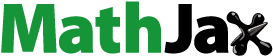
ABSTRACT
The performance of broadcasting networks employing Fountain codes with receiver diversity techniques is investigated in the present work. Particularly, we derive the closed-form expressions of the cumulative distribution function (CDF), the probability mass function (PMF), and the raw moments of the number of the needed time slots to deliver a common message to all users under two diversity schemes, namely, maximal ratio combining (MRC) and selection combining (SC). Numerical results are supplied to verify the accuracy of the considered networks and highlight the behaviours of these metrics as a function of some vital parameters such as the number of receivers, and the number of received antennae. Additionally, we also confirm the advantages of the MRC scheme compared with the SC scheme in the broadcasting networks.
1. Introduction
Improving spectral efficiency (SE) and/or energy efficiency (EE) in the contemporary ultra-dense wireless networks such as, cellular networks (Di Renzo et al., Citation2018; Di Renzo, Zappone, et al., Citation2019; Hossain, Citation2022), low power wide area networks (LPWAN) with LoRa (Nguyen et al., Citation2020; Tu et al., Citation2021; Tu, Bradai, et al., Citation2022), SigFox, and WiFi networks (Feng et al., Citation2022; Ragpot et al., Citation2019), is a challenging task owing to the strong interference. There are many approaches in the literature, for example, relaying networks (Nguyen and Kong, Citation2017; Pham et al., Citation2022), energy harvesting (Nguyen and Kong, Citation2016; N. T. Nguyen et al., Citation2019), full-duplex communications, satellite communications (N. T. Nguyen et al., Citationin press) and unmanned aerial devices (UAV) (Motlagh et al., Citation2021), are proposed to figure out this problem. Nevertheless, these techniques fairly boost up the SE and the EE since it only focuses on point-to-point communications rather than considering either multi-cast or broadcast networks. Broadcasting networks, differently, simultaneously broadcast information to all users instead of transmitting consecutively to all users. As a consequence, ones can theoretically facilitate the SE and the EE of the networks. Nevertheless, it is an extremely difficult task, in reality, to successfully broadcast messages to all users under limited resources (frequency and/or time). The principal reason is that each user suffers from different fading levels and different transmission distances. Consequently, there is a high probability that some users are not able to decode the error-free message. The sender, as a result, needs to re-send these lost packets until all users successfully receive those messages (Hassan et al., Citation2019). The situation gets worse if the number of devices goes without bound. Thus, the broadcasting networks is not attracted many researchers unless the re-transmission problem is figured out. Fortunately, a powerful coding scheme namely Fountain code has been proposed recently which can overcome such issue (Indoonundon & Fowdur, Citation2021). In fact, with Fountain code, the message is divided into many equal-length packets and each packet is encoded by a random generator matrix which ensures that each novel packet is independent of the previous one. On the receiver side, it only requires to collect a sufficient number of packets to decode the message and is uncorrelated with other users. As a result, it overcomes the re-transmission issue. Nevertheless, in the 5G-Advanced era, employing broadcasting networks is insufficient to ensure reliability owing to the strong interference. Therefore, other techniques which significantly facilitate the system diversity are required along with the broadcasting networks to achieve a comprehensive performance. One of the outstanding technologies to boost reliability is to employ the receiver diversity techniques such as maximal ratio combining (MRC) and selection combining (SC) (Tu et al., Citation2018). Under this context, in the present paper, we investigate the performance of the broadcasting networks utilizing Fountain code and receiver diversity techniques.
Before highlighting main contributions and novelties of the paper. Let us review the state-of-the-art of Fountain codes and receiver diversity schemes. Particularly, the performance of Fountain codes was studied extensively in Dang et al. (Citation2019), Danufane and Di Renzo (Citation2021), Li et al. (Citation2020), Lim et al. (Citation2021), Tran et al. (Citation2012), and Tran et al. (Citation2021) while the performance of receiver diversity was given in Arya and Chung (Citation2018), Liao et al. (Citation2018), Phu et al. (Citation2020), and Tsai et al. (Citation2022). Particularly, Dang et al. (Citation2019) derived the outage probability (OP) of the multiple-input multiple-output (MIMO) systems with and without employing non-orthogonal multiple access (NOMA). The results showed that with NOMA, ones can save a significant number of time slots to convey the message compared with non-NOMA systems. They, however, consider only the single-user scenario rather than multi-user cases. Numerical results unveiled that the power splitting protocol is better than the time switching protocol. They, nonetheless, do not take into account the receiver diversity scheme. The comparison between FC and random linear network coding (RLNC) in short packet communications (SPC) was conducted in Li et al. (Citation2020). Their outcomes stated that the SPC FC scheme provides better performance. Particularly, it has a smaller decoding error probability compared with RLNC. Besides, the authors in Lim et al. (Citation2021) proposed a novel Fountain code dedicated to the SPC. The results revealed that their proposed Fountain code attains a lower bit error rate and higher rate compared to the conventional FC scheme. The distribution of the average time slot to deliver a packet in cellular networks was studied in Danufane and Di Renzo (Citation2021) via tools from stochastic geometry (SG) (Al Hajj et al., Citation2020). Duy and others derived the average time slots in multi-hop communications with FCs in the closed-form equation (Tran et al., Citation2012) and the performance of the cooperative cognitive radio networks (CCRNs) employing FCs was investigated in Tran et al. (Citation2021). Authors in Tsai et al. (Citation2022) focussed on the diversity techniques at the receiver. The application of the receiver diversity technique in ultraviolet (UV) communications was studied in Arya and Chung (Citation2018). More precisely, the switch and stay combining (SSC) was applied to improve the outage probability and symbol error probability (SEP). The maximal ratio combining scheme was used in Liao et al. (Citation2018) to improve the coverage area as well as the diversity gain. The performance of transmit antenna selection (TAS) combined with Fountain code was studied in Phu et al. (Citation2020). They, however, consider point-to-point communications and transmit diversity which requires the perfect channel state information at the transmitter (CSIT) that is difficult to obtain in practice.
Different with the above-mentioned works, in the present paper, we study the performance of the broadcasting networks utilizing Fountain code and receiver diversity techniques. Additionally, we consider different metrics the statistics of the number of the needed time slot to broadcast packets to all users rather than the outage probability and/or the average rate as like works in the literature. The main contributions and novelties are summarized as follows:
We derive the cumulative distribution function (CDF), the probability mass function (PMF), the raw moments of the needed time slot to deliver messages to an arbitrary number of end user.
We consider two popular diversity techniques namely, the maximal ratio combining and the selection combining.
We provide simulation results based on Monte-Carlo method to verify the correctness of the considered networks as well as to unveil the impact of some important parameters on the performance of all considered metrics.
The remainder of the manuscript is organized as follows: Section 2 provides the system model. The main derivations and trends are given in Section 3. Numerical results are provided in Section 4. Section 5 concludes the paper.
2. System model
Let us consider a wireless networks where an access point (AP) denoted by A sends a common message to users associated with it as shown in Figure . AP employs Fountain code to encode the message. More precisely, AP splits the message into
equal-length packets and encodes each packet with a random generator matrix. At each time slot, AP broadcasts a novel packet and all users will collect these packets until successfully decode the message. After decoding the message, each user will send back to the AP an acknowledgment (ACK) message via a high accuracy feedback channel (N.-L. Nguyen et al., Citation2023; Tu, Nguyen, et al., Citation2022). The AP stops transmitting once receiving all ACK packets. For simplicity, we assume that the AP only sends a packet per time slot. The AP is equipped with a single antenna while users are equipped with an arbitrary number of antennae. Specifically, the uth user,
, is equipped with
antennae,
is the maximal number of antennae can be installed on the user devices.
2.1. Channel modelling
We consider both large-scale path-loss and small-scale fading in the present work. The influence of shadowing is not taken into consideration since it is a popular case in the literature (Tu & Di Renzo, Citation2020).
2.1.1. Small-scale fading
Let us denote as the channel coefficient from AP to the oth antenna at the uth user at the
time slot, it is modelled by a complex Gaussian random variable (RV) with zero mean and
variance, i.e.
. The channel gain is then followed by an exponential random variable with scale parameter
. In this work, we assume that small-scale fading follows by a flat fading which is unchanged in a time slot and varies independently between time slots (N. T. Nguyen, Tran, Tran, et al., Citation2022).
2.1.2. Large-scale path-loss
Let us denote as the large-scale path-loss from AP to the uth user, it is formulated as follows:
(1)
(1) where
is the path-loss constant,
is the wavelength,
is the speed of light,
is the carrier frequency and
is the Euclidean distance from the AP to the uth user. υ is the path-loss constant and its value relies on the transmission environment, i.e. urban, suburban, rural areas, etc.
Remark 2.1
It is noted that when the transmission distance approaches zero or the distance between transmitter and receiver reaches zero, the adopted path-loss model in (Equation1(1)
(1) ) does not go to infinity. It, as a result, tackles the popular issue of the unbounded path-loss model in the literature (Di Renzo, Tu, et al., Citation2019).
2.2. Receiver diversity
In this work, if the number of antennae at the receiver is greater than 2, it can then utilize diversity techniques such as the maximal ratio combining or selection combining to facilitate the transmission reliability (Tu et al., Citation2018). The former linearly combines the channel gain from all received antenna while the latter simply chooses the antenna having the largest channel gain. As a consequence, theoretically, the MRC scheme always outperforms the SC. From the implementation perspective, the MRC, however, is more challenging since it requires perfect synchronizations between antennae and the adder while the SC solely employs a comparator and a switch to realize this technique. In the present work, we assume that all users can deploy both schemes.
The received signals at the uth receiver and the ω time-slots under the MRC and SC schemes denoted by , are given as follows:
(2)
(2) Here
is the transmit power of the AP, and
is the transmitted packet at ω time slot. We consider the unit power signal, i.e.
and
is the expectation operator.
2.3. Signal-to-noise ratio (SNR)
From (Equation2(2)
(2) ), the signal-to-noise ratio (SNR) at the uth receiver at the ω time slot under the
diversity scheme denoted by
is formulated as follows:
(3)
(3) Here
is the variance of the additive white Gaussian noise (AWGN) and computed as
, Bw (in [Hz]) is the transmission bandwidth, and NF (in [dB]) is the noise figure at the receiver.
is the channel gain at the receiver and counts on the adopted diversity scheme. Particularly, if the MRC scheme is employed,
is computed as follows (Tu et al., Citation2018):
(4)
(4) and if SC is considered,
is rewritten as (Tu et al., Citation2018):
(5)
(5) where
is the maximum function. As mentioned in Section 2.1.1, we consider the scenario where fading changes across time slots. Consequently, the superscript ω in (Equation3
(3)
(3) ) can be omitted and the SNR is rewritten as
(6)
(6)
3. Performance analysis
In this section, we are going to address the performance of the number of the required time slot to dispatch the message to all users. More precisely, we study the cumulative distribution function, the probability mass function, and the raw moments of the number of the needed time slot to deliver the message. Before going to derive these metrics, let us begin with the probability of successfully sending a packet to a user denoted by . For the MRC scheme, it is denoted by
and is computed as follows (N. T. Nguyen, Nguyen, et al., Citation2022):
(7)
(7) where
,
is the expected rate,
is the probability operator,
is the CDF of RV
. To derive
we commence with the definition of the moment generating function (MGF) of
as follows:
(8)
(8) where
is the MGF of the RV
,
is the joint PDF of
RVs; (a) is held by yielding the uncorrelated property between RVs
, (b) is attained by using the MGF of the exponential RV, and the last equation is obtained as the channel gain between antennae is equal, i.e.
. Looking at (Equation8
(8)
(8) ), it is straightforward to realize that it is the MGF function of the Gamma distribution. As a result, the complement cumulative distribution function (CCDF) of
in (Equation7
(7)
(7) ) is rewritten as
(9)
(9) Here
is the Gamma function (Gradshteyn & Ryzhik, Citation2007, Equation 8.310.1) and
is the upper incomplete Gamma function (Gradshteyn & Ryzhik, Citation2007, Equation 350.2).
Next, the with SC technique is defined and is given below (N. T. Nguyen, Nguyen, et al., Citation2022)
(10)
(10) where (a) is attained by substituting the CDF of the RV
.
3.1. CDF of the needed time slot to deliver 
packets
In the considered broadcasting networks with Fountain codes, the number of the required time slot to dispatch a common message to all users is equivalent to the number of the needed time slot of the worst user. Mathematical speaking, the CDF of the needed time slot to deliver packets under the
scheme denoted by
is computed as follows (N.-L. Nguyen et al., Citation2023):
(11)
(11) where
is the CDF of the required time slot of receiving
packets of the uth user under the θ scheme. (a) is held by the independence of receiving packets between users and (b) is held by utilizing the CDF of the negative binomial distribution,
is the binomial coefficient.
3.2. PMF of the needed time slot to deliver 
packets
Having obtained the CDF, the PMF denoted by can be obtained straightforwardly and computed as follows:
(12)
(12)
3.3. Raw moments of the needed time slot to deliver 
packets
Moments are used to describe the characteristic of a distribution. Particularly, the first moment is the expected value of the distribution while the second moment measures how the distribution spreads out around the mean value. The third moment is called ‘Skewness’ which measures the symmetric of the distribution and the fourth moment is ‘kurtosis’ measuring whether the distribution is heavy tails or not. In this section, we address the raw moments of the needed time slot to dispatch the message to all users. The kth raw moments of the needed time slot under the θ diversity scheme denoted by
is calculated as follows
(13)
(13)
Corollary 3.1:
The first raw moment or the expectation of the considered RV can be directly obtained from (Equation13(13)
(13) ) by setting k = 1. Mathematical speaking, the expectation can be computed as
(14)
(14)
Corollary 3.2:
The variance of the needed time slot to broadcast packets to all users denoted by
is computed as follows:
(15)
(15)
4. Numerical results
In this section, numerical results are given to confirm the accuracy of the proposed mathematical framework as well as to unveil the behaviours of these metrics as a function of some key parameters such as the number of received antennae, the number of users, and the number of packets, etc. Without loss of generality, following parameters are employed throughout this section: dB,
MHz,
,
GHz,Footnote1
bits/s/Hz,
dBm,
,
packets,
. We consider 4 users, i.e.
and the number of received antennae of these users are
, respectively. The position of AP is at
[m] and the locations of all users are
,
,
, and
, respectively. Figures and investigate the impact of the expected rate α on the performance of the CDF, the PMF, the expectation, and the variance of the number of the needed time slots to dispatch the message. Generally, we observe a good agreement between the derived mathematical framework and simulation results via the Monte-Carlo method. Figure (a) illustrates that the CDF is a monotonic decrease function regarding to α. This can be explained effortlessly from the definition of the success probability in (Equation9
(9)
(9) ) and (Equation10
(10)
(10) ) that
,
always decreases with α. As a consequence, the CDF in (Equation11
(11)
(11) ) reduces too as it is a product of numbers between zero and one. Additionally, we also see that increasing
will scale up the CDF as it is a monotonic increasing function with
. Besides, it is obvious that the MRC scheme outperforms the SC scheme for example when
, the CDF of the SC scheme is solely under 0.1 for
while the MRC scheme is approximately 0.25. Figure (b) stretches the performance of the PMF versus the expected rate α. We notice that it is a unimodal function with respect to α. We see that the peak of the SC scheme is the left-hand side of the MRC scheme. Moreover, increasing the transmit power will shift the peak towards the right-hand side. Both expectation and variance are monotonically increasing with α as showed in Figure . The explanation is straightforward as the expected rate goes large, it becomes more difficult to receive packets without errors, and as a consequence, the expectation goes up without bound. Regarding the variance, we observe a similar trend as the expectation but it approaches infinity faster owing to the second order.
Figures and study the influence of the transmit power on the performance of all considered metrics. It is certain that raising the transmit power will consistently improve the CDF. Moreover, the larger the transmitted packets the lower the CDF. Similar to the influence of α, the PMF is also a unimodal function with respect to
. We see that the peak of MRC scheme is at the left-hand side of the SC scheme. Additionally, the peak of case
is higher than case
. It can be explained that the probability of successfully delivering 2 packets required
is small compared to delivering 8 packets since it is likely to dispatch 2 packets with smaller
.
Figure 2. Solid and dash-dot lines are plotted by employing (Equation11(11)
(11) ), (Equation12
(12)
(12) ), (Equation14
(14)
(14) ), and (Equation15
(15)
(15) ). Markers are Monte-Carlo simulations. (a) CDF vs. α with various values of
. and (b) PMF vs. α with various values of
.

Figure 3. Solid and dash-dot lines are plotted by employing (Equation11(11)
(11) ), (Equation12
(12)
(12) ), (Equation14
(14)
(14) ), and (Equation15
(15)
(15) ). Markers are Monte-Carlo simulations. (a) Expectation vs. α. and (b) Variance vs. α with different values of
.

Figure 4. Solid and dash-dot lines are plotted by employing (Equation11(11)
(11) ), (Equation12
(12)
(12) ), (Equation14
(14)
(14) ), and (Equation15
(15)
(15) ). Markers are Monte-Carlo simulations. (a) CDF vs.
with various values of
. and (b) PMF vs.
with various values of
.

Figure 5. Solid and dash-dot lines are plotted by employing (Equation11(11)
(11) ), (Equation12
(12)
(12) ), (Equation14
(14)
(14) ), and (Equation15
(15)
(15) ). Markers are Monte-Carlo simulations. (a) Expectation vs.
with various values of
. and (b) Variance vs.
with different values of
.

We observe the contrary behaviours of mean with the CDF with respect to . Particularly, we see that by increasing
the mean will approach the lower bound, i.e.
packets. The rationale behind this phenomenon is following: when
is small, there is a high probability that all users can not decode packets thus the expectation is very high. On the other hand, when
, it is obvious that all users can receive packets without error and thus requiring exactly
time slots to deliver
packets. Besides, the variance approaches zero when
goes to infinity. This can be explained directly from the definition of the variance that the subtraction of two tiny numbers is a very tiny number and it means that the system convergences.
Figures and address the performance of the number of received antennae on the performance of these metrics. We see that increasing simply boosts the CDF and the larger the transmit power the sooner the CDF reaching one. More precisely, with
dBm, it solely requires 3 antennae to reach one for both schemes while for
, it needs 4 antennae for the MRC scheme and larger than 8 antennae for the SC scheme. Again, there exists a peak for the PMF, the main reason is that when
is small it requires more effort to successfully deliver
packets in exactly
time slots. On the other hand, when
is large, the probability to broadcast
packets in the same
time slots will be zero because there is a high probability that the transmitter has already delivered all transmitted packets less than
. Figure (a) stretches the performance of the expectation on the
with different values of
. We see that there also exists a lower bound which is equal to
packets for both schemes when
goes large. The explanation is similar to Figure (a). However, the pace of reaching its lower bound is different between schemes and transmit power. More particular, the larger the transmit power the sooner the expectation reaches its lower bound and the MRC scheme always approaches faster than the SC scheme regardless of the transmit power. As the expectation goes to a constant number, the variance then goes to zero. It can be justified immediately from the definition of the variance.
Figure 6. Solid and dash-dot lines are plotted by employing (Equation11(11)
(11) ), (Equation12
(12)
(12) ), (Equation14
(14)
(14) ), and (Equation15
(15)
(15) ). Markers are Monte-Carlo simulations. (a) CDF vs.
with various values of
. and (b) PMF vs.
with various values of
.

Figure 7. Solid and dash-dot lines are plotted by employing (Equation11(11)
(11) ), (Equation12
(12)
(12) ), (Equation14
(14)
(14) ), and (Equation15
(15)
(15) ). Markers are Monte-Carlo simulations. (a) Expectation vs.
with various values of
. and (b) Variance vs.
with different values of
.

The impact of the number of transmitted packets on the performance of the considered broadcasting networks is given in Figures and . Particularly, Figure (a) shows the behaviour of the CDF concerning on with a constant number of
. We see that under this scenario, the CDF monotonically decreases, and the smaller the transmit power the smaller the CDF. Additionally, we observe that the CDF of the MRC scheme is substantially better than the SC scheme. Also, fixing
the PMF in Figure (b) is also a unimodal function with respect to
. On the other hand, both the expectation and the variance go up with
in Figure . The reason is obvious that broadcasting more packets, for sure, requires more time slots. However, the required time slots for MRC and SC schemes are not the same. The SC scheme, of course, needs more time slots than the MRC scheme to deliver the same
packets. For example, with
packets and
dBm, SC needs approximately 12 time slots while the MRC scheme requires around 8 time slots. We observe the same trend for the variance that it simply increases with
. Nevertheless, the increasing pace is different and relies on the transmit power. Particularly, the variance increases slowly with large
.
Figure 8. Solid and dash-dot lines are plotted by employing (Equation11(11)
(11) ), (Equation12
(12)
(12) ), (Equation14
(14)
(14) ), and (Equation15
(15)
(15) ). Markers are Monte-Carlo simulations. (a) CDF vs.
with various values of
. and (b) PMF vs.
with various values of
.

Figure 9. Solid and dash-dot lines are plotted by employing (Equation11(11)
(11) ), (Equation12
(12)
(12) ), (Equation14
(14)
(14) ), and (Equation15
(15)
(15) ). Markers are Monte-Carlo simulations. (a) Expectation vs.
with various values of
. and (b) Variance vs.
with different values of
.

5. Conclusion
This paper investigated the performance of the broadcasting network employing Fountain code and receiver diversity techniques. Particularly, we derive the closed-form equations of the CDF, PMF, expectation, and variance of the needed time slots to dispatch an arbitrary packets to all users under both MRC and SC schemes. Numerical results confirmed that MRC scheme always outperformed the SC scheme and an increasing number of received antennae as well as the transmit power are beneficial for the systems. The paper can be extended in several directions such as employing relays (N. T. Nguyen, Tran, Trinh et al., Citation2022) and/or reconfigurable intelligent surfaces (RISs) to further improve the system performance (Trinh, Papazafeiropoulos, et al., Citation2021; Trinh, Tu, et al., Citation2021). On the other hand, the paper can also apply tools from stochastic geometry to model the randomness of the wireless nodes (Aravanis et al., Citation2019; Kumar & Tyagi, Citation2021) or apply the deep learning to further optimize the system by exploiting the power of data-driven approach (Tu et al., Citationin press; Zappone et al., Citation2019). Furthermore, we can also combine with other techniques/systems such as energy harvesting, full-duplex, device-to-device communications, cognitive radio networks, etc.
Disclosure statement
The authors declare that there is no conflict of interest in this paper.
Correction Statement
This article has been corrected with minor changes. These changes do not impact the academic content of the article.
Additional information
Funding
Notes on contributors
Lam-Thanh Tu
Lam-Thanh TU (Email: [email protected]) received the B.Eng. degree in electronics and telecommunications engineering from the Ho Chi Minh City University of Technology, Vietnam, in 2009, the M.Sc. degree in telecommunications engineering from the Posts and Telecommunications Institute of Technology, Vietnam, in 2014, and the Ph.D. degree from the University of Paris Sud, Paris-Saclay University, France, in 2018. From 2015 to 2018, he was with the French National Center for Scientific Research (CNRS), Paris, as an Early Stage Researcher of the European-Funded Project H2020 ETN-5Gwireless. From 2019-2021, he was with the Xlim Research Institute, University of Poitiers, France as a postdoctoral research fellow. From 2022, he is with the Faculty of Electrical and Electronics Engineering, at Ton Duc Thang University, Vietnam. His research interests include stochastic geometry, LoRa networks, reconfigurable intelligent surfaces, and artificial intelligence applications for wireless communications.
Tan N. Nguyen
Tan N. Nguyen was born in 1986 in Nha Trang City, Vietnam. He received a BS degree in electronics in 2008 from Ho Chi Minh University of Natural Sciences and an MS degree in telecommunications engineering in 2012 from Vietnam National University. He received a Ph.D. in communications technologies in 2019 from the Faculty of Electrical Engineering and Computer Science at VSB - Technical University of Ostrava, Czech Republic. He joined the Faculty of Electrical and Electronics Engineering of Ton Duc Thang University, Vietnam, in 2013, and since then has been lecturing. His major interests are cooperative communications, cognitive radio, physical layer security and signal processing.
Phuong T. Tran
Phuong T. Tran received the B.Eng. and M.Eng. degrees in electrical engineering from the Ho Chi Minh City University of Technology, Ho Chi Minh City, in 2002 and 2005, respectively, and the M.S. degree in mathematics and the Ph.D. degree in electrical and computer engineering from Purdue University, West Lafayette, IN, USA, in 2013. In 2007, he became a Vietnam Education Foundation Fellow at Purdue University. In 2013, he joined the Faculty of Electrical and Electronics Engineering, Ton Duc Thang University, Vietnam, and he has been served as the Vice Dean of Faculty since October 2014. His major interests are in the area of wireless communications and network information theory.
Tran Trung Duy
Tran Trung Duy received the Ph.D. degree in electrical engineering from University of Ulsan, South Korea. In 2013, he joined Posts and Telecommunications Institute of Technology, Ho Chi Minh city campus (PTIT-HCM), as a lecturer. From 2022, he is an associate Professor of Wireless Communications at PTIT-HCM. His major research interests are cooperative communications, cooperative multi-hop, cognitive radio, physical-layer security, energy harvesting, hardware impairments and Fountain codes.
Quang-Sang Nguyen
Quang-Sang Nguyen received the B.E. degree from Ho Chi Minh City University of Transport, Ho Chi Minh City, Vietnam, in 2010, the M.E. degree from Ho Chi Minh City University of Technology, Ho Chi Minh City, in 2013, and the Ph.D. degree in electrical engineering from the University of Ulsan, Ulsan, South Korea, in 2017. From January 2017 to June 2017, he was a Postdoctoral Research Fellow with Queen's University Belfast, Belfast, U.K. From June 2017 until May 2021, he has been a Lecturer at Duy Tan University, Ho Chi Minh City. Since May 2021, He is currently the Dean of the Faculty of Electrical and Electronics Telecommunications Engineering at Ho Chi Minh City University of Transport. His major research interests are cooperative communication, cognitive radio network, physical layer security, energy harvesting, and non-orthogonal multiple access, Artificial intelligence.
Notes
1 Here, the carrier frequency is selected to align with the current 4G systems. However, the proposed mathematical framework can be applied to all sub-6 Ghz carrier frequencies (Phu et al., Citation2021).
References
- Al Hajj, M., Wang, S., Tu, L.T., Azzi, S., & Wiart, J. (2020). A statistical estimation of 5G massive MIMO networks' exposure using stochastic geometry in mmWave bands. Applied Sciences, 10(23), 8753. https://doi.org/10.3390/app10238753
- Aravanis, A. I., Tu, L.-T., Muñoz, O., Pascual-Iserte, A., & Di Renzo, M. (2019). A tractable closed form approximation of the ergodic rate in Poisson cellular networks. EURASIP Journal on Wireless Communications and Networking, 2019(1), 1–16. https://doi.org/10.1186/s13638-019-1499-9
- Arya, S., & Chung, Y. H. (2018). Non-line-of-sight ultraviolet communication with receiver diversity in atmospheric turbulence. IEEE Photonics Technology Letters, 30(10), 895–898. https://doi.org/10.1109/LPT.2018.2823502
- Dang, T. H., Tran, T. D., Tran, P. T., Do, Q. T., & Tan, H. (2019). Performance comparison between Fountain codes-based secure MIMO protocols with and without using non-orthogonal multiple access. Entropy, 21(10), 982. https://doi.org/10.3390/e21100982
- Danufane, F. H., & Di Renzo, M. (2021). Analysis of the delay distribution in cellular networks by using stochastic geometry. https://doi.org/10.48550/arXiv.2112.12971
- Di Renzo, M., Tu, L.-T., Zappone, A., & Debbah, M. (2019). A tractable closed-form expression of the coverage probability in Poisson cellular networks. IEEE Wireless Communications Letters, 8(1), 249–252. https://doi.org/10.1109/LWC.2018.2868753
- Di Renzo, M., Zappone, A., Tu, L.-T., & Debbah, M. (2018). System-level modeling and optimization of the energy efficiency in cellular networks – a stochastic geometry framework. IEEE Transactions on Wireless Communications, 17(4), 2539–2556. https://doi.org/10.1109/TWC.2018.2797264
- Di Renzo, M., Zappone, A., Tu, L.-T., & Debbah, M. (2019). Spectral-energy efficiency Pareto front in cellular networks: A stochastic geometry framework. IEEE Wireless Communications Letters, 8(2), 424–427. https://doi.org/10.1109/LWC.5962382
- Feng, X., Nguyen, K. A., & Luo, Z. (2022). A survey of deep learning approaches for WiFi-based indoor positioning. Journal of Information and Telecommunication, 6(2), 163–216. https://doi.org/10.1080/24751839.2021.1975425
- Gradshteyn, I. S., & Ryzhik, I. M. (2007). Table of integrals, series, and products (D. Zwillinger & V. Moll (Ed.)). (7th ed.). Elsevier/Academic Press.
- Hassan, M. U., Rehmani, M. H., & Faheem, Y. (2019). Performance evaluation of broadcasting strategies in cognitive radio networks. Wireless Networking, 25( 3), 999–1016. https://doi.org/10.1007/s11276-017-1647-2
- Hossain, M. N. (2022). Performance evaluation of MIMO DFT-Spread WR-OFDM system for spectrum efficiency and power efficiency. Journal of Information and Telecommunication. https://doi.org/10.1080/24751839.2022.2066847
- Indoonundon, M., & Fowdur, T. P. (2021). Overview of the challenges and solutions for 5G channel coding schemes. Journal of Information and Telecommunication, 5(4), 460–483. https://doi.org/10.1080/24751839.2021.1954752
- Kumar, V., & Tyagi, N. (2021). An integrated-multi-RAT framework for multipath-computing in heterogeneous-wireless network. Journal of Information and Telecommunication, 5(3), 350–374. https://doi.org/10.1080/24751839.2021.1871819
- Li, Y., Tang, B., Wang, J., & Bao, Z. (2020). On multi-hop short-packet communications: Recoding or end-to-end Fountain coding? IEEE Transactions on Vehicular Technology, 69(8), 9229–9233. https://doi.org/10.1109/TVT.2020.3005409
- Liao, W., Tuan, S., Lee, Y., & Ho, M. (2018). A diversity receiver-based high-gain broad-beam reception array antenna. IEEE Antennas and Wireless Propagation Letters, 17(3), 410–413. https://doi.org/10.1109/LAWP.2018.2792438
- Lim, W. J., Abbas, R., Li, Y., Vucetic, B., & Shirvanimoghaddam, M. (2021). Analysis and design of analog Fountain codes for short packet communications. IEEE Transactions on Vehicular Technology, 70(12), 12662–12674. https://doi.org/10.1109/TVT.2021.3118792
- Motlagh, N. H., Bagaa, M., & Taleb, T. (2021). UAV-based IoT platform: A crowd surveillance use case. IEEE Communications Magazine, 55(2), 128–134. https://doi.org/10.1109/MCOM.2017.1600587CM
- Nguyen, N.-L., Tu, L.-T., Nguyen, T. N., Nguyen, P.-L. T., & Nguyen, Q.-S. (2023). Performance on cognitive broadcasting networks employing Fountain codes and maximal ratio transmission. Radioengineering, 32(1), 1–10.https://doi.org/10.13164/re.2023.0001
- Nguyen, N. T., Nguyen, V. S., Nguyen, H. G., Tu, L.-T., Trinh, V. C., & Nguyen, T. H. (2022). On the performance of underlay device-to-device communications. Sensors, 22(4), 1456. https://doi.org/10.3390/s22041456
- Nguyen, N. T., Tran, D.-H., Trinh, V. C., Phan, V.-D., Voznak, M., & Chatzinotas, S. (2022). Security and reliability analysis of satellite-terrestrial multirelay networks with imperfect CSI. IEEE Systems Journal. https://doi.org/10.1109/JSYST.2022.3201128
- Nguyen, N. T., Tran, H. Q. M., Tran, T. P., Voznak, M., Tran, T. D., Nguyen, T.-L., & Phu, T. T. (2019). Performance enhancement for energy harvesting based two-way relay protocols in wireless ad-hoc networks with partial and full relay selection methods. Ad Hoc Networks, 84, 178–187. https://doi.org/10.1016/j.adhoc.2018.10.005
- Nguyen, N. T., Tran, T. D., Tran, T. P., Voznak, M., Li, X., & Poor, H. V. (2022). Partial and full relay selection algorithms for AF multi-relay full-duplex networks with self-energy recycling in non-identically distributed fading channels. IEEE Transactions on Vehicular Technology, 71(6), 6173–6188. https://doi.org/10.1109/TVT.2022.3158340
- Nguyen, N. T., Tu, L.-T., Tran, D.-H., Phan, V.-D., Voznak, M., Chatzinotas, S., & Ding, Z. (in press). Outage performance of satellite terrestrial full-duplex relaying networks with co-channel interference. IEEE Wireless Communications Letters. https://doi.org/10.1109/LWC.2022.3175734
- Nguyen, S. Q., & Kong, H. Y. (2016). Generalized diversity combining of energy harvesting multiple antenna relay networks: Outage and throughput performance analysis. Annals of Telecommunications, 71(5-6), 265–277. https://doi.org/10.1007/s12243-016-0508-9
- Nguyen, S. Q., & Kong, H. Y. (2017). Secrecy enhancement in two-hop DF relaying system under hardware impairment. International Journal of Electronics, 104(3), 442–461. https://doi.org/10.1080/00207217.2016.1218063
- Nguyen, T. H., Jung, W.-S., Tu, L.-T., Trinh, V. C., Yoo, D., & Ro, S. (2020). Performance analysis and optimization of the coverage probability in dual hop LoRa networks with different fading channels. IEEE Access, 8, 107087–107102. https://doi.org/10.1109/Access.6287639
- Pham, M. N., Hung, H. D., Tu, L.-T., P. V. Tuan, Duy, T. T., & Hanh, T. (2022). Outage performance of interference cancellation-aided two-way relaying cognitive network with primary TAS/SC communication and secondary partial relay selection. Electronics, 11(22), 3645. https://doi.org/10.3390/electronics11223645
- Phu, T. T., Nguyen, N. T., Nguyen, Q. S., Tran, T. D., Tran, P. T., & Voznak, M. (2020). Rateless codes-based secure communication employing transmit antenna selection and harvest-to-jam under joint effect of interference and hardware impairments. Entropy, 21(7), 700. https://doi.org/10.3390/e21070700
- Phu, T. T., Phan, V.-D., Nguyen, T. N., Tu, L.-T., Minh, B. V., Voznak, M., & Fazio, P. (2021). Outage analysis of the power splitting based underlay cooperative cognitive radio networks. Sensors, 21(22), 7653. https://doi.org/10.3390/s21227653
- Ragpot, P., Fowdur, T. P., & Soyjaudah, K. M. S. (2019). Enhanced MP3 transmission over Wi-Fi and LTE networks using unequal error protection and varying frequency transforms. Journal of Information and Telecommunication, 3(4), 508–532. https://doi.org/10.1080/24751839.2019.1647489
- Tran, T. D., Anpalagan, A., & Kong, H.-Y. (2012). Multi-hop cooperative transmission using Fountain codes over Rayleigh fading channels. Journal of Communications and Networks, 14(3), 267–272. https://doi.org/10.1109/JCN.5449605
- Tran, T. D., Le, K., Nguyen, T. B., & Nguyen, L. N. (2021). Intercept probability analysis of cooperative cognitive networks using Fountain codes and cooperative jamming. EAI Endorsed Transactions on Industrial Networks and Intelligent Systems, 8( 26), 168229. https://doi.org/10.4108/eai.26-1-2021.168229
- Trinh, V. C., Papazafeiropoulos, A. K., Tu, L.-T., Chopra, R., Chatzinotas, S., & Ottersten, B. (2021). Outage probability analysis of IRS-assisted systems under spatially correlated channels. IEEE Wireless Communications Letters, 10(8), 1815–1819. https://doi.org/10.1109/LWC.2021.3082409
- Trinh, V. C., Tu, L.-T., Chatzinotas, S., & Ottersten, B. (2021). Coverage probability and ergodic capacity of intelligent reflecting surface-enhanced communication systems. IEEE Communications Letters, 25(1), 69–73. https://doi.org/10.1109/COML.4234
- Tsai, M.-C., Bashir, M. S., & Alouini, M.-S. (2022). Data combining schemes for a detector array receiver in free-space optical communications. IEEE Open Journal of the Communications Society, 3, 1090–1102. https://doi.org/10.1109/OJCOMS.2022.3181812
- Tu, L.-T., Bradai, A., Ahmed, O. B., Garg, S., Pousset, Y., & Kaddoum, G. (in press). Energy efficiency optimization in LoRa networks – a deep learning approach. IEEE Transactions on Intelligent Transportation Systems. https://doi.org/10.1109/TITS.2022.3183073
- Tu, L.-T., Bradai, A., Pousset, Y., & Aravanis, A. I. (2021). Energy efficiency analysis of LoRa networks. IEEE Wireless Communications Letters, 10(9), 1881–1885. https://doi.org/10.1109/LWC.2021.3084996
- Tu, L.-T., Bradai, A., Pousset, Y., & Aravanis, A. I. (2022). On the spectral efficiency of LoRa networks: Performance analysis, trends and optimal points of operation. IEEE Transactions on Communications, 70(4), 2788–2804. https://doi.org/10.1109/TCOMM.2022.3148784
- Tu, L.-T., & Di Renzo, M. (2020). On the energy efficiency of heterogeneous cellular networks with renewable energy sources – a stochastic geometry framework. IEEE Transactions on Wireless Communications, 19(10), 6752–6770. https://doi.org/10.1109/TWC.7693
- Tu, L.-T., Di Renzo, M., & Coon, J. P (2018). Stochastic geometry analysis of receiver diversity in cellular networks with SWIPT. In 2018 IEEE 19th International Workshop on Signal Processing Advances in Wireless Communications (SPAWC). https://doi.org/10.1109/SPAWC.2018.8445877
- Tu, L.-T., Nguyen, N. T., Tran, T. D., Tran, P. T., Voznak, M., & Aravanis, A. I. (2022). Broadcasting in cognitive radio networks: A Fountain codes approach. IEEE Transactions on Vehicular Technology, 71(10), 11289–11294. https://doi.org/10.1109/TVT.2022.3188969
- Zappone, A., Di Renzo, M., Debbah, M., Tu, L.-T., & Qian, X. (2019). Model-aided wireless artificial intelligence: Embedding expert knowledge in deep neural networks for wireless system optimization. IEEE Vehicular Technology Magazine, 14(3), 60–69. https://doi.org/10.1109/MVT.10209