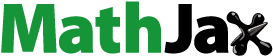
ABSTRACT
The average transmit power and coverage probability (Pcov) of uplink energy harvesting-enabled long-range networks are investigated in the present paper. Particularly, we model the end-device (EDs) according to the homogeneous Poisson point process while the power beacon is randomly distributed on the circle in the middle of the network. All EDs rely on the harvested energy to perform their operations and transmissions. Under this context, the upper bound of the average transmit power of an end-device is derived in the closed-form expression. The signal-to-noise-ratio condition of the coverage probability is given in the closed-form expression as well. Simulation results are provided to corroborate the accuracy of the derived mathematical framework as well as to feature the impact of some key parameters on the considered metrics. Our findings unveil that increasing either the number of power beacons or their transmit power will monotonically ameliorate the Pcov. Nevertheless, rising the average number of EDs will significantly decline the Pcov's performance.
1. Introduction
Low power wide area network (LPWAN) has emerged as one of the most effective networks to implement Internet of Things (IoTs) (Raza et al., Citation2017). More precisely, compared with conventional wireless networks such as cellular networks and local area networks (LAN), that is, WiFi, that require high power consumption as well as well-organized signalling and synchronization. The LPWAN, on the other hand, requires low power consumption at end devices (EDs) and aims for a long transmission distance. It, as a consequence, is suitable for remote terminals like sensors that are hard to connect to the power grid and/or replace the battery. Among all available LPWAN networks, for example, SigFox, LoRa, NB-IoTs, WiFi Halow, etc. (Goursaud & Gorce, Citation2015). Long-range (LoRa) has gained much attention from both academia and industry. The main advantage of LoRa networks is its patented chirp spread spectrum (CSS) modulation that attains better performance compared with conventional modulations like quadrature amplitude modulation (QAM), and phase shift keying (PSK) (Huan et al., Citation2022). Another superiority of the LoRa networks is that it allows transmitting over a long transmission distance with ultra-low power consumption by properly configuring a set of parameters such as spreading factor (SF), coding rate (CR), payload, and so on.
Energy harvesting (EH), on the other hand, is one of the most advanced techniques to significantly ameliorate the energy efficiency (EE) of wireless networks (Tu & Di Renzo, Citation2020). Particularly, by harvesting the ambient radio frequency (RF) signals to recharge the battery, it can not only prolong the devices' lifetime but also be independent of the power grid. Generally, there are two approaches to implementing EH-enabled networks which are power beacon (PB) based and simultaneous wireless information and power transfer (SWIPT) approaches (Lu et al., Citation2015). The latter permits the receiver to concurrently decode information and absorb energy from the incoming RF signals by properly splitting the received signals in time and/or frequency and/or space. The main drawback of this approach is that instead of directly sending received signals to the decoding circuit like non-EH-enabled networks, they need to part with the harvesting circuit. As a consequence, compared with the performance of the conventional networks in terms of spectral efficiency (SE), the EH-enabled network is not comparable. The former approach, on the other hand, separates the RF signals for harvesting energy and decoding information. More precisely, there are dedicated RF signals broadcast by power beacons to power up wireless devices and are different from conventional RF signals for decoding information. Thus, it does not degrade the performance of the networks like the SWIPT-based approach.
As a result, in the present work, we consider the EH-enabled LoRa networks to facilitate the benefits of both networks and technologies. The performance of LoRa networks was largely investigated in (Bor & Roedig, Citation2017; Croce et al., Citation2018; Georgiou & Raza, Citation2017; Mahmood et al., Citation2019; Nguyen et al., Citation2020; Tu et al., Citation2021, Citation2022c). More precisely, authors in (Georgiou & Raza, Citation2017) investigated the coverage probability (Pcov) of an uplink LoRa networks. They, however, ignored the correlation between the signal-to-noise ratios (SNR) and signal-to-interference ratio (SIRs) at the receiver. To overcome such an issue, a novel definition of the Pcov was proposed in (Tu et al., Citation2022c) where the authors addressed the performance of not only the Pcov but also area spectral efficiency (ASE). A set of LoRa parameters was optimally selected to minimize transmission energy cost in (Bor & Roedig, Citation2017) while the energy efficiency of the LoRa networks was addressed in (Tu et al., Citation2021). The authors in (Croce et al., Citation2018) investigated the imperfect orthogonality between spreading factors. The performance of dual-hop LoRa networks and their scalability were investigated in (Nguyen et al., Citation2020) and (Mahmood et al., Citation2019). On the other hand, the performance of the EH-enabled wireless networks was given in (Lakshmi & Jibukumar, Citation2021; Nasir et al., Citation2013; Nguyen et al., Citation2016, Citation2022, Citation2023a; Tu & Di Renzo, Citation2017; Tu et al., Citation2016). Particularly, Nasir and other authors proposed two protocols to realize the SWIPT-based networks, that is, the time switching and power splitting protocols. The Pcov under the SWIPT mmWave communications was conducted in (Tu & Di Renzo, Citation2017) while the user selection under the EH-based random networks was investigated in (Nguyen et al., Citation2016). Nguyen and other authors investigated the trade-off between reliability and security in SWIPT-enabled full-duplex relaying networks (Nguyen et al., Citation2023a) and a hybrid protocol for SWIPT cooperative networks was proposed in (Lakshmi & Jibukumar, Citation2021). The receiver diversity under SWIPT cellular networks was investigated in (Tu et al., Citation2016) while the Pcov of the non-orthogonal multiple access (NOMA) IoT networks with Nakagami-m distribution was derived in (Nguyen et al., Citation2022).
All the above-mentioned works separately study the performance of the LoRa and EH-enabled networks. The performance of the EH-enabled LoRa networks, on the other hand, is still in the infancy stage with a few works (Hamdi et al., Citation2022; Hamdi & Qaraqe, Citation2021). More particular, by optimally allocating the SF and device scheduling, the maximization of the EE in LoRa networks where EDs were powered by a PB was addressed in (Hamdi & Qaraqe, Citation2021). The power grid minimization was formulated and solved in (Hamdi et al., Citation2022) by a heuristic algorithm and deep reinforcement learning approach. Regarding the applications of stochastic geometry (SG) and energy harvesting techniques into LoRa networks, only a work from Georgiou and his colleague in (Georgiou et al., Citation2021) studied such networks. More precisely, they addressed the steady-state distribution of the capacitor voltage and the impact of co-SF interference on the outage probability (OP). We, on the other hand, derived the coverage probability and the average harvested energy. Hence, different from the all above-mentioned works, in the present paper, we investigate the power beacon-based LoRa networks. All power beacon stations are randomly distributed on a circle in the middle of the networks and EDs are modelled according to the homogeneous Poisson point process (PPP). More precisely, the main contributions and novelties are summarized as follows:
The positions of EDs are modelled according to the Poisson point process while the positions of PBs are randomly distributed on a circle in the middle of the networks.
We derive the upper bound of the average transmit power of an ED in the closed-form expression. The SNR condition of the Pcov is presented in the closed-form equation as well.
We employ extensively computer-based simulations to verify the accuracy of the derived mathematical frameworks and to unveil the impact of several key parameters on the considered metrics.
The remainder of the manuscript is organized as follows: Section 2 provides the system model. The main derivations are given in Section 3. Numerical results are provided in Section 4. Section 5 concludes the paper.
2. System model
Let us consider an uplink EH-enabled LoRa network comprises of a single gateway that is located at the centre of the two-dimensional plane with a radius RFootnote1 and multiple end-devices which are distributed around the gateway as shown in . The end-devices are modelling according to the homogeneous Poisson point process denoted by with intensity
(Di Renzo et al., Citation2019b). Additionally,
power beacons are randomly deployed on the circle with centre at the gateway and radius
. It is emphasized that the PPP is employed instead of other point processes such as Matérn cluster point processes owing to its mathematical tractability. Moreover, all nodes including the LoRa gateway, power beacon, and end-devices are equipped with only a single antenna. The considered network is operated at the industrial, scientific, and medical (ISM) band. Yet the interference from other technologies operating with the same band is not taken into consideration owing to the advantages of CSS modulations. Different from the conventional LoRaWAN protocol, we adopt the slotted-ALOHA protocol at the medium access control (MAC) layer since its performance is better than the conventional one (Beltramelli et al., Citation2021).
2.1. Channel modelling
In this work, the transmission link from EDs to the gateway is suffered from both small-scale fading and large-scale path loss. The impact of the shadowing can be implicitly studied by modifying the density of the EDs (Di Renzo et al., Citation2018).
2.1.1. Small-scale fading
Considering a generic transmission link between EDs and the gateway, the channel coefficient denoted by h is modelled by a complex Gaussian random variable (RV) with zero mean and Ω variance, that is, . As a consequence, the channel gain denoted by
is followed by an exponential distribution with mean Ω. We further assume that the small-scale fading is a block flat fading thus, it remains stable for a whole time slot and changes independently between time slots (Tu et al., Citation2023b).
2.1.2. Large-scale path-loss
Besides the effects of the small-scale fading, the transmission link from EDs to the gateway is also subjected to the large-scale path-loss denoted by Λ and is calculated as follows:
(1)
(1) where
is the maximum function and
is the path-loss constant,
is the path-loss exponent relying on the transmission environment. For example, under the urban environment α will be generally between 3.5−4.5 while in the rural areas, its value will approach 2.
is the wavelength,
(in Hz) and
are the carrier frequency and the speed of light. d is the transmission distance from EDs to the gateway.
Remark 2.1
It is certain that the adopted large-scale path-loss model has overcome the singularity issue in the popular unbounded path-loss model where the transmission distance approaches zero, and the received power then approaches infinity (Aravanis et al., Citation2019).
2.2. Spreading factor allocation
In this work, the fair-collision probability allocation is adopted. Under this scheme, the probability that an ED is allocated to the kth SF, , is computed by the following equation:
(2)
(2)
Remark 2.2
It is emphasized that the adopted fair-collision probability SF allocation achieves better performance compared with the popular distance-based approach (Reynders et al., Citation2017).
2.3. Energy harvesting modelling
We assume that the ED is not connected to the power grid and counts solely on the harvested energy from all power beacons during sleep mode. Let us denote by the harvested energy of the oth ED utilized SFk, it is formulated as follows (Nguyen et al., Citation2017):
(3)
(3) where
is the transmit power of the power beacon. Here, we assume that all power beacons emit the same transmit power.
is the channel gain from the ith power beacon to the oth ED and
is the sleeping period of the ED utilized SFk and is the function of the transmission duration
and the total transmission duration
. Here,
is the notification duration between two transmissions, and ρ is the duty cycle. Generally, ρ is defined according to government regulation and is not greater than 1%.
is the energy conversion efficiency.
2.4. Signal-to-Noise ratio and signal-to-interference ratio
2.4.1. Signal-to-Noise ratio
The signal-to-noise ratio at the gateway from the desired ED denoted by subscript ‘0’ operated at SFk is formulated as (4)
(4) Here
is the transmit power of the intended ED and is a function of the harvested energy. It is noted that we skip the energy consumption for other activities since it is negligible compared with the transmit power.
is the noise variance of the additive white Gaussian noise (AWGN); NF (in dB) is the noise figure of the gateway, BW (in Hz) is the transmission bandwidth,
is the logarithm function;
is the channel gain from desired ED to the gateway.
2.4.2. Signal-to-Interference Ratio
Besides the SNR, the received signals at the gateway also suffer from interference from co-SF interference. The signal-to-interference ratio is formulated as follows:
(5)
(5) where
is the channel gain from the ith ED utilized SFk,
is its transmit power, and
is the set of active EDs utilized SFk.
2.5. Coverage probability
In this work, we study the coverage probabilityFootnote2, Pcov, of the ED. The coverage probability is the probability that the received signals at the gateway are greater than a predefined threshold. It is noted that different from other wireless networks the predefined threshold of LoRa networks is a discrete set and relies on the utilized SF. Additionally, the Pcov comprises two conditions, the SNR and SIR conditions, instead of a single condition in the form of the signal-to-interference-plus-noise (SINR). Mathematical speaking, the Pcov under SFk is formulated as follows (Di Renzo et al., Citation2019a):
(6)
(6) Here
is the probability operator;
(in dBm),
is the target threshold;
is the co-SF interference threshold and is equal to 1 dB (Croce et al., Citation2018, Table II).
3. Performance analysis
Before going to compute the Pcov, the two following useful Lemmas that address the performance of the average transmit power of each ED and the characteristics of the ratio of the channel gain and path-loss from an ED to the gateway are given as follows:
Lemma 3.1
Let us denote by the upper bound of the average transmit power from (Equation3
(3)
(3) ) and (Equation4
(4)
(4) ) of ED utilized SFk, it then computes as
(7)
(7)
where is the expectation operator.
Proof.
The proof is given in Appendix A.
Lemma 3.2
Let us denote random variable , its complementary cumulative distribution function (CCDF), CDF, and PDF are computed as follows:
(8)
(8) Here
is the CCDF of RV Y.
Proof.
The proof is given in Appendix B.
Remark 3.1
It is emphasized that the outcomes in Lemma 3.2 can be straightforwardly applied to the Nakagami-m distribution provided that m is an integer number, that is, .
Having obtained the average transmit power and the CCDF, CDF, and PDF of the ratio of the channel gain and large-scale path-loss, we are ready to compute the considered metric, that is, the coverage probability. Let us begin with the definition of Pcov in (Equation6(6)
(6) ) as follows:
(9)
(9) where
is held by assuming that the SNR and SIR are uncorrelated random variablesFootnote3. The integration
and
refer to the SNR and SIR conditions of the Pcov and are computed as follows:
(10)
(10) where
and
is achieved with the help of Lemma 3.2 and
comes from Lemma 3.1. The second integration,
is computed as follows:
(11)
(11) where
is held by approximating the aggregate interference by the strongest interferer,
, O is the number of active EDs. The CDF and PDF of RV Z are calculated by
and
. The last equation can be effectively computed by employing popular software such as Matlab, Mathematica, and Python.
4. Numerical results
In this section, computer-based simulation results are provided to justify the precision of the developed mathematical framework as well as to reveal the influences of some key parameters such as the average number of end devices, the number of power beacons, and the transmit power of the PB. Without loss of generality, the following set of parameters are used in this section: Bw = 125 kHz, α = 2.5, = 920 MHz,
= 30 dBm, N = 5, NF = 6 dB, R = 2000 m, Ω = 3.5,
,
= 500, ρ = 0.01,
,
,
, respectively.
stretches the average transmit power of the ED w.r.t. the number of power beacons. We first observe that there is a good agreement between the derived mathematical framework and the simulation results. Second, the average transmit power is a monotonic increasing function of the N which can be straightforwardly seen in (Equation7(7)
(7) ). Third, the higher the path-loss exponent the smaller the average transmit power. This phenomenon can be explained that with the larger the path-loss exponent the higher the path-loss and the smaller the receiver power at the ED.
Figure 2. Average transmit power vs. number of power beacons, N with various values of path-loss exponent. Solid lines are computed by (Equation7(7)
(7) ) while markers are from Monte-Carlo simulations.

illustrates the CDF of RV X defined in (EquationA1(A1)
(A1) ) with different values of the network radius R. We see that the larger the network radius the smaller the CDF. The rationale behind this observation is that the transmission distance is getting further hence the RV X becomes smaller so achieves a higher probability. We experience again that the curves from the mathematical framework absolutely align with the Monte Carlo results.
Figure 3. CDF of RV X in (EquationA1(A1)
(A1) ) with various values of the network radius R. Solid lines are computed by (EquationA2
(A2)
(A2) ) while markers are from Monte-Carlo simulations.
![Figure 3. CDF of RV X in (EquationA1(A1) E{Pi,k}=∑i=1NχPP|hi,o|2Tsleep,kmax{1,‖po−pi‖α}Ttrans,k≤Pk=∑i=1NχPP|hi,o|2Tsleep,kmax{1,toα}Ttrans,k=NχPPTsleep,kTtrans,kE{X=|hi,o|2max{1,toα}}.(A1) ) with various values of the network radius R. Solid lines are computed by (EquationA2(A2) FX(x)=Pr{X=|hi,o|2max{1,toα}≤x}=(a)∫0R/2(1−exp(−xmax{1,tα}Ω))2Rdt=1−2R∫0R/2exp(−xmax{1,tα}Ω)dt=1−2R[∫01exp(−xΩ)dt+∫1R/2exp(−xtαΩ)dt]=(b)1−2Rexp(−xΩ)−2R1α(Ωx)1α[Γ(1α,xΩ)−Γ(1α,xΩ(R2)α)],(A2) ) while markers are from Monte-Carlo simulations.](/cms/asset/407d6b40-1635-419a-b95e-997cf738f071/tjit_a_2281144_f0003_oc.jpg)
The influences of the transmit power of ED on the performance of coverage probability is given in . We discover that the mathematical framework denoted by ‘Framework’ is a lower bound of both simulation results. The curves with dot red markers are simulated results that consider the strongest interference while the curves with diamond black markers consider the aggregate interference. All curves plotted by Monte Carlo simulations take into account the correlation between the SNR and SIR in (Equation9(9)
(9) ). It is no doubt that the curves considering the strongest interferer are an upper bound of the aggregate interference. Nevertheless, the gap between these cases is minor. Thus, it is reasonable to approximate the aggregate interference by the strongest interferer in LoRa networks. From , the Pcov of SF12 achieves a higher performance compared with the ones utilized by SF7 under the current setup. It is expected that increasing the transmit power of the power beacon is beneficial for the Pcov. However, there exists an upper limit of the Pcov if
keeps increasing.
Figure 4. Coverage probability vs. transmit power of power beacon, . Solid lines are computed by (Equation9
(9)
(9) ) while markers are from Monte-Carlo simulations. Dot red markers consider the strongest interferer while diamond black markers consider the aggregate interference. All simulation curves take into account the correlation between SNR and SIR.

addresses the performance of the Pcov w.r.t the number of power beacons. Inspecting this figure, we observe that the Pcov behaviours w.r.t. the number of power beacon N is similar to that it is a monotonic increasing function. Nonetheless, the increasing pace is nonlinear. More precisely, the Pcov dramatically goes up when N is small, that is, N<10, it then rises at a lower pace. We also witness a negligible gap between curves considering all interference, the strongest interferer, and the framework, especially for SF12.
Figure 5. Coverage probability vs. the number of power beacon. Solid lines are computed by (Equation9(9)
(9) ) while markers are from Monte-Carlo simulations. Dot red markers consider the strongest interferer while diamond black markers consider the aggregate interference. All simulation curves take into account the correlation between SNR and SIR.

The impact of the inter-arrival between two transmissions on the Pcov performance is provided in . It is evident that the longer the notification between two transmissions (or the fewer the number of transmissions), the better the Pcov. It can be effortlessly explained that the ED will harvest more energy when the duration between two transmissions extends, boosting the transmit power and the Pcov. Moreover, it is interesting to point out that the Pcov changes from a noise-limited regime to an interference-limited regime when increases. Particularly, when
is small, that is,
minutes, the Pcov is in a noise-limited regime because the amount of harvested energy is modest which leads to a limited transmit power. Nonetheless, when
is getting larger, the system gradually changes to an interference-limited regime. Because the harvested energy is enough to overcome the negative impact of the background noise. Finally, we experience again that the larger the SF the higher the Pcov.
Figure 6. Coverage probability vs. the notification duration between two transmissions. Solid lines are computed by (Equation9(9)
(9) ) while markers are from Monte-Carlo simulations. Dot red markers consider the strongest interferer while diamond black markers consider the aggregate interference. All simulation curves take into account the correlation between SNR and SIR.

studies the Pcov performance regarding the average number of EDs. We see that increasing will decline the Pcov as the interference scales up with
. The gap between the proposed mathematical framework and the case considering the maximal interference is indistinguishable for both SF7 and SF11.
Figure 7. Coverage probability vs. the average number of EDs, that is, . Solid lines are computed by (Equation9
(9)
(9) ) while markers are from Monte-Carlo simulations. Dot red markers consider the strongest interferer while diamond black markers consider the aggregate interference. All simulation curves take into account the correlation between SNR and SIR.

5. Conclusion
We studied the performance of the average transmit power and the coverage probability of an uplink EH-enabled LoRa network. More particular, we presented in explicit form the average transmit power and the SNR condition of the Pcov. Numerical results revealed that increasing the number of power beacons and their transmit power is beneficial for the Pcov. Nonetheless, rising the average number of EDs is harmful for this metric. The present work can be extended in several directions. One of the feasible directions is to apply the Fountain codes to enhance the transmission efficiency (Ngoc et al., Citation2022; Tu et al., Citation2022d, Citation2023a). Another interesting direction is to apply MIMO and/or transmit/receive diversity techniques to significantly ameliorate the system performance (Hossain, Citation2022; Mahey & Malhotra, Citation2015). Other possible extensions can be considered such as mmWave communications to scale up the spectral efficiency, reconfigurable intelligent surfaces (RIS) (Al Hajj et al., Citation2020; Chien et al., Citation2022; Tu et al., Citation2015; Van Chien et al., Citation2021). Applying deep learning (DL) into LoRa networks is also an interesting extension (Tu et al., Citation2022a).
Latex_source.zip
Download Zip (1.2 MB)Disclosure statement
No potential conflict of interest was reported by the author(s).
Additional information
Funding
Notes on contributors
Thi-Tuyet-Hai Nguyen
Thi-Tuyet-Hai Nguyen (Email: [email protected]) got the Ph.D. degree from La Rochelle University, La Rochelle, France, in 2020. She has been working at the Faculty of Information Technology 2, Posts and Telecommunications Institute of Technology, HCM campus, Vietnam since 2012. Her research interests are natural language processing, machine learning, information retrieval, chatbot, and LoRa networks.
Tan N. Nguyen
Tan N. Nguyen (Email: [email protected]) was born in 1986 in Nha Trang City, Vietnam. He received a BS degree in electronics in 2008 from Ho Chi Minh University of Natural Sciences and an MS degree in telecommunications engineering in 2012 from Vietnam National University. He received a Ph.D. in communications technologies in 2019 from the Faculty of Electrical Engineering and Computer Science at VSB – Technical University of Ostrava, Czech Republic. He joined the Faculty of Electrical and Electronics Engineering of Ton Duc Thang University, Vietnam, in 2013, and since then has been lecturing. His major interests are cooperative communications, cognitive radio, physical layer security and signal processing.
Tran Trung Duy
Tran Trung Duy (Email: [email protected]) received the Ph.D degree in electrical engineering from University of Ulsan, South Korea in 2013. In 2013, he joined Posts and Telecommunications Institute of Technology, HoChiMinh city campus (PTIT-HCM), as a lecturer. From 2022, he is an associate Professor of Wireless Communications at PTIT-HCM. His major research interests are cooperative communications, cooperative multi-hop, cognitive radio, physical-layer security, energy harvesting, hardware impairments and Fountain codes.
Nguyen Hong Son
Nguyen Hong Son (Email: [email protected]) received his B.Sc. in Computer Engineering from The University of Technology in HCM city, and his M.Sc. and Ph.D. in Communication Engineering from the Post and Telecommunication Institute of Technology in Hanoi, Vietnam. His research interests include communication engineering, machine learning, network security, and cloud computing.
Tan Hanh
Tan Hanh (Email: [email protected]) received the PhD degree from Grenoble Institute of Technology, France. Currently, he is vice president of Posts and Telecommunications Institute of Technology (PTIT), in charge of Ho Chi Minh city campus. His research interests are machine learning, information retrieval, and data mining.
Minh Bui Vu
Minh Bui Vu (Email: [email protected]) was born on March 02, 1991 in Dong Nai, Vietnam. He graduated in Electrical and Electronic Engineering in 2014 from Nguyen Tat Thanh University, Ho Chi Minh City, Vietnam. End of 2014, he joined the Faculty of Electrical and Electronics of Nguyen Tat Thanh University as Laboratory-Practice management, until in 2017 he was a lecturer. In 2019, he received Master degree in Engineering Electrical from Ho Chi Minh City University of Technology and Education. His major research interests are Artificial Neuron Network, Fuzzy Logic, Wireless Networks.
Lam-Thanh Tu
Lam-Thanh Tu (Email: [email protected]) received the Ph.D. degree from the University of Paris Sud, Paris-Saclay University, France, in 2018. From 2022, he has been with the Faculty of Electrical and Electronics Engineering, at Ton Duc Thang University, Vietnam. His research interests include stochastic geometry, LoRa networks, reconfigurable intelligent surfaces, covert communications, and artificial intelligence applications for wireless communications.
Notes
1 Here we consider a finite network radius , if the network's radius approaches infinity, the system performance will, of course, degrade owing to the severe impact of large-scale path-loss. To mitigate such an ultra-long transmission, multiple gateways are one of the most effective solutions since they dramatically reduce the transmission distance (Tu et al., Citation2022b). Nonetheless, we leave such an interesting network for future work.
2 Other performance metrics such as average lifetime, and spectral efficiency can be effortlessly extended from the coverage probability (Nguyen et al., Citation2023b). Nonetheless, to comprehensively study these metrics, we leave it for the future work.
3 It is noted that the derived framework can be considered as an upper bound of the exact framework (Tu et al., Citation2020).
References
- Al Hajj, M., Wang, S., Tu, L. T., Azzi, S., & Wiart, J. (2020). A statistical estimation of 5g massive mimo networks' exposure using stochastic geometry in mmwave bands. Applied Sciences, 10(23), 8753. https://doi.org/10.3390/app10238753
- Aravanis, A. I., Tu, L. T., Muñoz, O., Pascual-Iserte, A., & Renzo, M. D. (2019). A tractable closed form approximation of the ergodic rate in poisson cellular networks. EURASIP Journal on Wireless Communications and Networking, 2019(1). https://doi.org/10.1186/s13638-019-1499-9
- Beltramelli, L., Mahmood, A., Österberg, P., & Gidlund, M. (2021). Lora beyond aloha: An investigation of alternative random access protocols. IEEE Transactions on Industrial Informatics, 17(5), 3544–3554. https://doi.org/10.1109/TII.9424
- Bor, M., & Roedig, U. (2017). Lora transmission parameter selection. In 2017 13th International Conference on Distributed Computing in Sensor Systems (DCOSS), (pp. 27–34).
- Chien, T. V., Tu, L. T., Tran, D. H., Nguyen, H. V., Chatzinotas, S., Renzo, M. D., & Ottersten, B. (2022). Controlling smart propagation environments: Long-term versus short-term phase shift optimization. In ICASSP 2022 – 2022 IEEE International Conference on Acoustics, Speech and Signal Processing (ICASSP), (pp. 5348–5352).
- Croce, D., Gucciardo, M., Mangione, S., Santaromita, G., & Tinnirello, I. (2018). Impact of lora imperfect orthogonality: Analysis of link-level performance. IEEE Communications Letters, 22(4), 796–799. https://doi.org/10.1109/LCOMM.2018.2797057
- Di Renzo, M., Tu, L. T., Zappone, A., & Debbah, M. (2019a). A tractable closed-form expression of the coverage probability in Poisson cellular networks. IEEE Wireless Communications Letters, 8(1), 249–252. https://doi.org/10.1109/LWC.2018.2868753
- Di Renzo, M., Zappone, A., Tu, L. T., & Debbah, M. (2018). System-level modeling and optimization of the energy efficiency in cellular networks – a stochastic geometry framework. IEEE Transactions on Wireless Communications, 17(4), 2539–2556. https://doi.org/10.1109/TWC.2018.2797264
- Di Renzo, M., Zappone, A., Tu, L. T., & Debbah, M. (2019b). Spectral-energy efficiency pareto front in cellular networks: A stochastic geometry framework. IEEE Wireless Communications Letters, 8(2), 424–427. https://doi.org/10.1109/LWC.5962382
- Georgiou, O., Psomas, C., Demarchou, E., & Krikidis, I. (2021). Lora network performance under ambient energy harvesting and random transmission schemes. In ICC 2021 – IEEE International Conference on Communications (pp. 1–6).
- Georgiou, O., & Raza, U. (2017). Low power wide area network analysis: Can lora scale?. IEEE Wireless Communications Letters, 6(2), 162–165. https://doi.org/10.1109/LWC.5962382
- Goursaud, C., & Gorce, J. M. (2015). Dedicated networks for IoT: PHY / MAC state of the art and challenges. EAI Endorsed Transactions on Internet of Things, 1(1), 150597. https://doi.org/10.4108/eai.26-10-2015.150597
- Gradshteyn, I. S., & Ryzhik, I. M. (2007). Table of integrals, series, and products. 7th ed., Amsterdam, Elsevier/Academic Press.
- Hamdi, R., Baccour, E., Erbad, A., Qaraqe, M., & Hamdi, M. (2022). Lora-rl: deep reinforcement learning for resource management in hybrid energy lora wireless networks. IEEE Internet of Things Journal, 9(9), 6458–6476. https://doi.org/10.1109/JIOT.2021.3110996
- Hamdi, R., & Qaraqe, M. (2021). Resource management in energy harvesting powered lora wireless networks. In ICC 2021 – IEEE International Conference on Communications (pp. 1–6).
- Hossain, M. N. (2022). Performance evaluation of MIMO DFT-spread WR-OFDM system for spectrum efficiency and power efficiency. Journal of Information and Telecommunication, 6(4), 465–481. https://doi.org/10.1080/24751839.2022.2066847
- Huan, N. T., Duy, T. T., Tu, L. T., Sang, N. Q., Ta, Q. H., & Tuan, P. V. (2022). Incremental cooperation based multi-hop relaying scheme with fountain codes, wirelessly energy harvesting and partial relay selection. In 2022 International Conference on Advanced Technologies for Communications (ATC) (pp. 338–343).
- Lakshmi, P. S., & Jibukumar, M. G. (2021). A hybrid protocol for SWIPT in cooperative networks. Advances in Electrical and Electronic Engineering, 19(1). https://doi.org/10.15598/aeee.v19i1.3901
- Lu, X., Wang, P., Niyato, D., Kim, D. I., & Han, Z. (2015). Wireless networks with rf energy harvesting: A contemporary survey. IEEE Communications Surveys and Tutorials, 17(2), 757–789. https://doi.org/10.1109/COMST.9739
- Mahey, R., & Malhotra, J. (2015). Upper capacity bounds of MIMO wireless systems through fading channels. Advances in Electrical and Electronic Engineering, 13(5). https://doi.org/10.15598/aeee.v13i5.1407
- Mahmood, A., Sisinni, E., Guntupalli, L., Rondón, R., Hassan, S. A., & Gidlund, M. (2019). Scalability analysis of a lora network under imperfect orthogonality. IEEE Transactions on Industrial Informatics, 15(3), 1425–1436. https://doi.org/10.1109/TII.2018.2864681
- Mathematica. (n.d.). Online function, https://functions.wolfram.com/06.06.21.0002.01, accessed: 2023-08-13.
- Nasir, A. A., Zhou, X., Durrani, S., & Kennedy, R. A. (2013). Relaying protocols for wireless energy harvesting and information processing. IEEE Transactions on Wireless Communications, 12(7), 3622–3636. https://doi.org/10.1109/TWC.2013.062413.122042
- Ngoc, L. N., Tu, L. T., Tan, N., Nguyen, P. L., & Nguyen, Q. S. (2022). Performance on cognitive broadcasting networks employing fountain codes and maximal ratio transmission. Radioengineering, 32(1), 1–10. https://doi.org/10.13164/re.2023.0001
- Nguyen, Q. S., Nguyen, T. N., & Tu, L. T. (2023a). On the security and reliability performance of SWIPT-enabled full-duplex relaying in the non-orthogonal multiple access networks. Journal of Information and Telecommunication, 7(4), 462–476. https://doi.org/10.1080/24751839.2023.2218046
- Nguyen, T. H., Jung, W. S., Tu, L. T., Chien, T. V., Yoo, D., & Ro, S. (2020). Performance analysis and optimization of the coverage probability in dual hop lora networks with different fading channels. IEEE Access, 8, 107087–107102. https://doi.org/10.1109/Access.6287639
- Nguyen, T. N., Duy, T. T., Luu, G. T., Tran, P. T., & Voznak, M. (2017). Energy harvesting-based spectrum access with incremental cooperation, relay selection and hardware noises. Radioengineering, 26(1), 240–250. https://doi.org/10.13164/re
- Nguyen, T. N., Duy, T. T., Tran, P. T., & Voznak, M. (2016). Performance evaluation of user selection protocols in random networks with energy harvesting and hardware impairments. Advances in Electrical and Electronic Engineering, 14(4). https://doi.org/10.15598/aeee.v14i4.1783
- Nguyen, T. T., Nguyen, S. Q., Nguyen, P. X., & Kim, Y. H. (2022). Evaluation of full-duplex SWIPT cooperative NOMA-based IoT relay networks over Nakagami-m Fading channels. Sensors, 22(5), 1974. https://doi.org/10.3390/s22051974
- Nguyen, T. T. H., Vu, L. A. U., Duy, T. T., & Quang, S. N. (2023b). On the performance of potential rate and lifetime in LoRa networks. In 2023 International Conference on System Science and Engineering (ICSSE). IEEE.
- Raza, U., Kulkarni, P., & Sooriyabandara, M. (2017). Low power wide area networks: An overview. IEEE Communications Surveys and Tutorials, 19(2), 855–873. https://doi.org/10.1109/COMST.2017.2652320
- Reynders, B., Meert, W., & Pollin, S. (2017). Power and spreading factor control in low power wide area networks. In 2017 IEEE International Conference on Communications (ICC) (pp. 1–6).
- Tu, L. T., Bradai, A., Ahmed, O. B., Garg, S., Pousset, Y., & Kaddoum, G. (2022a). Energy efficiency optimization in lora networks – a deep learning approach. IEEE Transactions on Intelligent Transportation Systems, 1–13. https://doi.org/10.1109/TITS.2022.3183073
- Tu, L. T., Bradai, A., & Pousset, Y. (2020). A new closed-form expression of the coverage probability for different qos in lora networks. In ICC 2020 – 2020 IEEE International Conference on Communications (ICC) (pp. 1–6).
- Tu, L. T., Bradai, A., & Pousset, Y. (2022b). Coverage probability and spectral efficiency analysis of multi-gateway downlink lora networks. In ICC 2022 – IEEE International Conference on Communications (pp. 1–6).
- Tu, L. T., Bradai, A., Pousset, Y., & Aravanis, A. I. (2021). Energy efficiency analysis of lora networks. IEEE Wireless Communications Letters, 10(9), 1881–1885. https://doi.org/10.1109/LWC.2021.3084996
- Tu, L. T., Bradai, A., Pousset, Y., & Aravanis, A. I. (2022c). On the spectral efficiency of lora networks: Performance analysis, trends and optimal points of operation. IEEE Transactions on Communications, 70(4), 2788–2804. https://doi.org/10.1109/TCOMM.2022.3148784
- Tu, L. T., & Di Renzo, M. (2017). Analysis of millimeter wave cellular networks with simultaneous wireless information and power transfer. In 2017 International Conference on Recent Advances in Signal Processing, Telecommunications & Computing (SigTelCom) (pp. 39–43).
- Tu, L. T., & Di Renzo, M. (2020). On the energy efficiency of heterogeneous cellular networks with renewable energy sources – a stochastic geometry framework. IEEE Transactions on Wireless Communications, 19(10), 6752–6770. https://doi.org/10.1109/TWC.7693
- Tu, L. T., Di Renzo, M., & Coon, J. P. (2016). System-level analysis of receiver diversity in swipt-enabled cellular networks. Journal of Communications and Networks, 18(6), 926–937. https://doi.org/10.1109/JCN.5449605
- Tu, L. T., Nguyen, T. N., Duy, T. T., Tran, P. T., Voznak, M., & Aravanis, A. I. (2022d). Broadcasting in cognitive radio networks: A fountain codes approach. IEEE Transactions on Vehicular Technology, 71(10), 11289–11294. https://doi.org/10.1109/TVT.2022.3188969
- Tu, L. T., Nguyen, T. N., Tran, P. T., Duy, T. T., & Nguyen, Q. S. (2023a). Performance statistics of broadcasting networks with receiver diversity and fountain codes. Journal of Information and Telecommunication, 7(4), 477–493. https://doi.org/10.1080/24751839.2023.2225254
- Tu, L. T., Phan, V. D., Nguyen, T. N., Tran, P. T., Duy, T. T., Nguyen, Q. S., Nguyen, N. T., & Voznak, M. (2023b). Performance analysis of multihop full-duplex NOMA systems with imperfect interference cancellation and near-field path-loss. Sensors, 23(1), 524. https://doi.org/10.3390/s23010524
- Tu, L. T., Quoc Bao, V. N., Tien Dat, P., Kanno, A., & Kawanishi, T. (2015). 10-gb/s wireless signal transmission over a seamless im/dd fiber-mmw system at 92.5 ghz. In 2015 IEEE International Conference on Communications (ICC) (pp. 1364–1369).
- Van Chien, T., Papazafeiropoulos, A. K., Tu, L. T., Chopra, R., Chatzinotas, S., & Ottersten, B. (2021). Outage probability analysis of irs-assisted systems under spatially correlated channels. IEEE Wireless Communications Letters, 10(8), 1815–1819. https://doi.org/10.1109/LWC.2021.3082409
Appendix A.
Proof of Lemma 3.1
Let us begin the proof with the definition of the average transmit power as follows: (A1)
(A1) Here
and
are the position of the kth ED and ith power beacon,
is the Euclidean distance and random variable (RV)
refers to
. To obtain the mean of a random variable X, one first needs to obtain its cumulative distribution function (CDF) and probability density function (PDF) denoted by
and
that are given as follows:
(A2)
(A2) where
is the upper incomplete Gamma function (Gradshteyn & Ryzhik, Citation2007, (8.350.2));
is held by applying the CDF and PDF of the
while
is attained by computing the integration. The PDF of X is then derived by taking the first-order derivative with respect to (w.r.t.) to x as follows:
(A3)
(A3) The mean of X is then computed as follows:
(A4)
(A4) Here the integration related to the incomplete Gamma function is attained with the help of (CitationMathematica, Citationn.d.) and we close the proof here.
Appendix B.
Proof of Lemma 3.2
Let us begin the proof with the definition of the CCDF as follows:
(A5)
(A5) Here
is attained by employing the CCDF of the exponential distribution and the PDF of a random point inside the disk while
is held by changing variable
and the last equation is achieved by using the definition of the upper incomplete Gamma function. The CDF is defined as the complement of the CCDF and is evaluated as
(A6)
(A6) The PDF is obtained by taking the first-order derivative w.r.t y as follows:
(A7)
(A7) We complete the proof here.