day (U.S. Department of Health and Human Services Citation2018). Among the studied population, 27 respected the time spent in MVPA fixed by the US federal guidelines for youth, more than 60 minutes a day. Concurrent validity studies utilizing heart rate monitoring, direct observation, indirect calorimetry, whole room calorimetry and doubly labelled water have demonstrated the Actigraph® to be a valid measure of physical activity in TD children and adolescent (Krishnaveni et al. Citation2009; Crouter et al. Citation2013; Romanzini et al. Citation2014; De Craemer et al. Citation2015; Johansson et al. Citation2016; Meltzer et al. Citation2016; Migueles et al. Citation2017), and in youth with CP (Capio et al. Citation2010; Clanchy et al. Citation2011; Gorter et al. Citation2012; O’Neil et al. Citation2014; Oftedal et al. Citation2014). Clanchy et al. showed that the Freedson’s algorithm is sufficiently sensitive (81,8%), specific (67,5%) and accurate (74,6%) for MVPA threshold in this population (Clanchy et al. Citation2011). However, Gaba et al. showed that, out of a population of 306 healthy children, differences in the level of MVPA, varied from 27 min/day (Puyau algorithm Citation2002) to 231 min/day (Freedson algorithm Citation2005) (Gába et al. Citation2016).
Regarding their daily diary, only one third of children have claimed that they have practiced sports or exercise. Personal barriers to explain the lack of exercises were related to physical abilities (lake of energy, fatigue, pain, fear of an injury, difficulty to learn required motor skill) or related to psychological factors (decreased motivation, feeling like an outsider, insecure or ashamed, cognitive impairment, not to be accepted by the peers) (Verschuren et al. Citation2012).
Children with GMFCS I practiced significantly more daily physical activity than GMFCS II–III (Van Wely et al. Citation2012; Bjornson et al. Citation2014; Balemans et al. Citation2015). Our results as well as the daily diary data collected confirm this.
In this study, if few spatio-temporal and clinical examination parameters correlated with intensity and quantity of physical activity, the links found were not enough to strongly correlate these parameters (R2 Є [0.43–0.54]; moderate R2 for each model). The correlated variables selected for the multiple regression analysis were based on previous paper on the impact of gait analysis and clinical examination parameters on gait impairments in children with CP (Desloovere et al. Citation2006; Gage et al. Citation2009).
Moderate correlations were found between the hip range of motion, strength and selectivity and MVPA (0.39 < r < 0.52). Kinematics and spatio-temporal parameters did not correlate with the physical activity. Furthermore, the GDI, a multivariate measure of gait abnormality, did not correlate with the number of steps per days, or with the time spent in sedentary activity or in MVPA.
Wilson et al. shown there was a correlation between the GDI and percentage spent in moderate intensity of physical activity (r = 0.47), the GDI and high physical activity (r = 0.51) and the GDI and the average steps per day (r = 0.58) (Wilson et al. Citation2015). Their results were not confirmed in this study. The methodological differences could explain that. First, inclusion criteria were not the same (GMFCS I–III included in our final analysis). Second, the tool used (Actigraph GT3X versus Step Watch Activity Monitor) was not worn during the same period (7 days vs. 2 days for Wilson et al.). Lastly, we considered the more impaired lower limb for the statistical analysis, whereas Wilson et al. used the mean GDI of both lower limbs. In our opinion, we considered that the more impaired lower limb may be the main barrier in practicing physical activity.
According to the results, it could be hypothesised that improving the gait quality may not systematically improve the level of physical activity. Reciprocally, abnormal quality of walking may not be a personal barrier to practice a physical activity.
Furthermore, the data recorded on gait abnormality in the laboratory, and the data collected by accelerometer in free-living situation should be considered as complementary.
Although this was an exploratory study, unable to determine a causal link between the quality of walking and the quantity/intensity of physical activity, this was a first approach to explore this relation. It appeared that the parameters of clinical examination and gait analysis were not sufficiently correlated to physical activity. Specific evaluation of physical activity appeared essential to complete the follow-up of children with CP. Furthermore, the study of physical activity in typical children showed that there were large disparities in the practice of physical activity, even though they were not affected by walking disturbances. It was important to see, however, whether it was the same in children with CP. Other studies could be carried out before-after treatment to study if there is a causal relation between an improvement in walking quality and physical activity.
Study limitations
The analysis of more impaired lower limb was a potential limitation, because of the heterogeneity of population, participants with both unilateral and bilateral CP were included.
The validity of measures in sedentary activity with accelerometer is recently discussed, and more research is needed to validate this tool in children with CP (Innes and Darrah Citation2013). All included children were undergoing a clinically indicated three-dimensional gait analysis for future treatment (including single event multi-level surgery or botulinum neurotoxin treatment). It could be a bias avoiding generalization of the results. This study is an exploratory study where multiple parameters were observed in a specific population. This hypothesis-generating approach, could be biased, but it was chosen as the first step in order to understand the relationship between physical activity and clinical parameters.
Conclusion
The correlation between kinematics, spatio-temporal parameters and clinical examination parameters are low to moderate.
Gait analysis and clinical examination could only partially reflect the overall level and quantity of daily physical activity. None of the tested models from three-dimensional gait analysis and clinical examination strongly determines physical activity. Regarding the weakness of the observed correlations, it is recommended to associate gait analysis and actimetry in the longitudinal follow-up of patients with CP. Futhermore, if the benefits of treatments such as Single Event Multi Level Surgery have already been demonstrated concerning gait, it would be interesting to test its effects on physical activity on children with CP.
Acknowledgements
We thank the study participants, the members of the movement analysis unit of the Ellen Poidatz Foundation and the Société d’Etudes et de Soins pour les Enfants Paralysés et Polymalformés.
Disclosure statement
No potential conflict of interest was reported by the authors.
Correction Statement
This article has been republished with minor changes. These changes do not impact the academic content of the article.
References
- Altman D. 1990. Practical statistics for medical research. New York: Chapman and Hall/CRC. ISBN: 9780412276309.
- Avers D, Brown M. 2013. Daniels and Worthingham’s muscle testing: techniques of manual examination and performance testing. Missouri: Elsevier; p. 10e.
- Balemans ACJ, van Wely L, Becher JG, Dallmeijer AJ. 2015. Longitudinal relationship among physical fitness, walking-related physical activity, and fatigue in children with cerebral palsy. Phys Ther. 95:996–1005. doi:10.2522/ptj.20140270.
- Benedetti MG, Catani F, Leardini A. 1998. Data management in gait analysis for clinical applications. Clin Biomech. 13:204–215. doi:10.1016/S0268-0033(97)00041-7.
- Bjornson KF, Belza B, Kartin D, Logsdon R, McLaughlin JF. 2007. Ambulatory physical activity performance in youth with cerebral palsy and youth who are developing typically. Phys Ther. 87:248. doi:10.2522/ptj.20060157.
- Bjornson KF, Zhou C, Stevenson R, Christakis D, Song K. 2014. Walking activity patterns in youth with cerebral palsy and youth developing typically. Disabil Rehabil. 36:1279–1284. doi:10.3109/09638288.2013.845254.
- Bleasdale HNO. 1984. Bar-Or “Pediatric sports medicine for the practitioner”. Br J Sports Med. 18:206. doi:10.1136/bjsm.18.3.206.
- Capio CM, Sit CH, Abernethy B. 2010. Physical activity measurement using MTI (Actigraph) among children with cerebral palsy. Arch Phys Med Rehabil. 91:1283–1290. doi:10.1016/j.apmr.2010.04.026.
- Caspersen CJ, Powell KE, Christenson GM. 1985. Physical activity, exercise, and physical fitness: definitions and distinctions for health-related research. Public Health Rep. 100:126.
- Clanchy KM, Tweedy SM, Boyd RN, Trost SG. 2011. Validity of accelerometry in ambulatory children and adolescents with cerebral palsy. Eur J Appl Physiol. 111(12):2951–2959. doi:10.1007/s00421-011-1915-2.
- Crouter SE, Horton M, Bassett DR. 2013. Validity of ActiGraph child-specific equations during various physical activities. Med Sci Sports Exerc. 45:1403–1409. doi:10.1249/MSS.0b013e318285f03b.
- De Craemer M, De Decker E, Santos-Lozano A, Verloigne M, De Bourdeaudhuij I, Deforche B, Cardon G. 2015. Validity of the Omron pedometer and the actigraph step count function in preschoolers. J Sci Med Sport. 18:289–293. doi:10.1016/j.jsams.2014.06.001.
- Desloovere K, Molenaers G, Feys H, Huenaerts C, Callewaert B, Walle PVD. 2006. Do dynamic and static clinical measurements correlate with gait analysis parameters in children with cerebral palsy? Gait Posture. 24:302–313. doi:10.1016/j.gaitpost.2005.10.008.
- Fowler EG, Kolobe TH, Damiano DL, Thorpe DE, Morgan DW, Brunstrom JE, Coster WJ, Henderson RC, Pitetti KH, Rimmer JH, et al. 2007. Promotion of physical fitness and prevention of secondary conditions for children with cerebral palsy: section on pediatrics research summit proceedings. Phys Ther. 87(11):1495–1510. doi:10.2522/ptj.20060116.
- Freedson P, Pober D, Janz K. 2005. Calibration of accelerometer output for children. Medicine & Science in Sports & Exercise. 37:S523–S530.
- Freedson P, Pober D, Janz KF. 2005. Calibration of accelerometer output for children. Med Sci Sports Exerc. 37:S523–S530. doi:10.1249/01.mss.0000185658.28284.ba.
- Gába A, Dygrýn J, Mitáš J, Jakubec L, Frömel K. 2016. Effect of accelerometer cut-off points on the recommended level of physical activity for obesity prevention in children. PLoS ONE. 11:e0164282. doi:10.1371/journal.pone.0164282.
- Gage JR. 1983. Gait analysis for decision-making in cerebral palsy. Bull Hosp Jt Dis Orthop Inst. 43(2):147–163.
- Gage JR, Schwartz MH, Koop SE, Novacheck TF. 2009. The identification and treatment of Gait problems in cerebral palsy. London: MacKeith Press.
- Gorter JW, Noorduyn SG, Obeid J, Timmons BW. 2012. Accelerometry: a feasible method to quantify physical activity in ambulatory and nonambulatory adolescents with cerebral palsy. Int J Pediatr. 2012:1–6. doi:10.1155/2012/329284.
- Hajna S, Ross NA, Dasgupta K. 2017. Steps, moderate-to-vigorous physical activity, and cardiometabolic profiles. Prev Med. doi:10.1016/j.ypmed.2017.11.007.
- Innes J, Darrah J. 2013. Sedentary behavior: implications for children with cerebral palsy. Pediatr Phys Ther Off Publ Sect Pediatr Am Phys Ther Assoc. 25:402–408.
- Ishikawa S, Kang M, Bjornson KF, Song K. 2013. Reliably measuring ambulatory activity levels of children and adolescents with cerebral palsy. Arch Phys Med Rehabil. 94:132–137. doi:10.1016/j.apmr.2012.07.027.
- Johansson E, Larisch L-M, Marcus C, Hagströmer M, Hind K. 2016. Calibration and validation of a wrist- and hip-worn actigraph accelerometer in 4-year-old children. PloS One. 11:e0162436. doi:10.1371/journal.pone.0162436.
- Johnson DC, Damiano DL, Abel MF. 1997. The evolution of gait in childhood and adolescent cerebral palsy. J Pediatr Orthop. 17:392–396. doi:10.1097/01241398-199705000-00022.
- Krishnaveni GV, Veena SR, Kuriyan R, Kishore RP, Wills AK, Nalinakshi M, Kehoe S, Fall CHD, Kurpad AV. 2009. Relationship between physical activity measured using accelerometers and energy expenditure measured using doubly labelled water in Indian children. Eur J Clin Nutr. 63:1313–1319. doi:10.1038/ejcn.2009.95.
- Maher CA, Williams MT, Olds T, Lane AE. 2007. Physical and sedentary activity in adolescents with cerebral palsy. Dev Med Child Neurol. 49:450–457. doi:10.1111/j.1469-8749.2007.00450.x.
- Meltzer LJ, Wong P, Biggs SN, Traylor J, Kim JY, Bhattacharjee R, Narang I, Marcus CL. 2016. Validation of Actigraphy in middle childhood. Sleep. 39:1219–1224. doi:10.5665/sleep.5836.
- Migueles JH, Cadenas-Sanchez C, Ekelund U, Delisle Nyström C, Mora-Gonzalez J, Löf M, Labayen I, Ruiz JR, Ortega FB. 2017. Accelerometer data collection and processing criteria to assess physical activity and other outcomes: a systematic review and practical considerations. Sports Med Auckl NZ. 47:1821–1845. doi:10.1007/s40279-017-0716-0.
- O’Neil ME, Fragala-Pinkham MA, Forman JL, Trost SG. 2014. Measuring reliability and validity of the ActiGraph GT3X accelerometer for children with cerebral palsy: a feasibility study. J Pediatr Rehabil Med. 7:233–240. doi:10.3233/PRM-140292.
- Oftedal S, Bell KL, Davies PSW, Ware RS, Boyd RN. 2014. Validation of accelerometer cut points in toddlers with and without cerebral palsy. Med Sci Sports Exerc. 46:1808–1815. doi:10.1249/MSS.0000000000000299.
- Piercy K, Troiano R, Olson R, et al. 2018. The physical activity guidelines for Americans. JAMA. 320(19):2020-2028. doi:10.1001/jama.2018.14854.
- Puyau MR, Adolph AL, Vohra FA, Butte NF. 2002. Validation and calibration of physical activity monitors in children. Obesity Research. 10(3):150–157.
- Romanzini M, Petroski EL, Ohara D, Dourado AC, Reichert FF. 2014. Calibration of ActiGraph GT3X, Actical and RT3 accelerometers in adolescents. Eur J Sport Sci. 14:91–99. doi:10.1080/17461391.2012.732614.
- Rosenbaum P, Paneth N, Leviton A, Goldstein M, Bax M, Damiano D, Dan B, Jacobsson B. 2007. A report: the definition and classification of cerebral palsy April 2006. Dev Med Child Neurol Suppl. 109:8–14.
- Ryan JM, Forde C, Hussey JM, Gormley J. 2015. Comparison of patterns of physical activity and sedentary behavior between children with cerebral palsy and children with typical development. Phys Ther. 95:1609–1616. doi:10.2522/ptj.20140337.
- Sangeux M, Wolfe R, Graham HK. 2013. One side or two? Dev Med Child Neurol. 55:786–787. doi:10.1111/dmcn.12230.
- Schwartz MH, Rozumalski A. 2008. The gait deviation index: A new comprehensive index of gait pathology. Gait Posture. 28:351–357. doi:10.1016/j.gaitpost.2008.05.001.
- Sellier E, Platt MJ, Andersen GL, Krägeloh-Mann I, De La Cruz J, Cans C. 2016. Decreasing prevalence in cerebral palsy: a multi-site European population-based study, 1980 to 2003. Dev Med Child Neurol. 58(1):85–92. doi:10.1111/dmcn.12865.
- Trost SG, Way R, Okely AD. 2006. Predictive validity of three ActiGraph energy expenditure equations for children. Med Sci Sports Exerc. 38:380–387. doi:10.1249/01.mss.0000183848.25845.e0.
- Van Wely L, Becher JG, Balemans ACJ, Dallmeijer AJ. 2012. Ambulatory activity of children with cerebral palsy: which characteristics are important? Dev Med Child Neurol. 54:436–442. doi:10.1111/j.1469-8749.2012.04251.x.
- Verschuren O, Wiart L, Hermans D, Ketelaar M. 2012. Identification of facilitators and barriers to physical activity in children and adolescents with cerebral palsy. J Pediatr. 161:488–494. doi:10.1016/j.jpeds.2012.02.042.
- Viehweger E, Jouve J-L, Simeoni M-C. 2014. Outcome evaluation in pediatric orthopedics. Orthop Traumatol Surg Res. 100:S113–S123. doi:10.1016/j.otsr.2013.06.012.
- Wilson NC, Signal N, Naude Y, Taylor D, Stott NS. 2015. Gait deviation index correlates with daily step activity in children with cerebral palsy. Arch Phys Med Rehabil. 96:1924–1927. doi:10.1016/j.apmr.2015.05.024.
- World Health Organization. 2010. Global recommendations on physical activity for health.
- Zwier JN, van Schie PEM, Becher JG, Smits D-W, Gorter JW, Dallmeijer AJ. 2010. Physical activity in young children with cerebral palsy. Disabil Rehabil. 32(18):1501–1508. doi:10.3109/09638288.2010.497017.